Analysis of the regulation of metastasis-associated lung adenocarcinoma transcript 1 on the biological behavior of breast cancer
Introduction
Breast cancer is the most common female malignancy worldwide and the leading cause of cancer-related death in women. Previous studies suggested that the occurrence and development of breast cancer were closely related to genes, hormones, and dietary habits (1,2). With the rapid development of Genechip and high-throughput sequencing technology, the role of genes in the occurrence, development, and metastasis of tumor diseases has drawn people’s close attention. In recent years, many studies revealed that long non-coding ribonucleic acid (lncRNA) played an important role in the regulation of transcription, post-transcription, and protein expression (3). It was also found to be involved in biological processes such as cell differentiation, genomic imprinting, and epigenetic regulation (4). LncRNA is known to be involved in the occurrence, development, and metastasis of cancer (5). Metastasis-associated lung adenocarcinoma transcript 1 (MALAT1) is a highly conserved lncRNA, which is expressed in mammalian multi-tissue cells and can participate in epigenetic regulation and cell cycle regulation (6-8). In addition, many studies confirmed that MALAT1 was closely related to malignant tumors such as lung cancer, liver cancer, gastric cancer, and colorectal cancer. The high expression of MALAT1 was mostly found in tumor cells, which can promote the proliferation, metastasis, and invasion of tumor cells (9,10). Research of Zhang et al. proved that the expression of MALAT1 was correlated with the overall survival of patients after surgery (11). Studies also revealed that MALAT1 can regulate angiogenesis through the extracellular regulated protein kinase/matrix metalloprotease pathway, thereby promoting cancer metastasis (12). However, the role of MALAT1 in the pathogenesis and development of breast cancer is still relatively rare. Therefore, the abnormal expression of MALAT1 as a biological marker for breast cancer diagnosis is still to be explored.
CiteSpace is employed in this research to analyze lncRNA MALAT1 and its potential value in the diagnosis of cancer and breast cancer. Then, meta-analysis is performed to systematically evaluate the results of relevant studies, aiming to explore the correlation between MALAT1 expression and breast cancer survival rate. This study provides a new way of thinking for the follow-up study of MALAT1 in the occurrence and metastasis of breast cancer and its effect on the prognosis of patients. We present the following article in accordance with the MDAR reporting checklist (available at http://dx.doi.org/10.21037/tcr-20-3221).
Methods
CiteSpace
The most powerful feature of the CiteSpace was that the software could perform co-cited analysis. Through the citations contained in the database, the frequency of these citations was used to be cited by other documents to perform cluster analysis. Then, the most influential citations in this field could be analyzed and obtained. Finally, the front-burner issues in this research field and the corresponding research process could be comprehended (13). The analysis process using CiteSpace was shown in Figure 1.
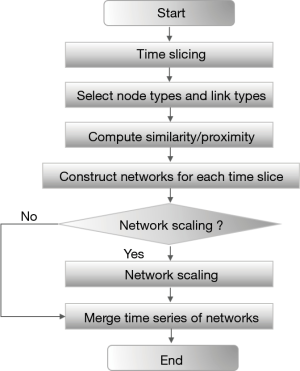
Data collection and preprocessing
The data used for CiteSpace analysis could be collected from the Web of Science (http://webofscience.com), Chinese Social Science Citation Index, and China National Knowledge Infrastructure database (14). This study adopted the data in the Web of Science database to study the regulation of MALAT1 in cancer and breast cancer.
First, the subject terms “MALAT1” and “Cancer”, and “MALAT1” and “Breast Cancer” were searched in the core database of Web of Science. Then, “Article” was selected as the document type, and time span from 1996 to 2020 was determined. Finally, a total of 1,180 articles with the subject word “MALAT1” and “Cancer”, and 215 with “MALAT1” and “Breast Cancer” were retrieved. Each record was saved as a plain text document, the record format was as follows “author, title, source publication, and abstract”, and the file name was “Download.txt”. Then, the data-import/export function in CiteSpace was taken to transform the input.
CiteSpace parameter setting
After the CiteSpace was started, Web of Science was selected and a new program in the “Projects” column was created.
(I) Time Scaling: The time span in this research was set as from 1996 to 2020, and # Years Per Slice is set to 1. (II) Text Process It needed to select “Title, Abstract, Author Keywords (DE), and Keywords Plus (ID)” in “Term Source”. “Burst Terms” in “Term Type” was selected and “Detect Bursts”. (III) Network Configuration: “Author, Institution, Country, Keywords, Cited References, and Cited Author” in “Node Types” were selected for analysis. “Top N% per slice” option in “Selection Criteria” was selected, and set as 1% and 100, respectively. The specific parameter setting was shown in Figure 2. After the parameters were set, the program was started by clicking “GO!”.
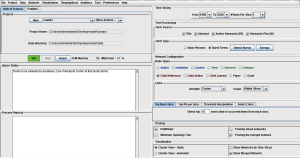
Meta-analysis
Breast cancer data sets containing MALAT1 gene expression were extracted from the GEO database (https://www.ncbi.nlm.nih.gov/gds). This study adopted the data in Affymetrix human genome U133 plus 2.0 array and U133A array. Then, a total of six data sets were finally selected, namely GSE6532, GSE9195, GSE20711, GSE31448, GSE42568, and GSE1456. Third-quantile distribution meant that the MALAT1 expression in the chip data was arranged from large to small, it was classified into low (values from 0% to 33.3%) and medium (33.3% to 66.6%) and high (66.6% to 100%). Using the study-specific third-quantile distribution as the cutoff value, the MALAT1 expression data was finally divided into 3 categories, which were “High” (MALAT1 expression > high tertile cutoff), “Mid” (low tertile cutoff ≤ MALAT1 expression < high tertile cutoff), and “Low” (MALAT1 expression ≤ low tertile cutoff).
In this study, we used R language to build a proportional hazard model and used proportional hazard regression analysis to compare “High” and “Low”. RevMan 5.3 software was employed for meta-analysis, and in the meta-analysis, a random effects model (DerSimonian and Laird method) was used to calculate the combined hazard ratio (HR) and 95% confidence interval (95% CI).
Statistical analysis
CiteSpace software was used to construct knowledge maps related to the subject terms “MALAT1” and “Cancer”, and “MALAT1” and “Breast Cancer” to understand and summarize the research trend of “MALAT1” in recent years. The differential expression of MALAT1 in the chip data was classified as “high”, “medium” and “low” according to the third-quantile distribution. The Cox proportional-hazards regression model was used to calculate HRs and 95% CI. Meta-analysis of MALAT1 expression and survival and recurrence of breast cancer patients was performed via using RevMan5.3 software. When I2>50% and P>0.05, it indicated that there was heterogeneity in the data, and the random-effects model was used for analysis. When I2<50% and P<0.05, there was no heterogeneity in the data, and the fixed-effects model was used for analysis. P<0.05 was considered significant.
Results
MALAT1 and Cancer post trend analysis
The number of articles published on the keywords “MALAT1” and “Cancer” from 1996 to 2020 was derived from the core database of Web of Science. First, the changes in the number of articles published in different years that contained the subject terms “MALAT1” and “Cancer” were compared. Figure 3 presented that the number of publications from 2008 to 2011 was relatively small, while that from 2011 to 2018 showed an increasing trend year by year. As of August 2020, the number of publications with keywords of “MALAT1” and “Cancer” was 145, accounting for 12.288% of the total number in recent years. It revealed that the role of MALAT1 gene in cancer had become the research focus of experts in related fields and the trend of future research. Then, the differences between different countries/regions in the number of publications on the keywords “MALAT1” and “Cancer” were analyzed. The collaborative articles were classified based on the information of the first author. Figure 4 showed that Chinese accounted for the largest proportion in studying “MALAT1” and “Cancer”, which was more than half of the studies in recent years, followed by the American.
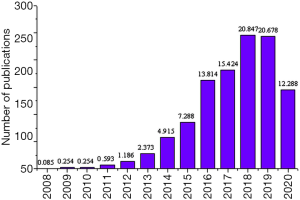
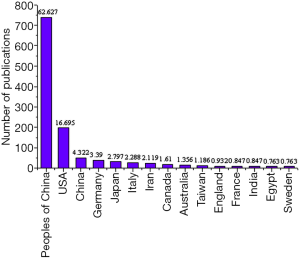
The number of publications on the keywords of “MALAT1” and “Breast Cancer” from 1996 to 2020 was derived from the core database of Web of Science. Firstly, the changes in the number of publications related to the publication of the keywords of “MALAT1” and “Breast Cancer” in different years were compared. Figure 5 showed the number of publications from 2008 to 2015 was small, while the number from 2015 to 2019 increased rapidly. By August 2020, the number of articles with the keywords of “MALAT1” and “Breast Cancer” in 2020 had reached 23, accounting for about 10.698% of the total number of articles with the keywords of “MALAT1” and “Breast Cancer” from 1996 to 2020. It indicated that the role of MALAT1 gene in breast cancer had become a front-burner issue among experts in relevant fields and the future research trend.
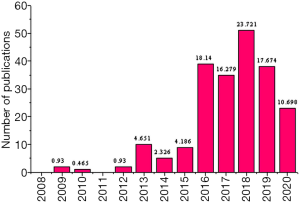
Then, the differences in the number of articles published on the keywords of “MALAT1” and “Breast Cancer” in different countries/regions were analyzed. The collaborative articles were classified based on the information of the first author. Figure 6 showed that Chinese accounted for the largest proportion in studying “MALAT1” and “Breast Cancer”, which was more than half of the studies in recent years, followed by the American.
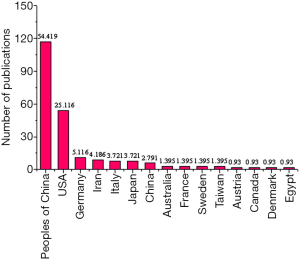
Visualization results of CiteSpace knowledge map
In CiteSpace, it set the “Top N%” and “per slice” as 1% and 100, respectively, to visualize the knowledge map of keywords. Figure 7 showed that the keywords were mainly MALAT1, expression, metastasis, and proliferation. Different keywords were closely related to each other (15).
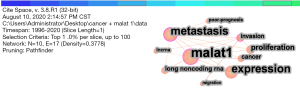
Through the calculation of high-frequency keywords, the centrality of high-frequency keywords in this field was obtained. Table 1 suggested the higher the centrality, the more studies around the keyword. The keyword “MALAT1” had the highest frequency and centrality, followed by “expression” with 0.77 centrality. The keyword “metastasis” was the 3rd. These results revealed that most studies on MALAT1 gene and cancer had focused on the expression of its lncRNA in cancer cell metastasis, proliferation, and poor prognosis of patients (16).
Table 1
Rank | Frequency | Centrality | Year | Keywords |
---|---|---|---|---|
1 | 248 | 0.96 | 2018 | MALAT1 |
2 | 209 | 0.77 | 2018 | Expression |
3 | 209 | 0.75 | 2018 | Metastasis |
4 | 140 | 0.68 | 2018 | Proliferation |
5 | 117 | 0.59 | 2018 | LncRNA |
6 | 113 | 0.57 | 2018 | Cancer |
7 | 112 | 0.53 | 2018 | Invasion |
8 | 85 | 0.46 | 2018 | Poor-prognosis |
9 | 84 | 0.44 | 2018 | LncRNA |
10 | 77 | 0.42 | 2019 | Migration |
MALAT1, metastasis associated lung adenocarcinoma transcript 1; lncRNA, long noncoding RNA. Year: the year when the keyword appears frequently.
Log-likelihood rate (LLR) is a clustering label word extraction algorithm, which can cluster according to the citing documents. The larger the cluster label word, the more representative the word is. Subsequently, through the LLR method, clustering of high-frequency keywords had been achieved using a total of three cluster labels (long, clinicopathology, and micRNA, respectively). Thus, MALAT1 gene was a type of long non-coding RNA (lncRNA), which might regulate miRNAs associated with certain diseases, and then played a role in regulating the clinicopathological changes of cancer. Moreover, the description of the three keyword cluster tags was given in Table 2. It could be known that the key words based on clustering, MALAT1, and cancer-related published key word clustering tags were mostly asthmatic diseases or clinicopathological studies.
Table 2
ID | Size | Silhouette | Mean (year) | Terms (mutual information) |
---|---|---|---|---|
0# | 5 | 0.913 | 2018 | Asthmatic |
1# | 3 | 0.532 | 2018 | Clinicopathology |
2# | 2 | 0.487 | 2018 | Clinicopathology |
MALAT1, metastasis associated lung adenocarcinoma transcript 1.
By calculating the highly cited authors, the centrality of the highly cited authors in this field could be obtained (11,17-26), as shown in Table 3.
Table 3
Rank | Frequency | Centrality | Year | Cited references |
---|---|---|---|---|
1 | 139 | 0.47 | 2003 | Ji P, 2003, Oncogene, V22, P8031, DOI 10.1038/S.J.ONC.1206928 |
2 | 110 | 0.27 | 2013 | Gutschner T, 2013, Cancer Res, V73, P1180, DOI 10.1158/0008-5472.CAN-12-2850 |
3 | 84 | 0.15 | 2013 | Gutschner T, 2013, J Mol Med (Berl), V91, P791, DOI 10.1007/S00109-013-1028-Y |
4 | 77 | 0.22 | 2010 | Tripathi V, 2010, Mol Cell, V39, P925, DOI 10.1016/J.MOLCEL.2010.08.011 |
5 | 65 | 0.95 | 2015 | Hirata H, 2015, Cancer Res, V75, P1322, DOI 10.1158/0008-5472.CAN-14-2931 |
6 | 61 | 0.17 | 2010 | Gupta RK, 2010, Nature, V464, P1071, DOI 10.1038/NATURE08975 |
7 | 52 | 0.11 | 2011 | Schmidt LH, 2011, J Thorac Oncol, V6, P1984, DOI 10.1097/JTO.0B013E3182307EAC |
8 | 51 | 1.03 | 2014 | Fan Y, 2014, Clin Cancer Res, V20, P1531, DOI 10.1158/1078-0432.CCR-13-1455 |
9 | 50 | 0.11 | 2016 | Arun G, 2016, Gene Dev, V30, P34, DOI 10.1101/GAD.270959.115 |
10 | 46 | 0.30 | 2014 | Zheng HT, 2014, Int J Clin Exp Patho, V7, P3174 |
MALAT1, metastasis associated lung adenocarcinoma transcript 1.
Then, the highly cited literature clustering was performed by the LLR method, and a total of nine clustering tags (sunitinib, regulates, tumorigenesis, characterization, migration, metastasis-associated, transition, suppressor, and mir-9) were obtained in Figure 8. The clustering tags of the highly cited publications focused on the characterization and regulation of MALAT1 gene in tumors (27). It was suggested that MALAT1 was closely related to the progression of cancer and might interact with the regulation of cancer through sunitinib or mir-9 gene. Since this study was based on big data, analysis software was adopted to obtain assumptions, experiments were still needed for verifying.
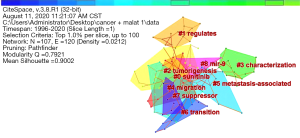
Through the calculation of high-frequency keywords, the centrality of high-frequency keywords in this field was obtained. Table 4 showed that the keyword “MALAT1” had the highest frequency and the highest centrality.
Table 4
Rank | Frequency | Centrality | Year | Keywords |
---|---|---|---|---|
1 | 84 | 1.00 | 2016 | MALAT1 |
2 | 62 | 0.86 | 2018 | Expression |
3 | 59 | 0.75 | 2016 | Metastasis |
4 | 59 | 0.75 | 2017 | Breast-cancer |
MALAT1, metastasis associated lung adenocarcinoma transcript 1. Year: the year when the keyword appears frequently.
Visualization of knowledge map of highly cited articles was performed, and the centrality of highly cited articles in this field was calculated (17-21,24,25,28,29), as shown in Table 5. The articles of the author Ji P [2003] had the highest citation frequency, followed by those published by Gutschner T [2013]. The Centrality of Tripathi V [2010], Arun G [2016], and Yang LQ [2011] was lower than 0.1, and the Centrality of other high-frequency citation was no less than 0.1.
Table 5
Rank | Frequency | Centrality | Year | Cited references |
---|---|---|---|---|
1 | 64 | 0.55 | 2003 | Ji P, 2003, Oncogene, V22, P8031, DOI 10.1038/S.J.ONC.1206928 |
2 | 54 | 0.43 | 2013 | Gutschner T, 2013, Cancer Res, V73, P1180, DOI 10.1158/0008-5472.CAN-12-2850 |
3 | 41 | 0.07 | 2010 | Tripathi V, 2010, Mol Cell, V39, P925, DOI 10.1016/J.MOLCEL.2010.08.011 |
4 | 35 | 0.27 | 2010 | Gupta RK, 2010, Nature, V464, P1071, DOI 10.1038/NATURE08975 |
5 | 32 | 0.32 | 2013 | Gutschner T, 2013, J Mol Med (Berl), V91, P791, DOI 10.1007/S00109-013-1028-Y |
6 | 30 | 0.35 | 2015 | Hirata H, 2015, Cancer Res, V75, P1322, DOI 10.1158/0008-5472.CAN-14-2931 |
7 | 30 | 0.01 | 2016 | Arun G, 2016, Gene Dev, V30, P34, DOI 10.1101/GAD.270959.115 |
8 | 26 | 0.10 | 2014 | Fan Y, 2014, Clin Cancer Res, V20, P1531, DOI 10.1158/1078-0432.CCR-13-1455 |
9 | 25 | 0.02 | 2011 | Yang LQ, 2011, Cell, V147, P773, DOI 10.1016/J.CELL.2011.08.054 |
10 | 23 | 0.08 | 2009 | Ponting CP, 2009, Cell, V136, P629, DOI 10.1016/J.CELL.2009.02.006 |
MALAT1, metastasis associated lung adenocarcinoma transcript 1.
Then, through the clustering of highly cited publications by the LLR method, 5 clustering tags (breast, long, PI3K/AKT/mTOR, coding, and -coding) were obtained. Figure 9 revealed that the clustering tags of highly cited publications were concentrated in MALAT1 gene, breast, and PI3K/AKT/mTOR signaling pathway. It was suggested that MALAT1 might regulate breast cancer through PI3K/AKT/mTOR signaling pathway (30).
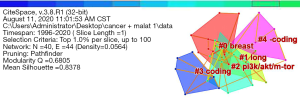
Results of meta-analysis
A meta-analysis of the association between MALAT1 expression and breast cancer survival was explored based on the analysis results of 6 GEO datasets. Meta-analysis results in Figure 10 showed that high expression of MALAT1 was significantly correlated with relapse-free survival (HR: 1.51, 95% CI: 0.79–2.29, Figure 10A), while there was no significant correlation between MALAT1 expression and overall survival (HR: 1.09, 95% CI: 0.63–1.72), as shown in Figure 10B.
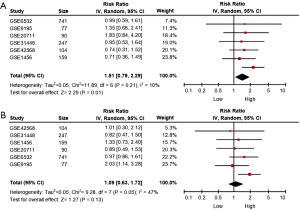
Discussion
Studies have revealed that elevated MALAT1 expression levels are closely related to the prognosis of breast cancer patients. Zheng et al. [2019] found that the expression of MALAT1 in breast cancer tissues increased significantly, and the overall survival rate was negatively correlated with the expression of MALAT1, indicating that MALAT1 was a potential prognostic factor (31). Sun et al. [2020] detected a significant increase in the expression level of MALAT1 in the serum of breast cancer patients (32). Then, they found that the MALAT1 level of patients was significantly reduced after treatment, and that the MALAT1 level of patients with good prognosis was significantly lower than that of patients with poor prognosis. In our study, MALAT1 expression data from the GEO database were used, and the results showed that high MALAT1 expression was significantly associated with relapse-free survival, but not with overall survival. This conclusion is slightly different from previous studies and may be caused by the selection and sample size of MALAT1 data in GEO database. This association may remain significant after adjustment for age at surgery, disease stage, tumor grade, and hormone receptor status due to the complexity of breast cancer staging and typing. Totally, it could be inferred that the increase of MALAT1 expression level could lead to poor prognosis of breast cancer patients, and then increased the mortality rate. Unfortunately, there has been no report on the role of MALAT1 in male breast cancer. The reason for this is that the incidence of male breast cancer is much lower than in women. As a result, in the actual research process, the isolated tumor samples of male breast cancer are difficult to obtain and is relatively of small number. Besides, the research value is far lower than that of female breast cancer. However, it is possible from existing studies that MALAT1 may also be a pro-tumor regulator in male breast cancer. Of course, this question is worth exploring further.
Conclusions
The core data of Web of Science were taken to collect the literature related to MALAT1, cancer, and breast cancer in recent years. CiteSpace was adopted to visualize the correlation of different keywords. The results revealed that the lncRNA expression level of MALAT1 was closely related to cancer progression and prognosis of cancer patients. Moreover, it was also found that the expression of MALAT1 was closely related to the survival rate of recurrence-free breast cancer. However, it only adopted the existing literature for visual analysis, and subsequent experiments needed to be done to verify this conclusion. We hope that the results can provide evidence for lncRNA MALAT1 as a biological marker for diagnosis and treatment of breast cancer.
Acknowledgments
Funding: This work was supported by
Footnote
Reporting Checklist: The authors have completed the MDAR reporting checklist. Available at http://dx.doi.org/10.21037/tcr-20-3221
Peer Review File: Available at http://dx.doi.org/10.21037/tcr-20-3221
Conflicts of Interest: All authors have completed the ICMJE uniform disclosure form (available at http://dx.doi.org/10.21037/tcr-20-3221). The authors have no conflicts of interest to declare.
Ethical Statement: The authors are accountable for all aspects of the work in ensuring that questions related to the accuracy or integrity of any part of the work are appropriately investigated and resolved.
Open Access Statement: This is an Open Access article distributed in accordance with the Creative Commons Attribution-NonCommercial-NoDerivs 4.0 International License (CC BY-NC-ND 4.0), which permits the non-commercial replication and distribution of the article with the strict proviso that no changes or edits are made and the original work is properly cited (including links to both the formal publication through the relevant DOI and the license). See: https://creativecommons.org/licenses/by-nc-nd/4.0/.
References
- Prowell TM, Beaver JA, Pazdur R. Neoadjuvant or adjuvant chemotherapy in early breast cancer? J Pathol 2019;247:612-5.
- Egger SS, Bachmann A, Hubmann N, et al. Prevalence of potentially inappropriate medication use in elderly patients: comparison between general medical and geriatric wards. Drugs Aging 2006;23:823-37. [Crossref] [PubMed]
- Jathar S, Kumar V, Srivastava J, et al. Technological Developments in lncRNA Biology. Adv Exp Med Biol 2017;1008:283-323. [Crossref] [PubMed]
- Kwong JC, Schwartz KL, Campitelli MA, et al. Acute Myocardial Infarction after Laboratory-Confirmed Influenza Infection. N Engl J Med 2018;378:345-53. [Crossref] [PubMed]
- Assefa AT, De Paepe K, Everaert C, et al. Differential gene expression analysis tools exhibit substandard performance for long non-coding RNA-sequencing data. Genome Biol 2018;19:96. [Crossref] [PubMed]
- Dai W, Tian Y, Jiang B, et al. Down-regulation of long non-coding RNA AFAP1-AS1 inhibits tumor growth, promotes apoptosis and decreases metastasis in thyroid cancer. Biomed Pharmacother 2018;99:191-7. [Crossref] [PubMed]
- Yang H, Wang S, Kang YJ, et al. Long non-coding RNA SNHG1 predicts a poor prognosis and promotes colon cancer tumorigenesis. Oncol Rep 2018;40:261-71. [Crossref] [PubMed]
- Shu J, Li S, Chen YB, et al. Long non-coding RNA EPB41L4A-AS2 inhibited non-small cell lung cancer proliferation, invasion and promoted cell apoptosis. Neoplasma 2018;65:664-72. [Crossref] [PubMed]
- Jin Y, Feng SJ, Qiu S, et al. LncRNA MALAT1 promotes proliferation and metastasis in epithelial ovarian cancer via the PI3K-AKT pathway. Eur Rev Med Pharmacol Sci 2017;21:3176-84. [PubMed]
- Xiao Y, Pan J, Geng Q, et al. LncRNA MALAT1 increases the stemness of gastric cancer cells via enhancing SOX2 mRNA stability. FEBS Open Bio 2019;9:1212-22. [Crossref] [PubMed]
- Zhang HM, Yang FQ, Chen SJ, et al. Upregulation of long non-coding RNA MALAT1 correlates with tumor progression and poor prognosis in clear cell renal cell carcinoma. Tumour Biol 2015;36:2947-55. [Crossref] [PubMed]
- Lei L, Zeng Q, Lu J, et al. MALAT1 participates in ultraviolet B-induced photo-aging via regulation of the ERK/MAPK signaling pathway. Mol Med Rep 2017;15:3977-82. [Crossref] [PubMed]
- Liu GF. Visualization of patents and papers in terahertz technology: a comparative study. Scientometrics 2013;94:1037-56. [Crossref]
- Peterson AE, Bond GR, Drake RE, et al. Predicting the Long-Term Sustainability of Evidence-Based Practices in Mental Health Care: An 8-Year Longitudinal Analysis. J Behav Health Serv Res 2014;41:337. [Crossref] [PubMed]
- Lin Q, Guan W, Ren W, et al. MALAT1 affects ovarian cancer cell behavior and patient survival. Oncol Rep 2018;39:2644-52. [Crossref] [PubMed]
- Stone JK, Kim JH, Vukadin L, et al. Hypoxia induces cancer cell-specific chromatin interactions and increases MALAT1 expression in breast cancer cells. J Biol Chem 2019;294:11213-24. [Crossref] [PubMed]
- Ji P, Diederichs S, Wang W, et al. MALAT-1, a novel noncoding RNA, and thymosin beta4 predict metastasis and survival in early-stage non-small cell lung cancer. Oncogene 2003;22:8031-41. [Crossref] [PubMed]
- Gutschner T, Hämmerle M, Diederichs S. MALAT1--a paradigm for long noncoding RNA function in cancer. J Mol Med (Berl) 2013;91:791-801. [Crossref] [PubMed]
- Gutschner T, Hämmerle M, Eissmann M, et al. The noncoding RNA MALAT1 is a critical regulator of the metastasis phenotype of lung cancer cells. Cancer Res 2013;73:1180-9. [Crossref] [PubMed]
- Tripathi V, Ellis JD, Shen Z, et al. The nuclear-retained noncoding RNA MALAT1 regulates alternative splicing by modulating SR splicing factor phosphorylation. Mol Cell 2010;39:925-38. [Crossref] [PubMed]
- Hirata H, Hinoda Y, Shahryari V, et al. Long noncoding RNA MALAT1 promotes aggressive renal cell carcinoma through Ezh2 and interacts with miR-205. Cancer Res 2015;75:1322-31. [Crossref] [PubMed]
- Gupta RK, Arany Z, Seale P, et al. Transcriptional control of preadipocyte determination by Zfp423. Nature 2010;464:619-23. [Crossref] [PubMed]
- Schmidt LH, Spieker T, Koschmieder S, et al. The long noncoding MALAT-1 RNA indicates a poor prognosis in non-small cell lung cancer and induces migration and tumor growth. J Thorac Oncol 2011;6:1984-92. [Crossref] [PubMed]
- Fan Y, Shen B, Tan M, et al. TGF-β–Induced Upregulation of malat1 Promotes Bladder Cancer Metastasis by Associating with suz12. Clin Cancer Res 2014;20:1531-41. [Crossref] [PubMed]
- Arun G, Diermeier S, Akerman M, et al. Differentiation of mammary tumors and reduction in metastasis upon Malat1 lncRNA loss. Genes Dev 2016;30:34-51. [Crossref] [PubMed]
- Zheng HT, Shi DB, Wang YW, et al. High expression of lncRNA MALAT1 suggests a biomarker of poor prognosis in colorectal cancer. Int J Clin Exp Pathol 2014;7:3174-81. [PubMed]
- Kim J, Piao HL, Kim BJ, et al. Long noncoding RNA MALAT1 suppresses breast cancer metastasis. Nat Genet 2018;50:1705-15. [Crossref] [PubMed]
- Yang L, Lin C, Liu W, et al. ncRNA- and Pc2 methylation-dependent gene relocation between nuclear structures mediates gene activation programs. Cell 2011;147:773-88. [Crossref] [PubMed]
- Ponting CP, Oliver PL, Reik W. Evolution and functions of long noncoding RNAs. Cell 2009;136:629-41. [Crossref] [PubMed]
- Xu S, Sui S, Zhang J, et al. Downregulation of long noncoding RNA MALAT1 induces epithelial-to-mesenchymal transition via the PI3K-AKT pathway in breast cancer. Int J Clin Exp Pathol 2015;8:4881-91. [PubMed]
- Zheng L, Zhang Y, Fu Y, et al. Long non-coding RNA MALAT1 regulates BLCAP mRNA expression through binding to miR-339-5p and promotes poor prognosis in breast cancer. Biosci Rep 2019;39:BSR20181284. [Crossref] [PubMed]
- Sun Z, Liu J, Liu J. The expression of lncRNA-MALAT1 in breast cancer patients and its influences on prognosis. Cell Mol Biol 2020;66:72-8. [Crossref] [PubMed]