Mutational status of main driver genes influences the prognosis of stage I–III lung adenocarcinoma patients underwent radical surgery
Introduction
Lung cancer is the most common types of cancer with highest morbidity and mortality in the world (1). Great progress has been made in the development of therapeutic strategies for local advanced or metastatic lung cancer, including targeted therapy, immunotherapy and combined therapy (2). Late-stage lung cancer has attached much attention and clinical research resource due to the therapeutic difficulties and its high mortality and complications. In contrast, early stage lung cancer, especially for those who have opportunity for radical surgery, was not the focus of systematic therapy, although post-surgical adjuvant therapy is needed for these patients. Recent advances in adjuvant therapy by tyrosine kinase inhibitors (TKIs) for surgically resected lung cancer rendered new therapeutic strategies for early-stage lung cancer patients and enhanced patients recurrent free survival (RFS) and potentially overall survival (OS) (3-5).
The correlation between mutational landscape and patient prognosis in late-stage lung cancer patients has been extensive investigated in various scenarios including targeted therapy, immunotherapy and combined therapy (6,7). Main driver gene mutations and a series of markers, including EGFR, KRAS, TP53 and TMB have been suggested as stratification markers for therapeutic response or prognosis in systematic therapy (8,9). Since radical surgery is the main therapeutic strategy for early-stage lung cancer, the correlation between these markers and the response or prognosis of early-stage patients has not been thoroughly investigated.
In order to clarify the relationship between main driver gene mutational status and the prognosis of early-stage lung cancer patients, we designed a study and recruited 447 stage I–III Chinese lung adenocarcinoma (LUAD) patients who underwent radical surgery, and examined the mutational landscape of these patients by whole-exome sequencing (WES). We investigated the stratification of prognosis by main driver gene mutations and discovered interesting correlation between mutational status and prognosis. The clinicopathological factors were also examined with the prognosis of these patients.
We present the following article in accordance with the REMARK reporting checklist (available at https://dx.doi.org/10.21037/tcr-21-240).
Methods
Ethical statement
The study was conducted in accordance with the Declaration of Helsinki (as revised in 2013). The study was approved by the ethics committee of Yuebei People’s Hospital and the First Affiliated Hospital of Jinan University (NO. 2019YBPH0518) and individual consent for this retrospective analysis was waived.
Study design, patients and samples
The study was designed and implemented in Yuebei People’s Hospital and the First Affiliated Hospital of Jinan University. Cancer tissue and blood samples were collected retrospectively. The study was designed to include stage IA-IIIB (T1-4, N0-2, M0) LUAD patients who received radical surgery as the first-line therapy (Table 1). The staging of lung cancer patients was based on the Eighth Edition of the TNM Classification of Lung Cancer. The primary outcomes included the difference in OS among different groups of patients based on mutational status, stage, tumor mutational burden (TMB) and clincopathological factors. The secondary outcome involved stage-dependent mutational frequency. Patients were followed up to 7,248 days (median at 658 days, ranging from 4 to 7,248 days). Samples from 447 qualified lung adenocarcinoma patients were obtained and the demographic and clinicopathological information are shown in Table 1. Samples consisted of frozen tissue from surgery or needle biopsy and FFPE samples. Samples with cancer cell content more than 20% were sent for WES test. All technicians were blinded to subject clinical information. Confirmation of pathological types was based on diagnosis from imaging examinations and subsequent pathological examinations. None of the subjects received chemotherapy, radiotherapy, targeted therapy, or immunotherapy before tissue samples were collected. The somatic sequencing data presented in this study were from lung tumor tissue DNA, with germline sequencing data from the corresponding peripheral blood lymphocytes (PBLs) as the control.
Table 1
Items | Number of patients |
---|---|
Sex | |
Male | 207 |
Female | 240 |
Age | |
30–39 | 3 |
40–49 | 35 |
50–59 | 117 |
60–69 | 149 |
70–79 | 122 |
≥80 | 21 |
T grading | |
T1 | 152 |
T2 | 235 |
T3 | 43 |
T4 | 17 |
N grading | |
N0 | 304 |
N1 | 84 |
N2 | 59 |
Clinical staging | |
I | 279 |
II | 124 |
III | 44 |
Location | |
Upper lung | 266 |
Middle lung | 26 |
Lower lung | 155 |
Cancer history | |
Prior malignancy | 80 |
No prior malignancy | 367 |
Smoking status | |
Non-smoker | 142 |
Smoker <40 packs/year | 151 |
Smoker ≥40 packs/year | 154 |
Smoking years | |
0 years (non-smoker) | 142 |
<33 years | 149 |
≥33 years | 156 |
Mutational status | |
EGFR | |
mut | 176 |
WT | 271 |
TP53 | |
mut | 191 |
WT | 256 |
KRAS | |
mut | 72 |
WT | 375 |
STK11 | |
mut | 27 |
WT | 420 |
ATM | |
mut | 29 |
WT | 418 |
NF1 | |
mut | 34 |
WT | 413 |
Adjuvant therapy | |
Chemotherapy | 53 |
Chemo/radiotherapy | 22 |
TKI therapy | 48 |
Unknown | 324 |
Sample preparation, targeted NGS and data processing for WES
DNA was extracted from the fresh tissue, FFPE tissue or needle biopsy samples using the QIAamp DNA Tissue Kit (QIAGEN, Valencia, CA, USA) following the manufacturer’s instructions. 2 ml of peripheral blood was collected and DNA was extracted from PBLs using the RelaxGene Blood DNA system (Tiangen Biotech Co., Ltd., Beijing, China). Both cancer tissue and white blood cell genomic DNA was quantified with the Qubit 2.0 Fluorometer and the Qubit dsDNA HS assay kit (Thermo Fisher Scientific, Inc., Waltham, MA, USA) according to manufacturer's instructions. In brief, fragmented genomic DNA underwent end-repairing, A-tailing and ligation with indexed adapters sequentially, followed by size selection using Agencourt AMPure XP beads (Beckman Coulter Inc., Brea, CA, USA), and DNA fragments were used for library construction with the KAPA Library Preparation kit (Kapa Biosystems, Inc., Wilmington, MA, USA) according to the manufacturer's protocol. Hybridization-based target enrichment was conducted with standard WES panel (HaploX Biotechnology, Shenzhen, China) for cancer tissue sequencing. Seven to eight PCR cycles, depending on the amount of DNA input, were performed on Pre-LM-PCR Oligos (Kapa Biosystems, Inc.) in 50 µL reactions. The DNA sequencing was then performed on the Illumina Novaseq 6000 system according to the manufacturer's instructions at an average depth of 300×.
Sequencing data were de-multiplexed and aligned to the hg38 genome (GRCh38) using Burrows-Wheeler Aligner (http://bio-bwa.sourceforge.net/) version 0.7.15-r1140 with default settings. Pileup files for properly paired reads with mapping quality ≥60 were generated using Samtools (http://www.htslib.org/). Somatic variants were determined using VarScan2 (http://varscan.sourceforge.net/). Allele frequencies were calculated for all Q30 bases. Using a custom Python script, previously identified tumor DNA mutations were intersected with a Samtools mpileup file generated for each sample, and the number and frequency were then calculated for each mutation. A mutation was declared if ≥5 mutant reads were identified, and ≥1 mutant read was found on each strand. Matched genomic DNA from white blood cells was used as the control.
Statistical analysis
Statistical analysis was performed, and figures were plotted with GraphPad PRISM version 5.0 software (GraphPad Software, Inc, La Jolla, CA 92037, USA). Student t-test or non-parametric test was performed when two groups were compared, and ANOVA and post hoc tests were performed when three or more groups were compared. Chi-square test and Fisher test were performed when rate or percentage was compared for significance. Kaplan-Meier analysis was performed and the survival curves were compared using the Log-rank test. ‘*’ represents P<0.05 (significant), ‘**’ represents P<0.01 (highly significant), and ‘***’ represents P<0.001 (very highly significant). Tissue TMB was calculated by dividing the total number of tissue non-synonymous SNV and INDEL variations (allele frequency > 2%) by the full length of the WES panel.
Results
Mutational status of main driver genes influenced the prognosis of stage I–III LUAD patients
The mutational status of stage I–III LUAD patients was examined first. Distinct stage-dependent mutational frequency was revealed for main driver genes. Figure 1A shows the distinct mutational frequency (size of dots) and variant allele frequency (color of dots) across many genes. The highly mutated driver genes include TP53, KRAS, EGFR, etc. We then examined the mutational frequency of representative driver genes for each stage. It can be seen from Figure 1B that the mutational frequency of EGFR in stage I was significantly higher than that of the stage II and III. In contrast, the TP53 mutational frequency increased with the elevation of stage, while KRAS exhibited higher frequency in stage II than stage I and III. Other driver genes, such as STK11, ATM and NF1, also exhibited characteristic mutational frequency across stages. These observations suggest that the main driver genes were differentially mutated across stages of LUAD in Chinese population.
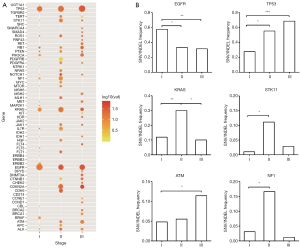
We further investigated whether the distinct stage-dependent mutational frequency was correlated with patient long-term survival. Figure 2 shows the stratification of patient survival by mutational status (wild type or mutant) across each stage for the main driver genes. When all stage I–III patients were involved (Figure 2A), no significant stratification by mutational status was observed for main driver genes, although a trend of better survival for wild type EGFR (P=0.117) and mutant TP53 (P=0.066) can be found. When stage I, II or III was studied individually (Figure S1), significant stratification was only observed in STK11 in stage III (P=0.031), although a trend of stratification was also observed in other genes and other stages, as indicated by the P values in individual panels. Cancer stage is a widely accepted stratification factor for survival, and higher stage generally leads to worse survival. We therefore compared the across-stage survival in wild type and mutant driver genes (Figure 2B,C). It is interesting to find that the stratification by stage vanished in EGFR mutant (Figure 2B, first row), while still existed in other genes, regardless of mutational status. These observations suggested that TP53, STK11 and EGFR mutations may affect the long-term survival of LUAD patients.
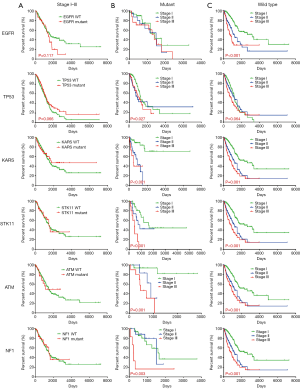
The stratification by different driver genes was also investigated. Figure 3 shows the comparison of survival across wild type (upper row) or mutant (lower row) driver genes. It was interesting to find that stage I–III patients with wild type TP53 exhibited significantly worse survival (P<0.001) than patients with other wild type genes. Further analysis showed that this difference in survival originated from the worse survival in stage I patients with wild type TP53, not the stage II and stage III patients. In contrast, no significant difference in survival was observed across mutant driver genes in stage I–III patients (Figure 3 lower row). However, a trend of worse survival was observed in stage I with EGFR or TP53 mutations than those with KRAS or ATM mutations (P=0.05).
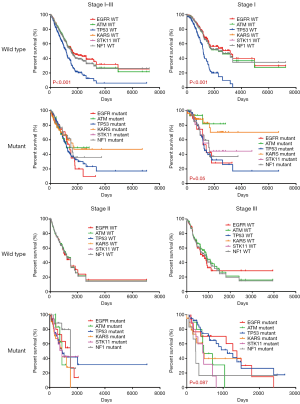
Tumor mutational burden (TMB) is a widely accepted marker in first and multiple lines of immunotherapy for local advanced or metastatic lung cancer. Here we examined the correlation between TMB and survival in stage I–III LUAD patients. No significant difference in TMB was observed across stage I, II and III (Figure 4A). When TMB =10 was defined as the threshold for high and low TMB, no statistically significant stratification by TMB was observed in stage I–III patients or each individual stage (Figure 4B), although a trend of better survival with high TMB may exist in stage III (P=0.165).
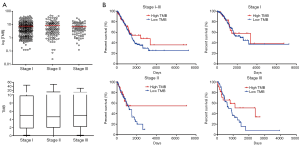
Clinicopathological factors influenced the prognosis of stage I–III patients
The influence of patient demographic factors, lifestyle factors, cancer history and cancer pathological factors on survival was also investigated. It can be seen from Figure 5 that age and gender had no stratification effect on survival, although a trend of younger patients with better survival may exist (P=0.179). Although smoking status is a definite risk factor for lung cancer incidence, it appeared to have no influence on patient survival. The duration of smoking may have an influence (P=0.111), but this may be related to patient age. Furthermore, prior cancer history and tumor location did not affect the patient survival. However, patients with ≤5 cm tumor (T1 and T2) had better survival than those with >5 cm tumor (T3 and T4) (P<0.001), and patients with no lymph node metastasis (N0) had better survival than those with lymph node metastasis (N1 and N2) (P<0.001). These observations suggest that T and N grading predicted the survival of stage I–III LUAD patients.
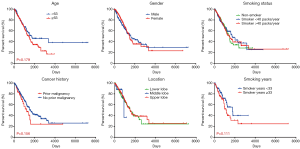
Both univariate and multivariate regression analyses were performed with clinicopathological and mutational factors, including clinical stage, pathological T grading, pathological N grading, EGFR mutations, TP53 mutations and STK11 mutations. It can be clearly seen from Table 2 that clinical stage and pathological T grading were risk factors in univariate analysis, while pathological T grading was the only independent risk factor for stage I–III LUAD patients in multivariate analysis, with a hazard ratio (HR) of 1.675 (95% CI: 1.148–2.442).
Table 2
Variables | Category | Univariate | Multivariate | |||||
---|---|---|---|---|---|---|---|---|
HR | 95% CI | P value | HR | 95% CI | P value | |||
Clinical stage | I, II,I II | 1.170 | 0.951–1.438 | 0.020 | 1.182 | 0.852–1.634 | 0.310 | |
Pathological T | T1, T2, T3, T4 | 1.379 | 1.086–1.751 | 0.000 | 1.675 | 1.148–2.442 | 0.000 | |
Pathological N | N0, N1, N2 | 0.902 | 0.576–1.413 | 0.161 | 0.879 | 0.539–1.433 | 0.194 | |
EGFR mutation | Mutant/WT | 0.932 | 0.716–1.190 | 0.538 | 0.964 | 0.738–1.259 | 0.788 | |
TP53 mutation | Mutant/WT | 0.892 | 0.767–1.039 | 0.142 | 0.874 | 0.731–1.016 | 0.127 | |
KRAS mutation | Mutant/WT | 1.032 | 0.721–1.477 | 0.864 | 1.056 | 0.743–1.481 | 0.795 | |
STK11 mutation | Mutant/WT | 1.034 | 0.725–1.238 | 0.596 | 1.046 | 0.6975–1.256 | 0.629 | |
ATM mutation | Mutant/WT | 0.971 | 0.536–1.762 | 0.924 | 0.934 | 0.506–1.729 | 0.882 | |
NF1 mutation | Mutant/WT | 1.035 | 0.654–1.638 | 0.884 | 1.013 | 0.628–1.617 | 0.835 |
Discussion
Influence of main driver gene mutational status on patients therapeutic response and prognosis
The influence of driver gene mutations in local advanced or metastatic lung cancer has been intensively investigated in targeted therapy and immunotherapy. EGFR mutations have been correlated with better survival as TKIs can be used for patients with the mutations (10). KRAS mutations are also targets for TKIs in both colorectal cancer and lung cancer (11,12). Since systematic therapy was not conventionally used for patients with early stage lung cancer undergoing radical surgery, the roles of these driver gene mutations in therapy and survival of these patients have not been investigated in detail. In this study, we investigated the potential roles of main driver gene mutations in OS stratification in stage I–III LUAD patients.
We identified distinct stage-dependent mutational frequency for main driver genes in this study in Chinese population, suggesting that the frequency of mutations in each stage was not consistent. EGFR appeared to have higher mutational frequency in stage I than stage II and III, while the mutational frequency of TP53 and ATM increased with stage, and stage II had the highest mutational frequency in KRAS, STK11 and NF1. The reason is not clear for the stage-dependent difference and across-gene differences in mutational frequency, but it may be related to distinct clonal expansion during carcinogenesis, reflecting the Darwin evolution and subsequent parallel evolution in tumor development (13,14). Some clones containing EGFR may expand very early in carcinogenesis, and therefore exhibited a high mutational ratio in early stage cancers, but its ratio decreased when other clones start to expand at later cancer stages, such as those with TP53 and ATM. Clones with KRAS, STK11 and NF1 may preferentially expand at stage II, and parallel evolved at later stages.
The influence of the distinct stage-relevant mutational frequency was investigated in our study. It was interesting to find that EGFR mutations eliminated the difference in survival among stage I–III patients, which appeared to be a protective effect for patients with EGFR mutations at higher stages, while this effect was not seen in other wild type and mutant genes. Patients involved in this study had conventional post-surgical adjuvant therapy but not TKI-based therapy (3-5), therefore, it can be suggested this effect was not due to post-surgical treatment but EGFR itself. However, recent strong evidence suggested disease-free survival was significantly longer among patients who received Osimertinib as post-surgical adjuvant therapy than among those who received placebo for stage IB to IIIA EGFR mutation-positive non-small cell lung cancer (NSCLC) (3-5). This opens a new era for adjuvant TKI therapy in early stage lung cancer patients with radical surgery, which may significantly enhance PFS and OS for these patients.
It was also interesting to find that wild type TP53 exhibited worse survival than their counterparts of other genes in stage I alone, while this was not that obvious in mutant TP53. TP53 mutations also exhibited a trend of better survival than its wild type. These observations suggested that wild type TP53 was an indicator for worse survival in stage I LUAD. Indeed, the amount of TP53 mutations was found to be positively correlated with TMB, which has been suggested as a predicting indicator for survival of early-stage lung cancer patients (15,16). Therefore, less TP53 mutations may indicate poorer survival in this scenario. The correlation among main driver gene mutations and the roles of their co-mutations in survival prediction are worth more investigation in early stage patients.
Influence of TMB on patient prognosis
TMB has been extensively reported as one effective marker for stratifying the therapeutic response in first-line or multiple lines of immunotherapy in local advanced and metastatic cancer patients including lung malignancies (15,17). This is mainly due to the fact that mutational burden is in positive correlation with the amount of cancer neoantigens, generating tumor immunogenicity and conditioning the response to ICIs (6). Patients with higher TMB were found to benefit more from immunotherapy than those with lower TMB (18). More recently, it was reported that TMB subtyping can predict the prognosis of first-line chemotherapy in local advanced and metastatic NSCLC patients, in which higher TMB correlated with worse PFS and OS in chemotherapy (19). In contrast to the extensive investigation of TMB in systematic therapy, its roles in early-stage lung cancer with radical surgery has not been fully defined. Although we did not find significant difference in survival between high and low TMB patients in our study, a trend of higher TMB with better survival can be observed, which is consistent with a recent study with large TCGA cohort (20). This is interesting in early-stage lung cancer patients with radical surgery, since our observation and recent evidence suggest that TMB itself is capable of predicting the OS of these patients, regardless of the strategy of post-surgical adjuvant therapy. Similar correlation can also be observed in stage II colon cancer patients with surgical resection, in which mismatch repair deficiency and high TMB have been utilized as good prognostic biomarkers (21). The reason for this correlation has not been clearly defined, but it was suggested that high neoantigen burden and intense TIL infiltration may be associated with favorable survival outcomes in early-stage lung cancer (22,23). Indeed, high TMB may reflect the individual immunogenicity and mediate the profile of tumor-host immune interactions.
Influence of clincopathological factors on patient prognosis
Smoking is a widely accepted risk factor for lung cancer and increases lung cancer incidence. However, whether smoking affects the patient’s long-term survival has not been fully investigated. We found no difference in OS between non-smoker and smoker in stage I–III LUAD patients, but this does not mean that smoking has no influence on survival of lung cancer patients, as evidence suggest that ever-smokers exhibited significantly lower RFS rate than never smokers (24). We also found a trend of better OS in patients with less smoking years. Since young smokers tend to have fewer smoking years, it is not known whether the trend was caused by age effect.
It appeared that previous cancer history did not affect the survival of stage I–III LUAD patients. The possibility of second primary lung cancer should always be considered when new lesions are found in patients with previous cancer history. It has been reported that patients with secondary lung cancer associated other primary malignancies have non-inferior survival than those with single lung cancer (25). However, metachronous primary cancers may represent higher susceptibility to cancer for individuals, and more intensive screening for other cancers should be considered. If cancers are found at early stages and appropriate therapeutic strategies are implemented, the survival may not be different to those patients with only one primary cancer. Moreover, for those with family history of cancers, screening for germline mutations should be considered, as it has been shown that pathogenic or likely pathogenic germline mutations increased the risk of lung cancer (26).
Location appeared to have no influence on patient survival in our study, while it was reported that a small proportion of lung cancer patients have synchronous multiple primary lung cancer (SMPLC) or intrapulmonary metastasis, representing two distinct conditions of multiple lung lesions (27-29). One recent meta-analysis found that the pooled 5-year OS was 45% for SMPLC patients and 62% for stage I lung cancer, which was different from the 5-year OS of 93% of patients with multifocal ground-glass/lepidic (GG/L) lung cancers. The poor prognostic factors for SMPLC were lymph node metastasis and pneumonectomy, whereas histology, laterality, sublobar resection and adjuvant therapy were not found to influence the outcome (27). Therefore, it seems that the long-term prognosis of SMPLC patients after surgery is dependent on staging and metastasis, and is acceptable in patients with early-stage disease. SMPLC should be distinguished from multifocal GG/L lung cancer to perform accurate surgical evaluation.
T and N grading have long been known to be factors affecting the survival of lung cancer patients (30). We found in this study that patients with less than 5 cm lesions and with no lymph node metastasis had significantly better survival, which confirmed that early stage cancer with no lymph node metastasis have better survival than late stage cancer with lymph node metastasis. Furthermore, T grading was the only independent risk factor for stage I–III LUAD patients, although TP53, EGFR and STK11 mutational status, T and N grading all appeared to affect the prognosis. This observation suggested that N grading may be correlated with T grading to influence prognosis.
This study had some limitations. The follow-up information was not complete in this study. This was because a large majority of patients left our hospital after surgery and went back to local hospitals for subsequent systematic therapy, including chemotherapy, chemo- and radiotherapy and TKI therapy (Table 1). This made it difficult for us to collect information on therapeutic response assessment, progression free survival and cancer recurrence. Therefore, it was not possible to ensure enough statistical confidence for stratification of survival by post-surgical therapy or progression free survival due to the incomplete information.
Acknowledgments
Funding: This study was supported by
Footnote
Reporting Checklist: The authors have completed the REMARK reporting checklist. Available at https://dx.doi.org/10.21037/tcr-21-240
Data Sharing Statement: Available at https://dx.doi.org/10.21037/tcr-21-240
Conflicts of Interest: All authors have completed the ICMJE uniform disclosure form (available at https://dx.doi.org/10.21037/tcr-21-240). Dr. Wan and Dr. Xu report that this study was supported by the Special Funds for Strategic Emerging Industry Development of Shenzhen (Grant No.: 20170922151538732), and the Science and Technology Project of Shenzhen (Grant No.: JSGG20180703164202084). All funders did not participate in the study design, study implementation, data collection, data analysis, data interpretation and manuscript writing of the study. The other authors have no conflicts of interest to declare.
Ethical Statement: The authors are accountable for all aspects of the work in ensuring that questions related to the accuracy or integrity of any part of the work are appropriately investigated and resolved. The study was conducted in accordance with the Declaration of Helsinki (as revised in 2013). The study was approved by the ethics committee of Yuebei People’s Hospital and the First Affiliated Hospital of Jinan University (NO. 2019YBPH0518) and individual consent for this retrospective analysis was waived.
Open Access Statement: This is an Open Access article distributed in accordance with the Creative Commons Attribution-NonCommercial-NoDerivs 4.0 International License (CC BY-NC-ND 4.0), which permits the non-commercial replication and distribution of the article with the strict proviso that no changes or edits are made and the original work is properly cited (including links to both the formal publication through the relevant DOI and the license). See: https://creativecommons.org/licenses/by-nc-nd/4.0/.
References
- Jemal A, Bray F, Center MM, et al. Global cancer statistics. CA Cancer J Clin 2011;61:69-90. [Crossref] [PubMed]
- Nagasaka M, Gadgeel SM. Role of chemotherapy and targeted therapy in early-stage non-small cell lung cancer. Expert Rev Anticancer Ther 2018;18:63-70. [Crossref] [PubMed]
- Wu YL, Tsuboi M, He J, et al. Osimertinib in Resected EGFR-Mutated Non-Small-Cell Lung Cancer. N Engl J Med 2020;383:1711-23. [Crossref] [PubMed]
- Zhong WZ, Chen KN, Chen C, et al. Erlotinib Versus Gemcitabine Plus Cisplatin as Neoadjuvant Treatment of Stage IIIA-N2 EGFR-Mutant Non-Small-Cell Lung Cancer (EMERGING-CTONG 1103): A Randomized Phase II Study. J Clin Oncol 2019;37:2235-45. [Crossref] [PubMed]
- Zhong WZ, Wang Q, Mao WM, et al. Gefitinib versus vinorelbine plus cisplatin as adjuvant treatment for stage II-IIIA (N1-N2) EGFR-mutant NSCLC (ADJUVANT/CTONG1104): a randomised, open-label, phase 3 study. Lancet Oncol 2018;19:139-48. [Crossref] [PubMed]
- Rizvi NA, Hellmann MD, Snyder A, et al. Cancer immunology. Mutational landscape determines sensitivity to PD-1 blockade in non-small cell lung cancer. Science 2015;348:124-8. [Crossref] [PubMed]
- VanderLaan PA, Rangachari D, Mockus SM, et al. Mutations in TP53, PIK3CA, PTEN and other genes in EGFR mutated lung cancers: Correlation with clinical outcomes. Lung Cancer 2017;106:17-21. [Crossref] [PubMed]
- Kadara H, Choi M, Zhang J, et al. Whole-exome sequencing and immune profiling of early-stage lung adenocarcinoma with fully annotated clinical follow-up Ann Oncol 2017;28:75-82. Erratum in: Ann Oncol 2018;29:1072. [Crossref] [PubMed]
- Shang Y, Li X, Liu W, et al. Comprehensive genomic profile of Chinese lung cancer patients and mutation characteristics of individuals resistant to icotinib/gefitinib. Sci Rep 2020;10:20243. [Crossref] [PubMed]
- Vaid AK, Gupta A, Momi G. Overall survival in stage IV EGFR mutation-positive NSCLC: Comparing first-, second- and third-generation EGFR-TKIs Int J Oncol 2021;58:171-84. (Review). [Crossref] [PubMed]
- Therkildsen C, Bergmann TK, Henrichsen-Schnack T, et al. The predictive value of KRAS, NRAS, BRAF, PIK3CA and PTEN for anti-EGFR treatment in metastatic colorectal cancer: A systematic review and meta-analysis. Acta Oncol 2014;53:852-64. [Crossref] [PubMed]
- Tomasini P, Walia P, Labbe C, et al. Targeting the KRAS Pathway in Non-Small Cell Lung Cancer. Oncologist 2016;21:1450-60. [Crossref] [PubMed]
- Angelova M, Mlecnik B, Vasaturo A, et al. Evolution of Metastases in Space and Time under Immune Selection. Cell 2018;175:751-65.e16. [Crossref] [PubMed]
- Yates LR, Campbell PJ. Evolution of the cancer genome. Nat Rev Genet 2012;13:795-806. [Crossref] [PubMed]
- Willis C, Fiander M, Tran D, et al. Tumor mutational burden in lung cancer: a systematic literature review. Oncotarget 2019;10:6604-22. [Crossref] [PubMed]
- Sun H, Liu SY, Zhou JY, et al. Specific TP53 subtype as biomarker for immune checkpoint inhibitors in lung adenocarcinoma. EBioMedicine 2020;60:102990. [Crossref] [PubMed]
- Carbone DP, Reck M, Paz-Ares L, et al. First-Line Nivolumab in Stage IV or Recurrent Non-Small-Cell Lung Cancer. N Engl J Med 2017;376:2415-26. [Crossref] [PubMed]
- Klempner SJ, Fabrizio D, Bane S, et al. Tumor Mutational Burden as a Predictive Biomarker for Response to Immune Checkpoint Inhibitors: A Review of Current Evidence. Oncologist 2020;25:e147-59. [Crossref] [PubMed]
- He Y, Song L, Wang H, et al. Mutational Profile Evaluates Response and Survival to First-Line Chemotherapy in Lung Cancer. Adv Sci (Weinh) 2020;8:2003263. [Crossref] [PubMed]
- Tian Y, Xu J, Chu Q, et al. A novel tumor mutational burden estimation model as a predictive and prognostic biomarker in NSCLC patients. BMC Med 2020;18:232. [Crossref] [PubMed]
- Le DT, Durham JN, Smith KN, et al. Mismatch repair deficiency predicts response of solid tumors to PD-1 blockade. Science 2017;357:409-13. [Crossref] [PubMed]
- Forde PM, Chaft JE, Smith KN, et al. Neoadjuvant PD-1 Blockade in Resectable Lung Cancer. N Engl J Med 2018;378:1976-86. Erratum in: N Engl J Med 2018;379:2185. [Crossref] [PubMed]
- Mazzaschi G, Madeddu D, Falco A, et al. Low PD-1 Expression in Cytotoxic CD8+ Tumor-Infiltrating Lymphocytes Confers an Immune-Privileged Tissue Microenvironment in NSCLC with a Prognostic and Predictive Value. Clin Cancer Res 2018;24:407-19. [Crossref] [PubMed]
- Shima T, Kinoshita T, Uematsu M, et al. How long is cessation of preoperative smoking required to improve postoperative survival of patients with pathological stage I non-small cell lung cancer? Transl Lung Cancer Res 2020;9:1924-39. [Crossref] [PubMed]
- Shan S, She J, Xue ZQ, et al. Clinical characteristics and survival of lung cancer patients associated with multiple primary malignancies. PLoS One 2017;12:e0185485. [Crossref] [PubMed]
- Liu M, Liu X, Suo P, et al. The contribution of hereditary cancer-related germline mutations to lung cancer susceptibility. Transl Lung Cancer Res 2020;9:646-58. [Crossref] [PubMed]
- Nie Y, Wang X, Yang F, et al. Surgical Prognosis of Synchronous Multiple Primary Lung Cancer: Systematic Review and Meta-Analysis. Clin Lung Cancer 2020; [Epub ahead of print]. [Crossref] [PubMed]
- Wang X, Gong Y, Yao J, et al. Establishment of Criteria for Molecular Differential Diagnosis of MPLC and IPM. Front Oncol 2021;10:614430. [Crossref] [PubMed]
- Chen K, Zhao H, Shi Y, et al. Perioperative Dynamic Changes in Circulating Tumor DNA in Patients with Lung Cancer (DYNAMIC). Clin Cancer Res 2019;25:7058-67. [Crossref] [PubMed]
- Goldstraw P, Chansky K, Crowley J, et al. The IASLC Lung Cancer Staging Project: Proposals for Revision of the TNM Stage Groupings in the Forthcoming (Eighth) Edition of the TNM Classification for Lung Cancer. J Thorac Oncol 2016;11:39-51. [Crossref] [PubMed]