The role of NLRP3 in the prognosis and immune infiltrates of skin cutaneous melanoma (SKCM)
Introduction
Skin cutaneous melanoma (SKCM) is a major public health problem worldwide, the mortality of SKCM patients is still increasing in many countries (1), it causes 55,500 deaths annually (2). Therefore, early detection is very important, and the detection of early prognostic markers is important for SKCM patients. Recently, numerous studies have shown that the dysfunction of the immune system plays a key role in the progression of SKCM (3,4). It has been demonstrated that tumor-infiltrating lymphocyte grade is an independent predictor of sentinel lymph node status and survival in SKCM patients (5). Therefore, immunotherapy is popularly used for treating melanoma. HD IL-2 was the first systemic immunotherapy approved for patients with advanced melanoma but efficacy was observed in a select number of patients (6). Thus, it is still necessary to explore novel immune-related therapeutic targets in SKCM.
The NLRP3 inflammasome consists of NLRP3, ASC, and caspase-1. NLRP3 is a key role of inflammation in the development and progression of various cancers. However, its exact role in tumorigenesis is unclear, recent studies have shown that the excessive expression of NLRP3 and structural activation could lead to a variety of cancer occurrence and development, including malignant melanoma, lung cancer, and breast cancer. In an independent study, Melvin et al. showed that NLRP3 is an important suppressor of natural killer cell-mediated control of metastases and carcinogenesis (7). However, the precise prognostic relevance of immune infiltration in cutaneous melanoma remained unclear.
Thus, we downloaded the data from The Cancer Genome Atlas (TCGA). Meanwhile, the correlation between NLRP3 and the prognosis of SKCM was determined by Gene Expression Profiling Interactive Analysis (GEPIA) and COX regression analysis. Additionally, CIBERSORT was used to detect the relative proportions of different types of tumor-infiltrating immune cells (TIICs) to investigate the relationship between NLRP3 and tumor-infiltrating immune cells in SKCM (8). The findings of this study help us shed light on a potential correlation as well as a possible mechanism between NLRP3 and tumor-immune interactions. Thus, NLRP3 had the potential to become a novel predictor to evaluate prognosis and immune infiltration for SKCM patients.
We present the following article in accordance with the MDAR reporting checklist (available at http://dx.doi.org/10.21037/tcr-20-3135).
Methods
Data download and preprocessing
Gene expression profile and clinical information of SKCM patients, including 471 tumor samples and 1 normal sample, were acquired from the Cancer Genome Atlas (TCGA) (https://tcga-data.nci.nih.gov/tcga/), which serves as a public repository for archiving high-throughput microarray experimental data (9). Subsequent processing excluded cases with insufficient or missing data on age, overall survival time, local invasion, lymph node metastasis, distant metastasis, and TNM stage. Finally, 322 cases with eligible clinical information were devoted to Cox regression analysis. The study was conducted in accordance with the Declaration of Helsinki (as revised in 2013).
NLRP3 expression and survival analysis of SKCM by GEPIA
The analysis of NLRP3 expression from the TCGA database was conducted with the GEPIA website (http://gepia.cancer-pku.cn/). GEPIA is a public platform for analyzing the RNA sequencing expression data of 9,736 tumors and 8,587 normal samples from the TCGA and the GTEx projects (10). We obtained samples from TCGA and used GEPIA to analyze the correlation between overall survival and NLRP3 expression in SKCM.
Immune infiltrates analysis
TIMER is a comprehensive resource for systematic analysis of immune infiltrates for diverse cancer types (https://cistrome.shinyapps.io/timer/) (11). To approximate TIICs abundance, the TIMER database used TCGA data on 10,897 samples from 32 types of cancer. We evaluated NLRP3 expression in SKCM and its correlation with the abundance of TIICs, including B cells, CD4+ T cells, CD4+ T cells, macrophages, neutrophils, and Dendritic cells by gene modules. TIMER produced a graph showing the effect of gene expression level on tumor purity (12).
Analysis of the relative abundance of TIICs
CIBERSORT (http://cibersort.stanford.edu/) (13) is an online analytical platform that has been widely accepted and it is used to analyze the relevance of correlation between gene expression and TIICs in tumor. This analysis can be used to characterize the heterogeneity of cells according to the gene expression profile of complex tissues (14). Additionally, the results have been shown to be highly consistent with the basic truth estimates in various cancers (8). Based on the gene annotation matrix of 22 immune cell subtypes provided by the CIBERSORT web platform, we calculated the P-value of each sample according to the deconvolution algorithm. P<0.05 were considered statistically significant (15). Thus, it can be further analyzed. To assess the effect of NLRP3 expression, we used 471 samples from TCGA and divided them into NLRP3 high and low expression groups, and then used these data to make a violin diagram by R.
Gene set enrichment analysis (GSEA)
GSEA is commonly used to assess whether a particular gene set is significantly different in any two biological states. In this study, GSEA was used to analyze the differential signaling pathways of the activation of NLRP3 low and high expression groups in SKCM patients. A sequence listing was subsequently generated based on the correlation of all genes with NLRP3 expression and it was performed 1,000 times in this analysis. Thus, we use standardized enrichment scores to classify each phenotypic enrichment pathway. Absolute values of FDR <0.05 or P<0.05 were statistically significant.
The Human Protein Atlas
The Human Protein Atlas (https://www.proteinatlas.org/) (16,17) has both mRNA and protein expression data on 44 different human tissues. The antibody-based protein profiling shows the protein expression level and location. Additionally, its protein expression score is based on immunohistochemical data manually scored with regard to staining intensity (negative, weak, moderate, or strong) and the fraction of stained cells (<25%, 25–75%, or >75%). Thus, we used the intensity of the staining to evaluate the expression of the NLRP3 protein in skin tissues and SKCM.
Statistical analysis
Statistical data obtained by TCGA was combined by R3.6.3. Logistic regression was used to analyze the correlation between clinical data and NLRP3 expression. In addition, multivariate Cox analysis was used to assess the influence of NLRP3 expression and other pathological and clinical factors (age, gender, lymph node, distant metastasis, tumor status, and stage) on OS. P value <0.05 was set up as the cut-off criterion. Moreover, to detect the correlation between 22 types of immune cells, we made the correlation heatmap, a chart of the correlation between every two different immune cells in samples.
Results
Patient characteristics and multivariate analysis
NLRP3 expression was significantly higher in the SKCM compared to normal tissues (Log2FC <1, P<0.01) (Figure 1A). Through GEPIA, we found that the reduced expression of NLRP3 was significantly related to low overall survival (P=0.0014) (Figure 1B) and advanced pathological stage (P=0.00213) (Figure 1C). As shown in Table 1, univariate analysis using Cox regression revealed that some factors, including age (HR =1.02, P=0.000), pathological stage (HR =1.57, P=0.000), tumor status (HR =1.57, P=0.000) and lymph node status (HR =1.59, P=0.000) were significantly associated with overall survival. In multivariate analysis (Table 1, Figure 1D), the worse tumor status and less lymph node metastasis were independent prognostic factors with good prognosis. Thus, up-regulated NLRP3 expression (HR =0.86, P=0.188) cannot be an independent prognostic factor.
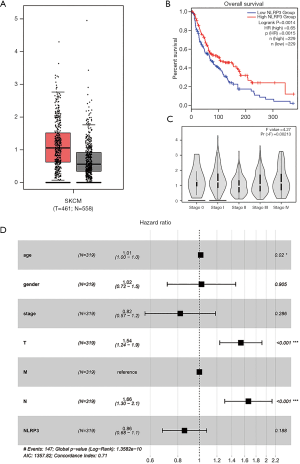
Table 1
Parameter | Univariate analysis | Multivariate analysis | |||||
---|---|---|---|---|---|---|---|
HR | 95% CI | P | HR | 95% CI | P | ||
Age | 1.02 | 1.01−1.03 | 0.000 | 1.01 | 1.00−1.03 | 0.020 | |
Gender | 1.05 | 0.74−1.48 | 0.794 | 1.02 | 0.72−1.46 | 0.905 | |
Stage | 1.57 | 1.29−1.91 | 0.000 | 0.82 | 0.57−1.18 | 0.286 | |
T classification | 1.57 | 1.32−1.87 | 0.000 | 1.54 | 1.24−1.91 | 0.000 | |
N classification | 1.59 | 1.35−1.86 | 0.000 | 1.66 | 1.30−2.13 | 0.000 | |
NLRP3 | 0.83 | 0.66−1.04 | 0.106 | 0.86 | 0.68−1.08 | 0.188 |
NLRP3 expression is associated with SKCM level of immune infiltration and cumulative survival
Multiple studies reported high lymphocytic infiltration to be associated with better patient survival outcomes. Therefore, we attempted to investigate whether the expression of NLRP3 was associated with immune infiltration of SKCM. We assessed the correlation between NLRP3 expression and the level of immune invasion by TIMER. What’s more, our results showed that NLRP3 expression was associated with a better prognosis and higher immune infiltration of SKCM. Moreover, the expression level of NLRP3 was negatively correlated with the infiltration level of B cells (r=0.235, P=4.65e-07), CD8+ T cells (r=0.488, P=1.53e-27), CD4+ T cells (r=0.337, P=2.43e-13), Macrophages (r=0.573, P=5.79e-41), Neutrophils (r=0.672, P=9.40e-61), and DCs (r=0.637, P=4.16e-52) in SKCM (Figure 2A). The B cell, CD8+ T cells, Neutrophils and DCs were related factors to the cumulative survival rate of SKCM over time (Figure 2B). These results suggested that NLRP3 plays a key role in SKCM immune infiltration. Additionally, our findings strongly support the significant role of NLRP3 in immune infiltration.
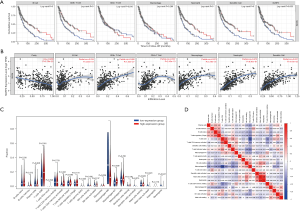
Difference in the proportion of immune infiltrating cells
We filtered out samples with P<0.05 from the results of 22 immune cell components in each sample that was obtained, and we divided the high and low expression groups based on the median NLRP3 expression. Then we used CIBERSORT to explore gene expression profiles of downloaded samples to infer the density of 22 types of immune cells. The CIBERSORT algorithm applied to the 22 immune cell subtypes helped assess differences in their expression levels in the high and low NLRP3 expression groups (Figure 2C). Results showed that CD8+ T cells and Macrophages M0 were the main immune cells affected by NLRP3 expression. Additionally, we assessed possible correlations between 22 types of immune cells (Figure 2D). As shown in Figure 2D, the correlation heatmap reflected a higher correlation within the proportions of different TIIC subgroups. Positive correlations were shown in red, whereas negative correlations were shown in blue. As in the above analysis, CD8+ T cells and Macrophages M0 were negatively correlated (−0.65). By contrast, Neutrophils and Mast cells activated presented a significant positive correlation (0.78).
GSEA investigation of NLRP3
GSEA revealed significant differences (FDR <0.05 or P<0.05) in enrichment of GO terms and KEGG pathways in samples with high levels of NLRP3. We explored the potential biological functions of NLRP3 through GO Term and KEGG Pathway analysis. As shown in Table 2, KEGG pathway analysis showed eight pathways that had the strongest positive correlation with NLRP3 expression: calcium signaling pathway, glioma, NON-small cell lung cancer, pancreatic cancer, pathways in cancer, small cell lung cancer, T cell receptor signaling pathway and Toll-like receptor signaling pathway. The four pathways with the strongest negative correlation were oxidative phosphorylation, Parkinsons disease, Huntingtons disease, and RNA polymerase (Figure 3A). GO annotation showed eight categories that were positively correlated with high levels of NLRP3: external side of plasma membrane, interleukin 6 production, negative regulation of immune system process, regulation of inflammatory response, regulation of leukocyte mediated immunity, regulation of T cell activation, T cell activation involved in immune response and tumor necrosis factor superfamily cytokine production. GO analysis also uncovered five negatively correlated categories: melanin metabolic process, mitochondrial gene expression, mitochondrial respiratory chain complex assembly, mitochondrial translation, and oxidative phosphorylation (Figure 3B). All these results indicated the potential role of NLRP3 in the development of SKCM.
Table 2
Gene set name | NES | NOM p-val | FDR q-val |
---|---|---|---|
High expression | |||
KEGG_CALCIUM_SIGNALING_PATHWAY | 2.1069617 | 0 | 4.88E-04 |
KEGG_GLIOMA | 2.0191584 | 0 | 0.001337478 |
KEGG_NON_SMALL_CELL_LUNG_CANCER | 2.0142043 | 0 | 0.001321252 |
KEGG_PANCREATIC_CANCER | 2.2465017 | 0 | 0 |
KEGG_PATHWAYS_IN_CANCER | 2.2282844 | 0 | 4.77E-05 |
KEGG_SMALL_CELL_LUNG_CANCER | 1.9993234 | 0 | 0.001549201 |
KEGG_T_CELL_RECEPTOR_SIGNALING_PATHWAY | 2.3618245 | 0 | 0 |
KEGG_TOLL_LIKE_RECEPTOR_SIGNALING_PATHWAY | 2.4893427 | 0 | 0 |
GO_EXTERNAL_SIDE_OF_PLASMA_MEMBRANE | 2.6949873 | 0 | 0 |
GO_INTERLEUKIN_6_PRODUCTION | 2.7184777 | 0 | 0 |
GO_NEGATIVE_REGULATION_OF_IMMUNE_SYSTEM_PROCESS | 2.7642787 | 0 | 0 |
GO_REGULATION_OF_INFLAMMATORY_RESPONSE | 2.7052493 | 0 | 0 |
GO_REGULATION_OF_LEUKOCYTE_MEDIATED_IMMUNITY | 2.6305406 | 0 | 0 |
GO_REGULATION_OF_T_CELL_ACTIVATION | 2.6855686 | 0 | 0 |
GO_T_CELL_ACTIVATION_INVOLVED_IN_IMMUNE_RESPONSE | 2.5701654 | 0 | 0 |
GO_TUMOR_NECROSIS_FACTOR_SUPERFAMILY_CYTOKINE_PRODUCTION | 2.78174 | 0 | 0 |
Low expression | |||
KEGG_OXIDATIVE_PHOSPHORYLATION | −2.192871 | 0 | 3.50E-04 |
KEGG_PARKINSONS_DISEASE | −2.0404372 | 0 | 0.003454411 |
KEGG_HUNTINGTONS_DISEASE | −2.0444586 | 0 | 0.005181618 |
KEGG_RNA_POLYMERASE | −1.9101619 | 0.007677543 | 0.008911205 |
GO_MELANIN_METABOLIC_PROCESS | −2.1573868 | 0 | 6.30E-04 |
GO_MITOCHONDRIAL_GENE_EXPRESSION | −2.1840587 | 0 | 4.39E-04 |
GO_MITOCHONDRIAL_RESPIRATORY_CHAIN_COMPLEX_ASSEMBLY | −2.2938614 | 0 | 2.08E-04 |
GO_MITOCHONDRIAL_TRANSLATION | −2.2089524 | 0 | 3.10E-04 |
GO_OXIDATIVE_PHOSPHORYLATION | −2.2029662 | 0 | 3.33E-04 |
NES, normalized enrichment score; NOM, nominal; FDR, false discovery rate.
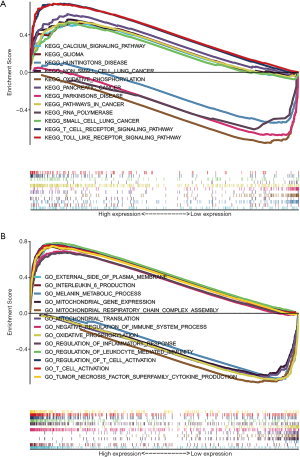
Immunohistochemical staining
Using the Human Protein Atlas database (https://www.proteinatlas.org), we explored the IHC staining of NLRP3 in skin tissues and skin cutaneous melanoma tissues. Immunohistochemistry analysis available from the HPA showed that in SKCM, NLRP3 has higher levels of expression compared to normal skin tissues (Figure 4).
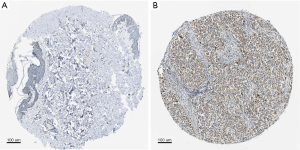
Discussion
SKCM is a highly aggressive tumor with apparent lethality, although the 5-year relative survival rate for early-stage melanoma is more than 95%, the reported survival for stage IV melanoma is rarely longer than a year (18). Recently developed immunotherapy has been applied for multiple cancers, including SKCM, and demonstrated its superiority in some context (19). However, extensive prognostic heterogeneity among SKCM patients requires individualized treatment options, thus requiring effective prognostic stratification. There was new data suggesting that NLRP3 inflammasome polymorphisms are related to different malignancies such as colon cancer and melanoma (20). The precise clinical function of NLRP3 in the role of the initiation and promotion of differing neoplasms also highlights the therapeutic potential of inflammasomes, and as prognostic markers. Inflammation and persistent infection may contribute to various human malignancies (21,22). Evidence has accrued on the role that inflammation has in cancer initiation, development, progression, angiogenesis, and invasion (23,24). Moreover, inflammation may induce an immune response involving T cells, B cells, NK cells, DC, macrophages, and neutrophils (25,26). In this study, we demonstrated for the first time the regulatory potential in immune microenvironment and prognostic significance of the NLRP3 complex as a whole in SKCM.
Additionally, we found high expression of NLRP3 predicted good prognosis and the low expression of NLRP3 affected the clinical characteristics of tumor pathological stage, lymph node metastasis status, and primary tumor status. Moreover, we found that different immune markers and different levels of immune cell infiltration were associated with differences in NLRP3 expression in SKCM. Thus, to further investigate the possible mechanism of NLRP3 expression in SKCM and its relationship to clinical characteristics, we downloaded the current data from the public database TCGA. Then we used CIBERSORT to detect the relative proportions of multiple tumor-infiltrating immune cells (TIIC) in the tumor microenvironment of SKCM patients. These findings may help us to further investigate the application value of NLRP3 in SKCM, and reveal the potential correlation and possible mechanism of NLRP3 expression and immune interaction in tumor microenvironment. Therefore, NLRP3 may be a new indicator to evaluate the prognosis and immune infiltration of SKCM patients.
The NLRP3 inflammasome comprises the sensor molecule NLRP3, the adaptor protein ASC, and pro-caspase 1 (27). Pan et al. (28) found that TP had no influence on NLRP3 or ASC oligomerization, but that it could inhibit NLRP3 and ASC expression, as well as NLRP3-ASC interactions, to inhibit inflammasome assembly. Melanoma research demonstrated that the development of cancer cells was inhibited by reduced inflammasome and IL-1β expression (29). Recent studies suggested that the upregulation of NLRP3 inflammasome may aggravate inflammatory responses in skin neoplasms. Mice models with NLRP3, caspase-1, and ASC adaptor deficiencies show protection against cancer progression (30,31). Others have reported that NLRP1 can activate caspase 2 and 9 in neoplasm cells resulting in tumorigenesis, but NLRP3 did not appear to be tumorigenic (32). In our study, it’s worth noting that the expression of NLRP3 in SKCM correlated with the level of immune infiltration. CIBERSORT analysis suggested that the expression of NLRP3 had a significant effect on the infiltration levels of B cells, neutrophils, and T cells in the SKCM tumor microenvironment. Compared with the low expression group, the macrophage M0 of the high expression group was decreased. CD8+ T cells are key effectors of tumor cell destruction in a variety of cancers, its high proportion of infiltration correlates well with a good clinical prognosis (33,34). The presence of T cells in the tumor parenchyma is a characteristic of immunoinvasive tumors, highlighting the antitumor effect of antitumor antigen T cells in the immunosuppressive microenvironment (35,36). Increasing evidence indicated that defective T cells migrate into tumors, which is one of the drug resistance mechanisms of immunotherapy (37,38). In summary, our study found that the expression of NLRP3 may affect the composition ratio of B, T cells, and macrophages in the immune microenvironment of SKCM tumor tissue, thus indirectly regulating immune monitoring and affecting tumor progression.
What’s more, we explored the expression of NLRP3 that is associated with many known cancer processes and immune response pathways. It included calcium signaling pathway, glioma, NON-small cell lung cancer, pancreatic cancer, pathways in cancer, small cell lung cancer, T cell receptor signaling pathway, and Toll-like receptor signaling pathway. All these results suggested that NLRP3 may be used as a prognostic and therapeutic target not only in SKCM but also in many cancers.
Conclusions
In conclusion, although the relationship between NLRP3 and SKCM has not been explained in detail, based on our results and previous studies on NLRP3, it was reasonable to believe that NLRP3 played a key role in the regulation and recruitment of SKCM immune-infiltrated cells. It will influence the development of the pathophysiological mechanisms of SKCM, especially the development of immune infiltration. We strongly recommended that further research be conducted on this topic to gradually refine the evidence for the biological effects of NLRP3. In conclusion, NLRP3 was a prognostic biomarker with potential application prospects, related to immune infiltration of SKCM, and maybe a new target for regulating immunosuppression.
Acknowledgments
Thanks to Jonathan Eagle for the language modifications to this article.
Funding: This study was supported by
Footnote
Reporting Checklist: The authors have completed the MDAR reporting checklist. Available at http://dx.doi.org/10.21037/tcr-20-3135
Conflicts of Interest: All authors have completed the ICMJE uniform disclosure form (available at http://dx.doi.org/10.21037/tcr-20-3135). The authors have no conflicts of interest to declare.
Ethical Statement: The authors are accountable for all aspects of the work in ensuring that questions related to the accuracy or integrity of any part of the work are appropriately investigated and resolved. The study was conducted in accordance with the Declaration of Helsinki (as revised in 2013). All data sets were retrieved from both published literature and publicly available data sets. Methods and results are linked to the data set. No written authority is required.
Open Access Statement: This is an Open Access article distributed in accordance with the Creative Commons Attribution-NonCommercial-NoDerivs 4.0 International License (CC BY-NC-ND 4.0), which permits the non-commercial replication and distribution of the article with the strict proviso that no changes or edits are made and the original work is properly cited (including links to both the formal publication through the relevant DOI and the license). See: https://creativecommons.org/licenses/by-nc-nd/4.0/.
References
- Thompson JF, Scolyer RA, Kefford RF. Cutaneous melanoma in the era of molecular profiling. Lancet 2009;374:362-5. [Crossref] [PubMed]
- Schadendorf D, van Akkooi ACJ, Berking C, et al. Melanoma. Lancet 2018;392:971-84. [Crossref] [PubMed]
- Bogunovic D, O'Neill DW, Belitskaya-Levy I, et al. Immune profile and mitotic index of metastatic melanoma lesions enhance clinical staging in predicting patient survival. Proc Natl Acad Sci U S A 2009;106:20429-34. [Crossref] [PubMed]
- Selitsky SR, Mose LE, Smith CC, et al. Prognostic value of B cells in cutaneous melanoma. Genome Med 2019;11:36. [Crossref] [PubMed]
- Azimi F, Scolyer RA, Rumcheva P, et al. Tumor-infiltrating lymphocyte grade is an independent predictor of sentinel lymph node status and survival in patients with cutaneous melanoma. J Clin Oncol 2012;30:2678-83. [Crossref] [PubMed]
- Clark JI, Singh J, Ernstoff MS, et al. A multi-center phase II study of high dose interleukin-2 sequenced with vemurafenib in patients with BRAF-V600 mutation positive metastatic melanoma. J Immunother Cancer 2018;6:76. [Crossref] [PubMed]
- Melvin JC, Holmberg L, Rohrmann S, et al. Serum lipid profiles and cancer risk in the context of obesity: four meta-analyses. J Cancer Epidemiol 2013;2013:823849. [Crossref] [PubMed]
- Newman AM, Liu CL, Green MR, et al. Robust enumeration of cell subsets from tissue expression profiles. Nat Methods 2015;12:453-7. [Crossref] [PubMed]
- Blum A, Wang P, Zenklusen JC. SnapShot: TCGA-Analyzed Tumors. Cell 2018;173:530. [Crossref] [PubMed]
- Tang Z, Li C, Kang B, et al. GEPIA: a web server for cancer and normal gene expression profiling and interactive analyses. Nucleic Acids Res 2017;45:W98-W102. [Crossref] [PubMed]
- Li T, Fan J, Wang B, et al. TIMER: A Web Server for Comprehensive Analysis of Tumor-Infiltrating Immune Cells. Cancer Res 2017;77:e108-10. [Crossref] [PubMed]
- Aran D, Sirota M, Butte AJ. Systematic pan-cancer analysis of tumour purity. Nat Commun 2015;6:8971. [Crossref] [PubMed]
- Xiong TF, Pan FQ, Liang Q, et al. Prognostic value of the expression of chemokines and their receptors in regional lymph nodes of melanoma patients. J Cell Mol Med 2020;24:3407-18. [Crossref] [PubMed]
- Liu X, Wu S, Yang Y, et al. The prognostic landscape of tumor-infiltrating immune cell and immunomodulators in lung cancer. Biomed Pharmacother 2017;95:55-61. [Crossref] [PubMed]
- Goletzke J, Herder C, Joslowski G, et al. Habitually higher dietary glycemic index during puberty is prospectively related to increased risk markers of type 2 diabetes in younger adulthood. Diabetes Care 2013;36:1870-6. [Crossref] [PubMed]
- Pontén F, Schwenk JM, Asplund A, et al. The Human Protein Atlas as a proteomic resource for biomarker discovery. J Intern Med 2011;270:428-46. [Crossref] [PubMed]
- Uhlen M, Oksvold P, Fagerberg L, et al. Towards a knowledge-based Human Protein Atlas. Nat Biotechnol 2010;28:1248-50. [Crossref] [PubMed]
- Fecher LA, Cummings SD, Keefe MJ, et al. Toward a molecular classification of melanoma. J Clin Oncol 2007;25:1606-20. [Crossref] [PubMed]
- Franklin C, Livingstone E, Roesch A, et al. Immunotherapy in melanoma: Recent advances and future directions. Eur J Surg Oncol 2017;43:604-11. [Crossref] [PubMed]
- Karki R, Man SM, Kanneganti TD. Inflammasomes and Cancer. Cancer Immunol Res 2017;5:94-9. [Crossref] [PubMed]
- Grivennikov SI, Greten FR, Karin M. Immunity, inflammation, and cancer. Cell 2010;140:883-99. [Crossref] [PubMed]
- Hussain SP, Harris CC. Inflammation and cancer: an ancient link with novel potentials. Int J Cancer 2007;121:2373-80. [Crossref] [PubMed]
- Hanahan D, Weinberg RA. Hallmarks of cancer: the next generation. Cell 2011;144:646-74. [Crossref] [PubMed]
- McAllister SS, Weinberg RA. Tumor-host interactions: a far-reaching relationship. J Clin Oncol 2010;28:4022-8. [Crossref] [PubMed]
- Berraondo P, Minute L, Ajona D, et al. Innate immune mediators in cancer: between defense and resistance. Immunol Rev 2016;274:290-306. [Crossref] [PubMed]
- de Visser KE, Eichten A, Coussens LM. Paradoxical roles of the immune system during cancer development. Nat Rev Cancer 2006;6:24-37. [Crossref] [PubMed]
- Fu C, Zhang X, Zeng Z, et al. Neuroprotective Effects of Qingnao Dripping Pills Against Cerebral Ischemia via Inhibiting NLRP3 Inflammasome Signaling Pathway: In Vivo and In Vitro. Front Pharmacol 2020;11:65. [Crossref] [PubMed]
- Pan XC, Liu Y, Cen YY, et al. Dual Role of Triptolide in Interrupting the NLRP3 Inflammasome Pathway to Attenuate Cardiac Fibrosis. Int J Mol Sci 2019;20:360. [Crossref] [PubMed]
- Dunn JH, Ellis LZ, Fujita M. Inflammasomes as molecular mediators of inflammation and cancer: potential role in melanoma. Cancer Lett 2012;314:24-33. [Crossref] [PubMed]
- Drexler SK, Bonsignore L, Masin M, et al. Tissue-specific opposing functions of the inflammasome adaptor ASC in the regulation of epithelial skin carcinogenesis. Proc Natl Acad Sci U S A 2012;109:18384-9. [Crossref] [PubMed]
- Guarda G, Zenger M, Yazdi AS, et al. Differential expression of NLRP3 among hematopoietic cells. J Immunol 2011;186:2529-34. [Crossref] [PubMed]
- Zhai Z, Liu W, Kaur M, et al. NLRP1 promotes tumor growth by enhancing inflammasome activation and suppressing apoptosis in metastatic melanoma. Oncogene 2017;36:3820-30. [Crossref] [PubMed]
- Joyce JA, Fearon DT. T cell exclusion, immune privilege, and the tumor microenvironment. Science 2015;348:74-80. [Crossref] [PubMed]
- Sato E, Olson SH, Ahn J, et al. Intraepithelial CD8+ tumor-infiltrating lymphocytes and a high CD8+/regulatory T cell ratio are associated with favorable prognosis in ovarian cancer. Proc Natl Acad Sci U S A 2005;102:18538-43. [Crossref] [PubMed]
- Trujillo JA, Sweis RF, Bao R, et al. T Cell-Inflamed versus Non-T Cell-Inflamed Tumors: A Conceptual Framework for Cancer Immunotherapy Drug Development and Combination Therapy Selection. Cancer Immunol Res 2018;6:990-1000. [Crossref] [PubMed]
- Woo SR, Corrales L, Gajewski TF. The STING pathway and the T cell-inflamed tumor microenvironment. Trends Immunol 2015;36:250-6. [Crossref] [PubMed]
- Anderson KG, Stromnes IM, Greenberg PD. Obstacles Posed by the Tumor Microenvironment to T cell Activity: A Case for Synergistic Therapies. Cancer Cell 2017;31:311-25. [Crossref] [PubMed]
- Chen DS, Mellman I. Elements of cancer immunity and the cancer-immune set point. Nature 2017;541:321-30. [Crossref] [PubMed]