A nomogram combined with radiomics features, albuminuria, and metabolic syndrome to predict the risk of myometrial invasion of bladder cancer
Introduction
Bladder cancer (BC) is the most common and fatal tumor in the urinary system. Uroepithelioma is the most common histological type of BC, and it is more common in older men (1-3). Studies showed that 70% to 75% of patients with primary BC are limited to mucosa and submucosa, called non-muscular invasive BC (NMIBC). Radiotherapy, chemotherapy, and transurethral cystectomy are often used. The 5-year survival rate is 96% (4).
Nevertheless, about 25% to 30% of patients diagnosed with muscular infiltrating BC (MIBC) are often treated with radical cystectomy. The 5-year survival rate is only 33% and 75% (3,5). Data also showed that about 50% to 70% of patients with NMIBC suffer recurrences of the bladder tumor within 18 months after surgery or even progress to MIBC (6). Therefore, to achieve early preoperative prediction of MIBC, it is critical to select surgical methods and subsequent treatment. At present, there are very few reports predicting the risk of MIBC before surgery, and there are no specific indicators for predicting MIBC. However, there are many studies on the survival and treatment of MIBC. Measurements of PD-L1 and O-6-methylguanine methyltransferase are used in MIBC surgery, demonstrating adequate predictive ability (1,7). At present, the evaluation of preoperative MIBC depends on cystoscopic biopsy; however, this is an invasive examination accompanied by the risk of urethral infection and injury. Furthermore, because of the amount and location of samples, the accuracy of preoperative biopsy is low. Therefore, there is an urgent need to identify a new preoperative non-invasive diagnostic method to detect MIBC.
In recent years, with the development of medical image information processing technology, a large number of radiological features related to specific cancer have been extracted from images to provide clinicians with profound information that the human eye cannot directly obtain; these data assist clinicians in the diagnosis of cancer characteristics, creating an intense area of research (8-10). Lambin first proposed radiomics. The term refers to high-throughput extraction of radiological features converted into computable spatial data and texture classification using supervised or unsupervised methods; the technique has a solid ability to quantify heterogeneity (11). Many studies showed excellent effectiveness in differential diagnosis and pathological grading of diseases through imaging combined with machine learning algorithms (12). Investigators suggested that diffusion weighted imaging (DWI)-based radiological characteristics are independent predictors of progression-free survival (PFS) in MIBC patients (13). Although the role of magnetic resonance imaging (MRI) in the diagnosis of BC has been reported (14), conventional images with echo-plane MRI sequences cannot recognize primary tumors. They may not be accurate in identifying local staging (15). In addition, MRI requires long acquisition times, high economic costs, and involves many scanning parameters, while computed tomography (CT) is faster and less expensive. Enhanced thin-slice CT images can reveal lesions more clearly, and they do not often omit edge texture information. Therefore, in this study, we built a prediction model based on multi-parameter CT combined with clinical risk factors to predict whether BC has invaded the myometrium before surgery accurately. We also developed a nomogram including radiological features and clinical risk factors.
We present the following article in accordance with the TRIPOD reporting checklist (available at https://dx.doi.org/10.21037/tcr-21-426).
Methods
Patients
We gathered data of 100 patients with BC diagnosed after surgery in the first affiliated Hospital of Suzhou University from January 2014 to January 2018, including 50 cases of NMIBC and 50 cases of MIBC. According to the proportion of 7:3,100 patients were randomly divided into training and verification groups. The training group included 70 patients, and the verification group included 30 patients. Of these, 35 patients with MIBC were in the training group and 15 patients with MIBC were in the verification group. The clinical data in the training and verification groups showed no significant differences in the distribution of clinical characteristic variables between the two groups (Table 1, P>0.05).
Table 1
Clinical features | Training group (n=70) | verification group (n=30) | |||||
---|---|---|---|---|---|---|---|
MIBC (n=35) | NMIBC (n=35) | P | MIBC (n=15) | NMIBC (n=15) | P | ||
Age | 70.00±10.99 | 66.2±13.52 | 0.202 | 64.67±10.57 | 64.47±7.01 | 0.952 | |
Gender | 0.042 | 1 | |||||
Male | 31 (88.6) | 24 (68.6) | 12 (80.0) | 12 (80.0) | |||
Female | 4 (11.4) | 11 (31.4) | 3 (20.0) | 3 (20.0) | |||
BMI | 22.49±3.09 | 23.43±2.94 | 0.196 | 25.84±3.90 | 24.03±3.33 | 0.183 | |
Urine PH | 6.16±0.76 | 6.17±0.74 | 0.937 | 6.03±0.69 | 6.03±0.67 | 1 | |
Neutrophils | 3.54±1.22 | 3.64±1.73 | 0.780 | 3.62±0.66 | 3.18±1.05 | 0.181 | |
Lymphocyte | 1.67±0.55 | 1.80±0.64 | 0.388 | 1.93±0.58 | 1.79±0.70 | 0.579 | |
NLR | 2.22±0.78 | 2.27+1.52 | 0.851 | 1.99±0.51 | 2.04±1.12 | 0.047 | |
Urinary infection | 0.019 | 0.716 | |||||
Yes | 29 (82.9) | 20 (57.1) | 9 (60.0) | 10 (66.7) | |||
No | 6 (17.1) | 15 (42.9) | 6 (40.0) | 5 (33.3) | |||
Hematuria | 0.364 | 0.702 | |||||
Yes | 30 (85.7) | 27 (77.1) | 10 (66.7) | 11 (73.3) | |||
No | 5 (14.3) | 8 (22.9) | 5 (33.3) | 4 (26.7) | |||
Proteinuria | 0.008* | 0.028* | |||||
Yes | 23 (65.7) | 12 (34.3) | 10 (66.7) | 4 (26.7) | |||
No | 12 (34.3) | 23 (65.7) | 5 (33.3) | 11 (73.3) | |||
Number | 0.502 | 1 | |||||
Single | 31 (88.6) | 29 (82.9) | 14 (93.3) | 14 (93.3) | |||
Multiple (≥2) | 4 (11.4) | 6 (17.1) | 1 (6.7) | 1 (6.7) | |||
Diameter (mm) | 28.58±13.50 | 20.63±11.29 | 0.009* | 30.80±17.00 | 14.40±3.54 | 0.001* | |
MS | 0.006* | 0.002* | |||||
Yes | 18 (51.4) | 7 (20.0) | 10 (66.7) | 2 (13.3) | |||
No | 17 (48.6) | 28 (80.0) | 5 (33.3) | 13 (86.7) | |||
HBP | 0.032 | 0.726 | |||||
Yes | 22 (62.9) | 13 (37.1) | 7 (46.7) | 8 () | |||
No | 13 (37.1) | 22 (62.9) | 8 (53.3) | 7 () | |||
Triglyceride | 1.49±0.87 | 1.37±0.66 | 0.510 | 1.82±0.91 | 1.81±1.18 | 0.985 | |
HDL-C | 1.21±0.29 | 1.31±0.58 | 0.368 | 1.14±0.25 | 1.14±0.24 | 0.988 | |
Diabetes mellitus | 0.399 | 0.481 | |||||
Yes | 18 (51.4) | 14 (40.0) | 8 (53.3) | 6 (40.0) | |||
No | 17 (48.6) | 21 (60.0) | 7 (46.7) | 9 (60.0) |
*, significant difference. Data presented as mean ± SD or n (%). BMI, Body mass index; MIBC, muscular infiltrating bladder cancer; NMIBC, non-muscular invasive bladder cancer; NLR, neutrophil-to-lymphocyte ratio; MS, metabolic syndrome; HBP, high blood pressure; HDL-C, High density lipoprotein cholesterol.
The corresponding preoperative multi-parameter CT data were collected, including arterial and venous phases. Using a semi-manual outline, two physicians delineated the three-dimensional (3D) tumor region of interest (ROI) from the calibrated CT images of each patient. The study was conducted in accordance with the Declaration of Helsinki (as revised in 2013). The study was approved by the Ethics Committee of the first affiliated Hospital of Suzhou University (2021162). All patients gave written informed consent.
Selection criteria
Inclusion criteria were as follows: (I) BC diagnosed pathologically after surgery with complete clinical and imaging data; (II) enhanced CT examinations of abdomen and pelvis performed on the same type of CT machine within two weeks before surgery, and the bladder was filled well; and (III) the diameter of the focus ≥1 cm, with the ability to examine an area of interest. Exclusion criteria were as follows: (I) CT showing poor bladder filling or the smallest diameter of the tumor <1 cm; (II) major organ dysfunction, such as kidney, liver, or heart; and (III) other inflammatory diseases or psychiatric disorders.
A total of 100 patients were enrolled and were randomly divided into training and verification groups according to the proportion of 7:3. The patient recruitment pathway is shown in Figure 1.
Image processing and data acquisition
All patients underwent abdominal pelvic (bladder) arteriovenous phase CT examinations using Siemens 64-row spiral CT at our hospital. Afterimage reconstruction, the uncompressed Digital Imaging and Communications in Medicine (DICOM) format data were uploaded to a picture archiving and communication system (PACS) system. The arterial phase and venous thin-layer image data of all patients were downloaded from the PACS system. The thin-slice images of the arterial and venous phases were imported into Slicer 3D software in DICOM format. The arterial- and venous-phase images were calibrated and combined into one image. Under the condition that the pathological results were unknown, a senior physician with more than five years' experience in urological imaging diagnosis and a urologist resident used the threshold and sphere brush functions of the texture analysis Slicer 3D software to outline the half-manual ROI of the bladder tumor lesions and showed them in the form of 3D imaging. The sketched 3D images were processed using unified smoothing. We selected gray-level dependence matrix, Shape2D, gray-level co-occurrence matrix (GLCM), Shape3D, First-order, gray-level run-length matrix (GLRLM), gray-level size zone matrix, and neighboring gray-tone difference matrix feature parameters. Set resampling and filtering criteria were as follows: resampled voxel size (1, 1, 1), LoG kernel sizes (1, 1.5, 2, 2.5), and wavelet-based features (Figure 2). For multiple lesions, considering the heterogeneity among different individuals, only the most significant lesions were included.
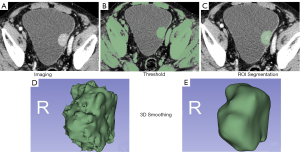
Data processing and feature extraction
The Pyradiomics package based on Python was used to extract 3D image features from all segmented images, including first-order features, second-order features, high-order features, and wavelet-based features: (I) first-order features such as histogram features can describe the global characteristics of the whole ROI that are closely related to the frequency distribution of image gray-scale; (II) second-order features such as those based on GLCM can describe the local distribution of image gray-scale; (III) high-order features such as those based on GLRLM can describe the regional distribution characteristics of gray-scale of an image. In the present study, 80 features were extracted from the specific gray-level tumor ROI of each mode, of which 1,223 features were extracted from first-order statistics (18 features), Shape3D (16 features), Shape 2D (14 features), GLCM (24 features), GLRLM (16 features), gray-level size zone matrix (16 features), neighboring gray-tone difference matrix (five features), and gray-level dependence matrix (14 features). To facilitate the processing of a large amount of data, the radiomics features were numbered as X1, X2, …X1223. The preprocessing of characteristic data is essential. First, all the null values were processed and replaced by the median. To avoid over-fitting the model, two radiologists sketched the image data with ROI. The extracted texture parameters’consistency was evaluated using the intra-class correlation coefficient (ICC >0.8). P<0.05 indicated that the correlation was good. The mean values of two groups of parameters were included in the study. The dimensions of radiomics 1,223 features were reduced using the Z-score so that all features were normalized to [–1, 1], and the operation efficiency was improved. The data set was randomly divided into ten points to prevent over-fitting in the machine learning algorithm, combined with ten-fold cross-validation; seven groups were taken as training data and three groups as test data. We used operator least absolute shrinkage and selection operator (LASSO) and stepwise regressions to screen radiomics features from the training group and obtain practical features. Logical regression, decision tree, support vector machine (SVM), and Adaboost algorithm were used to build the model. The accuracy, sensitivity, specificity, and AUC were used as the evaluation indexes of the model.
Selection and assignment of clinical-related factors
To construct the prediction model of bladder myometrial infiltration and perfect the model, we added gender, age, neutrophil-to-lymphocyte ratio (NLR), urinary pH, urinary tract infection, hematuria, albuminuria, body mass index (BMI), hypertension, diabetes, high-density lipoprotein (HDL-C), triglycerides, location, quantity, diameter, and other clinical traits. We screened out independent risk factors using univariate and multivariate analyses. Considering that we required substantial amounts of data and complex parameters to improve the operation efficiency and intuitive display, all the study parameters were assigned. The postoperative pathological diagnosis of bladder tumor with NMIBC was marked as “1” at the time of classification and otherwise was marked as “2”. For the assignment of other clinical-related factors, please see Table 2. For the assignment of radiomics features, see https://cdn.amegroups.cn/static/public/tcr-21-426-1.xls.
Table 2
Characteristics | Assignment 1 | Assignment 2 |
---|---|---|
Gender | Female | Male |
Urinary tract infection | No | Yes |
Hematuria | No | Yes |
Albuminuria | No | Yes |
Quantity | Single | Multiple |
MS | No MS | MS |
BMI | BMI <25 | BMI ≥25 |
Triglyceride (mmol/L) | <1.7 | ≥1.7 |
High blood pressure | SBP <140 or DBP <90 mmHg | SBP ≥140 or DBP ≥90 mmHg |
Diabetes mellitus | Fasting blood glucose <6.1 mmol/L, Postprandial blood glucose two hours <7.8 mmol/L, Random blood glucose <11.1 mmol/L | Fasting blood glucose≥6.1 mmol/L, Postprandial blood glucose two hours≥7.8 mmol/L, Random blood glucose ≥11.1 mmol/L |
MS, metabolic syndrome; BMI, body mass index; SBP, systolic blood pressure; DBP, diastolic blood pressure.
Prediction model construction
The construction of the model was based on the optimal feature set of each ROI, and the final muscle penetration prediction model was constructed. The radiomics feature prediction model with the highest prediction efficiency was selected by comparing the accuracy, sensitivity, specificity, the area under the receiver operating characteristic (ROC) curve (AUC). To verify the four predictive models' predictive ability, the 10-fold cross-validation method was used, and 100 iterations were carried out. The predictive efficacy evaluation indicators included sensitivity, specificity, accuracy, and AUC. To establish a radiomics model of multiple logistic regression analysis based on radiomics markers and clinical features, all models were built on the training group and were tested on the verification group. The area under the ROC curve was used to test the model's performance and calculate its specificity, sensitivity, and accuracy. By comparing the clinical feature prediction model based on clinical feature index, the radiomics model based on image-marking, and the comprehensive model combined with clinical index, the comprehensive model with the best prediction ability was selected.
Statistical software and methods
The software used in this study included 3D-Slicer (4.10.2-Win-amd 64), R-Studio (1.2.1335), and related software packages. The clinical-related factors were analyzed using SPSS22.0 software (International Business Machines Corporation, IBM), and the measured data was expressed as . T-test was used to compare the two groups of measurement data, and the counting data was compared by chi-square test. Independent risk factors were found by logistic regression. We compared the values of radiological features in the differential diagnosis of NMIBC and MIBC using a single-factor analysis of variance. The LASSO regression model was analyzed using the “glmnet” software package. We used the “proc” software package to draw ROC curves. The differences in AUC values among models were tested using the Delong test. P<0.05 (two-sided) was considered to indicate significance. The effectiveness of the model is expressed by the C-index, and the model is verified by the decision curve (DCA).
Results
Correlation analysis of clinical features
There were 70 patients in the training group and 30 patients in the verification group. Clinical information included gender, age, NLR, urinary pH, urinary tract infection, hematuria, albuminuria, BMI, hypertension, diabetes, HDL-C, triglycerides, location, quantity, diameter, and others. Using univariate analysis, clinical features with P>0.05 were excluded and included proteinuria, metabolic syndrome (MS), and diameter (Table 1). Multivariate analysis showed that only albuminuria and MS were independent predictors in the clinical factor model.
Correlation analysis of radiomics characteristics
Of the 1,223 radiomics features extracted from CT images (arteriovenous-phase calibrated images), 841 had good inter-group and intra-group consistency (ICC ≥0.75, mean =0.77, median =0.84; Figure 3A). We found significant differences between MIBC and MIBC in 624 radiomics features (P<0.05). We entered these into the LASSO regression model to select the most valuable features (Figure 3B). Finally, six features were used to construct the radiomics model (Table 3, P<0.05). This process used pROC and glmnetR software packages.
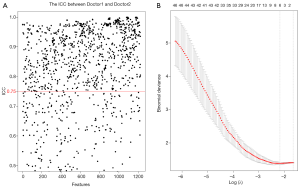
Table 3
Radiological characteristics | Coef | Train (P) | Test (P) | 95% CI |
---|---|---|---|---|
original_shape_Flatness | –2.5580 | 0.007 | 0.046 | 0.698–0.951 |
log-sigma-1-5-mm-3D_glszm_ZoneEntropy | –0.2429 | 0.002 | 0.064 | 0.730–0.933 |
wavelet-HLH_glrlm_RunVariance | –2.0495 | 0.001 | 0.059 | 0.635–0.906 |
wavelet-HHH_gldm_DependenceNonUniformityNormalized | 3.9635 | 0.004 | 0.029 | 0.812–0.961 |
wavelet-LLL_gldm_DependenceVariance | 2.0187 | 0.005 | 0.248 | 0.586–0.924 |
wavelet- LLL_glszm_ZoneEntropy | 7.5914 | 0.002 | 0.011 | 0.842–0.963 |
Construction and selection of the model
We used six practical features to build the model. We built four models: logistic regression, decision tree, SVM, and Adaboost. Combining the accuracy, sensitivity, and specificity of the four models, we found that the model based on SVM was the most valuable (Table 4). The SVM model's accuracy, sensitivity, and specificity in the training group were 80.005%, 82.35%, and 80.56%, respectively, and the AUC was 0.867 (95% CI: 0.781–0.953). In the verification group, the accuracy, sensitivity, and specificity were 73.33%, 81.82%, and 68.42%, respectively, and the AUC was 0.782 (95% CI: 0.615–0.949; Figure 4).
Table 4
Machine algorithm | Group | Accuracy (%) | Sensitivity (%) | Specificity (%) | 95% CI | AUC |
---|---|---|---|---|---|---|
Logistic regression | Train | 74.28 | 70.70 | 79.31 | 0.692–0.899 | 0.796 |
Test | 73.33 | 76.90 | 70.59 | 0.605–0.950 | 0.778 | |
Decision tree | Train | 80.00 | 82.35 | 80.56 | 0.781–0.953 | 0.867 |
Test | 73.33 | 81.82 | 68.42 | 0.615–0.949 | 0.782 | |
SVM | Train | 81.42 | 76.19 | 89.28 | 0.820–0.976 | 0.898 |
Test | 70.00 | 69.23 | 66.67 | 0.495–0.909 | 0.702 | |
Adaboost | Train | 74.28 | 65.71 | 82.85 | 0.641–0.844 | 0.743 |
Test | 63.33 | 53.33 | 73.33 | 0.459–0.808 | 0.633 |
SVM, support vector machine; Adaboost, adaptive boosting.
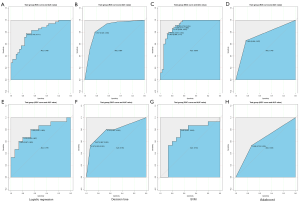
Performance evaluation of clinical prediction model and radiomics prediction model
Univariate and multivariate analysis showed that proteinuria and multiple sclerosis were independent risk factors for myometrial invasion in patients with BC. These findings suggest that patients with albuminuria before surgery are more likely to develop myometrial invasion. Similarly, patients with multiple sclerosis are more likely to have myometrial invasive BC. The training group established a clinical predictive model according to two risk factors. We evaluated and verified the model. Compared with the clinical predictive model, the ROC values of the radiomics model and the clinical predictive model in the training group were 0.796 and 0.747, respectively. The performance of the radiomics model was better than that of the clinical model, and the difference was not statistically significant (P=0.531). We added clinical factors to the radiomics model to obtain a comprehensive model (radiomics + clinical) and found that its prediction performance was the highest of the three models (AUC =0.8457). Figure 5A shows the AUC curves of the three models. It can be seen that the efficacy of the clinical model combined with the radiomics model in predicting myometrial invasion of BC was significantly higher than that of the clinical and radiomics single model.
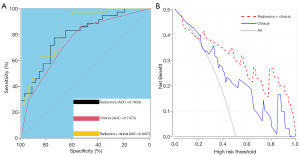
We then verified the decision curve of the comprehensive model (radiomics + clinical) and found that between 20% and 100% of the high-risk threshold, both models achieved better net returns than all of them; however, the integrated model was more valuable than the radiomics model. The decision curve analysis of the two models is shown in Figure 5B.
Establishment of radiological nomogram combined with clinical practice
Albuminuria, MS, and Rad-score were incorporated into the construction of a radiomics nomogram (Figure 6). The calibration curve and Hosmer-Lemeshow test showed an excellent correction effect in the training set (Figure 7A, P=0.041) and the verification set (Figure 7B, P=0.03). To better apply the comprehensive model to clinical practice, we improved the model's accuracy and drew a nomogram to be more intuitive. The clinical model was combined with the radiomics model, and six radiomics features were used to simplify the model, in which we reduced the dimensions of six image parameters and expressed them as Rad-scores, as follows:
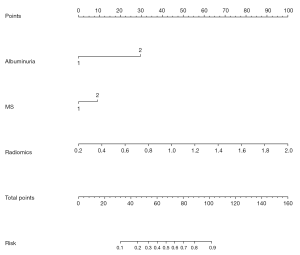
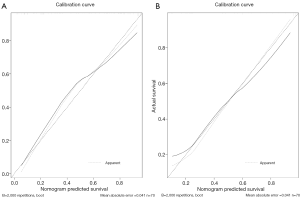
Rad-scores: Log-sigma-1-5-mm-3D_glszm_ZoneEntropy*-0.2429
+wavelet-HLH_glrlm_RunVarianc*-2.0495
+waveletHHH_gldm_DependenceNonUniformityNormalized*3.9635
+wavelet_LLL_gldm_DependenceVariance*2.0187
+wavelet- LLL_glszm_ZoneEntropy*7.5914
-original_shape_Flatness*-2.5580
The decision curve analysis showed that the radiomics nomogram combined with clinical factors had a higher overall net benefit in distinguishing myometrial invasion from non-myometrial invasion than the clinical factor model and the radiomics model.
Discussion
BC is a common malignant tumor of the urinary system. Identification of the muscular layer's infiltration is critical for the choice of treatment and outcome evaluation (16). A cystoscopic biopsy is often performed; however, there is a risk of urethral infection, injury, and implant metastasis (17,18). Over recent decades, many efforts have been made to develop diagnostic and predictive tools for MIBC; nevertheless, BC's recurrence and mortality rates remain high. In recent years, medical radiomics have shown great potential in the clinical diagnosis and prediction of BC. Many radiomics features related to specific cancers have been extracted from images, providing clinicians with profound information that human eyes can not directly obtain and help them diagnose cancer characteristics, becoming a current research hotspot. Studies showed that radiomics features predict the outcome and differentiation of MIBC (19,20). Other studies found that imaging parameters such as MRI distinguished BC from cystitis and aided differentiation of pathological grades of BC (21,22). There is an urgent need to develop objective and non-invasive techniques to distinguish NMIBC from MIBC before surgery and provide information to determine the necessity and adequacy of adjuvant therapy.
Radiological features based on enhanced CT were constructed and validated to predict myometrial invasion in patients with BC before surgery. We developed a predictive nomogram that includes radiomics features and clinical risk factors. There are two primary innovations in our study: (I) based on multi-parameter thin-layer CT, a large number of radiomics features were extracted, and a radiomics feature set was constructed to characterize the tumor heterogeneity differences between NMIBC and MIBC quantitatively; (II) based on feature recursive algorithm selection and the classification strategy of SVM, the feature subset with optimal prediction efficiency was identified, and a prediction model was constructed to achieve accurate prediction.
To effectively solve the problem of gray-scale differences between different thin layers of CT that cause severe impacts on the results of texture feature extraction, we first identified the largest tumor layer in multi-parameter enhanced CT images and completely outlined the whole tumor ROI. The three-dimensional ROI was used, which improved the resolution efficiency over two-dimensional regions of interest because the three-dimensional ROI considers all available slices. Whole tumor analysis appears to be a better indicator of tumor heterogeneity than the maximum cross-sectional area (23). Then, we extracted 1,223 radiomics features. Feature redundancy significantly affects the model’s prediction performance; therefore, we sought to reduce redundancy and the degree of over-fitting of the model to effectively improve the model’s all-around performance and generalization ability to predict myometrial invasion model BC. Based on 1,223 features extracted, the SVM-RFE method was used to select the optimal feature subset, which we then used to describe the image difference between NMIBC and MIBC quantitatively. We then showed that the optimal features selected using this feature selection method improved the model’s prediction accuracy for muscle invasion of BC to 81.42%, and AUC increased to 0.898. Two of the six optimal features were gray-level difference matrix (GLDM) features, which quantify the gray-scale dependence in the image. Based on the gray-scale dependence in the quantized image, these features more effectively quantify the heterogeneity between NMIBC and MIBC. This finding suggests that image parameters can more effectively record and reflect the differences between NMIBC and MIBC, which can be used for feature extraction and prediction model construction.
The radiological features were screened from the training group using LASSO regression and stepwise regression. The six useful features that constitute the imaging labels represent different textures and intensities in the image, reflecting the tumor tissue's heterogeneity. The “wavelet-LLL_gldm_DependenceVariance” reflects the variance of the size of dependencies in the measured ROI in CT images. The higher the eigenvalue, the finer the texture. In myometrial invasive BC, the eigenvalue was smaller (P=0.005, 95% CI: 0.586–0.924). “waveletHHH_gldm_DependenceNonUniformityNormalized” reflects the similarity of the correlation in the whole image. The lower the value, the higher the homogeneity of the correlation in the image. The characteristic value was lower in the MIBC, indicating that the tumor has a higher homogeneity with the bladder myometrium. The tumor tissue has invaded and mixed with the myometrium (P=0.029, 95% CI: 0.812–0.961). The “wavelet-LLL_glszm_ZoneEntropy” and “log-sigma-1–5-mm-3D_glszm_ZoneEntropy” are randomness in measuring region size and gray-scale distribution; higher values indicate higher heterogeneity in texture patterns. In NMIBC, its value is higher, which can be understood as the tumor not invading the muscular layer, and the heterogeneity between the tumor and the muscular layer is high (P=0.02, 95% CI: 0.842–0.963 or P=0.02, 95% CI: 0.730–0.933). The “wavelet-HLH_glrlm_RunVariance” is a measure of the change in run-length, and the eigenvalue was smaller in MIBC (P=0.059, 95% CI: 0.635–0.906). The “original_shape_Flatness” flatness shows the relationship between the largest and smallest principal components in an ROI shape. This value was higher in myometrial infiltration (P=0.007, 95% CI: 0.698–0.951). We constructed the four prediction models based on the training group and tested them in the verification group to achieve robust classification performance. Based on the accuracy, sensitivity, specificity, and AUC value of the model, the comprehensive effect of the model based on the SVM feature recursion algorithm was the best among the four models. The accuracy, specificity, and AUC value of the SVM model were the highest. For specific comparisons, see Table 4. In the model training group based on SVM, the accuracy, sensitivity, specificity, and AUC were 81.42%, 76.19%, 89.28%, and 0.898, respectively 95% CI: 0.820–0.976. The accuracy, sensitivity, specificity, and AUC value in the verification group were 70.006%, 69.23%, 66.67%, and 0.702, respectively. 95% CI: 0.495–0.909. Based on the verification group, the overall effectiveness of the image group model based on SVM was better.
We included clinical data from 100 patients. Univariate and multivariate analyses showed that only albuminuria, MS, and diameter were significant independent predictors. Patients with albuminuria and MS before surgery were more likely to have MIBC after surgery. Considering that there was some overlap between diameter and shape in imaging features, it was not considered a related factor for constructing the clinical model. We established a clinical factor model by combining the clinical characteristics of albuminuria and MS as significant factors. The clinical factor model achieved a higher AUC value for distinguishing NMIBC from MIBC (0.747 in the training group and 0.80 in the verification group).To further improve the prediction model, we successfully constructed a new radiological-clinical prediction model based on radiological characteristics and clinical factors and developed a nomogram (Figure 6). The radiological-clinical nomogram had an excellent predictive value for distinguishing NMIBC from MIBC and calibrating them to obtain the calibration curve shown by Figure 7.
The novelty of this study can be summarized as follows: (I) this is the first study to use multi-parameter thin-layer enhanced CT radiological features to predict the myometrial invasion of bladder tumor before surgery; (II) the features based on signal intensity histogram and three-dimensional ROI were used to reflect the intensity and spatial changes of various maps to characterize the heterogeneity of tumors better; (III) the methods of sample rebalancing and feature selection were used to enhance the prediction performance; (IV) combined with clinical specific factors, a radiological-clinical prediction model was constructed, and a predictive nomogram was developed.
The radiological features based on multi-parameter enhanced CT have great potential for predicting BC recurrence. This study was a retrospective analysis. There are some limitations, including low original sample size, slightly different drug metabolic cycles in patients with CT enhancement, and individual differences in the diffusion of contrast agents. In a follow-up study, we will collect more CT data from eligible patients to increase the collection of myometrial infiltration samples and carry out a more comprehensive analysis and verification of these findings.
Conclusions
Based on the multi-parameter thin-layer enhanced CT radiomics feature can be used as a potential independent predictor of BC myometrial invasion, the model based on parameters can initially quantitatively characterize the risk of myometrial invasion, and has excellent potential for predicting myometrial invasion of BC.
Acknowledgments
Funding: This work was supported by
Footnote
Reporting Checklist: The authors have completed the TRIPOD reporting checklist. Available at https://dx.doi.org/10.21037/tcr-21-426
Data Sharing Statement: Available at https://dx.doi.org/10.21037/tcr-21-426
Conflicts of Interest: All authors have completed the ICMJE uniform disclosure form (available at https://dx.doi.org/10.21037/tcr-21-426). The authors have no conflicts of interest to declare.
Ethical Statement: The authors are accountable for all aspects of the work in ensuring that questions related to the accuracy or integrity of any part of the work are appropriately investigated and resolved. The study was conducted in accordance with the Declaration of Helsinki (as revised in 2013). The study was approved by the Ethics Committee of the first affiliated Hospital of Suzhou University (2021162). All patients gave written informed consent.
Open Access Statement: This is an Open Access article distributed in accordance with the Creative Commons Attribution-NonCommercial-NoDerivs 4.0 International License (CC BY-NC-ND 4.0), which permits the non-commercial replication and distribution of the article with the strict proviso that no changes or edits are made and the original work is properly cited (including links to both the formal publication through the relevant DOI and the license). See: https://creativecommons.org/licenses/by-nc-nd/4.0/.
References
- Kaseb H, Aeddula NR. Bladder Cancer. StatPearls. Treasure Island (FL): StatPearls Publishing, StatPearls Publishing LLC.; 2020.
- Kobayashi T, Owczarek TB, McKiernan JM, et al. Modelling bladder cancer in mice: opportunities and challenges. Nat Rev Cancer 2015;15:42-54. [Crossref] [PubMed]
- Sanli O, Dobruch J, Knowles MA, et al. Bladder cancer. Nat Rev Dis Primers 2017;3:17022. [Crossref] [PubMed]
- Babjuk M, Burger M, Compérat E, et al. Reply to Harry Herr's Letter to the Editor re: Marko Babjuk, Andreas Böhle, Maximilian Burger, et al. EAU Guidelines on Non-muscle-invasive Urothelial Carcinoma of the Bladder: Update 2016. Eur Urol 2017;71:447-61. Eur Urol 2017;71:e173-4. [Crossref] [PubMed]
- Alfred Witjes J, Lebret T, Compérat EM, et al. Updated 2016 EAU Guidelines on Muscle-invasive and Metastatic Bladder Cancer. Eur Urol 2017;71:462-75. [Crossref] [PubMed]
- Fritsche HM, Burger M, Svatek RS, et al. Characteristics and outcomes of patients with clinical T1 grade 3 urothelial carcinoma treated with radical cystectomy: results from an international cohort. Eur Urol 2010;57:300-9. [Crossref] [PubMed]
- Lee DH, Jeong JY, Song W. Prognostic Value of Programmed Death Ligand-1 Expression on Tumor-Infiltrating Immune Cells in Patients Treated with Cisplatin-Based Combination Adjuvant Chemotherapy Following Radical Cystectomy for Muscle-Invasive Bladder Cancer: A Retrospective Cohort Study. Onco Targets Ther 2021;14:845-55. [Crossref] [PubMed]
- Lambin P, Leijenaar RTH, Deist TM, et al. Radiomics: the bridge between medical imaging and personalized medicine. Nat Rev Clin Oncol 2017;14:749-62. [Crossref] [PubMed]
- Luo C, Huang B, Wu Y, et al. Use of Vesical Imaging-Reporting and Data System (VI-RADS) for detecting the muscle invasion of bladder cancer: a diagnostic meta-analysis. Eur Radiol 2020;30:4606-14. [Crossref] [PubMed]
- Wang H, Hu D, Yao H, et al. Radiomics analysis of multiparametric MRI for the preoperative evaluation of pathological grade in bladder cancer tumors. Eur Radiol 2019;29:6182-90. [Crossref] [PubMed]
- Shaikh F, Franc B, Allen E, et al. Translational Radiomics: Defining the Strategy Pipeline and Considerations for Application-Part 2: From Clinical Implementation to Enterprise. J Am Coll Radiol 2018;15:543-9. [Crossref] [PubMed]
- Lu Y, Liu L, Luan S, et al. The diagnostic value of texture analysis in predicting WHO grades of meningiomas based on ADC maps: an attempt using decision tree and decision forest. Eur Radiol 2019;29:1318-28. [Crossref] [PubMed]
- Zhang S, Song M, Zhao Y, et al. Radiomics nomogram for preoperative prediction of progression-free survival using diffusion-weighted imaging in patients with muscle-invasive bladder cancer. Eur J Radiol 2020;131:109219. [Crossref] [PubMed]
- Panebianco V, De Berardinis E, Barchetti G, et al. An evaluation of morphological and functional multi-parametric MRI sequences in classifying non-muscle and muscle invasive bladder cancer. Eur Radiol 2017;27:3759-66. [Crossref] [PubMed]
- van der Pol CB, Chung A, Lim C, et al. Update on multiparametric MRI of urinary bladder cancer. J Magn Reson Imaging 2018;48:882-96. [Crossref] [PubMed]
- Flaig TW, Spiess PE, Agarwal N, et al. NCCN Guidelines Insights: Bladder Cancer, Version 5.2018. J Natl Compr Canc Netw 2018;16:1041-53. [Crossref] [PubMed]
- Jakse G, Algaba F, Malmström PU, et al. A second-look TUR in T1 transitional cell carcinoma: why? Eur Urol 2004;45:539-46; discussion 546. [Crossref] [PubMed]
- Miladi M, Peyromaure M, Zerbib M, et al. The value of a second transurethral resection in evaluating patients with bladder tumours. Eur Urol 2003;43:241-5. [Crossref] [PubMed]
- Wang HJ, Pui MH, Guo Y, et al. Diffusion-weighted MRI in bladder carcinoma: the differentiation between tumor recurrence and benign changes after resection. Abdom Imaging 2014;39:135-41. [Crossref] [PubMed]
- Xu X, Liu Y, Zhang X, et al. Preoperative prediction of muscular invasiveness of bladder cancer with radiomic features on conventional MRI and its high-order derivative maps. Abdom Radiol (NY) 2017;42:1896-905. [Crossref] [PubMed]
- Wang HJ, Pui MH, Guo Y, et al. Preliminary study of diffusion-weighted MRI in the preoperative diagnosis of cystitis glandularis. Clin Radiol 2016;71:937.e1-4. [Crossref] [PubMed]
- Wang HJ, Pui MH, Guo Y, et al. Multiparametric 3-T MRI for differentiating low-versus high-grade and category T1 versus T2 bladder urothelial carcinoma. AJR Am J Roentgenol 2015;204:330-4. [Crossref] [PubMed]
- Ng F, Kozarski R, Ganeshan B, et al. Assessment of tumor heterogeneity by CT texture analysis: can the largest cross-sectional area be used as an alternative to whole tumor analysis? Eur J Radiol 2013;82:342-8. [Crossref] [PubMed]