Progesterone receptor gene serves as a prognostic biomarker associated with immune infiltration in gastric cancer: a bioinformatics analysis
Introduction
Gastric cancer (GC) remains one of the most common malignancies worldwide with a poor prognosis, posing a risk to human health (1). The pathogenesis and mechanism of GC are complex and immunological mechanisms play a role in regulating GC development (2,3). By targeting the host immune system, immunotherapy has shown great promise in several solid cancers and has evolved into a future therapeutic option in GC (4). Immunotherapies, including several immune checkpoints such as cytotoxic T-lymphocyte-associated antigen 4 (CTLA-4), programmed cell death-1 (PD-1) and programmed death ligand-1 (PD-L1), have proven efficacious in several solid cancers (4,5). However, unlike other solid tumors, clinical responses to immunotherapy in GC still needs to be evaluated because immunotherapy has shown poor clinical efficacy in some clinical trials (4,6,7). The tumor microenvironment has significant influences on the therapeutic response, clinical outcome and immune cells within the microenvironment (8). Several studies have shown that tumor-associated macrophages (TAMs), regulatory T-lymphocytes (Tregs) and other tumor-infiltrating lymphocytes are associated with the development and outcome of cancers (9-11). To date, however, there has been little discussion about the regulation of immune cells and immunotherapy targets of GC, which still needs to be clarified.
Biomarkers contribute greatly to the diagnosis and management of cancer and related follow-up programs. The conventional biomarkers of GC are widely used in clinical practice, and mainly include CEA, CA19-9, CA72-4, CA125, etc. (12). However, because of their insufficient specificity and sensitivity, more novel biomarkers need to be investigated. So far, PI3K/Akt/mTOR (13), long noncoding RNAs (14), PD-L1 (15) and other biomarkers are related to the prognosis in GC patients.
The progesterone receptor gene (PGR), which is located at 11q22-q23, is a member of the steroid receptor superfamily of nuclear receptors (16). It encodes the human progesterone receptor (PR), which is involved in establishing and maintaining a pregnancy (17). Two main PR isoforms, PR-A and PR-B, have different expressions and functions (18). Progesterone, which can promote the downregulation of proinflammatory cytokines and chemokines, must bind to the PR to exert its effect (19). Mutations and polymorphisms of PGR are related to tumorigenesis and risks for cancers, including ovarian, breast and endometrial cancers (16,20,21). However, the relationship between PGR expression and the GC prognosis remains unclear. So far, PGR regulation in GC patients remains controversial, according to one study, PR may play a role in the progression of GC (22) but others have reported that PR was not significantly related to GC prognosis (23). PR can modulate the immune response by altering Th1 and Treg activity (24). Also, studies have found that the PR is related to infiltration of a subpopulation of Tregs, which indicated a poorer prognosis for breast cancer (25,26). A higher percentage of CD25+/FOXP3+/CD127 T cells within CD3+/CD4+ T cells has been observed in PR (–) breast cancer patients (19), and Treg cell infiltration was more prominent in breast cancer patients with estrogen receptor (ER) (+) and PR (–) tumors (26). Until now, few studies have examined the association between PGR and immune cell infiltration in GC.
The purpose of this study was to analyze the relationship between PGR expression and prognosis of cancer patients using databases, including Oncomine, PrognoScan, and Kaplan-Meier plotter. Also, the correlation of PGR and immune cell infiltration of tumors was investigated by the Tumor Immune Estimation Resource (TIMER). The findings of this study bring new insights into the potential prognostic role of PGR in GC and the possible mechanisms of PGR and immune cell interaction, which may help improve the early diagnosis and prognosis of GC in clinical practice.
We present the following article in accordance with the MDAR checklist (available at https://dx.doi.org/10.21037/tcr-21-218).
Methods
Data collection from The Cancer Genome Atlas (TCGA)
The gene expression profiles of GC patients, including clinical data including sex, tumor stage, TNM classification, and survival status, were downloaded from the TCGA portal (v22.0, https://portal.gdc.cancer.gov/) with project ID: TCGA-GC.
PGR differential expression
Oncomine (http://www.oncomine.org) is an online database that contains 715 datasets and 86,733 samples to support various analyses for cancer research (27). To evaluate the relationship between PGR expression levels and cancer patient prognosis in different types of cancers, we used the Oncomine database, with the threshold of P value of 0.001, fold change of 1.5, and gene ranking of all.
Survival analyses of PGR
PrognoScan (http://www.prognoscan.org/) is a large public dataset of gene expression and clinical information, providing a novel platform for evaluating potential tumor markers and therapeutic targets (28). The relationship between PGR expression and patient survival in various types of cancers was analyzed through a minimum P value approach. Kaplan-Meier plotter (http://kmplot.com/analysis/) is an online platform that can be used to evaluate the effect of 54,675 genes on survival based on 10,461 cancer samples, including 5,143 breast, 1,816 ovarian, 2,437 lung, and 1,065 gastric cancers (29). In this study, the associations between survival and PGR expression in patients with breast, ovarian, lung and gastric cancers were analyzed using the Kaplan-Meier plotter database by the log-rank method. To investigate whether PGR is an independent prognostic biomarker in GC, Cox analysis was performed. All clinical variables were obtained for a multivariate Cox analysis, aiming to evaluate whether each of the variables had an independent prognostic value.
Correlation between PGR and infiltrating immune cells
TIMER (http://cistrome.org/TIMER/) is an analytical web tool that includes 10,897 samples of 32 cancer types from TCGA, supporting the comprehensive analysis of tumor-infiltrating immune cells (30). We used it to analyze the relationship between PGR expression levels with abundant immune infiltrates, including B cells, CD4+ T cells, CD8+ T cells, neutrophils, macrophages, and dendritic cells (DCs). Correlations between PGR expression and gene markers of tumor-infiltrating immune cells reported in previous studies (31,32) were investigated using this database. The Gene Expression Profiling Interactive Analysis (GEPIA) (http://gepia.cancer-pku.cn/index.html), which incorporates 9,736 tumor samples and 8,587 normal controls from TCGA and the Genotype Tissue Expression (GTEx), is an online tool that is capable of integrated analysis of cancer expression profiling data (33). GEPIA was used to assess the relationship between PGR expression and patient survival and immune cell infiltration of tumors by the Spearman method in this study. The study was conducted in accordance with the Declaration of Helsinki (as revised in 2013).
Statistical analysis
Survival curves were calculated by PrognoScan and KaplanMeier plots, containing results with a hazard ratio (HR) and P or Cox P values from a log-rank test. P values, fold changes, and ranks are shown in the results from the Oncomine database. We used Spearman correlations to examine correlations between variables, with the strength of a correlation determined by the following r values: 0.00–0.19 “very weak”, 0.20–0.39 “weak”, 0.40–0.59 “moderate”, 0.60–0.79 “strong” and 0.80–1.0 “very strong”. Two-sided P<0.05 was considered significant.
Results
Expression levels of PGR in different cancers
To investigate the differences PGR expression in tumor and normal tissues in multiple cancers, we first used the Oncomine database for analysis. Compared with normal tissues, the expression levels of PGR showed difference in bladder, breast, cervical, colorectal, gastric, head and neck, lung, ovarian and prostate cancers (Figure S1A). Table S1 shows the detailed information on the PGR expression in various types of cancers. We performed analysis using the TIMER database further to evaluate the different PGR expressions in different tumor tissues. The expression levels of PGR in tumor tissues of BLCA (urothelial bladder carcinoma), BRCA (invasive breast carcinoma), COAD (colon adenocarcinoma), HNSC (head and neck cancer), KIRC (kidney renal clear cell carcinoma), KIRP (kidney renal papillary cell carcinoma), LIHC (liver hepatocellular carcinoma), LUAD (lung adenocarcinoma), LUSC (lung squamous cell carcinoma), PRAD (prostate adenocarcinoma), READ (rectum adenocarcinoma), STAD (stomach adenocarcinoma), UCEC (uterine corpus endometrial carcinoma), KICH (kidney chromophobe) and THCA (thyroid carcinoma) showed significant difference compared with adjacent normal tissues (Figure S1B).
PGR expression and prognosis of multiple cancers
Because PGR expression was significantly different between cancer and normal tissues in multiple cancers, we then evaluated whether the PGR expression levels can predict the prognosis of cancer patients. We analyzed their correlations using the PrognoScan database (Table S2). The results demonstrated a significant statistical correlation between expression of PGR and the prognosis of various types of cancer (Figure S2). We then used the GEPIA database further to analyze the prognostic potential of PGR in different types of cancer. The results showed that high PGR expression correlated with poorer prognosis of ACC (adrenocortical carcinoma), MESO (mesothelioma) and STAD, but was linked to better prognosis of BRCA, KIRC, LGG (brain lower grade glioma), LIHC, SARC (sarcoma) and UCEC (Figure 1). We then performed a survival analysis using the Kaplan-Meier plotter database to evaluate further the association between expression of PGR and patients’ prognosis in different cancers (Figure 2). Higher PGR expression’s correlation with poorer prognosis in GC was observed, with overall survival (OS) HR of 1.74 [95% confidence interval (CI), 1.4–2.17, P value =6.2e-7] and progression-free survival (PFS) HR of 2.09 (95% CI, 1.58–2.78, P value =1.6e-7). However, PGR expression was found to be related with better prognosis in lung cancer (OS HR =0.56, 95% CI, 0.47–0.66, P=1.3e-11; PFS HR =0.53, 95% CI, 0.34–0.82, P=0.0036), breast cancer (OS HR =0.6, 95% CI, 0.44–0.83, P=0.0015; relapse-free survival HR =0.53, 95% CI, 0.45–0.62, P=1.6e-15) and ovarian cancer (OS HR =0.75, 95% CI, 0.61–0.91, P=0.0046; PFS HR =0.67, 95% CI, 0.56–0.81, P=3.4e-5). These results suggested that PGR expression was correlated with prognosis in multiple cancers.
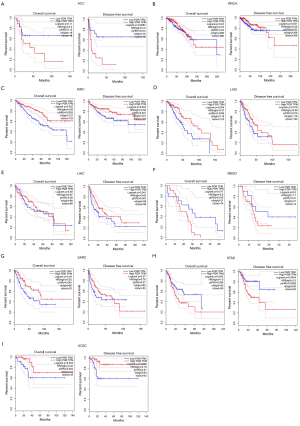
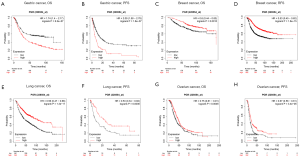
Relationship of elevated PGR expression levels and GC patients’ prognosis of different clinicopathological factors
To further explain the relationship between PGR expression and GC, we analyzed PGR expression levels and different clinicopathological factors in GC by the Kaplan-Meier plotter database. In both female and male patients, elevated PGR expression levels had a statistically significant relationship with worse OS and PFS (P<0.05) (Table 1). Also, except for stage 1, stage 2, stage M1 and poorly differentiated cancer, high expression of PGR was associated with worse OS and PFS in patients with different clinicopathological factors, such as stage, stage N, stage M, Lauren classification and differentiation (Table 1). Notably, PGR expression was related to each N stage in GC and had the highest OS and PFS HR values in stage N1 (OS HR =9.66, 95% CI, 1.29–72.12, P=0.0068; PFS HR =9.89, 95% CI, 1.33–73.78, P=0.006). Because stage N represents the different lymph node metastasis status, which is a strong risk factor for GC (34,35), these results indicated that PGR expression might be involved in GC patients with lymph node metastasis.
Table 1
Clinicopathological characteristics | Overall survival (n=881) | Progression-free survival (n=645) | |||||
---|---|---|---|---|---|---|---|
N | Hazard ratio | P value | N | Hazard ratio | P value | ||
Gender | |||||||
Female | 236 | 2.47 (1.53–4) | 0.0001 | 201 | 3.02 (1.56–5.8) | 0.0006 | |
Male | 544 | 1.79 (1.31–2.45) | 0.0002 | 437 | 1.8 (1.26–2.55) | 0.0009 | |
Stage | |||||||
1 | 67 | 1.87 (0.56–6.2) | 0.301 | 60 | 1.72 (0.52–5.74) | 0.3703 | |
2 | 140 | 2.3 (0.9–5.89) | 0.0747 | 131 | 1.6 (0.79–3.26) | 0.1914 | |
3 | 305 | 1.71 (1.11–2.64) | 0.0147 | 186 | 1.79 (1.14–2.79) | 0.0098 | |
4 | 148 | 2.02 (1.31–3.13) | 0.0013 | 141 | 1.74 (1.07–2.83) | 0.0247 | |
Stage T | |||||||
2 | 241 | 1.79 (1.17–2.74) | 0.0062 | 239 | 1.64 (1.02–2.63) | 0.0392 | |
3 | 204 | 1.58 (1.12–2.23) | 0.0085 | 204 | 1.51 (1.08–2.11) | 0.0144 | |
4 | 38 | 2.76 (1.15–6.67) | 0.0189 | 39 | 2.19 (0.98–4.89) | 0.0517 | |
Stage N | |||||||
0 | 74 | 9.66 (1.29–72.12) | 0.0068 | 72 | 9.89 (1.33–73.78) | 0.006 | |
1 | 225 | 2.16 (1.43–3.27) | 0.0002 | 222 | 2.55 (1.45–4.49) | 0.0007 | |
2 | 121 | 1.57 (0.97–2.52) | 0.0622 | 125 | 1.49 (0.94–2.35) | 0.0463 | |
3 | 76 | 2.26 (1.22–4.18) | 0.0079 | 76 | 2.05 (1.11–3.8) | 0.0194 | |
1+2+3 | 422 | 1.77 (1.36–2.31) | 1.7e-5 | 423 | 1.59 (1.23–2.05) | 0.0003 | |
Stage M | |||||||
0 | 444 | 1.97 (1.4–2.78) | 8.3e-5 | 443 | 1.89 (1.36–2.64) | 0.0001 | |
1 | 56 | 1.87 (0.93–3.73) | 0.073 | 56 | 1.64 (0.79–3.38) | 0.1799 | |
Lauren classification | |||||||
Intestinal | 320 | 2.08 (1.38–3.14) | 0.0003 | 263 | 1.99 (1.33–2.97) | 0.0006 | |
Diffuse | 241 | 2 (1.41–2.85) | 8.3e-5 | 231 | 2.05 (1.44–2.92) | 4.5e-5 | |
Mixed | 32 | 2.98 (0.96–9.2) | 0.0468 | 28 | 1.89 (0.69–5.16) | 0.2076 | |
Differentiation | |||||||
Poorly differentiated | 165 | 1.25 (0.77–2.03) | 0.3627 | 121 | 1.28 (0.81–2.03) | 0.288 | |
Moderately differentiated | 67 | 2.32 (0.9–5.97) | 0.0737 | 67 | 2.67 (1.04–6.82) | 0.0332 |
PGR, progesterone receptor gene.
To investigate whether PGR is an independent prognostic factor of GC, a Cox proportional-hazards model was constructed. The Kaplan-Meier plotter database was used to perform the multivariate Cox analyses, which can confirm the significant value of PGR in the prognosis of GC (Table S3). The results showed the critical value of stage T, stage N, Lauren classification and PGR as independent prognosis factors in OS (Table S3). The Cox analysis based on PFS showed that stage N, Lauren classification and PGR were risk factors. Taking together, PGR is a potential independent risk factor in GC.
Correlation of the expression of PGR in gastric cancer and immune infiltration
Tumor-infiltrating lymphocytes are an attempt by the host to eradicate malignancies, and be an independent predictor of survival in multiple cancer (36,37). Hence, we used the TIMER database to investigate the correlation between expression of PGR and immune cell infiltration in 39 types of cancer. We found that PGR expression had a significant relationship with tumor purity in 26 types of cancer, with B cells in 20 cancers, CD8+ T cells in 24 cancers, CD4+ T cells in 26 cancers, macrophages in 30 cancers, neutrophils in 23 cancers, and DCs in 23 cancers (Figure S3). Compared with PGR expression in DLBC (diffuse large B-cell lymphoma) which had no significant relationship with B cells, CD8+T cells, CD4+T cells, macrophages, neutrophils or DCs infiltration, PGR expression in STAD showed significant correlations with levels of B cells (r=0.225, P=1.26e-5), CD8+T cells (r=0.248, P=1.41e-6), CD4+T cells (r=0.524, P=3.27e-27), macrophages (r=0.652, P=3.30e-36), neutrophils (r=0.25, P=1.13e-6) and dendritic cells (r=0.421, P=2.19e-17) (Figure 3A,B). Also, Kaplan-Meier plots using the TIMER database were constructed to investigate further whether PGR expression affected immune infiltration in STAD and DLBC. It was observed that macrophage infiltration (P=0.004) and PGR expression (P=0.045) had significantly correlations with STAD prognosis, but no such significant relations were found in DLBC (P>0.05) (Figure 3C,D). Thus, these findings indicated that PGR expression was strongly related to immune infiltration in GC, especially in terms of macrophages.
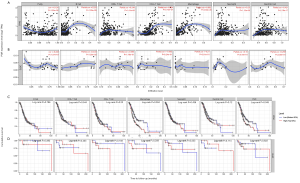
Correlation between PGR expression and immune markers
To investigate the relationship between the expression of PGR and multiple immune markers in STAD, their correlations were analyzed by the TIMER and GEPIA databases. Immune markers of different immune cells, including CD8+ T cells, T cells (general), B cells, monocytes, TAMs, M1 and M2 macrophages, neutrophils, natural killer (NK) cells, DCs, Th1 cells, Th2 cells, Tfh cells, Th17 cells, Tregs and exhausted T cells, were chosen to be analyzed in STAD, with DLBC as the control. Adjusted by tumor purity, the results showed that PGR expression had a significant relationship with most of the markers of CD8+ T cells, T cells (general), B cells, monocytes, TAMs, M1 macrophages, M2 macrophages, neutrophils, NK cells, DCs, Th1 cells, Th2 cells, Tfh cells, Th17 cells, Tregs and exhausted T cells in STAD (Table 2). However, PGR expression was only found to be related to three markers of M1 macrophages, NK cells and Th17 cells in DLBC.
Table 2
Description | Gene markers | STAD | DLBC | |||||||
---|---|---|---|---|---|---|---|---|---|---|
None | Purity | None | Purity | |||||||
Cor | P | Cor | P | Cor | P | Cor | P | |||
CD8+ T cell | CD8A | 0.308 | *** | 0.287 | *** | 0.206 | 0.16 | 0.126 | 0.431 | |
CD8B | 0.172 | *** | 0.153 | *** | 0.137 | 0.3533 | 0.099 | 0.539 | ||
T cell (general) | CD3D | 0.245 | *** | 0.217 | *** | 0.029 | 0.845 | −0.049 | 0.762 | |
CD3E | 0.295 | *** | 0.272 | *** | 0.136 | 0.356 | 0.036 | 0.821 | ||
CD2 | 0.32 | *** | 0.301 | *** | 0.102 | 0.491 | 0.02 | 0.9 | ||
B cell | CD19 | 0.377 | *** | 0.36 | *** | −0.05 | 0.733 | 0.076 | 0.637 | |
CD79A | 0.386 | *** | 0.365 | *** | 0.065 | 0.661 | 0.045 | 0.78 | ||
Monocyte | CD86 | 0.337 | *** | 0.324 | *** | 0.169 | 0.252 | 0.278 | 0.078 | |
CD115 (CSF1R) | 0.443 | *** | 0.475 | *** | 0.431 | * | 0.308 | 0.05 | ||
TAM | CCL2 | 0.438 | *** | 0.428 | *** | 0.273 | 0.06 | 0.282 | 0.0741 | |
CD68 | 0.195 | *** | 0.189 | ** | 0.194 | 0.187 | 0.083 | 0.605 | ||
IL10 | 0.385 | *** | 0.376 | *** | 0.343 | 0.017 | 0.223 | 0.161 | ||
M1 Macrophage | INOS (NOS2) | −0.037 | 0.456 | −0.042 | 0.415 | 0.238 | 0.104 | 0.114 | 0.477 | |
IRF5 | 0.254 | *** | 0.244 | *** | 0.394 | * | 0.331 | * | ||
COX2 (PTGS2) | 0.238 | *** | 0.231 | *** | 0.377 | * | 0.268 | 0.089 | ||
M2 Macrophage | CD163 | 0.388 | *** | 0.382 | *** | 0.121 | 0.413 | 0.152 | 0.343 | |
VSIG4 | 0.378 | *** | 0.384 | *** | 0.05 | 0.738 | 0.079 | 0.625 | ||
MS4A4A | 0.468 | *** | 0.466 | *** | 0.255 | 0.0789 | 0.295 | 0.0611 | ||
Neutrophils | CD66b (CEACAM8) | 0.124 | 0.0112 | 0.127 | 0.013 | 0.105 | 0.476 | 0.07 | 0.663 | |
CD11b (ITGAM) | 0.438 | *** | 0.433 | *** | 0.179 | 0.222 | 0.045 | 0.778 | ||
CCR7 | 0.456 | *** | 0.439 | *** | 0.211 | 0.151 | 0.105 | 0.514 | ||
Natural killer cell | KIR2DL1 | 0.174 | ** | 0.153 | ** | 0.181 | 0.217 | 0.034 | 0.832 | |
KIR2DL3 | 0.118 | 0.0157 | 0.078 | 0.13 | 0.352 | 0.0142 | 0.343 | 0.0279 | ||
KIR2DL4 | −0.042 | 0.398 | −0.07 | 0.171 | 0.403 | * | 0.292 | 0.0637 | ||
KIR3DL1 | 0.137 | * | 0.109 | 0.0338 | 0.173 | 0.238 | 0.167 | 0.298 | ||
KIR3DL2 | 0.17 | ** | 0.138 | * | 0.227 | 0.12 | 0.108 | 0.501 | ||
KIR3DL3 | −0.02 | 0.692 | −0.025 | 0.621 | 0.064 | 0.664 | 0.037 | 0.819 | ||
KIR2DS4 | 0.043 | 0.38 | 0.006 | 0.9 | 0.23 | 0.116 | 0.1 | 0.532 | ||
Dendritic cell | HLA-DPB1 | 0.298 | *** | 0.278 | *** | 0.188 | 0.201 | −0.139 | 0.387 | |
HLA-DQB1 | 0.138 | ** | 0.11 | 0.0324 | 0.168 | 0.254 | −0.079 | 0.622 | ||
HLA-DRA | 0.205 | *** | 0.185 | ** | 0.119 | 0.421 | 0.075 | 0.640 | ||
HLA-DPA1 | 0.242 | *** | 0.222 | *** | 0.094 | 0.526 | 0.089 | 0.581 | ||
BDCA-1 (CD1C) | 0.555 | *** | 0.563 | *** | 0.202 | 0.168 | 0.175 | 0.273 | ||
BDCA-4 (NRP1) | 0.599 | *** | 0.592 | *** | 0.515 | ** | −0.222 | 0.157 | ||
CD11c (ITGAX) | 0.388 | *** | 0.368 | *** | 0.278 | 0.055 | 0.102 | 0.524 | ||
Th1 | T-bet (TBX21) | 0.287 | *** | 0.261 | *** | 0.279 | 0.0545 | 0.268 | 0.0869 | |
STAT4 | 0.396 | *** | 0.382 | *** | 0.353 | 0.014 | 0.32 | 0.0415 | ||
STAT1 | 0.055 | 0.26 | 0.047 | 0.361 | 0.19 | 0.196 | 0.142 | 0.377 | ||
IFN-γ (IFNG) | −0.043 | 0.38 | −0.066 | 0.198 | 0.175 | 0.235 | 0.133 | 0.406 | ||
TNF-α (TNF) | 0.074 | 0.133 | 0.042 | 0.42 | 0.311 | 0.0315 | 0.179 | 0.262 | ||
Th2 | GATA3 | 0.403 | *** | 0.394 | *** | 0.202 | 0.169 | 0.158 | 0.324 | |
STAT6 | 0.251 | *** | 0.261 | *** | 0.593 | *** | 0.554 | ** | ||
STAT5A | 0.423 | *** | 0.414 | *** | 0.016 | 0.915 | −0.18 | 0.26 | ||
IL13 | 0.15 | * | 0.151 | * | 0.104 | 0.438 | 0.262 | 0.0978 | ||
Tfh | BCL6 | 0.537 | *** | 0.528 | *** | 0.106 | 0.472 | 0.258 | 0.104 | |
IL21 | 0.102 | 0.0369 | 0.079 | 0.126 | 0.094 | 0.527 | 0.088 | 0.586 | ||
Th17 | STAT3 | 0.429 | *** | 0.421 | *** | 0.477 | ** | 0.352 | 0.0239 | |
IL17A | −0.197 | *** | −0.212 | *** | 0.028 | 0.85 | −0.048 | 0.767 | ||
Treg | FOXP3 | 0.243 | *** | 0.214 | *** | 0.194 | 0.187 | 0.125 | 0.435 | |
CCR8 | 0.398 | *** | 0.389 | *** | 0.225 | 0.124 | 0.22 | 0.166 | ||
STAT5B | 0.604 | *** | 0.589 | *** | 0.341 | 0.176 | 0.224 | 0.159 | ||
TGFβ (TGFB1) | 0.496 | *** | 0.482 | *** | 0.418 | * | 0.286 | 0.0694 | ||
T cell exhaustion | PD-1 (PDCD1) | 0.149 | * | 0.116 | 0.0235 | 0.149 | 0.312 | 0.097 | 0.546 | |
CTLA4 | 0.12 | 0.0147 | 0.091 | 0.0769 | 0.136 | 0.357 | 0.132 | 0.412 | ||
LAG3 | 0.094 | 0.0558 | 0.061 | 0.236 | 0.102 | 0.491 | 0.098 | 0.542 | ||
TIM-3 (HAVCR2) | 0.32 | *** | 0.31 | *** | 0.232 | 0.112 | 0.234 | 0.141 | ||
GZMB | 0.041 | 0.401 | −0.086 | 0.0935 | 0.031 | 0.835 | 0.024 | 0.881 |
*P<0.01; **P<0.001; ***P<0.0001. PGR, progesterone receptor gene; STAD, stomach adenocarcinoma; DLBC, diffuse large B-cell lymphoma; TAM, tumor-associated macrophage; Th, T helper cell; Tfh, follicular helper T cell; Treg, regulatory T cell; Cor, r value of Spearman’s correlation; None, correlation without adjustment. Purity, correlation adjusted by purity.
Among these markers, PGR expression was strongly related to most of the markers of TAMs, M1 macrophages, and M2 macrophages in STAD, especially CD86, CD115, CCL2, CD68, IL10, IRF5, COX2, CD163, VSIG4 and MS4A4A (Figure S4A,B,C,D,E,F,G,H). We further analyzed PGR expression with these markers in STAD via the GEPIA database and similar results were found (Table 3). Also, elevated PGR expression was related to most markers of DCs and Tregs in STAD, including HLA-DPB1, HLA-DQB1, HLA-DRA, HLA-DPA1, BDCA-1, BDCA-4, CD11c, FOXP3, CCR8, STAT5B, and TGFβ (Tables 2,3). Therefore, our findings suggested that PGR expression may affect the immune mechanism and microenvironment of GC.
Table 3
Description | Gene markers | STAD | ||||
---|---|---|---|---|---|---|
Tumor | Normal | |||||
R | P | R | P | |||
Monocyte | CD86 | 0.31 | *** | −0.08 | 0.64 | |
CD115 (CSF1R) | 0.45 | *** | 0.31 | 0.065 | ||
TAM | CCL2 | 0.41 | *** | 0.61 | *** | |
CD68 | 0.18 | ** | −0.41 | 0.013 | ||
IL10 | 0.36 | *** | 0.17 | 0.32 | ||
M1 Macrophage | INOS (NOS2) | 0.02 | 0.69 | 0.26 | 0.12 | |
IRF5 | 0.23 | *** | −0.42 | 0.011 | ||
COX2 (PTGS2) | 0.27 | *** | 0.88 | *** | ||
M2 Macrophage | CD163 | 0.32 | *** | 0.71 | *** | |
VSIG4 | 0.36 | *** | 0.55 | ** | ||
MS4A4A | 0.44 | *** | 0.64 | *** | ||
Dendritic cell | HLA-DPB1 | 0.25 | *** | −0.11 | 0.53 | |
HLA-DQB1 | 0.11 | 0.03 | −0.29 | 0.089 | ||
HLA-DRA | 0.19 | ** | −0.21 | 0.22 | ||
HLA-DPA1 | 0.24 | *** | −0.13 | 0.46 | ||
BDCA-1 (CD1C) | 0.49 | *** | −0.014 | 0.94 | ||
BDCA-4 (NRP1) | 0.54 | *** | 0.87 | *** | ||
CD11c (ITGAX) | 0.35 | *** | 0.19 | 0.26 | ||
Treg | FOXP3 | 0.21 | *** | −0.45 | * | |
CCR8 | 0.38 | *** | −0.27 | 0.11 | ||
STAT5B | 0.57 | *** | 0.9 | *** | ||
TGFβ (TGFB1) | 0.47 | *** | 0.28 | 0.092 |
*P<0.01; **P<0.001; ***P<0.0001. PGR, progesterone receptor gene; STAD, stomach adenocarcinoma; DLBC, diffuse large B-cell lymphoma; TAM, tumor-associated macrophage; Treg, regulatory T cell; Cor, r value of Spearman’s correlation.
Discussion
PGR has been well recognized as the gene that encodes the PR to mediate the actions of the progesterone in preparation and completion of pregnancy (38). Emerging evidences has shown the distinct roles of progesterone and PR in the pathogenesis of multiple types of cancers (39). In this study, we found that PGR expression levels were related to the prognosis of different cancers and elevated PGR expression had a strong relationship with a poorer prognosis of GC. Also, PGR expression affected lymph node metastasis of GC patients, and it was observed that immune cell infiltration, as well as immune markers, were related to PGR expression. These results suggested that PGR is a potential prognostic biomarker in relation to immune cell infiltration in GC.
In this study, we investigated the PGR expression and its association with clinical prognosis in different cancers. Progesterone, mediated by the PR, has diverse effects on multiple tissues and organs and therefore influence different types of cancer such as breast and endometrial cancer (40,41). In this study, we found that PGR expression levels were significantly different in various cancer tissues in the Oncomine and TCGA databases when compared with normal tissues, indicating that PGR may affect the pathogenesis of multiple types of cancer (Figure S1). Several researchers have found that the PR can be used as a favorable prognostic predictor in ovarian, prostate and breast cancers (42-44). However, until now, the prognostic role of PGR in GC has been controversial (45-49). In this study, analysis of the prognostic relationships between PGR and cancer patients’ survival indicated that PGR expression was correlated with poorer prognosis of multiple cancers including ACC, MESO and STAD, and with better prognosis of BRCA, KIRC, LGG, LIHC, SARC and UCEC (Figure 1). Following this result, the Kaplan-Meier plotter database analysis showed that elevated PGR expression correlated with poor OS and PFS in GC (Figure 2). As shown in Table 1, high expression PGR was significantly related to different clinicopathological factors of GC, including stages 2–4, stage T2–T4, stage N0–N3, and stage M0, and the highest OS and PFS HR values were observed in stage N1. Taken together, these results indicated that PGR might be a prognostic predictor of GC.
Tumor-infiltrating immune cells, including macrophages, DCs, and Tregs, actively participate in tumor progression and metastatic processes (50). Previous reports have shown that progesterone and the PR elicit various functional effects on immune cell types, including DCs, monocytes, lymphocytes, and macrophages (51). However, the mechanism of PGR in immune cell infiltration regulation of cancer is still largely uncharacterized. Our current study found that PGR expression correlated with immune cell infiltration in different cancers, especially in GC. PGR expression in STAD was significantly correlated with levels of B cells, CD8+ T cells, CD4+ T cells, macrophages, neutrophils, and DCs, suggesting PGR might influence immune cell infiltration and the tumor microenvironment in STAD (Figure 3).
Further, the correlation between the expression of PGR and various immune cell markers was analyzed. Macrophages play an important role in carcinogenesis, and TAMs are active in promoting solid-tumor metastasis (52). TAM infiltration into GC tissue is positively correlated with cancer invasion, nodal status, and clinical stage (53). The PR was reported to modulate the differentiation of macrophages into M1 or M2 phenotypes (51). A strong and positive correlation between TAMs, M1 and M2 macrophage markers, including CD86, CD115, CCL2, CD68, IL10, IRF5, COX2, CD163, VSIG4 and MS4A4A, and PGR expression in STAD was found in this study (Table 2, Figure S4). This suggested that PGR may influence TAM polarization in cancer, resulting in the promotion of tumor proliferation.
Treg cells are closely associated with the progress of a variety of cancers (54). FOXP3+Tregs are reported to be closely related to the incidence and development of GC, and increase FOXP3+Tregs infiltration in GC tissues can predict poor prognosis (55). FOXP3, a key protein in Treg differentiation and function, may influence tumor differentiation and immune escape in GC patients (56). In this study, we found that PGR expression was positively related to markers of Tregs, such as FOXP3, CCR8, STAT5B, and TGFB1 (Tables 2,3). This result indicated that PGR could activate the Treg response in GC, promoting tumor pathogenesis. These findings suggested that PGR has the potential to play a role in regulating immune cell infiltration in STAD, and the relationship between immune cell infiltration and immune markers with PGR expression may partly explain the correlation of PGR expression with poor prognosis in STAD.
Conclusions
We found that elevated PGR expression was related to poor prognosis and immune cell infiltration in GC, indicating that PGR is a potential prognostic biomarker in GC patients.
Acknowledgments
Funding: This work is supported by
Footnote
Reporting Checklist: The authors have completed the MDAR reporting checklist. Available at https://dx.doi.org/10.21037/tcr-21-218
Conflicts of Interest: Both authors have completed the ICMJE uniform disclosure form (available at https://dx.doi.org/10.21037/tcr-21-218). The authors have no conflicts of interest to declare.
Ethical Statement: The authors are accountable for all aspects of the work in ensuring that questions related to the accuracy or integrity of any part of the work are appropriately investigated and resolved. The study was conducted in accordance with the Declaration of Helsinki (as revised in 2013).
Open Access Statement: This is an Open Access article distributed in accordance with the Creative Commons Attribution-NonCommercial-NoDerivs 4.0 International License (CC BY-NC-ND 4.0), which permits the non-commercial replication and distribution of the article with the strict proviso that no changes or edits are made and the original work is properly cited (including links to both the formal publication through the relevant DOI and the license). See: https://creativecommons.org/licenses/by-nc-nd/4.0/.
References
- Ferlay J, Soerjomataram I, Dikshit R, et al. Cancer incidence and mortality worldwide: sources, methods and major patterns in GLOBOCAN 2012. Int J Cancer 2015;136:E359-86. [Crossref] [PubMed]
- Bennett MW, O'Connell J, O'Sullivan GC, et al. Expression of Fas ligand by human gastric adenocarcinomas: a potential mechanism of immune escape in stomach cancer. Gut 1999;44:156-62. [Crossref] [PubMed]
- Zhang TT, Yuan XL, Li MX, et al. Mechanism of the immune suppression mediated by regulatory T cells in the microenvironment of gastric cancer. 2010.
- Coutzac C, Pernot S, Chaput N, et al. Immunotherapy in advanced gastric cancer, is it the future? Crit Rev Oncol Hematol 2019;133:25-32. [Crossref] [PubMed]
- Ribas A, Wolchok JD. Cancer immunotherapy using checkpoint blockade. Science 2018;359:1350-5. [Crossref] [PubMed]
- Bang YJ, Ruiz EY, Van Cutsem E, et al. Phase III, randomised trial of avelumab versus physician's choice of chemotherapy as third-line treatment of patients with advanced gastric or gastro-oesophageal junction cancer: primary analysis of JAVELIN Gastric 300. Ann Oncol 2018;29:2052-60. [Crossref] [PubMed]
- Le DT, Uram JN, Wang H, et al. PD-1 Blockade in Tumors with Mismatch-Repair Deficiency. N Engl J Med 2015;372:2509-20. [Crossref] [PubMed]
- Wu T, Dai Y. Tumor microenvironment and therapeutic response. Cancer Lett 2017;387:61-8. [Crossref] [PubMed]
- Cavnar MJ, Turcotte S, Katz SC, et al. Tumor-Associated Macrophage Infiltration in Colorectal Cancer Liver Metastases is Associated With Better Outcome. Ann Surg Oncol 2017;24:1835-42. [Crossref] [PubMed]
- Katz SC, Bamboat ZM, Maker AV, et al. Regulatory T cell infiltration predicts outcome following resection of colorectal cancer liver metastases. Ann Surg Oncol 2013;20:946-55. [Crossref] [PubMed]
- Waniczek D, Lorenc Z, Śnietura M, et al. Tumor-Associated Macrophages and Regulatory T Cells Infiltration and the Clinical Outcome in Colorectal Cancer. Arch Immunol Ther Exp (Warsz) 2017;65:445-54. [Crossref] [PubMed]
- Matsuoka T, Yashiro M. Biomarkers of gastric cancer: Current topics and future perspective. World J Gastroenterol 2018;24:2818-32. [Crossref] [PubMed]
- Matsuoka T, Yashiro M. The Role of PI3K/Akt/mTOR Signaling in Gastric Carcinoma. Cancers (Basel) 2014;6:1441-63. [Crossref] [PubMed]
- Chandra Gupta S, Nandan Tripathi Y. Potential of long non-coding RNAs in cancer patients: From biomarkers to therapeutic targets. Int J Cancer 2017;140:1955-67. [Crossref] [PubMed]
- Gu L, Chen M, Guo D, et al. PD-L1 and gastric cancer prognosis: A systematic review and meta-analysis. PLoS One 2017;12:e0182692. [Crossref] [PubMed]
- Yuan C, Wang C, Liu X, et al. Analyze association of the progesterone receptor gene polymorphism PROGINS with ovarian cancer risk. Mol Biol Rep 2013;40:6001-10. [Crossref] [PubMed]
- Marquina-Sánchez B, González-Jorge J, Hansberg-Pastor V, et al. The interplay between intracellular progesterone receptor and PKC plays a key role in migration and invasion of human glioblastoma cells. J Steroid Biochem Mol Biol 2017;172:198-206. [Crossref] [PubMed]
- Richer JK, Jacobsen BM, Manning NG, et al. Differential gene regulation by the two progesterone receptor isoforms in human breast cancer cells. J Biol Chem 2002;277:5209-18. [Crossref] [PubMed]
- Hall OJ, Nachbagauer R, Vermillion MS, et al. Progesterone-Based Contraceptives Reduce Adaptive Immune Responses and Protection against Sequential Influenza A Virus Infections. J Virol 2017;91:e02160-16. [Crossref] [PubMed]
- Lee E, Hsu C, Haiman CA, et al. Genetic variation in the progesterone receptor gene and risk of endometrial cancer: a haplotype-based approach. Carcinogenesis 2010;31:1392-9. [Crossref] [PubMed]
- Johnatty SE, Spurdle AB, Beesley J, et al. Progesterone receptor polymorphisms and risk of breast cancer: results from two Australian breast cancer studies. Breast Cancer Res Treat 2008;109:91-9. [Crossref] [PubMed]
- Wu CW, Chang HM, Kao HL, et al. The nontransformed progesterone and estrogen receptors in gastric cancer. Gastroenterology 1992;102:1639-46. [Crossref] [PubMed]
- Song MJ, Park YS, Song HJ, et al. Prognosis of Pregnancy-Associated Gastric Cancer: An Age-, Sex-, and Stage-Matched Case-Control Study. Gut Liver 2016;10:731-8. [Crossref] [PubMed]
- Hughes GC, Clark EA, Wong AH. The intracellular progesterone receptor regulates CD4+ T cells and T cell-dependent antibody responses. J Leukoc Biol 2013;93:369-75. [Crossref] [PubMed]
- Dziobek K, Biedka M, Nowikiewicz T, et al. Analysis of Treg cell population in patients with breast cancer with respect to progesterone receptor status. Contemp Oncol (Pozn) 2018;22:236-9. [Crossref] [PubMed]
- Wang Y, Sun J, Zheng R, et al. Regulatory T cells are an important prognostic factor in breast cancer: a systematic review and meta-analysis. Neoplasma 2016;63:789-98. [Crossref] [PubMed]
- Rhodes DR, Kalyana-Sundaram S, Mahavisno V, et al. Oncomine 3.0: genes, pathways, and networks in a collection of 18,000 cancer gene expression profiles. Neoplasia 2007;9:166-80. [Crossref] [PubMed]
- Mizuno H, Kitada K, Nakai K, et al. PrognoScan: a new database for meta-analysis of the prognostic value of genes. BMC Med Genomics 2009;2:18. [Crossref] [PubMed]
- Lánczky A, Nagy Á, Bottai G, et al. miRpower: a web-tool to validate survival-associated miRNAs utilizing expression data from 2178 breast cancer patients. Breast Cancer Res Treat 2016;160:439-46. [Crossref] [PubMed]
- Li T, Fan J, Wang B, et al. TIMER: A Web Server for Comprehensive Analysis of Tumor-Infiltrating Immune Cells. Cancer Res 2017;77:e108-10. [Crossref] [PubMed]
- Danaher P, Warren S, Dennis L, et al. Gene expression markers of Tumor Infiltrating Leukocytes. J Immunother Cancer 2017;5:18. [Crossref] [PubMed]
- Siemers NO, Holloway JL, Chang H, et al. Genome-wide association analysis identifies genetic correlates of immune infiltrates in solid tumors. PLoS One 2017;12:e0179726. [Crossref] [PubMed]
- Tang Z, Li C, Kang B, et al. GEPIA: a web server for cancer and normal gene expression profiling and interactive analyses. Nucleic Acids Res 2017;45:W98-W102. [Crossref] [PubMed]
- Gotoda T, Yanagisawa A, Sasako M, et al. Incidence of lymph node metastasis from early gastric cancer: estimation with a large number of cases at two large centers. Gastric Cancer 2000;3:219-25. [Crossref] [PubMed]
- Symeonidis D, Diamantis A, Bompou E, et al. Current role of lymphadenectomy in gastric cancer surgery. J BUON 2019;24:1761-7. [PubMed]
- Azimi F, Scolyer RA, Rumcheva P, et al. Tumor-infiltrating lymphocyte grade is an independent predictor of sentinel lymph node status and survival in patients with cutaneous melanoma. J Clin Oncol 2012;30:2678-83. [Crossref] [PubMed]
- Santoiemma PP, Powell DJ Jr. Tumor infiltrating lymphocytes in ovarian cancer. Cancer Biol Ther 2015;16:807-20. [Crossref] [PubMed]
- Wu SP, Li R, DeMayo FJ. Progesterone Receptor Regulation of Uterine Adaptation for Pregnancy. Trends Endocrinol Metab 2018;29:481-91. [Crossref] [PubMed]
- Check JH. The role of progesterone and the progesterone receptor in cancer. Expert Rev Endocrinol Metab 2017;12:187-97. [Crossref] [PubMed]
- Kim JJ, Kurita T, Bulun SE. Progesterone action in endometrial cancer, endometriosis, uterine fibroids, and breast cancer. Endocr Rev 2013;34:130-62. [Crossref] [PubMed]
- Mulac-Jericevic B, Mullinax RA, DeMayo FJ, et al. Subgroup of reproductive functions of progesterone mediated by progesterone receptor-B isoform. Science 2000;289:1751-4. [Crossref] [PubMed]
- Luo H, Li S, Zhao M, et al. Prognostic value of progesterone receptor expression in ovarian cancer: a meta-analysis. Oncotarget 2017;8:36845-56. [Crossref] [PubMed]
- Purdie CA, Quinlan P, Jordan LB, et al. Progesterone receptor expression is an independent prognostic variable in early breast cancer: a population-based study. Br J Cancer 2014;110:565-72. [Crossref] [PubMed]
- Grindstad T, Andersen S, Al-Saad S, et al. High progesterone receptor expression in prostate cancer is associated with clinical failure. PLoS One 2015;10:e0116691. [Crossref] [PubMed]
- Cho LY, Yang JJ, Ko KP, et al. Genetic susceptibility factors on genes involved in the steroid hormone biosynthesis pathway and progesterone receptor for gastric cancer risk. PLoS One 2012;7:e47603. [Crossref] [PubMed]
- Gan L, He J, Zhang X, et al. Expression profile and prognostic role of sex hormone receptors in gastric cancer. BMC Cancer 2012;12:566. [Crossref] [PubMed]
- Tan X, Wu X, Ren S, et al. A Point Mutation in DNA Polymerase β (POLB) Gene Is Associated with Increased Progesterone Receptor (PR) Expression and Intraperitoneal Metastasis in Gastric Cancer. J Cancer 2016;7:1472-80. [Crossref] [PubMed]
- Matsui M, Kojima O, Kawakami S, et al. The prognosis of patients with gastric cancer possessing sex hormone receptors. Surg Today 1992;22:421-5. [Crossref] [PubMed]
- Oshima CT, Wonraht DR, Catarino RM, et al. Estrogen and progesterone receptors in gastric and colorectal cancer. Hepatogastroenterology 1999;46:3155-8. [PubMed]
- Kitamura T, Qian BZ, Pollard JW. Immune cell promotion of metastasis. Nat Rev Immunol 2015;15:73-86. [Crossref] [PubMed]
- Shah NM, Lai PF, Imami N, et al. Progesterone-Related Immune Modulation of Pregnancy and Labor. Front Endocrinol (Lausanne) 2019;10:198. [Crossref] [PubMed]
- Sica A, Schioppa T, Mantovani A, et al. Tumour-associated macrophages are a distinct M2 polarised population promoting tumour progression: potential targets of anti-cancer therapy. Eur J Cancer 2006;42:717-27. [Crossref] [PubMed]
- Ishigami S, Natsugoe S, Tokuda K, et al. Tumor-associated macrophage (TAM) infiltration in gastric cancer. Anticancer Res 2003;23:4079-83. [PubMed]
- Sakaguchi S, Yamaguchi T, Nomura T, et al. Regulatory T cells and immune tolerance. Cell 2008;133:775-87. [Crossref] [PubMed]
- Liu X, Zhang Z, Zhao G. Recent advances in the study of regulatory T cells in gastric cancer. Int Immunopharmacol 2019;73:560-7. [Crossref] [PubMed]
- Jiang C, Wang W, Yan W, et al. Clinical implications and characteristics of factor forkhead box protein 3 in gastric cancer. Exp Ther Med 2011;2:667-73. [Crossref] [PubMed]