Using immune-related lncRNAs to construct novel biomarkers and investigate the immune landscape of breast cancer
Introduction
Breast cancer (BRCA) is the most common type of cancer in females worldwide. BRCA is highly heterogeneous, and there are significant differences in etiology, pathological manifestations, and the prognosis of individuals (1). Despite the developments of diagnostic methods and therapeutic regimens, the outcome of BRCA is still unsatisfactory, especially for progressed patients (2). Apart from being highly heterogeneous, the main reasons for this outcome may include metastasis, therapy resistance, and immune inhibition (3-5). Therefore, it is urgent to further understand the underlying mechanisms of these processes in BRCA, which may unveil potential therapeutic methods for advanced and refractory BRCA patients to lengthen their lifespan.
Long noncoding RNAs (lncRNAs) are a type of RNA which are characterized by more than 200 nucleotides in length, but do not code proteins. Recently, the dysregulation of lncRNAs has been observed in multiple cancer types, including colorectal cancer, lung cancer, and bladder cancer (6-8). Furthermore, lncRNAs act as important regulators of the progression of various cancers types (6,9,10). For BRCA, Liang et al.’s study indicated that lncRNA BCRT1 can promote BRCA progression via inhibiting the miR-1303/PTBP3 axis (11). Shi et al. demonstrated that lncRNA DILA1 can induce tamoxifen resistance via decreasing cyclin D1 degradation (12). However, Kim et al.’s study showed that lncRNA MALAT1 can inhibit BRCA metastasis (13). From these findings, we can conclude that lncRNAs may have dual roles in cancer processes.
To date, a number of studies have proven that the tumor microenvironment (TME) plays a significant role in BRCA progression (14-16). Apart from immune cells and stromal cells of the TME, several regulators such as lncRNAs have essential roles in the TME (17). Xu et al. showed that lncRNA SATB2-AS1 could regulate the tumor metastasis in colorectal cancer (18). LncRNA NEAT1 accelerates the progression of aggressive endometrial cancer by miR-361-regulated networks and TME-related genes (19). From these studies, lncRNAs may directly or indirectly affect the TME to regulate tumor processes. LncRNAs that regulate immune cell infiltration have been called immune-related lncRNAs (irlncRNAs). Several studies have proven that some irlncRNAs can be observed in BRCA patient samples such as lncRNA OSTN-AS1 and lncRNA TCL6 (20,21), and both of them affect the TME via regulating immune cell infiltration. Additionally, lncRNA TCL6 led to poor outcomes of BRCA patients (21), but the relationship between immune cell infiltration, lncRNAs, and drug resistance was not investigated. Compared with these studies, we explored the association between irlncRNAs and immune cell infiltration. We also constructed a novel modeling algorithm, estimated the predictive value, and explored drug sensitivity, as well as diagnostic sensitivity and specificity for BRCA patients.
We present the following article in accordance with the REMARK reporting checklist (available at https://dx.doi.org/10.21037/tcr-21-783).
Methods
Raw data
Transcriptome data and clinical data of 1,109 BRCA samples and 113 normal samples were extracted from The Cancer Genome Atlas (TCGA, https://portal.gdc.cancer.gov/). An immune-related gene list was collected from the Immport website (https://www.immport.org/). Immune cell abundance of the TCGA-BRCA cohort was downloaded from the Tumor IMmune Estimation Resource database (TIMER, https://cistrome.shinyapps.io/timer/).
Obtaining irlncRNAs
We extracted the lncRNAs of the TCGA-BRCA cohort from the RNAseq expression matrix, and analyzed the correlation between immune genes and lncRNAs. The threshold values of correlation analysis were set as coefficient R >0.4 and P value <0.001 (Spearman).
Screening differentially expressed immune-related lncRNAs (irlncRNAs)
The differences in irlncRNAs were analyzed (tumor vs. normal) via the limma package in R software (https://www.r-project.org/). The filtering standards were log-fold change |logFC| >0.5 and false discovery rate (FDR) <0.05. The ggplot2 package was used to generate the heatmap plot and volcano plot.
Construction of immune-related lncRNA pairs (IRLPs)
The differentially expressed IRLPs were paired one by one. In each IRLP, the expression of the former lncRNA was higher than that of the latter lncRNA, then we recorded the expression of this IRLP as 1, or otherwise recorded as 0.
Construction of a prognostic signature for IRLPs
Firstly, we performed univariate cox regression on all IRLPs (screening P value <0.001), then we used lasso regression to obtain a more refined signature by constructing a penalty function. Finally, multivariate cox regression (stepwise method) was used to construct a prognostic signature based on the screening genes of lasso regression. The Kaplan-Meier (KM) method was used to generate the survival curve. The maximum Youden index (sensitivity + specificity-1) of the 1-year receiver operating characteristic (ROC) was identified as the best cutoff value based on the R packages survivalROC and survminer. A nomogram and calibration curve of the prognosis signature were constructed via the R package rms. We only included patients with complete survival data for signature construction. P<0.05 was considered statistically significant.
Correlation analysis between the risk score and immune cells
The correlation between the risk score of the prognostic signature and immune cells was analyzed based on the TCGA-BRCA cohort obtained from the TIMER database, and the correlation bubble chart between the risk score and immune cells was generated via ggplot2, ggtext, and scales packages (Spearman). P<0.05 was considered statistically significant.
Analysis of risk score and drug sensitivity
The R package pRRophetic is based on gene expression and drug sensitivity data from a large number of cancer cell lines, and these models are applied to gene expression data from primary tumor biopsies (22). We analyzed the relationship between the risk score of the signature and drug sensitivity based on the pRRophetic package. P<0.05 was considered statistically significant.
Statistical analysis
The differential irlncRNAs were screened through the R package limma (https://www.r-project.org/), and the thresholds were set as |logFC| >1 and FDR <0.05. The KM plot was used to assess the differential survival between the different groups, and log-rank P<0.05 was considered statistically significant. Univariate and multivariate (stepwise) cox regression were used to construct the prognostic signature. The Wilcoxon test and Spearman correlation were used for difference analysis and correlation analysis, respectively. P<0.05 was considered statistically significant.
Ethical statement
The study was conducted in accordance with the Declaration of Helsinki (as revised in 2013).
Results
Results of IRLPs
We extracted 13,413 lncRNAs from the TCGA-BRCA cohort to analyze the correlation with immune genes, than we obtained 420 irlncRNAs. The heatmap plot demonstrates the top 50 up-regulated and down-regulated genes (Figure 1A). Differential expression analysis indicated that there were 130 up-regulated lncRNAs and 45 down-regulated lncRNAs (Figure 1B). Subsequently, we paired 175 immune-related differentially expressed lncRNAs and obtained a total of 10,084 IRLPs.
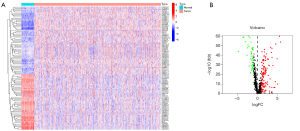
Prognostic signature results of IRLPs
Univariate cox regression results showed that 33 IRLPs had significant effects on the overall survival (OS) of BRCA patients. The results of lasso regression indicated that λ =−4.7 was the optimal value, and 22 IRLPs were obtained for further analysis (Figure 2A,B). Multivariate regression analysis obtained 12 lncRNA pairs to establish the IRLP prognostic signature (Figure 2C).
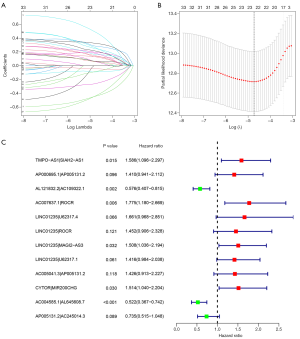
Optimal cutoff value determination and KM survival analysis of the IRLP signature
The 1-year ROC curve of the IRLP signature showed that the area under the curve (AUC) value was 0.832 and the optimal cutoff value of the risk score was 1.912 (Figure 3A). The AUC values of 1-, 3-, and 5-year ROC curves of the signature were 0.832, 0.803, and 0.794, respectively (Figure 3B). The risk score curve and survival status map showed that according to the optimal cutoff value, 1,090 BRCA patients were divided into a high-risk group (n=277) and low-risk group (n=813) (Figure 3C,D). KM survival analysis indicated that patients with low risk had a better OS for BRCA (log-rank P<0.001) (Figure 3E).
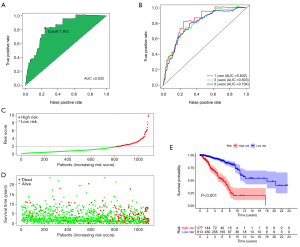
Relationship between signature risk scores and clinical features
The summary heatmap of correlation analysis between risk scores and clinical features (Figure 4A). The results demonstrated that risk scores had no significant correlation with age (Figure 4B); and a higher risk scores were correlated with higher stage (P<0.001, Figure 4C), tumor (T) size (P<0.001, Figure 4D), and node (N) staging (P<0.05, Figure 4E) of BRCA patients. Nevertheless, risk scores had no significant correlated with distant metastasis (Figure 4F).
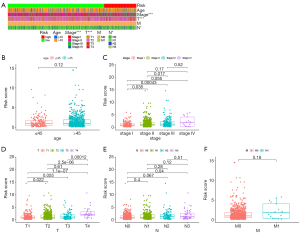
Prognostic analysis of signature risk scores and clinical features
Univariate and multivariate cox regression analyses of risk scores and clinical features indicated that risk scores were independent prognostic factors of clinical features (P<0.001, Figure 5A,B). Compared with other clinical features of BRCA, the 1-year AUC value of the ROC curve of risk scores was 0.832, which was higher than T (0.729), N (0.654), M (0.580), stage (0.732), and age (0.794) features (Figure 5C). Risk scores were better prognostic factors for BRCA.
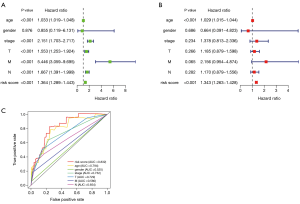
Relationship between risk scores and immune cells in BRCA
We downloaded the results of 7 types of software which contained various kinds of immune cells in BRCA from the TIMER database, and analyzed the correlation between risk scores and immune cells. The bubble chart demonstrated the correlation analysis results of significant differences between risk scores and immune cells (Figure 6).
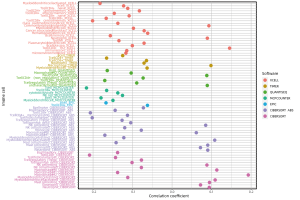
Based on the optimal cutoff value, risk scores were divided into high- and low-risk groups, and the relationship between high and low risk and M1 macrophages and M2 macrophages was analyzed. The results showed that the M1 macrophage content in BRCA was higher in the low-risk group (P=0.00015, Figure 7A), and M2 macrophage content in BRCA was higher in the high-risk group (P=0.0015, Figure 7B).
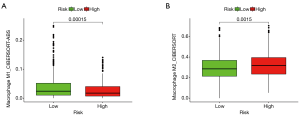
Results of risk scores and drug sensitivity analysis
We analyzed the half maximal inhibitory concentration (IC50) of some drugs which are commonly used as chemotherapeutic agents in BRCA based on the R package pRRophetic. The results indicated that doxorubicin (P=0.0011, Figure 8A), cisplatin (P=0.032, Figure 8B), docetaxel (P=0.061, Figure 8C), and gemcitabine (P=2e-07, Figure 8D) had lower IC50 values in BRCA patients with low risk, suggesting that these drugs have better efficacy in the low-risk group.
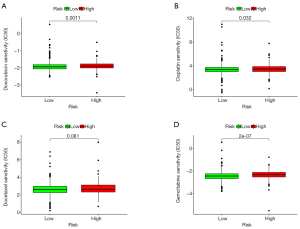
Discussion
With the better understanding of the association between lncRNAs and the immune system (23,24), more and more evidence indicates that lncRNAs can not only act as diagnostic biomarkers, but also prognostic signatures for cancer patients. Several studies have focused on using coding genes and noncoding RNAs to construct models to assess the prognostic and diagnostic values for BRCA (25-27). Most of the signatures in these studies were determined via quantifying transcriptional expression levels. In the present study, instead of using transcriptional expression levels, we used immune-related gene pairing to generate a reliable model with lncRNA pairs.
We downloaded transcriptome and clinical data of BRCA patients from TCGA, obtained immune genes from the Immport database, and extracted immune genes and lncRNAs for correlation analysis. Then, the differential expression of IRLPs was determined and the prognostic signature was established by the IRLPs. The immune cell abundance of the TCGA-BRCA cohort was downloaded from the TIMER database to analyze the relationship between the risk score of the IRLP signature and immune cell abundance. Finally, we explored the relationship between risk scores and drug sensitivity based on the R package pRRophetic. Shen et al. used the transcriptional expression levels of 11 irlncRNAs as a biomarker for predicting the prognosis of BRCA patients (28). It is now known that lncRNAs are the most abundant non-coding RNAs, with a number of significant biological functions. Our study indicated that we could select significant IRLPs from the initial identification differentially expressed irlncRNAs (DEirlncRNA). We could detect significant differential expression of IRLPs but not every lncRNA. Clinicians can take advantage of this novel model to divide patients into low or high risk for BRCA. Since these lncRNAs are related to immune-related genes, they may have a deep influence on the TME of BRCA. In our study, we found that the IRLPs TMPO−AS1|SIAH2−AS1, AP000695.1|AP005131.2, AL121832.2|AC109322.1, AC007637.1|ROCR, LINC01235|U62317.4, LINC01235|ROCR, LINC01235|MAGI2−AS3, LINC01235|U62317.1, AC005041.3|AP005131.2, CYTOR|MIR200CHG, AC004585.1|AL645608.7, and AP005131.2|AC245014.3 had significant roles in the prognosis of BRCA patients via univariate analysis. We further performed multivariate analysis which showed that TMPO−AS1|SIAH2−AS1, AL121832.2|AC109322.1, AC007637.1|ROCR, LINC01235|MAGI2−AS3, CYTOR|MIR200CHG, and AC004585.1|AL645608.7 had essential roles in the prognosis of BRCA patients. Several studies have proven that lncRNA TMPO−AS1 is a cancer promoter for BRCA (29,30). Additionally, lncRNA TMPO−AS1 plays a key role in ovarian cancer and bladder cancer (31,32). MAGI2−AS3 is a tumor suppressor for BRCA, and Du et al. showed that MAGI2−AS3 inhibited the migration and invasion of BRCA cells by sponging microRNA-374a (33). Liu et al. showed that CYTOR can enhance the tamoxifen resistance of BRCA cells through sponging miR-125a-5p (34). Besides having a significant effect on BRCA patients, Moradi et al. showed that CYTOR also acted as a diagnostic biomarker (35). Furthermore, a review by Liang et al. showed that CYTOR was an adverse signature for multiple cancer types (36). Other irlncRNAs have not been investigated in regards to their biological functions in cancer.
We further used lasso regressionto increase the predictive model accuracy and efficacy. We also improved the procedure of modeling by calculating every AUC value to identify the maximum value for an optimal model followed by comparison with other clinical parameters. The AUC values were also used to achieve an optimal point for model fitting instead of distinguishing the risk just by the median value. Apart from exploring the relationship between risk scores and the prognosis of BRCA patients, we also reanalyzed the outcome of BRCA patients based on clinical features such as stage, age, and gender via univariate and multivariate analyses. We also analyzed the relationship between immune cell infiltration and risk scores via multiple software. The final goal of cancer research is always to find novel potential therapeutic regimens for cancer patients, so we further investigated the drug sensitivity of BRCA. The results showed that DEirlncRNA pairs were more positively related to tumor-infiltrating immune cells, and have a positive correlation with common lymphoid progenitors, neutrophils, CD4+ Th2 T cells, macrophages, activated NK cells, macrophage M0 infiltration, but a negative correlation with CD4+ effector memory T cells, CD4+ central memory T cells, CD4+ naive T cells, B cells, and activated myeloid dendritic cells, among others. From the various immune cells of the TME for BRCA, our past study showed that macrophages play a key role in BRCA progression (37). In the present study, we mainly focused on the role of macrophage cell types in BRCA. The results showed that the high-risk group had higher M2 macrophage infiltration than the low-risk group, while the low-risk group had higher M1 macrophage infiltration. These results indicated that M2 macrophages may act as suppressors of BRCA while M1 macrophages may act as promoters of BRCA. Chen et al. showed that M2 macrophages promoted BRCA metastasis by the M2 macrophage-produced CHI3L1 protein (38). Sousa et al. revealed that BRCA cells can induce macrophages toward M2 polarization (39). These results indicate that BRCA can adjust the macrophage status to affect the tumor process. Li et al. showed that promoting M1 macrophages could inhibit the growth of BRCA cells via activating the NF-κB pathway (40). However, Guo et al. showed that M1 macrophages can induce BRCA stem cells by regulation of the Lin-28B-let-7-HMGA2 axis (41). These findings indicate that the roles of macrophages may be adjusted according to different TMEs. Wang et al. indicated chemotherapy and immunotherapy therapy responses of cancer via immune scores. Furthermore, a number of studies have shown that the TME has a significant influence on therapy response (42-44). In our study, our model showed that the high-risk group was related to sensitivity to chemotherapeutic regimens such as cisplatin, docetaxel, doxorubicin, and gemcitabine. Our results recommend clinicians to select chemotherapeutic regimens based on risk scores to obtain better therapeutic responses.
Conclusions
In conclusion, the present study showed that a novel signature constructed by irlncRNAs, that did not require an analysis of lncRNA expression levels, could assess the prognosis of BRCA patients and help clinicians to select chemotherapeutic regimens based on risk scores.
Acknowledgments
Funding: None.
Footnote
Reporting Checklist: The authors have completed the REMARK reporting checklist. Available at https://dx.doi.org/10.21037/tcr-21-783
Conflicts of Interest: All authors have completed the ICMJE uniform disclosure form (available at https://dx.doi.org/10.21037/tcr-21-783). The authors have no conflicts of interest to declare.
Ethical Statement: The authors are accountable for all aspects of the work in ensuring that questions related to the accuracy or integrity of any part of the work are appropriately investigated and resolved. The study was conducted in accordance with the Declaration of Helsinki (as revised in 2013).
Open Access Statement: This is an Open Access article distributed in accordance with the Creative Commons Attribution-NonCommercial-NoDerivs 4.0 International License (CC BY-NC-ND 4.0), which permits the non-commercial replication and distribution of the article with the strict proviso that no changes or edits are made and the original work is properly cited (including links to both the formal publication through the relevant DOI and the license). See: https://creativecommons.org/licenses/by-nc-nd/4.0/.
References
- Harbeck N, Penault-Llorca F, Cortes J, et al. Breast cancer. Nat Rev Dis Primers 2019;5:66. [Crossref] [PubMed]
- Saad ED, Katz A, Buyse M. Overall survival and post-progression survival in advanced breast cancer: a review of recent randomized clinical trials. J Clin Oncol 2010;28:1958-62. [Crossref] [PubMed]
- Duan J, Wang Y, Jiao S. Checkpoint blockade-based immunotherapy in the context of tumor microenvironment: Opportunities and challenges. Cancer Med 2018;7:4517-29. [Crossref] [PubMed]
- Osborne CK. Tamoxifen in the treatment of breast cancer. N Engl J Med 1998;339:1609-18. [Crossref] [PubMed]
- Peart O. Metastatic Breast Cancer. Radiol Technol 2017;88:519M-539M. [PubMed]
- Cao HL, Liu ZJ, Huang PL, et al. lncRNA-RMRP promotes proliferation, migration and invasion of bladder cancer via miR-206. Eur Rev Med Pharmacol Sci 2019;23:1012-21. [PubMed]
- Wang L, Cho KB, Li Y, et al. Long Noncoding RNA (lncRNA)-Mediated Competing Endogenous RNA Networks Provide Novel Potential Biomarkers and Therapeutic Targets for Colorectal Cancer. Int J Mol Sci 2019;20:5758. [Crossref] [PubMed]
- Zhang YX, Yuan J, Gao ZM, et al. LncRNA TUC338 promotes invasion of lung cancer by activating MAPK pathway. Eur Rev Med Pharmacol Sci 2018;22:443-9. [PubMed]
- Li J, Meng H, Bai Y, et al. Regulation of lncRNA and Its Role in Cancer Metastasis. Oncol Res 2016;23:205-17. [Crossref] [PubMed]
- Rajagopal T, Talluri S, Akshaya RL, et al. HOTAIR LncRNA: A novel oncogenic propellant in human cancer. Clin Chim Acta 2020;503:1-18. [Crossref] [PubMed]
- Liang Y, Song X, Li Y, et al. LncRNA BCRT1 promotes breast cancer progression by targeting miR-1303/PTBP3 axis. Mol Cancer 2020;19:85. [Crossref] [PubMed]
- Shi Q, Li Y, Li S, et al. LncRNA DILA1 inhibits Cyclin D1 degradation and contributes to tamoxifen resistance in breast cancer. Nat Commun 2020;11:5513. [Crossref] [PubMed]
- Kim J, Piao HL, Kim BJ, et al. Long noncoding RNA MALAT1 suppresses breast cancer metastasis. Nat Genet 2018;50:1705-15. [Crossref] [PubMed]
- Deepak KGK, Vempati R, Nagaraju GP, et al. Tumor microenvironment: Challenges and opportunities in targeting metastasis of triple negative breast cancer. Pharmacol Res 2020;153:104683. [Crossref] [PubMed]
- Deshmukh SK, Srivastava SK, Poosarla T, et al. Inflammation, immunosuppressive microenvironment and breast cancer: opportunities for cancer prevention and therapy. Ann Transl Med 2019;7:593. [Crossref] [PubMed]
- Monteith RS, Carron JM, Warren CW, et al. Contraceptive use and fertility in Paraguay, 1987. Stud Fam Plann 1988;19:284-91. [Crossref] [PubMed]
- Botti G, Scognamiglio G, Aquino G, et al. LncRNA HOTAIR in Tumor Microenvironment: What Role? Int J Mol Sci 2019;20:2279. [Crossref] [PubMed]
- Xu M, Xu X, Pan B, et al. LncRNA SATB2-AS1 inhibits tumor metastasis and affects the tumor immune cell microenvironment in colorectal cancer by regulating SATB2. Mol Cancer 2019;18:135. [Crossref] [PubMed]
- Dong P, Xiong Y, Yue J, et al. Long noncoding RNA NEAT1 drives aggressive endometrial cancer progression via miR-361-regulated networks involving STAT3 and tumor microenvironment-related genes. J Exp Clin Cancer Res 2019;38:295. [Crossref] [PubMed]
- Liu Z, Mi M, Li X, et al. lncRNA OSTN-AS1 May Represent a Novel Immune-Related Prognostic Marker for Triple-Negative Breast Cancer Based on Integrated Analysis of a ceRNA Network. Front Genet 2019;10:850. [Crossref] [PubMed]
- Zhang Y, Li Z, Chen M, et al. lncRNA TCL6 correlates with immune cell infiltration and indicates worse survival in breast cancer. Breast Cancer 2020;27:573-85. [Crossref] [PubMed]
- Geeleher P, Cox N, Huang RS. pRRophetic: an R package for prediction of clinical chemotherapeutic response from tumor gene expression levels. PLoS One 2014;9:e107468. [Crossref] [PubMed]
- Li B, Geng R, Wu Q, et al. Alterations in Immune-Related Genes as Potential Marker of Prognosis in Breast Cancer. Front Oncol 2020;10:333. [Crossref] [PubMed]
- Shafiee A, McGovern JA, Lahr CA, et al. Immune system augmentation via humanization using stem/progenitor cells and bioengineering in a breast cancer model study. Int J Cancer 2018;143:1470-82. [Crossref] [PubMed]
- Chang JT, Wang F, Chapin W, et al. Identification of MicroRNAs as Breast Cancer Prognosis Markers through the Cancer Genome Atlas. PLoS One 2016;11:e0168284. [Crossref] [PubMed]
- Wang J, Zhang G, Sui Y, et al. CD52 Is a Prognostic Biomarker and Associated With Tumor Microenvironment in Breast Cancer. Front Genet 2020;11:578002. [Crossref] [PubMed]
- Xiao B, Zhang W, Chen L, et al. Analysis of the miRNA-mRNA-lncRNA network in human estrogen receptor-positive and estrogen receptor-negative breast cancer based on TCGA data. Gene 2018;658:28-35. [Crossref] [PubMed]
- Shen Y, Peng X, Shen C. Identification and validation of immune-related lncRNA prognostic signature for breast cancer. Genomics 2020;112:2640-6. [Crossref] [PubMed]
- Mitobe Y, Ikeda K, Sato W, et al. Proliferation-associated long noncoding RNA, TMPO-AS1, is a potential therapeutic target for triple-negative breast cancer. Cancer Sci 2020;111:2440-50. [Crossref] [PubMed]
- Mitobe Y, Ikeda K, Suzuki T, et al. ESR1-Stabilizing Long Noncoding RNA TMPO-AS1 Promotes Hormone-Refractory Breast Cancer Progression. Mol Cell Biol 2019;39:e00261-19. [Crossref] [PubMed]
- He YC, Bi YG, Jiang L. LncRNA TMPO-AS1 promotes proliferation and migration in bladder cancer. Eur Rev Med Pharmacol Sci 2020;24:8740-6. [PubMed]
- Zhao H, Ding F, Zheng G. LncRNA TMPO-AS1 promotes LCN2 transcriptional activity and exerts oncogenic functions in ovarian cancer. FASEB J 2020;34:11382-94. [Crossref] [PubMed]
- Du S, Hu W, Zhao Y, et al. Long non-coding RNA MAGI2-AS3 inhibits breast cancer cell migration and invasion via sponging microRNA-374a. Cancer Biomark 2019;24:269-77. [Crossref] [PubMed]
- Liu Y, Li M, Yu H, et al. lncRNA CYTOR promotes tamoxifen resistance in breast cancer cells via sponging miR125a5p. Int J Mol Med 2020;45:497-509. [PubMed]
- Moradi MT, Hatami R, Rahimi Z. Circulating CYTOR as a Potential Biomarker in Breast Cancer. Int J Mol Cell Med 2020;9:83-90. [PubMed]
- Liang J, Wei X, Liu Z, et al. Long noncoding RNA CYTOR in cancer: A TCGA data review. Clin Chim Acta 2018;483:227-33. [Crossref] [PubMed]
- Ma YF, Chen Y, Fang D, et al. The immune-related gene CD52 is a favorable biomarker for breast cancer prognosis. Gland Surg 2021;10:780-98. [Crossref] [PubMed]
- Chen Y, Zhang S, Wang Q, et al. Tumor-recruited M2 macrophages promote gastric and breast cancer metastasis via M2 macrophage-secreted CHI3L1 protein. J Hematol Oncol 2017;10:36. [Crossref] [PubMed]
- Sousa S, Brion R, Lintunen M, et al. Human breast cancer cells educate macrophages toward the M2 activation status. Breast Cancer Res 2015;17:101. [Crossref] [PubMed]
- Li J, Cai H, Sun H, et al. Extracts of Cordyceps sinensis inhibit breast cancer growth through promoting M1 macrophage polarization via NF-kappaB pathway activation. J Ethnopharmacol 2020;260:112969. [Crossref] [PubMed]
- Guo L, Cheng X, Chen H, et al. Induction of breast cancer stem cells by M1 macrophages through Lin-28B-let-7-HMGA2 axis. Cancer Lett 2019;452:213-25. [Crossref] [PubMed]
- Hirata E, Sahai E. Tumor Microenvironment and Differential Responses to Therapy. Cold Spring Harb Perspect Med 2017;7:a026781. [Crossref] [PubMed]
- Jiang Y, Xie J, Huang W, et al. Tumor Immune Microenvironment and Chemosensitivity Signature for Predicting Response to Chemotherapy in Gastric Cancer. Cancer Immunol Res 2019;7:2065-73. [Crossref] [PubMed]
- Wu T, Dai Y. Tumor microenvironment and therapeutic response. Cancer Lett 2017;387:61-8. [Crossref] [PubMed]
(English Language Editor: C. Betlazar-Maseh)