Development of a novel autophagy-related gene prognostic signature for gastric cancer
Introduction
Gastric cancer (GC) is one of the most common types of cancer and a leading cause of cancer-related deaths worldwide (1). Despite the advances in early diagnosis, surgical resection and adjuvant chemotherapy in the last decade, GC is still notorious for dismal prognosis due to its significant heterogeneity and predisposition for invasion and distant metastasis. For advanced and metastatic GC, even though new therapeutic approaches, such as targeted therapies are currently being researched, clinical trials have been largely disappointing (2,3). Therefore, it is important to further elucidate the molecular pathogenesis involved in GC in order to identify novel prognostic indicators and potential therapeutic strategies.
Autophagy, a highly conserved multi-step catabolic process, can not only promote cell survival and energy homeostasis under metabolic stress through the maintenance of cellular synthetic pathways, but can also maintain long-period cellular integrity by inhibiting the accumulation of malfunctioning organelles and misfolded proteins (4). Growing findings have revealed the significance of autophagy in numerous physiopathological processes, such as vascular disorders, inflammation and cancer (5). Autophagy in cancer is an intensely debated concept in the field of translational research. Both pro-survival (tumor promotive) and anti-survival (tumor suppressive) properties of autophagy have been reported, of which the dual nature is mainly determined by specific tumor stage and genetic context (6). At early stages of tumor development, autophagy acts in an anti-tumorigenic manner by facilitating DNA damage repair and reducing reactive oxygen species, thus suppressing early tumorigenesis. On the other hand, during late stages of tumor development, autophagy increases the ability of tumor cells to handle endogenous stress and induces chemoresistance, thus promoting the development of existing tumors (7). The participation of autophagy in GC progression and chemoresistance has been previously reported (8-10). However, a number of studies only focused on investigating single pathway or limited genes of interest in GC, which only reflect partial functions of autophagy. Thus far, very few studies have investigated the clinical importance of autophagy in GC in a comprehensive manner. Therefore, an unbiased screening is urgently needed to characterize the gene signature of autophagy and address the association of autophagy with prognosis in GC.
Given that high-throughput expression data is now available, it has become feasible to utilize global gene expression data to examine the relationship between autophagy-related genes (ARGs) expression and the clinical outcomes of patients with cancer (11). Moreover, the establishment of The Cancer Genome Atlas (TCGA) database is helpful for the discovery and further understanding of gene profiles (12). Nevertheless, prognostic models based on ARGs expression profiles in GC have not yet been reported. To the best of our knowledge, no prognosis-related model composed of ARGs for GC has been constructed. The present study, for the first time, constructed a risk signature that contained seven ARGs and further developed a prognostic index (PI) model with a potential to independently and accurately predict the prognostic value for patients with GC. We present the following article in accordance with the REMARK reporting checklist (available at https://dx.doi.org/10.21037/tcr-21-191).
Methods
Data and information extraction
A variety of ARGs were obtained through the Human Autophagy Database (HADb, http://www.autophagy.lu/index.html). TCGA (https://tcga-data.nci.nih.gov/tcga/) provided 415 GC and 35 non-tumor cases with RNA-sequencing and clinical data. A total of 409 patients with GC with intact follow-up and gene expression data were subsequently enrolled in the present study. Additionally, raw data from GSE15460 dataset was downloaded from the gene expression omnibus (GEO) database (https://www.ncbi.nlm.nih.gov/geo/). A total of 248 cases with gene expression and survival data were enrolled from GSE15460 dataset. The study was conducted in accordance with the Declaration of Helsinki (as revised in 2013).
Identification of ARGs with differential expression in GC
To obtain the ARGs that were differentially expressed in GC compared with non-tumor cases, the edgeR package was utilized based on a standard fold-change >2 and P<0.05 after correction. Subsequently, Gene ontology (GO) as well as Kyoto Encyclopedia of Genes and Genomes (KEGG) annotations of these ARGs were conducted to further understand their biological functions. After the analysis of the enrichment of GO and KEGG pathways, the GO plot package was utilized in order to display high-dimensional results.
Establishment and evaluation of the ARGs-based PI model
Univariate Cox regression analyses were performed to examine the relationship between each differentially expressed ARG and the overall survival (OS) of patients with GC. Only those OS-related ARGs were subsequently analyzed using multivariate Cox regression analysis in order to discover the ARGs that act as independent indicators of poor prognosis. Finally, a PI model was constructed based on the combination of the regression coefficient, as well as the expression level of poor OS-related ARGs. A PI value was subsequently calculated for every patient with GC to classify the patients into high- or low-risk groups with the median value as the cut-off value. Then, a Kaplan-Meier (K-M) survival curve was plotted to explore the OS of patients with GC from high-risk or low-risk groups, with the survival differences evaluated by the log-rank test.
Difference of various immune cell subtypes between groups classified by PI model in GC
A total of 22 different types of tumor-infiltrating immune cells were extracted from TCGA (13). The abundance of every type of immune cells was examined using a Wilcoxon test to investigate the difference between the prognostically stratified risk groups.
Statistical analysis
SPSS 18.0 (IBM Corp.) and GraphPad Prism 7 (GraphPad Software, Inc.) were utilized for the statistical analyses. Univariate Cox regression analyses were performed to investigate the relationship between OS and gene expression profiles. Multivariate Cox regression analysis was performed to further identify independent predictors of poor OS from the candidate prognostic ARGs, so as to construct the PI model. P<0.05 was considered to indicate a statistically significant difference.
Results
ARGs with differential expression in GC
A total of 5,298 differentially expressed genes (DEGs) were found in GC compared with non-tumor cases, with 2,434 up-regulated and 2,864 down-regulated. Based on the cross reference with a total of 222 ARGs obtained from the HADb, 25 differentially expressed ARGs were identified (Figure 1A), including 19 up-regulated and 6 down-regulated genes (Figure 1B,C).
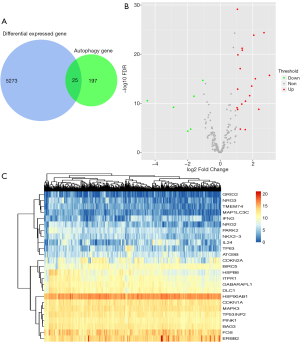
Functional annotations of the differentially expressed ARGs in GC
GO and KEGG analyses were performed for the 25 differently expressed ARGs in GC. In terms of “Molecular Function” (MF), these genes were enriched in “enzyme regulator activity”, “identical protein binding” and “enzyme inhibitor activity” (Figure 2A). The top enriched “Biological Process” (BP) terms were “phosphorylation”, “apoptosis go” and “programmed cell death” (Figure 2B), while there was no enriched “Cellular Component” (CC) terms. Additionally, the enriched KEGG pathways were related to cancer progression (Figure 2C).
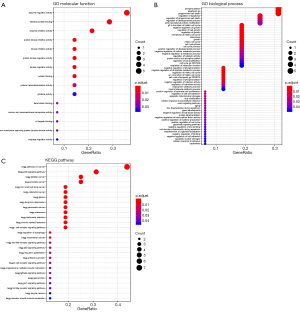
Identification of prognosis-related ARGs
The association of 25 differentially expressed ARGs with OS in GC was analyzed by univariate analyses, resulting in seven prognosis-related ARGs. Multivariate Cox regression analysis further demonstrated that all the seven prognosis-related ARGs (HSPB8, NRG2, GABARAPL1, TMEM74, DLC1, MAP1LC3C and NRG3) were independent indicators of poor OS. The findings from K-M analyses showed that the up-regulation of seven prognosis-related ARGs was significantly related to poor outcomes for patients with GC (Figure 3A,B,C,D,E,F,G).
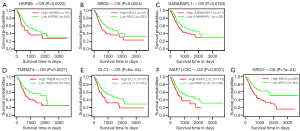
Construction of the ARGs-based PI model
On the basis of the regression coefficients that represented the relative weight of genes in the multivariate Cox regression analysis, the PI model was constructed by the following formula: PI = [0.1472 × expression value (EV) of HSPB8] + (0. 1212 × EV of NRG2) + (0.2196 × EV of GABARAPL1) + (0.1410 × EV of TMEM74) + (0.2565 × EV of DLC1) + (0.1906 × EV of MAP1LC3C) + (0.1240 × EV of NRG3). It was noted that all the coefficients were positive, suggesting the negative association of the seven ARGs with the OS of patients with GC. According to the median score of the PI signature, the patients with GC were sorted into high- or low-risk groups. Of note, the expression of all seven ARGs was observed to be up-regulated in the high-risk group compared with the low-risk group (Figure 4).
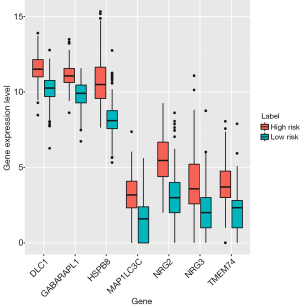
To determine the value of the PI model in predicting the outcome of patients with GC, K-M analyses were performed to compare the OS of the high-risk group with that of the low-risk group, which demonstrated worse outcomes in the high-risk group (HR=2.01, P<0.0001; Figure 5A). Figure 5B,C,D,E show the heatmap of expression profiles for the seven ARGs, the PI distribution and OS of patients, and the number of censor patients in TCGA. Furthermore, the value of the PI model was further evaluated in an external GEO validation cohort GSE15460. A total of 248 patients in the GSE15460 dataset were stratified into low- (n=124) or high-risk group (n=124). In accordance, the high-risk group showed a poor outcome compared with the low-risk group (P=0.0079; Figure 6).
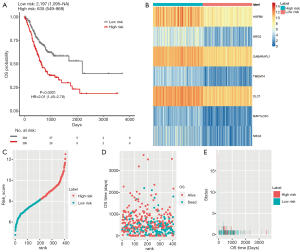
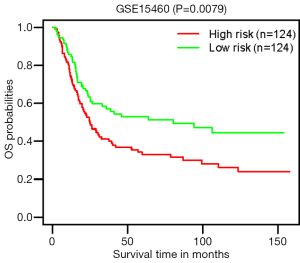
Association of PI model with immune cell infiltration estimates in GC
To investigate the association of the PI model with immune microenvironment, information on 22 different types of tumor-infiltrating immune cells was obtained from TCGA (13), which was calculated using the CIBERSORT algorithm. After a cross reference with our dataset, 385 samples were completely retracted and further classified into low- (n=189) or high-risk group (n=196). There was a difference between low- and high-risk groups regarding the infiltration ratio of different types of immune cells (Table 1). The amounts of “Eosinophils”, “B cells naive”, “T cells CD4 memory resting”, “Dendritic cells resting”, and “Mast cells resting” were significantly increased in the high-risk group, while those of “Macrophages M0”, “T cells follicular helper”, “T cells CD4 memory activated”, “Mast cells activated”, and “Macrophages M1” were significantly decreased (Figure 7A,B).
Table 1
Patterns | Mean of high risk | Mean of low risk | P value |
---|---|---|---|
B.Cells.Memory | 6.89% | 5.26% | 0.084 |
B.Cells.Naive | 7.76% | 5.98% | 0.001* |
Dendritic.Cells.Activated | 1.53% | 2.24% | 0.168 |
Dendritic.Cells.Resting | 2.12% | 1.52% | 0.011* |
Eosinophils | 2.56% | 0.76% | 0.001* |
Macrophages.M0 | 6.53% | 8.84% | 0.001* |
Macrophages.M1 | 5.42% | 6.45% | 0.014* |
Macrophages.M2 | 19.42% | 18.13% | 0.090 |
Mast.Cells.Activated | 6.72% | 7.78% | 0.013* |
Mast.Cells.Resting | 5.65% | 4.16% | 0.036* |
Monocytes | 2.56% | 2.33% | 0.469 |
Neutrophils | 2.66% | 2.73% | 0.071 |
NK.Cells.Activated | 4.07% | 4.79% | 0.279 |
NK.Cells.Resting | 2.79% | 3.70% | 0.094 |
Plasma.Cells | 4.61% | 4.30% | 0.917 |
T.Cells.CD4.Memory.Activated | 3.57% | 4.86% | 0.010* |
T.Cells.CD4.Memory.Resting | 16.88% | 15.09% | 0.009* |
T.Cells.CD4.Naive | 4.56% | 10.76% | 0.800 |
T.Cells.CD8 | 11.77% | 12.23% | 0.759 |
T.Cells.Follicular.Helper | 5.94% | 7.25% | 0.002* |
T.Cells.gamma.delta | 2.80% | 0.76% | 0.267 |
T.Cells.Regulatory.Tregs | 4.92% | 4.77% | 0.345 |
* statistically significant. PI, prognostic index; GC, gastric cancer; TCGA, The Cancer Genome Atlas.
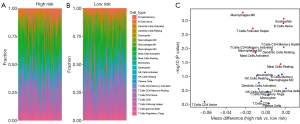
Discussion
GC is still among one of the most aggressive malignancies with high morbidity and mortality, therefore, discovering reliable biomarkers for novel diagnostic, therapeutic and preventive approaches for this disease is a necessity. In terms of cellular processes, autophagy is the primary regulator of homeostasis, the dysregulation of which contributes to the progression of GC. The present study highlighted the high-throughput comprehensive analyses of ARGs in patients with GC.
The present study screened 25 ARGs with differential expression in GC. It should be noted that not all the 25 differentially expressed ARGs were up-regulated, and there were 6 down-regulated genes in GC, which to a certain degree supports the notion that autophagy may play promotive and suppressive roles in tumor development. A total of seven OS-related ARGs (HSPB8, NRG2, GABARAPL1, TMEM74, DLC1, MAP1LC3C and NRG3) were determined and used to construct an OS-related PI model, which was demonstrated to be an independent predictor of poor prognosis for patients with GC. Notably, the seven ARGs in the PI model were upregulated in GC, indicating that autophagy may promote GC progression as a whole (14), through epithelial-to-mesenchymal transition, extracellular matrix degradation, tumor angiogenesis and through shaping the tumor microenvironment (15).
Among the genes in the PI signature, the most well-known function of HSPB8 is acting as a chaperone in the regulation of autophagy (16). HSPB8 has been reported to be indicative of poor prognosis and can promote tumor growth via ERK-CREB pathway activation in patients with GC (17), consistent with the present results. Besides HSPB8, NRG2, a member of neuregulin family, has been reported to be incorporated in disease free survival-related prognostic model for prostate cancer (18), and NRG2 is among the DEGs that can potentially predict biochemical recurrence after curative surgery in prostate cancer (19). Neuregulins have an EGF-like domain located in its extracellular portion, which is responsible for binding and activation of the ErbB receptors (20). In comparison with NRG2, little is known about NRG3, most likely due to its low affinity for receptors and poor signaling activity (21). At present, as NRG3 is exclusively expressed in the nervous system, most research concerning NRG3 is related to the nervous system (22). The present results indicated that NRG3 might be involved in cancer progression.
GABARAPL1 belongs to the ATG8 family and is associated with autophagic vesicles and acts with other GABARAP family members to regulate autophagosome maturation (23). GABARAPL1 participates in a variety of cancers, and has a promotive as well as suppressive function in breast (24,25) and prostate cancer (26,27). The other subfamily of ATG8 is MAP1LC3, one isoform of which is MAP1LC3C (28). It was recently found that cancer cells with low MAP1LC3C expression possessed enhanced Met stability, signaling and cell invasion (29). Furthermore, MAP1LC3C could mediate selective autophagy of the MET receptor tyrosine kinase, thus leading to the inhibition of tumor invasion (30). Therefore, the promotive or suppressive role of GABARAPL1 and MAP1LC3C in cancer progression may depend on different tumor stages and microenvironment. The present study showed the potential involvement of GABARAPL1 and MAP1LC3C in GC progression.
As a transmembrane protein, TMEM74 is reported to enhance proliferation by inducing autophagy. Furthermore, TMEM74 itself may become down-regulated through autophagic process, indicating the existence of a potential self-regulatory loop in order to sustain a suitable autophagic level, which prevents tumor cells from death caused by excessive autophagy (31). The overexpression of TMEM74 not only leads to accelerated proliferation of liver and lung cancer cells, but also correlates with reduced survival rates (32). TMEM74 could associate with BIK and inhibit the induction of apoptosis, providing an interaction or crosstalk between autophagy and apoptosis (33). At present, the role of TMEM74 in tumor development has not been widely studied, hence the underlying mechanism of TMEM74 in GC progression still needs further investigation. DLC1, a GTPase-activating protein for Rho family members, acts as a potential tumor suppressor and is frequently down-regulated or inactivated in numerous types of cancer, which facilitates tumor cells to proliferate and disseminate (34). DLC1 can promote senescence via the FoxO3a/NF-κB signaling pathway mediated by SIRT1 following treatment of breast cancer with resveratrol (35), whereas deficiency of DLC1 can drive cell contact inhibition via YAP signaling (36). However, recent studies have discovered the oncogenic role of DLC1 and that its expression is up-regulated in melanoma tissues, where nuclear DLC1 acts as an oncogene through association with FOXK1 to co-activate the expression of MMP9 (37). Furthermore, as a direct target of activated YAP/TAZ, DLC1 could drive sprouting angiogenesis and collective migration (38), suggesting that the role and function of DLC1 is highly dependent on the cellular microenvironment. Thus far, few studies have examined the role of DLC1 in autophagy, and DLC1 was reported to inhibit cancer progression and oncogenic autophagy in hepatocellular carcinoma (39). The present study indicated that DLC1 may be involved in autophagy-mediated tumor promotion in GC, therefore, further investigations are still needed to validate the role of DLC1 in GC autophagy.
The present study also investigated the association of the PI model with infiltrating immune cells in GC. The number of eosinophils and resting mast cells was significantly increased in the PI high-risk group, whereas the number of M1 macrophages decreased, suggesting the heterogeneity of immune infiltration in GC. Increased numbers of eosinophils and decreased anti-tumorigenic M1 macrophages are associated with poor prognosis of patients with colorectal cancer (40) and hepatocellular carcinoma (41). In addition, resting mast cells were related to the PI high-risk group based on ARG signature in pancreatic adenocarcinoma (42). Meanwhile, it should be noted that the present study did not find any association between the PI model and regulatory T cells, neutrophils or CD8+ T cells, which suggested that future studies are required to discover whether the ARGs have an effect on the infiltration of immune cells in GC.
In conclusion, the present study identified 25 ARGs that were differentially expressed in GC tissue samples through an unbiased and comprehensive method. A PI model was built that included seven prognostic ARGs, which could robustly estimate survival in patients with GC and was further validated in an external GEO validation cohort.
Acknowledgments
We thank Lindy Jiang for her generous help with the language edition.
Funding: This work was supported by
Footnote
Reporting Checklist: The authors have completed the REMARK reporting checklist. Available at https://dx.doi.org/10.21037/tcr-21-191
Conflicts of Interest: All authors have completed the ICMJE uniform disclosure form (available at https://dx.doi.org/10.21037/tcr-21-191). The authors have no conflicts of interest to declare.
Ethical Statement: The authors are accountable for all aspects of the work in ensuring that questions related to the accuracy or integrity of any part of the work are appropriately investigated and resolved. The study was conducted in accordance with the Declaration of Helsinki (as revised in 2013).
Open Access Statement: This is an Open Access article distributed in accordance with the Creative Commons Attribution-NonCommercial-NoDerivs 4.0 International License (CC BY-NC-ND 4.0), which permits the non-commercial replication and distribution of the article with the strict proviso that no changes or edits are made and the original work is properly cited (including links to both the formal publication through the relevant DOI and the license). See: https://creativecommons.org/licenses/by-nc-nd/4.0/.
References
- Siegel RL, Miller KD, Jemal A. Cancer statistics, 2020. CA Cancer J Clin 2020;70:7-30. [Crossref] [PubMed]
- Catenacci DVT, Tebbutt NC, Davidenko I, et al. Rilotumumab plus epirubicin, cisplatin, and capecitabine as first-line therapy in advanced MET-positive gastric or gastro-oesophageal junction cancer (RILOMET-1): a randomised, double-blind, placebo-controlled, phase 3 trial. Lancet Oncol 2017;18:1467-82. [Crossref] [PubMed]
- Hecht JR, Bang YJ, Qin SK, et al. Lapatinib in Combination With Capecitabine Plus Oxaliplatin in Human Epidermal Growth Factor Receptor 2-Positive Advanced or Metastatic Gastric, Esophageal, or Gastroesophageal Adenocarcinoma: TRIO-013/LOGiC--A Randomized Phase III Trial. J Clin Oncol 2016;34:443-51. [Crossref] [PubMed]
- Panda PK, Mukhopadhyay S, Das DN, et al. Mechanism of autophagic regulation in carcinogenesis and cancer therapeutics. Semin Cell Dev Biol 2015;39:43-55. [Crossref] [PubMed]
- Wen X, Klionsky DJ. At a glance: A history of autophagy and cancer. Semin Cancer Biol 2020;66:3-11. [Crossref] [PubMed]
- Mulcahy Levy JM, Thorburn A. Autophagy in cancer: moving from understanding mechanism to improving therapy responses in patients. Cell Death Differ 2020;27:843-57. [Crossref] [PubMed]
- Singh SS, Vats S, Chia AY, et al. Dual role of autophagy in hallmarks of cancer. Oncogene 2018;37:1142-58. [Crossref] [PubMed]
- Ma L, Wang Z, Xie M, et al. Silencing of circRACGAP1 sensitizes gastric cancer cells to apatinib via modulating autophagy by targeting miR-3657 and ATG7. Cell Death Dis 2020;11:169. [Crossref] [PubMed]
- Zhou J, Yang J, Fan X, et al. Chaperone-mediated autophagy regulates proliferation by targeting RND3 in gastric cancer. Autophagy 2016;12:515-28. [Crossref] [PubMed]
- Hu Y, Yu Y, You S, et al. Long noncoding RNA MALAT1 regulates autophagy associated chemoresistance via miR-23b-3p sequestration in gastric cancer. Mol Cancer 2017;16:174. [Crossref] [PubMed]
- Zhou Z, Mo S, Dai W, et al. Development and Validation of an Autophagy Score Signature for the Prediction of Post-operative Survival in Colorectal Cancer. Front Oncol 2019;9:878. [Crossref] [PubMed]
- Shih JH, Chen HY, Lin SC, et al. Integrative analyses of noncoding RNAs reveal the potential mechanisms augmenting tumor malignancy in lung adenocarcinoma. Nucleic Acids Res 2020;48:1175-91. [Crossref] [PubMed]
- Thorsson V, Gibbs DL, Brown SD, et al. The Immune Landscape of Cancer. Immunity 2018;48:812-830.e14. Erratum in: Immunity 2019;51:411-2. [Crossref] [PubMed]
- Levy JMM, Towers CG, Thorburn A. Targeting autophagy in cancer. Nat Rev Cancer 2017;17:528-42. [Crossref] [PubMed]
- Qian HR, Yang Y. Functional role of autophagy in gastric cancer. Oncotarget 2016;7:17641-51. [Crossref] [PubMed]
- Guilbert SM, Lambert H, Rodrigue MA, et al. HSPB8 and BAG3 cooperate to promote spatial sequestration of ubiquitinated proteins and coordinate the cellular adaptive response to proteasome insufficiency. FASEB J 2018;32:3518-35. [Crossref] [PubMed]
- Shen J, Li M, Min L. HSPB8 promotes cancer cell growth by activating the ERKCREB pathway and is indicative of a poor prognosis in gastric cancer patients. Oncol Rep 2018;39:2978-86. [PubMed]
- Hu D, Jiang L, Luo S, et al. Development of an autophagy-related gene expression signature for prognosis prediction in prostate cancer patients. J Transl Med 2020;18:160. [Crossref] [PubMed]
- Li F, Ji JP, Xu Y, et al. Identification a novel set of 6 differential expressed genes in prostate cancer that can potentially predict biochemical recurrence after curative surgery. Clin Transl Oncol 2019;21:1067-75. [Crossref] [PubMed]
- Czarnek M, Bereta J. Proteolytic Processing of Neuregulin 2. Mol Neurobiol 2020;57:1799-813. [Crossref] [PubMed]
- Müller T, Braud S, Jüttner R, et al. Neuregulin 3 promotes excitatory synapse formation on hippocampal interneurons. EMBO J 2018;37:e98858. [Crossref] [PubMed]
- Avramopoulos D. Neuregulin 3 and its roles in schizophrenia risk and presentation. Am J Med Genet B Neuropsychiatr Genet 2018;177:257-66. [Crossref] [PubMed]
- Chakrama FZ, Seguin-Py S, Le Grand JN, et al. GABARAPL1 (GEC1) associates with autophagic vesicles. Autophagy 2010;6:495-505. [Crossref] [PubMed]
- Ran L, Hong T, Xiao X, et al. GABARAPL1 acts as a potential marker and promotes tumor proliferation and metastasis in triple negative breast cancer. Oncotarget 2017;8:74519-26. [Crossref] [PubMed]
- Poillet-Perez L, Jacquet M, Hervouet E, et al. GABARAPL1 tumor suppressive function is independent of its conjugation to autophagosomes in MCF-7 breast cancer cells. Oncotarget 2017;8:55998-6020. [Crossref] [PubMed]
- Su B, Zhang L, Liu S, et al. GABARAPL1 Promotes AR+ Prostate Cancer Growth by Increasing FL-AR/AR-V Transcription Activity and Nuclear Translocation. Front Oncol 2019;9:1254. [Crossref] [PubMed]
- Su W, Li S, Chen X, et al. GABARAPL1 suppresses metastasis by counteracting PI3K/Akt pathway in prostate cancer. Oncotarget 2017;8:4449-59. [Crossref] [PubMed]
- Schaaf MB, Keulers TG, Vooijs MA, et al. LC3/GABARAP family proteins: autophagy-(un)related functions. FASEB J 2016;30:3961-78. [Crossref] [PubMed]
- Bell ES, Coelho PP, Ratcliffe CDH, et al. LC3C-Mediated Autophagy Selectively Regulates the Met RTK and HGF-Stimulated Migration and Invasion. Cell Rep 2019;29:4053-68.e6. [Crossref] [PubMed]
- Bell ES, Coelho PP, Park M. LC3C mediates selective autophagy of the MET RTK, inhibiting cancer cell invasion. Autophagy 2020;16:959-61. [Crossref] [PubMed]
- Sun Y, Chen Y, Zhang J, et al. TMEM74 promotes tumor cell survival by inducing autophagy via interactions with ATG16L1 and ATG9A. Cell Death Dis 2017;8:e3031. [Crossref] [PubMed]
- Sun Y, Deng J, Xia P, et al. The Expression of TMEM74 in Liver Cancer and Lung Cancer Correlating With Survival Outcomes. Appl Immunohistochem Mol Morphol 2019;27:618-25. [Crossref] [PubMed]
- Sun Y, Li Q, Zhang J, et al. Autophagy regulatory molecule, TMEM74, interacts with BIK and inhibits BIK-induced apoptosis. Cell Signal 2017;36:34-41. [Crossref] [PubMed]
- Zhang Y, Li G. A tumor suppressor DLC1: The functions and signal pathways. J Cell Physiol 2020;235:4999-5007. [Crossref] [PubMed]
- Ji S, Zheng Z, Liu S, et al. Resveratrol promotes oxidative stress to drive DLC1 mediated cellular senescence in cancer cells. Exp Cell Res 2018;370:292-302. [Crossref] [PubMed]
- Ritchey L, Ha T, Otsuka A, et al. DLC1 deficiency and YAP signaling drive endothelial cell contact inhibition of growth and tumorigenesis. Oncogene 2019;38:7046-59. [Crossref] [PubMed]
- Yang X, Hu F, Liu JA, et al. Nuclear DLC1 exerts oncogenic function through association with FOXK1 for cooperative activation of MMP9 expression in melanoma. Oncogene 2020;39:4061-76. [Crossref] [PubMed]
- van der Stoel M, Schimmel L, Nawaz K, et al. DLC1 is a direct target of activated YAP/TAZ that drives collective migration and sprouting angiogenesis. J Cell Sci 2020;133:jcs239947. [Crossref] [PubMed]
- Wu HT, Xie CR, Lv J, et al. The tumor suppressor DLC1 inhibits cancer progression and oncogenic autophagy in hepatocellular carcinoma. Lab Invest 2018;98:1014-24. [Crossref] [PubMed]
- Xiong Y, Wang K, Zhou H, et al. Profiles of immune infiltration in colorectal cancer and their clinical significant: A gene expression-based study. Cancer Med 2018;7:4496-508. [Crossref] [PubMed]
- Yu S, Wang Y, Hou J, et al. Tumor-infiltrating immune cells in hepatocellular carcinoma: Tregs is correlated with poor overall survival. PLoS One 2020;15:e0231003. [Crossref] [PubMed]
- Yue P, Zhu C, Gao Y, et al. Development of an autophagy-related signature in pancreatic adenocarcinoma. Biomed Pharmacother 2020;126:110080. [Crossref] [PubMed]