Identification of key mutations in central nervous diffuse large B-cell lymphoma (DLBCL) by comprehensive analysis between sequencing and TCGA database
Introduction
Diffuse large B-cell lymphoma (DLBCL) is the most common type of non-Hodgkin’s lymphoma, accounting for approximately 40% of the adult cases (1). Due to the considerable heterogeneity of pathology in various DLBCLs, patients exhibit different responses to treatment and prognosis (2). Therefore, a comprehensive understanding of DLBCL by the gene expression profile is necessary. Although several studies have identified some critical mutant genes of DLBCL, including EZH2, FOXP1, and TP53 (3-5), the disease in the central nervous system (CNS) is yet to be elucidated. Actually, the CNS DLBCL, to some degree is different from DLBCL in many aspects including original cells, pathological characteristics and pathogenic gene. Interestingly, CNS DLBCL, especially the primary CNS lymphoma (PCNSL) is a B-cell lymphoma in the CNS where very few B lymphocytes, if any, are found under normal circumstances (6). Some studies have indicated that PCNSL is of germinal center B cell (GCB) origin (2,3). According to a gene expression study, non-CNS DLBCL has been classified into three groups: GCB type, activated B cell (ABC) type, and type 3 (7,8). Studies have found that some gene mutations are closely related to the occurrence and development of CNS DLBCL, such as the deletion of human leukocyte antigen (HLA) epitopes on chromosome p21 and the deletion of chromosomes 6q21-6q25. B cell differentiation regulator, tumor suppressor PRDM1, tyrosine phosphatase PTPRK, and MyD88 have also been identified as the critical mutations (9-12). However, due to the different research background, race and sample collection method, many studies usually obtained widely varied results.
Thus, in the present study, we performed a genome-wide sequencing of 12 cases of DLBCL in the CNS and analyzed their characteristics to find some critical mutations involved in the development of the disease. In addition, we analyzed the TCGA database and compared the findings with the results of gene sequencing; finally, four mutations that overlapped in both methods were identified. We present the following article in accordance with the MDAR reporting checklist (available at https://dx.doi.org/10.21037/tcr-20-2525).
Methods
Patients
A total of 156 cases of central nervous tumors at the Naval General Hospital were collected from 2016 to 2018. All patients approved the specimen collection and experimental analysis. According to the classification criteria of 2016 WHO, DLBCL was identified through H&E staining and immunohistochemistry by two senior pathologists who specialized in lymphoma. Finally, 12 cases of tumor tissues with positive expression of CD10, Bcl-6, and MUM1 were selected, and the adjacent tissues were used as controls. The study was conducted in accordance with the Declaration of Helsinki (as revised in 2013). The study was approved by the Ethics Committee of Chinese Naval General Hospital (NGH31278239). This study was retrospectively registered and all participants consented to this trial.
Immunohistochemical staining and immunophenotyping
All specimens were embedded in paraffin, sliced into 4-µM sections, and stained by H&E. The immunophenotype of the tumor and background cells was detected by ChemMate EnVisionTM kit. After antigen retrieval, the sections were probed with the antibodies against CD20 (1:800, Catalog number: #48750), CD79a (1:100, Catalog number: #13333), CD3 (1:100, Catalog number: #85061), CD45RO (1:800, Catalog number: #55618), Bcl-6 (1:10, Catalog number: #89369), CD10 (1:200, Catalog number: #PA0131), Bcl-2 (1:50, Catalog number: #15071), CD30 (1:200, Catalog number: #54535), ALK (1:25, Catalog number: #3633), MUM1 (1:400, Catalog number: #62834), and CD138 (1:100, Catalog number: #12922), and developed using automated immunohistochemistry instrument (Dako). Except for CD10 (Novocastra), all the antibodies were obtained from CST with positive and negative controls. According to Hans’ typing method (13), DLBCL could be divided into GCB subtypes and non-GCB subtypes, among which, CD10(+)/bcl-6(+/−)/MUM1(+/−) and CD10(−)/bcl-6(+)/MUM1(−) are classified into GCB subtype, and the rest are non-GCB subtypes.
Sequencing experiment
Our panel was designed to encompass the whole exon regions (including parts of the intron regions). The TruSeq Custom Panel (Kaiao, Beijing, China) was designed using the online software Design Studio, which consisted of 291 pairs of probes. All regions were designed to bind the genomic target sequences of interest, spanning a length of 41.27 kb of genomic DNA. The panel used to sequence the patients with a diagnosis of DLBCL encompassed the complete exon regions of the genes. Referring to this 20-gene panel, a total of 531 amplicons targeting 338 coding sequences, 72.358 kbp size and 99.79% coverage, were designed using the Ion Torrent S5 sequencing platform. All specimen was tested three times.
Data analysis
The original data were analyzed by GCOS 1.2 software to evaluate the results of a single chip that were was input into GeneSprin97.2 for further analysis. The probe group with original signal value <50 in the 12 samples was removed and subjected to one-way ANOVA. Subsequently, the expression probe group with >2-fold the change in the expression level between any two groups (P<0.01) was selected, and the unsupervised hierarchical cluster analysis was performed according to these probe groups to divide the cases into four groups: A, B, C, and D. K indicated that the clustering method was used to analyze the genes with consistent expression trend in each group. According to Guoling You’s method (14). Reads that matched exonic regions, including exon-intron boundaries, were analyzed. Single nucleotide variants (SNVs) and insertions/deletions (Indels) were analyzed using different filtering steps. Genes with at least one heterozygous change in the DNA sequence were considered to be the most likely to cause disease. In each case, all variants listed in the most recent version of the National Center for Biotechnology Information (NCBI) single nucleotide polymorphism (SNP) database (dbSNP) were excluded. Silent mutations were also excluded. Low-frequency frameshift and truncating mutations were considered pathogenic. In addition, genes that were expressed in one of the samples and not expressed in the other two groups were also screened for specific expression in each group. All the screened genes were classified according to the biological functions and then categorized into their respective signaling pathways. Gene chip manipulation and data analysis personnel were blinded to the case immunophenotyping. Furthermore, enrichment annotation of unique Entrez Gene IDs using the selected functional categories, Gene Set Enrichment Analysis (GSEA) of Gene Ontology (GO) and Kyoto Encyclopedia of Genes and Genomes (KEGG) were performed using the Gene Set Analysis Toolkit (Gestalt, http://bioinfo.vanderbilt.edu/webgestalt), and the pathway files were compiled from the KEGG pathway (http://www.genome.jp/kegg/pathway.html).
Evaluation of gene expression via TCGA database
TCGA database (https://cancergenome.nih.gov/) has been made publicly available to provide genomic information on 33 types of cancer, which would be valuable for the researchers to improve the diagnosis, treatment, and prevention of the cancers. The gene mutation data of 34 DLBCL cases were downloaded from the database.
Statistical analysis
All data were analyzed using SPSS, version 19.0 software for Windows (SPSS, Chicago, IL, USA, Code OZNINMOO6TT8K87M54YUMJD9Z8YST44XHH99A8M6TE2KJ3) and R version 3.4.1. Continuous variables are presented as the mean and standard deviation. A chi-square test was performed to evaluate the differences in categorical variables among different defined groups. One-way ANOVA was used to determine the differences in numerical variables among the groups.
Results
Clinicopathological characteristics of patients
A total of 156 cases of central nervous tumors were collected from 2016 to 2018. H&E staining and immunohistochemistry revealed that 12 cases were designated as DLBCL, of which 1 case was classified into GCB subtype, and 11 cases were non-GCB subtypes according to CD10, Bcl-6, and MUM1 staining. Each DLBCL specimen of the 12 cases was numbered and named accordingly (Table 1). The cohort consisted of 83.3% (10 cases) males and 16.7% (2 cases) females, suggesting that males may be more susceptible to DLBCL than females (Figure 1A). The age of the patients was 30–70 years old (Figure 1B), with most 41.7% (5 cases) 61–70 years old.
Table 1
No. | Sample name | Pathological diagnosis |
---|---|---|
1 | LAADOE | DLBCL |
2 | LAAEH1 | DLBCL |
3 | LAAFVXT1 | DLBCL |
4 | LBE0702T | DLBCL (derived from germinal center) |
5 | LAAFT4 | DLBCL (right cerebellum) |
6 | LAL2051T | DLBCL |
7 | LBC7255T | DLBCL (derived from peripheral activated B cells) |
8 | LBC6300T | DLBCL |
9 | LBD9753T | DLBCL |
10 | LBD9748T | DLBCL (fourth ventricle) |
11 | LBG4713T | DLBCL (derived from peripheral activated B cells) |
12 | LBG6976T | DLBCL |
DLBCL, diffuse large B-cell lymphoma.
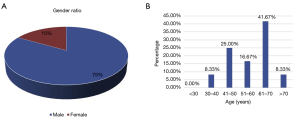
Analysis of the sequencing experiment of 12 cases
The whole-genome high-throughput sequencing results of the 12 clinical samples were analyzed. The gene mutation spectrum demonstrated that the most common substitutions of six single bases were C>T/G>A (Figure 2A,B). Subsequently, the pattern figure was performed, wherein the mutation frequency of C(C>T) G was the highest (Figure 2C). Furthermore, the enrichment analysis (GO and KEGG) of genes with somatic mutations in all samples revealed that the mutated genes are mainly involved in tumor proliferation and metabolism (Figure 2D). The signaling pathways included Wnt, Bcl-2, Rb, and other signaling pathways related to tumor proliferation, apoptosis, and cell cycle (Figure 2E). Finally, the mutations with frequencies significantly higher than the background mutation frequency were identified as significantly mutated genes (SMG), and the heat map was constructed accordingly. The results demonstrated that the most common type of mutation is missense, and the most frequently mutated genes included MYD88, LRP1B, CD79B, GNA13 and PIM1. However, it is worth noting that two samples named LAADOET1 and LBG4G13T didn’t detect the insertion-deletion (InDel) (Figure 2F).
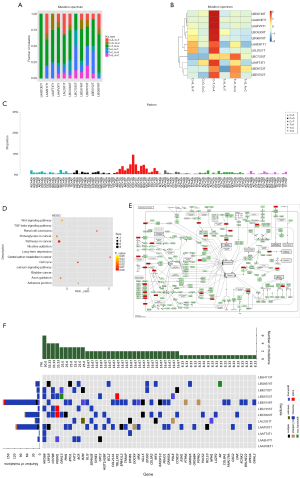
Identification of mutations associated with DLBCL based on TCGA database
In addition to sequencing analysis, we also utilized the TCGA database and analyzed the sequencing results of the DLBCL cases. Firstly, we retrieved all the DLBCL cases (without limitation of the primary site) and identified 34 cases involving 70 SMGs. Next, to specifically compare these results with those of our sequencing experiments, the DLBCL cases limited in the brain were also retrieved from the TCGA database. Consequently, only 2 cases were available, and 12 mutations were identified; hence, all DLBCL cases (without limitation of the primary site) were analyzed. A majority of these cases exhibited missense mutations (Figure 3A), and the most common substitution of the base was C>T/G>A (Figure 3B), which was consistent with the result of our sequencing experiments. Finally, a heat map indicating the SMGs was drawn (Figure 3C), wherein mutations in the PIM1, IRF4, CD79B, and KMT2D genes were overlapped.
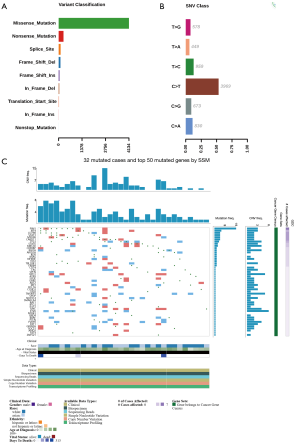
Comparative analysis between results from the sequencing experiment and TCGA database
The sequencing results of the 12 cases of the CNS DLBCL samples and the data analysis from the TCGA database were used for screening many high-frequency mutation genes. However, due to different sources of samples and processing methods, the selected SMGs also varied. Thus, we combined the mutated gene data obtained from 12 samples (Seq-DLBC-Brain) with the sequencing data from the TCGA database (TCGA-DLBC-Full) to identify the mutations in common. Moreover, to match the sequencing results, we also enrolled the data of two cases of DLBCL in the CNSs (TCGA-DLBC-Brain). Based on the above data, four mutations, including those in MYD88, PIM1, CD79B, and BTG1, were identified in common (Figure 4).
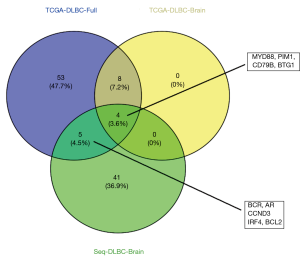
Discussion
In recent years, high-throughput sequencing technology has played a major role in the study of tumors. It has effectively detected mutant genes in tumors, and analyzed the mutations in detail, which has provided valuable clues for the follow-up of tumor treatment (15-17). However, due to the different sources of tumor samples and varied treatment methods, the results of detection by various institutions differ markedly. Therefore, comparing the sequencing results of different institutions to expand the sample size is critical for finding effective target genes (18,19). Aimed at DLBCL, this study firstly analyzed the sequencing results, and the high-frequency mutation gene was selected. These results were combined with the data of the TCGA database, and as a result, and mutant genes in common were identified that were designated as the potential target mutations.
The present study enrolled 12 cases of DLBCL in the CNS. Since only a few reports have specifically studied DLBCL in the CNS, the current data and analysis might provide an important reference for the research of DLBCL in the CNS. Interestingly, 10/12 cases were males, and the age of the cohort was 61–70 years. The results of high-throughput sequencing of the samples revealed that the most common mutations were missense with C>T/G>A, accounting for the highest rate of single base substitutions, which was consistent with the results obtained using the TCGA database. This finding indicated that the base substitution of C>T/G>A is common in DLBCL of the CNS and other tissues.
Furthermore, the screening for SMGs identified the majority of the mutations in MYD88, LRP1B, CD79B, and PRDM1 genes. However, it is worth noting that two samples named LAADOET1 and LBG4G13T didn’t detect the InDel. As the other variation detection including SNV, structural variation (SV) and copy number variation (CNV) all have been identified, therefor the informational loss in InDel may be due to the characteristic of the sample themselves.
In order to screen out the general and the targeted mutant genes, the intersection of the three groups of data, including 12 samples of sequencing experiment, all DLBCL (undefined tissue) data in the TCGA database, and the DLBCL data of the CNS in the TCGA database were analyzed. A pooled analysis indicated four mutant genes in common: MYD88, PIM1, CD79B, and BTG1. MYD88 is a cytosolic adapter protein that plays a central role in innate and adaptive immune responses (20,21). MYD88 mutations have been reported in DLBCL, suggesting its critical role in the development of DLBCL (22). Especially, the MYD88 L265P mutation rate in the CNS and testicular DLBCLs was reported to be 60% [3/5] (23). Although many studies have reported that MYD88 mutation is closely related to the occurrence of DLBCL, the mechanism remains unclear. However, as an adaptor of Toll-like receptors (TLRs), MYD88 mutation may not only affect the proliferation of lymphocyte, but also affect the surrounding immune environment, resulting in inactivation of tumor suppression (24). Zhu et al. (25) reported that a long-chain non-coding RNA, SNHG16, could promote the growth of DLBCL by up-regulating the expression of PIM1 via the miR-497-5p/PIM1 axis, thereby indicating that PIM1 may promote the proliferation of DLBCL. Takeuchi et al. (26) demonstrated that the CD79B mutation was also an important marker of CD5-positive DLBCL, and this mutation has been found in various tissues of DLBCL (27,28). B-cell translocation gene 1 (BTG1) is a member of the BTG/transducer of the Erb family. Previous studies have detected BTG1 mutations in DLBCL; however, the cases of DLBCL in the CNS have not been reported. The above results indicated that the mutant genes screened by sequencing and TCGA data had a correlation with DLBCL.
Though carried out at different background and population, the results of our studies conform to many studies, including Pouzoulet et al. (29). Klanova et al. (30), Schmitz et al. (31), Chapuy et al. (32), Wright et al. (33). Among them, Pouzoulet collected six cases of PCNSL, and the CD79A, CD79B, PIM1, and Card11 were the most significant mutation. The study of Klanova identified the CDKN2A, MYD88, and CD79B as the most prominent mutation in PCNS. Our study identified MYD88, PIM1, CD79B, and BTG1 as an important mutation gene by sequencing combining the analysis of TCGA database. Together above results, there are many similarities between our and other studies, while some different mutations also identified. All these findings provide important view for comprehensive understanding the relationship between CNS DLBCL and genetic mutation.
Taken together, the present study identified some novel and vital mutations to specifically indicate DLBCL in the CNS and subsequently provided potential treatment targets. Although four mutations detected in common between sequencing experiments and TCGA database were selected, there is no mutation could be associated with survival. Hence, additional clinical studies are essential for the identification of specific mutations.
Acknowledgments
Funding: None.
Footnote
Reporting Checklist: The authors have completed the MDAR reporting checklist. Available at https://dx.doi.org/10.21037/tcr-20-2525
Data Sharing Statement: Available at https://dx.doi.org/10.21037/tcr-20-2525
Peer Review File: Available at https://dx.doi.org/10.21037/tcr-20-2525
Conflicts of Interest: All authors have completed the ICMJE uniform disclosure form (available at http://dx.doi.org/10.21037/tcr-20-2525). The authors have no conflicts of interest to declare.
Ethical Statement: The authors are accountable for all aspects of the work in ensuring that questions related to the accuracy or integrity of any part of the work are appropriately investigated and resolved. The study was conducted in accordance with the Declaration of Helsinki (as revised in 2013). The study was approved by the Ethics Committee of Chinese Naval General Hospital (NGH31278239). This study was retrospectively registered and all participants consented to this trial.
Open Access Statement: This is an Open Access article distributed in accordance with the Creative Commons Attribution-NonCommercial-NoDerivs 4.0 International License (CC BY-NC-ND 4.0), which permits the non-commercial replication and distribution of the article with the strict proviso that no changes or edits are made and the original work is properly cited (including links to both the formal publication through the relevant DOI and the license). See: https://creativecommons.org/licenses/by-nc-nd/4.0/.
References
- Li S, Young KH, Medeiros LJ. Diffuse large B-cell lymphoma. Pathology 2018;50:74-87. [Crossref] [PubMed]
- Tang H, Zhou H, Wei J, et al. Clinicopathologic significance and therapeutic implication of de novo CD5+ diffuse large B-cell lymphoma. Hematology 2019;24:446-54. [Crossref] [PubMed]
- Liu Y, Yu K, Li M, et al. EZH2 overexpression in primary gastrointestinal diffuse large B-cell lymphoma and its association with the clinicopathological features. Hum Pathol 2017;64:213-21. [Crossref] [PubMed]
- Gascoyne DM, Banham AH. The significance of FOXP1 in diffuse large B-cell lymphoma. Leuk Lymphoma 2017;58:1037-51. [Crossref] [PubMed]
- Voropaeva EN, Pospelova TI, Voevoda MI, et al. Clinical aspects of TP53 gene inactivation in diffuse large B-cell lymphoma. BMC Med Genomics 2019;12:35. [Crossref] [PubMed]
- Anthony IC, Crawford DH, Bell JE. B lymphocytes in the normal brain: contrasts with HIV-associated lymphoid infiltrates and lymphomas. Brain 2003;126:1058-67. [Crossref] [PubMed]
- Braaten KM, Betensky RA, de Leval L, et al. BCL-6 expression predicts improved survival in patients with primary central nervous system lymphoma. Clin Cancer Res 2003;9:1063-9. [PubMed]
- Larocca LM, Capello D, Rinelli A, et al. The molecular and phenotypic profile of primary central nervous system lymphoma identifies distinct categories of the disease and is consistent with histogenetic derivation from germinal center-related B cells. Blood 1998;92:1011-9. [PubMed]
- Windsor WT, Syto R, Tsarbopoulos A, et al. Disulfide bond assignments and secondary structure analysis of human and murine interleukin 10. Biochemistry 1993;32:8807-15. [Crossref] [PubMed]
- Seki S, Osada S, Ono S, et al. Role of liver NK cells and peritoneal macrophages in gamma interferon and interleukin-10 production in experimental bacterial peritonitis in mice. Infect Immun 1998;66:5286-94. [Crossref] [PubMed]
- Siewe L, Bollati-Fogolin M, Wickenhauser C, et al. Interleukin-10 derived from macrophages and/or neutrophils regulates the inflammatory response to LPS but not the response to CpG DNA. Eur J Immunol 2006;36:3248-55. [Crossref] [PubMed]
- Murray PJ. Understanding and exploiting the endogenous interleukin-10/STAT3-mediated anti-inflammatory response. Curr Opin Pharmacol 2006;6:379-86. [Crossref] [PubMed]
- Hans CP, Weisenburger DD, Greiner TC, et al. Confirmation of the molecular classification of diffuse large B-cell lymphoma by immunohistochemistry using a tissue microarray. Blood 2004;103:275-82. [Crossref] [PubMed]
- You G, Zu B, Wang B, et al. Exome Sequencing Identified a Novel FBN2 Mutation in a Chinese Family with Congenital Contractural Arachnodactyly. Int J Mol Sci 2017;18:626. [Crossref] [PubMed]
- Jiang MF, Li L, Wang Z, et al. Preliminary study of exon sequence in pneumoconiosis and pneumoconiosis complicated with lung cancer using high-throughput sequencing technology. Zhonghua Lao Dong Wei Sheng Zhi Ye Bing Za Zhi 2017;35:801-6. [PubMed]
- Feng T, Zhang P, Sun Y, et al. High throughput sequencing identifies breast cancer-secreted exosomal LncRNAs initiating pulmonary pre-metastatic niche formation. Gene 2019;710:258-64. [Crossref] [PubMed]
- Zhang P, Xia JH, Zhu J, et al. High-throughput screening of prostate cancer risk loci by single nucleotide polymorphisms sequencing. Nat Commun 2018;9:2022. [Crossref] [PubMed]
- Vainshtein Y, Rippe K, Teif VB. NucTools: analysis of chromatin feature occupancy profiles from high-throughput sequencing data. BMC Genomics 2017;18:158. [Crossref] [PubMed]
- Paolillo C, Londin E, Fortina P. Next generation sequencing in cancer: opportunities and challenges for precision cancer medicine. Scand J Clin Lab Invest Suppl 2016;245:S84-91. [Crossref] [PubMed]
- Arnold-Schrauf C, Berod L, Sparwasser T. Dendritic cell specific targeting of MyD88 signalling pathways in vivo. Eur J Immunol 2015;45:32-9. [Crossref] [PubMed]
- Deguine J, Barton GM. MyD88: a central player in innate immune signaling. F1000Prime Rep 2014;6:97. [Crossref] [PubMed]
- Weber ANR, Cardona Gloria Y, Çınar Ö, et al. Oncogenic MYD88 mutations in lymphoma: novel insights and therapeutic possibilities. Cancer Immunol Immunother 2018;67:1797-807. [Crossref] [PubMed]
- Yu S, Luo H, Pan M, et al. High frequency and prognostic value of MYD88 L265P mutation in diffuse large B-cell lymphoma with R-CHOP treatment. Oncol Lett 2018;15:1707-15. [PubMed]
- New M, Sheikh S, Bekheet M, et al. TLR Adaptor Protein MYD88 Mediates Sensitivity to HDAC Inhibitors via a Cytokine-Dependent Mechanism. Cancer Res 2016;76:6975-87. [Crossref] [PubMed]
- Zhu Q, Li Y, Guo Y, et al. Long non-coding RNA SNHG16 promotes proliferation and inhibits apoptosis of diffuse large B-cell lymphoma cells by targeting miR-497-5p/PIM1 axis. J Cell Mol Med 2019;23:7395-405. [Crossref] [PubMed]
- Takeuchi T, Yamaguchi M, Kobayashi K, et al. MYD88, CD79B, and CARD11 gene mutations in CD5-positive diffuse large B-cell lymphoma. Cancer 2017;123:1166-73. [Crossref] [PubMed]
- Taniguchi K, Takata K, Chuang SS, et al. Frequent MYD88 L265P and CD79B Mutations in Primary Breast Diffuse Large B-Cell Lymphoma. Am J Surg Pathol 2016;40:324-34. [Crossref] [PubMed]
- Kraan W, van Keimpema M, Horlings HM, et al. High prevalence of oncogenic MYD88 and CD79B mutations in primary testicular diffuse large B-cell lymphoma. Leukemia 2014;28:719-20. [Crossref] [PubMed]
- Pouzoulet F, Alentorn A, Royer-Perron L, et al. Primary CNS lymphoma patient-derived orthotopic xenograft model capture the biological and molecular characteristics of the disease. Blood Cells Mol Dis 2019;75:1-10. [Crossref] [PubMed]
- Klanova M, Sehn LH, Bence-Bruckler I, et al. Integration of cell of origin into the clinical CNS International Prognostic Index improves CNS relapse prediction in DLBCL. Blood 2019;133:919-26. [Crossref] [PubMed]
- Schmitz R, Wright GW, Huang DW, et al. Genetics and Pathogenesis of Diffuse Large B-Cell Lymphoma. N Engl J Med 2018;378:1396-407. [Crossref] [PubMed]
- Chapuy B, Stewart C, Dunford AJ, et al. Author Correction: Molecular subtypes of diffuse large B cell lymphoma are associated with distinct pathogenic mechanisms and outcomes. Nat Med 2018;24:1290-1. [Crossref] [PubMed]
- Wright GW, Huang DW, Phelan JD, et al. A Probabilistic Classification Tool for Genetic Subtypes of Diffuse Large B Cell Lymphoma with Therapeutic Implications. Cancer Cell 2020;37:551-568.e14. [Crossref] [PubMed]