CENPA is one of the potential key genes associated with the proliferation and prognosis of ovarian cancer based on integrated bioinformatics analysis and regulated by MYBL2
Introduction
Ovarian cancer is a highly lethal disease, and the fifth leading cause of all cancer-related deaths in women. Furthermore, there have been approximately 295,414 (1.6%) new cases and 184,799 (1.9%) deaths in 185 countries (1), and 22,530 new cases and 13,980 deaths in the United States per year (2).Ovarian cancer can be classified as high-grade serous carcinoma (HGSC) and low-grade serous (LGSC), and that’s only a small fraction of all cases. HGSCs are characterized by rapid growth and aggressive behavior (3). The combination of cytoreductive surgery and chemotherapy has been the most important treatment strategy for HGSC, in terms of improving patient survival. However, the treatment efficacy remains unsatisfactory (4). Therefore, in order to better treat ovarian cancer, it is very important to understand the pathogenesis in greater detail.
MYB-related protein B (B-MYB/MYBL2) is a transcription factor and plays important roles in the regulation of cell cycle, survival and differentiation. In addition, it is often abnormally expressed in cancer, suggesting that it functions in driving the onset and/or progression of cancer (5,6). In glioma, demethylzeylasteral can inhibit glioma cell growth via the miR-30e-5p/MYBL2 axis (7). In addition, studies have shown that MYBL2 is regulated by the Akt/FoxM1 signaling pathway in gliomas. This may become a biomarker for glioma radiation therapy (8). In non-small-cell lung cancer (NSCLC), MYBL2 is aberrantly upregulated. Its overexpression can significantly promote NSCLC cell growth and motility by suppressing IGFBP3 (9). Furthermore, miR-30a may suppress the proliferation of NSCLC by targeting MYBL2 (10). In breast cancer, MYBL2 regulates cell proliferation and apoptosis, and is targeted by miR-143-3p (11). Mybl2 upregulation leads to an imbalance in cell cycle regulation that promotes liver cancer progression (12). In leukemic blasts, MYBL2 is regulated by CCNA1, and promotes cell proliferation (13).
The present study evaluates the prognostic value of MYBL2 in ovarian cancer, and explores the molecular mechanisms underlying its role in the disease. The results indicate that the abnormally high expression of MYBL2 promotes the proliferation of ovarian cancer cells mainly through the direct transcriptional regulation of centromere protein A (CENPA) expression. Therefore, targeting the MYBL2-CENPA axis may provide a new approach to the treatment of ovarian cancer.
We present the following article in accordance with the MDAR checklist (available at https://dx.doi.org/10.21037/tcr-21-175).
Methods
Gene expression profile data
Microarray data [GSE4122, GSE18520 (14), GSE12470 (15),GSE38666 (16), GSE27651 (17) and GSE26712 (18)] were downloaded from Gene Expression Omnibus (GEO). All of them meet the following criteria: (I) they all including human ovarian cancer samples. (II) Containing normal ovary samples. (III) Containing at least twenty samples. The R analysis process is borrowed from Liu et al. (19). The study was conducted in accordance with the Declaration of Helsinki (as revised in 2013).
Integrated analysis of microarray datasets
The Limma software package (20) in the R software was used to normalize the matrix data of each GEO data set and the base 2 logarithm conversion was used, and the DEGs between tumor and normal tissues was screened by Limma package. The R package “RobustRankAggreg” (21) is based on the Robust Rank Aggregation (RRA) method to perform genetic integration on DEGs determined from six data sets. Based on the null hypothesis of irrelevant inputs, the genes screened by this RRA method are always better than expected (21). |log2FC| ≥0.5, and adjust P value <0.05 were used.
Functional enrichment analysis of DEGs
To elucidate possible differences in biological processes, molecular functions, and cellular components with DEGs, GO enrichment analysis (22) and Kyoto Encyclopedia of Genes and Genomes (KEGG) pathway enrichment analysis (23) were performed.
Cell lines and culture
The SKOV3 and A2780 ovarian cell lines were purchased from ATCC (Manassas, VA, USA). Cells were cultured at 37 °C in DMEM (Hyclone, Logan, UT, USA) with 10% FBS (Hyclone) in a humidified incubator with 5% CO2.
Small interfering RNA transfection
Lipofectamine 3000 (Thermo Fisher Scientific, Waltham, MA, USA) was used to transfect the MYBL2 or CENPA siRNA (GenePharma, Shanghai, China) into cells, according to manufacturer’s instructions. The siRNA sequences were as follows: siMYBL2: 5′-CCCUGUCAGGUAUCAAAGA-3′ (24). siCENPA-1: 5′-UAACACAUAUUUCUCUUGCCA-3′; siCENPA-2: 5′-AAUUUAACACAUAUUUCUCUU-3′.
The qRT-PCR analysis
TRIzol reagent (Thermo Fisher Scientific) was used to extract the total RNA. Each experiment was performed for three times. The primer sequences were, as follows: GAPDH forward, 5′-CTGGGCTACACTGAGCACC-3′, GAPDH reverse, 5′-AAGTGGTCGTTGAGGGCAATG-3′; MYBL2 forward, 5′-CTTGAGCGAGTCCAAAGACTG-3′, MYBL2 reverse, 5′-AGTTGGTCAGAAGACTTCCCT-3′. CENPA forward, 5′-TTCCTCCCATCAACACAGTCG-3′; CENPA reverse, 5′-CACACCACGAGTGAATTTAACAC-3′.
Western blot
The total protein was extracted by RIPA buffer containing PMSF (Beyotime, Jiangsu, China). Then, 40 μg of total protein was separated on 10% polyacrylamide gels (Beyotime). Afterwards, After the 10% polyacrylamide gels (Beyotime) separation, the proteins were transferred onto PVDF membranes and blocked by 5% BSA. Subsequently, these PVDF membranes were incubated overnight. The antibodies are: mouse monoclonal anti-GAPDH (60004-1-Ig; Proteintech, Wuhan, China), Rabbit anti-MYBL2 (18896-1-AP; Proteintech), and Rabbit anti-CENPA (#2186; CST, Danvers, MA, USA). The protein bands were visualized using the ECL Western Blotting Substrate (Pierce, Rockford, IL, USA).
ChIP assay
The CHIP kit (Cell Signaling; Cat. #9005) was used. The sequences were, as follows: forward, 5′-TTATCTCAAAGCCCCGCCG-3′, reverse, 5′-TGACAACCCCGTGTCGTATC-3′;
Cell proliferation assay
Cells with siRNA-mediated knockdown were seeded in 96-well plates (6×103 per well). and was detected using a CCK-8Assay Kit (Dojindo, Kumamoto, Japan).
The EDU Assay Kit (GeneCopoeia, Rockville, MD, USA) was used to evaluate the proliferation of ovarian cells, according to manufacturer’s instructions. Briefly, these cells were treated with EdU for five hours.
Statistical analysis
SPSS Statistics was used for the analyses. All data are expressed as mean ± standard deviation (SD). Unpaired Student’s t-tests were used to evaluate the differences. P<0.05 was considered statistically significant.
Results
Identification of DEGs and functional enrichment analysis of DEGs
The details of the samples in the 6 data sets from the GEO datasets were listed in Table 1. After the integrated analysis, 291 DEGs were obtained, including 158 down-regulated genes (available online: https://cdn.amegroups.cn/static/public/tcr-21-175-1.xls) and 133 up-regulated genes (available online: https://cdn.amegroups.cn/static/public/tcr-21-175-2.xls). Top 20 down and up-regulated genes was showed in Figure 1A.
Table 1
Dataset | Reference | Platform | Number of samples (control/tumor) |
---|---|---|---|
GSE4122 | Tate DL, Mostafavi ZB, Mostafavi MT | GPL201 [HG-Focus] Affymetrix Human HG-Focus Target Array | 67 (14/34) |
GSE12470 | Yoshihara K, Tajima A, Komata D, Yamamoto T, et al. | GPL887 Agilent-012097 Human 1A Microarray (V2) G4110B (Feature Number version) |
53 (10/42) |
GSE18520 | Mok SC, Bonome T, Vathipadiekal V, Bell A, et al. | GPL570 [HG-U133_Plus_2] Affymetrix Human Genome U133 Plus 2.0 Array | 63 (10/53) |
GSE26712 | Bonome T, Levine DA, Shih J, Randonovich M, et al. | GPL96[HG-U133A] Affymetrix Human Genome U133A Array | 195 (10/185) |
GSE38666 | Lili LN, Matyunina LV, Walker LD, Benigno BB, et al. | GPL570 [HG-U133_Plus_2] Affymetrix Human Genome U133 Plus 2.0 Array | 45 (20/25) |
GSE27651 | King ER, Tung CS, Tsang YT, Zu Z, et al. | GPL570 [HG-U133_Plus_2] Affymetrix Human Genome U133 Plus 2.0 Array | 36 (6/30) |
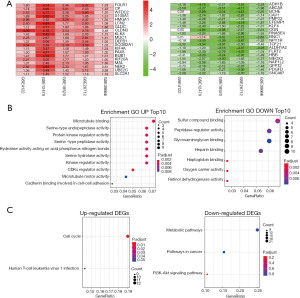
Enrichment analysis of GO and KEGG pathways was used to elucide the potential biological functions of the 291 DEGs. In the down-regulated group, they were significantly enriched in various biological binding (Figure 1B). In the up-regulated group, they showed most significantly enriched in microtubule binding. In addition, they showed a close correlation with biological activity, such as serine-type endopeptidase activity, protein kinase regulator activity, serine-type peptidase activity and so on (Figure 1B). The results of KEGG pathway enrichment analysis showed that the up-regulated genes were enriched in the cell cycle (Figure 1C). In addition, down-regulated genes are mainly involved in metabolic pathways, cancer pathways, and PI3K-Akt signaling pathways (Figure 1C).
Expression characteristics of CENPF, CENPA and CEP55 in human ovarian tissues
According to the results of GO enrichment and KEGG analysis, we know that the up-regulated DEGs are mainly related to the cell cycle, and the centromere protein had a very significant influence on cell cycle. Therefore, CENPF, CENPA and CEP55 proteins were chose for further research. We further studied the expression of these genes in the TCGA database. The GEPIA dataset (http://gepia.cancer-pku.cn/) was used to verified the expression patterns of the proteins in normal tissues and ovarian tissues. The mRNA expression levels of CENPF, CEP55 and CENPA in cancer tissues were higher than those in normal tissues (Figure 2A,B,C). The survival analysis was performed in the web (http://kmplot.com/analysis/index.php). Ovarian cancer patients with high expression of CENPA, CENPF or CEP55 had a lower overall survival rate than those with low expression (Figure 2D,E,F).
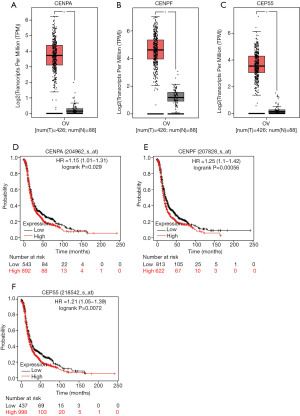
The inhibition of CENPA inhibits the proliferation of ovarian cells
Previous experiments have demonstrated that ovarian cancer patients with a high expression of CENPA have poor prognosis. In order to verify the role of CENPA in ovarian cancer, its expression was knocked down in SKOV3 and A2780 cell lines using two different small RNA sequences (siCENPA-1 and siCENPA-2). Compared with levels in the control group, the CENPA mRNA and protein levels were significantly lower in these two cell lines (Figure 3A,B). The CCK-8 results revealed that the decreased expression of CENPA significantly inhibits the proliferation of ovarian cell lines (Figure 3C). Similar results were obtained in the EDU assay (Figure 3D). These results indicate that CENPA greatly promotes the proliferation of ovarian cells.
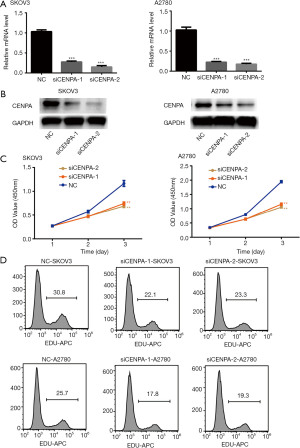
The expression of CENPA was regulated by MYBL2 in ovarian cancer cells
In order to study the regulatory mechanism of CENPA, we further studied CENPA in the TCGA database. We found that CENPA showed high consistency with MYBL2 (Figure 4A). At the same time, mRNA levels of MYBL2 were higher in cancer tissues compared to normal tissues (Figure 4B). Ovarian cancer patients with high MYBL2 expression showed worse overall survival (Figure 4C). The level of CENPA decreases with the knock down of MYBL2 in SKOVE cell line (Figure 4D).
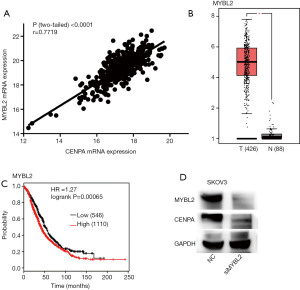
CENPA was directly regulated by MYBL2
In order to determine whether MYBL2 directly regulates the CENPA expression in ovarian cancer cells, ChIP assay was performed. The Cistrome database (http://cistrome.org/db/#/) was used to predict the binding ability. The results of the ChIP-seq from the Gertz et al. (25) revealed that MYBL2 can bind to the CENPA promotor in liver cells (Figure 5A). In order to verify the direct binding in ovarian cancer cells, the SKOV3 cell line was used for the ChIP assays. These results show that MYBL2 binds to the promoter region of CENPA (Figure 5B). In order to further confirm this result, the binding in the NC-SKOV3 and siMYBL2-SKOV3 cell lines was examined, and it was found that the binding of MYBL2 to the CENPA promoter significantly decreased (Figure 5B). Furthermore, the qRT-PCR results also revealed the same trend (P<0.01, Figure 5C). These data indicate that MYBL2 binds to the CENPA promoter region and promotes the CENPA expression.
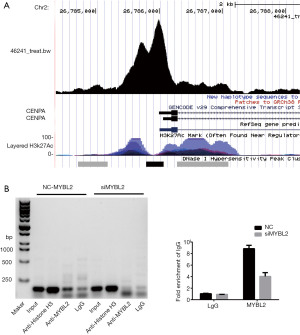
Discussion
Unlimited cell proliferation, metastatic, and drug resistance are all the characteristics of cancer cells that can lead to a malignant phenotype and affect prognosis. The inhibition of cell proliferation has become an important strategy for cancer treatment. MYBL2 is an important regulator of cell cycle. When it is dysregulated in cancer cells, abnormal proliferation occurs. In fact, MYBL2 is overexpressed in a variety of cancers and is associated with poor prognosis. Thus, Some investigators have used MYBL2 as a biomarker for prognosis and as a potential therapeutic target (6). For example, the high expression levels of CCNE1, KRT16 and MYBL2 are associated with worse relapse-free survival and overall survival (OS) in ER-negative/HER2-negative breast cancer (26). In patients with primary hepatocellular carcinoma, high expression of MYBL2 is associated with low OS (27). in human hepatocellular carcinoma, high expression of MYBL2 is associated with faster progression (28). MYBL2 can be used as a target for cancer therapy, according to some studies. Ginkgetin regulates b-Myb by modulating miR-34a, and may be used to induce G2 arrest for the treatment of colorectal cancer (29).
An important sign of cancer is the unlimited proliferation of cancer cells (30), and this is mainly caused by the disruption in cell cycle regulation (31). The overexpression of MYBL2 is associated with the increase in cell proliferation, and plays an important role in cancer progression (32). However, the molecular mechanism by which mybl2 promotes cell proliferation in lung cancer remains unclear. A previous study revealed that MYBL2 binds to the A3B promoter, causing the transactivation in breast cancer cells (33). In addition, in the present study, it was found that MYBL2 promotes ovarian cell proliferation by directly regulating the expression of CENPA. This pathway further contributes to the pathogenesis and progression of ovarian cancer, providing a basis for treatment.
CENPA is a fundamental component of the human centromere and plays an important role in cell cycle, survival and genetic stability. CENPA expression is altered in various types of human cancers. In epithelial ovarian cancer, high levels of CENPA are significantly correlated with poor survival (34). In osteosarcoma, the expression of CENPA is significantly associated with progression, and patients with a high expression of CENPA have poor overall and recurrence-free survival (35). In triple-negative breast cancer, BIRC5, CENPA and FAM64A are specifically upregulated, and the high levels of these three genes are associated with poor prognosis, suggesting the clinical application as therapeutic targets (36). In lung adenocarcinoma, CENPA upregulation predicts a poor OS in patients, and CENPA downregulation may attenuate the aggressive phenotype of lung adenocarcinoma cells (37,38). In glioblastoma, CENPA is consistently expressed in GSC cultures, but is not expressed in NSC cultures, suggesting that this should be further explored as therapeutic targets (39). In hepatocellular carcinoma, CENPA is strongly associated with hepatocellular carcinoma cell growth and division (40).
Acknowledgments
Funding: This work was supported by grants from
Footnote
Reporting Checklist: The authors have completed the MDAR checklist. Available at https://dx.doi.org/10.21037/tcr-21-175
Conflicts of Interest: All authors have completed the ICMJE uniform disclosure form (available at https://dx.doi.org/10.21037/tcr-21-175). The authors have no conflicts of interest to declare.
Ethical Statement: The authors are accountable for all aspects of the work in ensuring that questions related to the accuracy or integrity of any part of the work are appropriately investigated and resolved. The study was conducted in accordance with the Declaration of Helsinki (as revised in 2013). Institutional ethical approval and informed consent were waived.
Open Access Statement: This is an Open Access article distributed in accordance with the Creative Commons Attribution-NonCommercial-NoDerivs 4.0 International License (CC BY-NC-ND 4.0), which permits the non-commercial replication and distribution of the article with the strict proviso that no changes or edits are made and the original work is properly cited (including links to both the formal publication through the relevant DOI and the license). See: https://creativecommons.org/licenses/by-nc-nd/4.0/.
References
- Bray F, Ferlay J, Soerjomataram I, et al. Global cancer statistics 2018: GLOBOCAN estimates of incidence and mortality worldwide for 36 cancers in 185 countries. CA Cancer J Clin 2018;68:394-424. [Crossref] [PubMed]
- Siegel RL, Miller KD, Jemal A. Cancer statistics, 2019. CA Cancer J Clin 2019;69:7-34. [Crossref] [PubMed]
- Vang R, Shih IeM, Kurman RJ. Ovarian low-grade and high-grade serous carcinoma: pathogenesis, clinicopathologic and molecular biologic features, and diagnostic problems. Adv Anat Pathol 2009;16:267-82. [Crossref] [PubMed]
- Coleman RL, Monk BJ, Sood AK, et al. Latest research and treatment of advanced-stage epithelial ovarian cancer. Nat Rev Clin Oncol 2013;10:211-24. [Crossref] [PubMed]
- Roussel M, Saule S, Lagrou C, et al. Three new types of viral oncogene of cellular origin specific for haematopoietic cell transformation. Nature 1979;281:452-5. [Crossref] [PubMed]
- Musa J, Aynaud MM, Mirabeau O, et al. MYBL2 (B-Myb): a central regulator of cell proliferation, cell survival and differentiation involved in tumorigenesis. Cell Death Dis 2017;8:e2895. [Crossref] [PubMed]
- Zhang K, Fu G, Pan G, et al. Demethylzeylasteral inhibits glioma growth by regulating the miR-30e-5p/MYBL2 axis. Cell Death Dis 2018;9:1035. [Crossref] [PubMed]
- Zhang X, Lv QL, Huang YT, et al. Akt/FoxM1 signaling pathway-mediated upregulation of MYBL2 promotes progression of human glioma. J Exp Clin Cancer Res 2017;36:105. [Crossref] [PubMed]
- Fan X, Wang Y, Jiang T, et al. B-Myb Mediates Proliferation and Migration of Non-Small-Cell Lung Cancer via Suppressing IGFBP3. Int J Mol Sci 2018;19:1479. [Crossref] [PubMed]
- Geng GJ, Yang YT, Jiang J, et al. MicroRNA-30a suppresses non-small-cell lung cancer by targeting Myb-related protein B. Exp Ther Med 2018;15:1633-9. [PubMed]
- Chen J, Chen X. MYBL2 Is Targeted by miR-143-3p and Regulates Breast Cancer Cell Proliferation and Apoptosis. Oncol Res 2018;26:913-22. [Crossref] [PubMed]
- Frau M, Ladu S, Calvisi DF, et al. Mybl2 expression is under genetic control and contributes to determine a hepatocellular carcinoma susceptible phenotype. J Hepatol 2011;55:111-9. [Crossref] [PubMed]
- Müller-Tidow C, Wang W, Idos GE, et al. Cyclin A1 directly interacts with B-myb and cyclin A1/cdk2 phosphorylate B-myb at functionally important serine and threonine residues: tissue-specific regulation of B-myb function. Blood 2001;97:2091-7. [Crossref] [PubMed]
- Mok SC, Bonome T, Vathipadiekal V, et al. A gene signature predictive for outcome in advanced ovarian cancer identifies a survival factor: microfibril-associated glycoprotein 2. Cancer Cell 2009;16:521-32. [Crossref] [PubMed]
- Yoshihara K, Tajima A, Komata D, et al. Gene expression profiling of advanced-stage serous ovarian cancers distinguishes novel subclasses and implicates ZEB2 in tumor progression and prognosis. Cancer Sci 2009;100:1421-8. [Crossref] [PubMed]
- Lili LN, Matyunina LV, Walker LD, et al. Molecular profiling predicts the existence of two functionally distinct classes of ovarian cancer stroma. Biomed Res Int 2013;2013:846387. [Crossref] [PubMed]
- King ER, Tung CS, Tsang YT, et al. The anterior gradient homolog 3 (AGR3) gene is associated with differentiation and survival in ovarian cancer. Am J Surg Pathol 2011;35:904-12. [Crossref] [PubMed]
- Bonome T, Levine DA, Shih J, et al. A gene signature predicting for survival in suboptimally debulked patients with ovarian cancer. Cancer Res 2008;68:5478-86. [Crossref] [PubMed]
- Liu X, Wu J, Zhang D, et al. Identification of Potential Key Genes Associated With the Pathogenesis and Prognosis of Gastric Cancer Based on Integrated Bioinformatics Analysis. Front Genet 2018;9:265. [Crossref] [PubMed]
- Ritchie ME, Phipson B, Wu D, et al. limma powers differential expression analyses for RNA-sequencing and microarray studies. Nucleic Acids Res 2015;43:e47. [Crossref] [PubMed]
- Kolde R, Laur S, Adler P, et al. Robust rank aggregation for gene list integration and meta-analysis. Bioinformatics 2012;28:573-80. [Crossref] [PubMed]
- Huang da W. Sherman BT, Lempicki RA. Systematic and integrative analysis of large gene lists using DAVID bioinformatics resources. Nat Protoc 2009;4:44-57. [Crossref] [PubMed]
- Yu G, Wang LG, Han Y, et al. clusterProfiler: an R package for comparing biological themes among gene clusters. OMICS 2012;16:284-7. [Crossref] [PubMed]
- Hasson SA, Kane LA, Yamano K, et al. High-content genome-wide RNAi screens identify regulators of parkin upstream of mitophagy. Nature 2013;504:291-5. [Crossref] [PubMed]
- Gertz J, Savic D, Varley KE, et al. Distinct properties of cell-type-specific and shared transcription factor binding sites. Mol Cell 2013;52:25-36. [Crossref] [PubMed]
- Shao N, Yuan K, Zhang Y, et al. Identification of key candidate genes, pathways and related prognostic values in ER-negative/HER2-negative breast cancer by bioinformatics analysis. J BUON 2018;23:891-901. [PubMed]
- Guan Z, Cheng W, Huang D, et al. High MYBL2 expression and transcription regulatory activity is associated with poor overall survival in patients with hepatocellular carcinoma. Curr Res Transl Med 2018;66:27-32. [Crossref] [PubMed]
- Calvisi DF, Simile MM, Ladu S, et al. Activation of v-Myb avian myeloblastosis viral oncogene homolog-like2 (MYBL2)-LIN9 complex contributes to human hepatocarcinogenesis and identifies a subset of hepatocellular carcinoma with mutant p53. Hepatology 2011;53:1226-36. [Crossref] [PubMed]
- Lee YJ, Kang YR, Lee SY, et al. Ginkgetin induces G2-phase arrest in HCT116 colon cancer cells through the modulation of b-Myb and miRNA34a expression. Int J Oncol 2017;51:1331-42. [Crossref] [PubMed]
- Hainaut P, Plymoth A. Targeting the hallmarks of cancer: towards a rational approach to next-generation cancer therapy. Curr Opin Oncol 2013;25:50-1. [Crossref] [PubMed]
- Dominguez-Brauer C, Thu KL, Mason JM, et al. Targeting Mitosis in Cancer: Emerging Strategies. Mol Cell 2015;60:524-36. [Crossref] [PubMed]
- Iness AN, Felthousen J, Ananthapadmanabhan V, et al. The cell cycle regulatory DREAM complex is disrupted by high expression of oncogenic B-Myb. Oncogene 2019;38:1080-92. [Crossref] [PubMed]
- Chou WC, Chen WT, Hsiung CN, et al. B-Myb Induces APOBEC3B Expression Leading to Somatic Mutation in Multiple Cancers. Sci Rep 2017;7:44089. [Crossref] [PubMed]
- Qiu JJ, Guo JJ, Lv TJ, et al. Prognostic value of centromere protein-A expression in patients with epithelial ovarian cancer. Tumour Biol 2013;34:2971-5. [Crossref] [PubMed]
- Gu XM, Fu J, Feng XJ, et al. Expression and prognostic relevance of centromere protein A in primary osteosarcoma. Pathol Res Pract 2014;210:228-33. [Crossref] [PubMed]
- Zhang C, Han Y, Huang H, et al. Integrated analysis of expression profiling data identifies three genes in correlation with poor prognosis of triple-negative breast cancer. Int J Oncol 2014;44:2025-33. [Crossref] [PubMed]
- Wu Q, Chen YF, Fu J, et al. Short hairpin RNA-mediated down-regulation of CENP-A attenuates the aggressive phenotype of lung adenocarcinoma cells. Cell Oncol (Dordr) 2014;37:399-407. [Crossref] [PubMed]
- Liu WT, Wang Y, Zhang J, et al. A novel strategy of integrated microarray analysis identifies CENPA, CDK1 and CDC20 as a cluster of diagnostic biomarkers in lung adenocarcinoma. Cancer Lett 2018;425:43-53. [Crossref] [PubMed]
- Stangeland B, Mughal AA, Grieg Z, et al. Combined expressional analysis, bioinformatics and targeted proteomics identify new potential therapeutic targets in glioblastoma stem cells. Oncotarget 2015;6:26192-215. [Crossref] [PubMed]
- Li B, Pu K, Wu X. Identifying novel biomarkers in hepatocellular carcinoma by weighted gene co-expression network analysis. J Cell Biochem 2019; Epub ahead of print. [Crossref] [PubMed]