A seven-lncRNA signature for predicting prognosis in breast carcinoma
Introduction
Breast cancer is the most common malignancy other than cutaneous carcinoma and is the second main cause of carcinoma-related death for females worldwide (1). In 2017, there were almost 252,710 new confirmed cases of breast cancer in America, and approximately 40,610 deaths (2,3). Recent increasing evidence indicates that early diagnosis and treatment are beneficial to the prognosis of breast cancer. However, the currently used biological markers including BRCA1/2, CA549, carcinoembryonic antigen, and so on are hyposensitive and nonspecific for early breast cancer diagnosis (4). Thus, there is an urgent need for breast cancer detection and risk stratification to identify a sensitive and specific biosignature for early diagnosis, to distinguish patients with breast carcinoma. Previous studies have indicated that long non-coding RNAs (lncRNAs) play crucial roles in the tumorigenesis and progression of breast cancer, and that some lncRNAs could be used as latent diagnostic biomarkers (5,6). LncRNAs are RNA transcripts greater than 200 bp in length, with scarcely any ability to be translated into protein (7). Currently, increasing evidence indicates that lncRNAs play a significant role in the apoptosis, proliferation, development, metastasis, invasion, and recurrence of various tumors (8-10). Recent researches have shown that altered lncRNA expression profiles are associated with the disease progression and survival in patients with breast cancer, revealing the potency of lncRNA as biological markers for cancer progression (11-13). In this study, we applied TCGA database to explore the difference in lncRNA expression profiles between breast cancer and adjacent normal tissues, and to identify potential lncRNA biomarkers, for predicting the prognosis of breast cancer patients. These outcomes can offer new insights into the lncRNA-based molecular mechanisms of breast cancer. We present the following article in accordance with the REMARK reporting checklist (available at https://dx.doi.org/10.21037/tcr-21-747).
Methods
Ethical statement
The study was conducted in accordance with the Declaration of Helsinki (as revised in 2013).
Breast cancer patient datasets
IlluminaHiSeq RNA-Seq data including the mRNA expression and corresponding clinical information for 1,097 breast cancer tissues and 113 adjacent non-tumor tissues was acquired from TCGA (https://tcga-data.nci.nih.gov/tcga/). Among the 1,097 breast cancer samples, 184 patients with a final follow-up of 0, unclear last survival status, or samples with no corresponding expression values were excluded. Finally, 913 breast carcinoma cases were included in the study for further analysis. These cases were classified into two groups, namely the training dataset (n=608) and testing dataset (n=305), in line with the survival status by 3-fold cross validation.
LncRNA differential expression profiles
In this study, the original breast cancer mRNA expression profiles (level 3 data) were obtained from TCGA data. In accordance with the annotation from the GENCODE project (http://www.gencodegenes.org), we obtained the lncRNA expression data by repurposing the probes in the mRNA expression profiles for lncRNA. The converted data (antisense, lincRNA, and sense_intronic) were regarded as lncRNA. The RNA-Seq data for breast cancer included 14,441 lncRNA and 19754 mRNA expression profiles (14). The differential expression profile of lncRNA was calculated using R or the Bioconductor package in edgeR (15). The differentially expressed genes (DEGs) of the dataset with |log(fold change)| ≥1 and adjusted P value <0.05 were regarded as the selection criteria to identify differentially expressed lncRNAs between breast cancer and adjacent normal tissues in the testing and training datasets.
Establishment of a prognostic model associated with lncRNA
The relationship between the lncRNA expression levels and overall survival (OS) of patients with breast cancer was analyzed using the univariate Cox model with the “survival” analysis software package of R software. The common lncRNAs selected from the training and testing datasets were considered statistically significant in the univariate Cox analysis when P values <0.05. The stepwise multivariate Cox regression analysis of the AIC (Akaike Information Criterion, assessing the goodness of fit of a statistical model) test yielded a predictive model with the optimal interpretation and information effectiveness, and the risk scores of 608 patients in the training dataset were determined. Then, by the corresponding multivariate analysis of the identified lncRNA signature, the coefficients of each prognostic lncRNA in the risk scoring model were obtained (16). Finally, a prognostic model associated with lncRNA was established to assess survival risk, as described below:
In this formula, N represents the number of prognostic lncRNAs, Coei is the coefficient of the lncRNAi in the multivariate Cox regression analysis, EVi represents the expression value of the lncRNAi. LncRNAs with a Coei less than 0 are considered protective lncRNAs, whereas lncRNAs with a Coei greater than 0 are considered high-risk lncRNAs. The predictive lncRNA model was applied to count the risk scores of 608 patients. Using the median risk score as a threshold, we classified breast carcinoma patients into high-risk and low-risk groups. The OS curve was obtained using the Kaplan-Meier method, and the difference in OS between different groups was calculated using a two-sided log-rank test. P<0.05 was regarded as a statistically significant difference. To investigate the sensitivity and specificity of the lncRNA prognostic model for predicting clinical outcomes, we calculated the area under the curve (AUC) of the time-dependent ROC curve within 3 and 5 years of the survival ROC R package (17), and drew high- and low-risk heatmaps. All analyses were performed using R/BioConductor (version 3.5.1). The value and stability of the survival prognosis in patients with breast cancer were predicted using the test set and complete set validation regression models.
Use of prognostic characteristics independent of lncRNA for survival prediction of other clinical variables and the relationship between prognoses at different levels
Univariate Cox regression was applied to analyze the prognostic characteristics of lncRNA and the clinical variables (containing age, gender, race, TNM stage, estrogen receptor status, progesterone receptor status, Her2 receptor status, and triple-negative breast cancer) to determine their relationships with patient OS in the whole data set. Multivariate Cox regression analysis was then performed, with OS as the dependent variable and lncRNA risk scores and other clinical features as the explaining variables, to examine whether the predictive power of the lncRNA signature was independent of other clinical factors. Data stratification analysis was further conducted for clinical features with P values <0.01 to determine whether the lncRNA signatures could offer predictive capacity within the same clinical factor. According to age, progesterone receptor status, HER-2 expression of ER status, lymph node metastasis status, and pathological stage, the prognostic value using multiple lncRNA signatures in patients with breast cancer was calculated using the Kaplan-Meier method. The predictive value of the prognostic model of LncRNA signatures in breast cancer patients with overall and different subgroups (age, ER status, Progesterone receptor status, HER-2 expression, lymph node metastasis status, and pathological stage) was also accessed using the Kaplan-Meier method.
Construction of protein-encoding gene and predicted weighted co-expression network of lncRNA signatures
When we performed differential gene expression analysis, we also obtained protein-coding genes that were differentially expressed in breast carcinoma and adjacent normal tissues in the testing and training sets. The differentially expressed protein-coding genes common to the training and testing sets were applied to construct a weighted gene co-expression network for depicting the relevant pattern expression profile. Prognostic LncRNA signatures were linked together as external information to determine the coding genes associated with the respective LncRNA signatures. The WGCNA can be used to estimate the importance of predicting prognostic lncRNA signatures and their module members. We use paired Pearson correlation to evaluate the co-expression correlation between the whole data-set subjects in adjacent matrices. Based on the description of standard scale-free networks, an optimal soft threshold was automatically calculated and generated. In this research, the soft threshold was set as β=4 (no scale R2=0.85) (Figure S1). Co-expressed genes were searched using the function “networkScreening“ based on GS and MM(18). Using this function, a series of indicators were obtained, including the weighted P value (P. Weighted) of the correlation between the coding genes and lncRNAs, the FDR-corrected weighted P value (q.Weighted), the weighted correlation coefficient (cor. Weighted), and Fisher Z test results for weighted correlation. Similar to the normal P value, the smaller the p.Weighted, the stronger was the correlation between the coding gene and LncRNA. We used the corrected q.Weighted <0.0l to screen the protein-encoding genes highly associated with LncRNA.
Statistical analysis
KEGG functional enrichment analysis of these protein-encoding genes highly associated with LncRNA was performed using the R package “clusterProfiler” and “pathview”. The KEGG pathway with a false discovery rate (FDR) <0.05 was considered statistically significant. Gene Set Variation Analysis (GSVA) was performed between the high- and low-risk score groups (19), and the adjusted P value (adj. P Val) <0.05 was regarded statistically significant.
Results
Breast cancer patients’ sample information
The 913 included cases of breast cancer were divided into the training dataset with 608 cases and the testing dataset with 305 cases, based on the survival status by 3-fold cross validation. The clinical information of the patients included in the training and test datasets is shown in Table 1.
Table 1
Variable | Training dataset (n=608) | Testing dataset (n=305) |
---|---|---|
Age (year), | 58.00±13.02 | 58.45±13.46 |
Gender, n (%) | ||
Female | 602 (99.0) | 302 (99.0) |
Male | 6 (1.0) | 3 (1.0) |
Race, n (%) | ||
White | 427 (70.2) | 214 (70.2) |
Asian | 28 (4.6) | 10 (3.3) |
Black | 108 (17.8) | 58 (19.0) |
NA | 45 (7.4) | 23 (7.5) |
Stage, n (%) | ||
Stage I | 104 (17.1) | 53 (17.4) |
Stage II | 344 (56.6) | 162 (53.1) |
Stage III | 143 (23.5) | 71 (23.3) |
Stage IV | 14 (2.3) | 14 (4.6) |
NA | 3 (0.5) | 5 (1.6) |
Estrogen receptor status, n (%) | ||
Negative | 131 (21.5) | 68 (22.3) |
Positive | 458 (75.3) | 228 (74.8) |
NA | 19 (3.2) | 9 (2.9) |
Progesterone receptor status, n (%) | ||
Negative | 199 (32.7) | 93 (30.5) |
Positive | 389 (64.0) | 202 (66.2) |
NA | 20 (3.3) | 10 (3.3) |
Her2 receptor status, n (%) | ||
Negative | 448 (73.7) | 214 (70.2) |
Positive | 98 (16.1) | 50 (16.4) |
NA | 62 (10.2) | 41 (13.4) |
Number of lymph nodes positive (mean) | 2.37 | 2.42 |
Cancer status, n (%) | ||
Tumor free | 501 (82.4) | 242 (79.3) |
With tumor | 42 (6.9) | 26 (8.5) |
NA | 65 (10.7) | 37 (12.2) |
Subtype, n (%) | ||
Luminal A | 288 (47.4) | 144 (47.2) |
Luminal B | 56 (9.2) | 40 (13.1) |
HER2A enriched | 18 (3.0) | 11 (3.6) |
Triple negative | 95 (15.6) | 47 (15.4) |
NA | 151 (24.8) | 63 (20.7) |
Median survival time (month) | 14.6 | 17.3 |
Differentially expressed lncRNA profiles of breast cancer
The lncRNA expression profiles of breast cancer tissues (n=608) and adjacent normal tissues (n=113) in the training dataset obtained from TCGA were analyzed. All 3084 differentially expressed lncRNAs were discovered (logFC >1 or logFC <−1, p.adj <0.05). In the differentially expressed lncRNAs, 2288 lncRNAs were upregulated whereas 796 lncRNAs were decreased. The lncRNA expression profiles of breast cancer tissues (n=305) and adjacent normal (n=113) tissues in the testing dataset were also analyzed. Of the 2855 differentially expressed lncRNAs (logFC >1 or logFC <−1, p.adj <0.05), 1987 lncRNAs were upregulated and 868 lncRNAs were downregulated. After the intersection of different lncRNAs expressed in the training and testing datasets, 2,547 common differentially expressed lncRNAs were obtained, of which 1,807 lncRNAs were upregulated and 740 were downregulated. Unsupervised hierarchical clustering analysis results indicated that the expression of differentially expressed lncRNAs clearly distinguished the breast cancer samples from the normal samples (Figure 1).
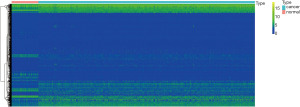
Establishment of the seven-lncRNA signature predictive model
Univariate Cox regression was applied between different lncRNA expression profiles and the prognoses of patients with breast carcinoma in the training and testing datasets and the combined cohort; The results showed that 179 lncRNAs in the training dataset and 216 lncRNAs in the testing dataset (P values <0.05 for both) were significantly related with the OS of patients with breast carcinoma. Further, 204 lncRNAs in the combined cohort were significantly related with the OS in patients with breast carcinoma (P value <0.05). Further, 10 lncRNAs (AC025016.1, LINC02037, MAPT-AS1, RP1-37C10.3, RP11-120K18.2, RP11-344E13.4, RP11-454P21.1, RP11-616M22.1, SPACA6P-AS, Xxyac-YM21GA2.7) screened out from the training and testing datasets and the common combined cohort were significantly related with the OS of patients with breast carcinoma (Table S1).
These 10 lncRNAs were analyzed by multivariate Cox regression, and the minimum AIC was 624.43; of these, 7 lncRNAs were screened out to construct a Cox proportional regression model (Table 2). Univariate Cox regression analysis demonstrated that the regression coefficients of 6 lncRNAs (RP11-344E13.4, LINC02037, RP11-454P21.1, RP11-616M22.1, SPACA6P-AS, RP1-37C10.3) were greater than 0, HR (hazard ratio) = exp(coef) >1, defining them as risk lncRNA, which are inversely related to breast cancer survival. The regression coefficient of one lncRNA (MAPT-AS1) was less than 0, and HR (hazard ratio) = exp(coef) <1, defining it as a protective lncRNA, which was positively correlated with breast cancer survival. In line with the regression coefficients of multivariate Cox analysis of the seven lncRNAs, the prognostic index was imputed by: LncRNA-based Risk Score =0.2906 × expression level of LINC02037-0.0724 × expression level of MAPT-AS + 0.3182 × expression level of RP1-37C10.3 + 0.1631 × expression level of RP11-344E13.4+0.1910 × expression level of RP11-454P21.1 +0.2308 × expression level of RP11-616M22.1+0.1674× expression level of SPACA6P-AS.
Table 2
LncRNAs | coef | exp (coef) | se (coef) | z | P |
---|---|---|---|---|---|
LINC02037 | 0.2906 | 1.3372 | 0.0911 | 3.19 | 0.0014 |
MAPT-AS1 | −0.0724 | 0.9302 | 0.0458 | −1.58 | 0.1137 |
RP1-37C10.3 | 0.3182 | 1.3746 | 0.1209 | 2.63 | 0.0085 |
RP11-344E13.4 | 0.1631 | 1.1772 | 0.0686 | 2.38 | 0.0173 |
RP11-454P21.1 | 0.1910 | 1.2105 | 0.1029 | 1.86 | 0.0633 |
RP11-616M22.1 | 0.2308 | 1.2595 | 0.0893 | 2.58 | 0.0098 |
SPACA6P-AS | 0.1674 | 1.1823 | 0.0995 | 1.68 | 0.0923 |
According to this, the risk value about each sample can be calculated, and the samples can be classified as high-risk (n=304) and low-risk groups (n=304) based on median risk value. Among these, the lncRNA-based Risk Score =0.957 is the cut-off value; the lncRNA-based risk score is greater than 0.957 for the high-risk group, and less than 0.957 for the low-risk group (Figure 2A-2C). The Kaplan-Meier curves of different groups based on the seven-lncRNA signature were notably different (Figure 2D); patients with high-risk scores had worse OS (median OS of 80 vs. 148 months, P<0.001) than those with a low-risk score. The HR of the risk score derived from the univariate Cox proportional hazards regression method was 4.15 (95% CI, 2.38–7.25), which was consistent with the results of multivariate Cox proportional hazards regression analysis, was 2.70 (95% CI, 1.43–5.12) after being adjusted for the clinical pathological feature covariate. The time-dependent ROC and AUC of ROC curves were applied to assess the prognostic capacity of the seven-lncRNA signature model. The AUC results indicated that each of these seven-lncRNA models had high diagnostic accuracy as a breast cancer biomarker. In the 3- and 5-year OS, the AUC about the seven-lncRNA biosignature prognostic model was 0.745 and 0.742 (Figure 2E), respectively, indicating the diagnostic accuracy of the seven lncRNA combination models in predicting prognosis. Table S2 shows the 1-, 2-, 3-, 4-, and 5-year survival rates of the high-risk and low-risk groups.
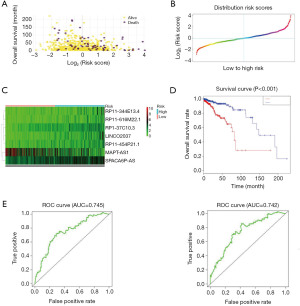
Performance evaluation of the seven-lncRNA features for survival prediction in the test and whole datasets
The risk calculation formula of the training dataset samples was applied to calculate the lncRNA risk scores of each sample in the test dataset. Based on the median of the lncRNA risk score value, samples were classified under the high- and low-risk groups. The survival curves of high- and low-risk groups were plotted respectively in the testing dataset, and ROC curves were drawn at the same time. The results showed that the overall risk in the high-risk group was lower than that in the low-risk group of the testing dataset (P<0.001) (Figure 3A). The risk assessment model was more stable in predicting the 3- and 5-year survival prognosis in patients with breast carcinoma (the AUC of the ROC curve of the 3- and 5-year survival rates was 0.86 and 0.867, respectively) (Figure 3B), and the OS rate in the high-risk group was also lower than that in the low-risk group of the combined cohort (P<0.001) (Figure 3C). Moreover, the risk assessment model is also stable for predicting 3- and 5-year survival prognosis in patients with breast carcinoma (the AUC of the ROC curve of the 3- and 5-year survival rates was 0.771 and 0.780, respectively) (Figure 3D).
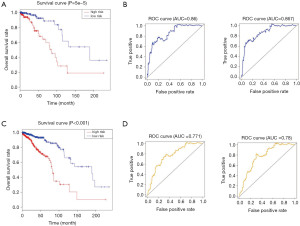
Independence of the seven-lncRNA signature in predicting survival of routine clinical factors and the relationship between the prognosis at different levels
To determine if the prognostic power of the seven-lncRNA feature is independent of routine clinical factors in patients with breast cancer, we used the seven-lncRNA features and other routine clinical factors as explanatory variables and the patient OS as the dependent variable to conduct multivariate Cox regression analysis. The results of the multivariate Cox regression analysis demonstrated that the seven-lncRNA feature showed an independent relevance with OS after modulation for routine clinical factors in the training dataset, testing dataset, and whole dataset, including age, gender, race, TNM stage Estrogen receptor status, Progesterone receptor status, Her2 receptor status, Triple-negative breast cancer (HR >1, P<0.01) (Table S3). The median OS in the low-risk group (12.64 years) was notably longer than that in the high-risk group (6.72 years) (Log rank χ2=42.256, P<0.01) (Figure 3C). Except for pathological stage I and positive Her2, the OS rate of patients with low-risk scores in the stratification was higher than that in high-risk group (P<0.05) (Table 3).
Table 3
Variable | Low risk | High risk | Log-rank χ2 |
---|---|---|---|
Age (year) | |||
<60 | 241 | 250 | 15.765** |
≥60 | 216 | 206 | 30.659** |
ER status | |||
ER positive | 378 | 308 | 26.403** |
ER negative | 66 | 133 | 6.311* |
PR status | |||
PR positive | 333 | 258 | 19.036** |
PR negative | 108 | 184 | 13.213** |
Her2 status | |||
Her2 positive | 56 | 92 | 0.392 |
Her2 negative | 345 | 317 | 27.954** |
Lymph node metastasis | |||
Lymph node negative | 208 | 188 | 19.314** |
Lymph node [1–3] positive | 122 | 128 | 9.838** |
Lymph node (4-) positive | 81 | 71 | 7.802** |
Pathological staging | |||
Stage I | 89 | 68 | 0.338 |
Stage II | 247 | 259 | 14.434** |
Stage III | 107 | 107 | 12.826** |
Stage IV | 10 | 18 | 9.829** |
*, P<0.05; **, P<0.01.
Construction of a weighted co-expression network of protein coding genes and the seven-LncRNA signature
In total, 5242 differentially expressed protein coding genes were identified in the training dataset (logFC >1 or logFC <−1, p.adj <0.05). In the testing dataset, 5042 differentially expressed protein encoding genes (logFC >1 or logFC <−1, p.adj <0.05) were identified. After intersecting the significant differentially expressed protein coding genes in the training and testing dataset, 4,340 common differentially expressed protein coding genes were obtained. The expression profiles of these 4,340 differentially expressed protein-encoding genes were screened out in the entire cohort and used to construct a weighted gene co-expression network. After removing 154 outlier samples, the remaining tumor samples were hierarchically clustered and the lncRNA expression profiles of the corresponding samples were plotted (Figure S2A,S2B). The linear regression results showed that when the soft threshold β=4, the connectivity correlation coefficient of each node in the network was 0.88, consistent with the scale-free network characteristics (Figure S3). By associating the seven-lncRNA expression profile data, the protein-coding genes (q. Weight <0.01) highly correlated with these seven lncRNAs was found. Among 4,340 DEGs, there were 756, 120, 84, 341, 346, 748, and 637 protein-coding genes were co-expressed with MAPT-AS1, RP11-344E13.4, LINC02037, RP11-454P21.1, RP11-616M22.1, SPACA6P-AS, and RP1-37C10.3, respectively.
Identifying the functions of the seven-lncRNA signature
The potential prognostic function of the lncRNAs was investigated using KEGG functional enrichment analysis, which showed that the co-expressed genes RP11.616M22.1 and LINC02037 were not enriched to obtain the KEGG pathway; the other lncRNA-enriched KEGG pathways are shown in Figure S4A-S4E. The KEGG pathways enriched by MAPT-AS1 and RP1-37C10.3 include cellular aging, p53 signal pathway, and Fanconi anemia pathway (Figure S4A,S4B); The KEGG pathways enriched by MAPT-AS1, RP1-37C10.3, and RP11-344E13.4 include cell cycle, DNA replication, homologous recombination, and progesterone-mediated oocyte maturation (Figure S4A-S4C); the KEGG pathways enriched by RP11-454P21.1 include Systemic lupus erythematosus, Alcoholism, and PPAR signaling pathway (Figure S4D); The KEGG pathways enriched by SPACA6P-AS includes cellular adhesion molecules (CAMs), Fanconi anemia pathway, cytokine-cytokine receptor interaction, and ABC transporters (Figure S4E). Gene set variation analysis (GSVA) results between the high- and low-risk scores of the training and testing dataset showed that high-risk scoring group in the training dataset was significantly increased and included maturity onset diabetes of the young, olfactory transduction, pentose and glucuronate interconversion, and so on. Further, in the low-risk group, the gene functions included acute myeloid leukemia (AML), purine metabolism, colorectal cancer, and so on (Figure S5A). The high-risk scoring group in the testing dataset was significantly increased and included maturity onset diabetes of the young, bladder cancer, ascorbate and aldarate metabolism, etc. Further, in the low-risk scoring group, the gene functions included AML, Erbb signal pathway, renal cell cancer, etc. (Figure S5B).
Discussion
Breast cancer is a serious hazard to women’s health, even if combination treatment has decreased the risk of relapse. Despite improvements in breast carcinoma therapy over the past few decades, there are limited treatments for advanced breast cancer because of the lack of accurate molecular targets. Hence, exploring the molecular mechanisms participating in breast carcinoma development and progression is rather significant. Further, there is an urgent need for improved biomarkers for tumor-specific prognosis and progression. So far, increasing evidences indicate that lncRNAs participate in tumorigenesis and prognosis. Integrated genomics research has demonstrated the role of lncRNA with increasing focus, and more potential lncRNAs need to be examined to improve the clinical outcomes in breast cancer patients. Malfunction of lncRNAs may be present in kinds of cancers and is notably associated with cancer prognosis (20-23). Some dysregulated lncRNAs like HOTTIP, HOTAIR, and LINC00978 have been found to be related to the prognosis of patients with breast cancer (24-26). These findings indicate that lncRNAs may act as potential biomarkers for survival prediction in breast cancer.
We conducted an integrated analysis of lncRNA expression profiles and the corresponding clinical information about patients with breast cancer from TCGA database. First, differentially expressed lncRNAs were filtered out between breast cancer and non-cancerous tissues. Then, a seven-lncRNA signature based on expression was developed in the training dataset using sample 3-fold cross validation and Cox proportional regression analysis, for differentiating patients into high- and low-risk groups with notable differences in OS. ROC curve analysis indicated that the seven-lncRNA combinatorial signature showed prognostic predictive ability. The prognostic performance of seven-lncRNA combinatorial signature was fully verified on the testing and complete dataset, indicating that the lncRNA combinatorial marker model had superior repeatability. Further multivariate Cox regression and stratified analysis showed that the features of seven-lncRNA had independent prognostic ability similar to other clinicopathological variables and these could be used to predict the survival of breast carcinoma patients. The currently established prognostic predictors for breast carcinoma include first-generation prognostic markers (21 gene test, MammaPrint, Genomic Grade Index) (27) and second-generation prognostic markers (Prosigna, EndoPredict, breast cancer index) (28). The National Cancer Network (NCCN) guidelines recommend breast cancer genetic testing for newly diagnosed breast carcinoma with stage I or II, ER-positive, and node-negative breast carcinoma; lymph node-positive [1–3], ER-positive postmenopausal invasive breast cancer patients can be evaluated for chemotherapy. At present, the existing prognostic markers for breast cancer are only suitable for ER-positive early breast cancer, and there are still no effective prognostic predictors for patients with ER-negative breast carcinoma showing lymph node metastases greater than three (29,30). In this study, the seven-lncRNA combination model showed good prognostic value for patients with different clinical pathologies, especially for patients with lymph node-positive, pathological stage II–IV, and HER-2 negative breast cancer.
Although the current functional studies of lncRNAs have received increasing attention, the functions of most lncRNAs remain unknown. Functional annotation of lncRNA-specific co-expressing protein-coding genes is considered a viable method for inferring the biological properties of lncRNAs (31). The annotation of lncRNA function by co-expressed genes has been proved to be effective (32). In research, we performed KEGG enrichment analysis of the gene co-expressed with seven lncRNAs to observe the latent functions of these lncRNAs. Based on the functional analysis results, MAPT-AS1, RP1-37C10.3, and RP11-344E13.4 are enriched into several important common signal pathways including the cell cycle, DNA replication, homologous recombination, progesterone-mediated oocyte maturation. Among them, MAPT-AS1 and RP1-37C10.3 are also enriched into three common signaling pathways including cellular senescence, p53 signaling pathway, and Fanconi anemia pathway. Molecular epidemiological analysis has revealed that the p53 mutation exists in almost all kinds of tumors, and that approximately 5% of patients with colorectal cancer, lung cancer, melanoma, sarcoma, head and neck carcinoma, leukemia, esophageal carcinoma, ovarian carcinoma, testicular carcinoma, and cervical carcinoma have been found to harbor p53 mutations (33,34). Several studies have demonstrated that P53 inactivation plays a significant part in the occurrence and progression of breast cancer (35,36). Therefore, the mechanisms of MAPT-AS1 and RP1-37C10.3 involvement in breast cancer are mainly related to changes in the cell cycle and cellular processes like DNA replication. In addition to MAPT-AS1 and RP1-37C10.3, SPACA6P-AS is also enriched in the Fanconi anemia pathway. The Fanconi anemia pathway is a necessary factor in repairing DNA cross-linking damage and maintaining genomic stability (37). Recent evidence suggests that gene instability is a key factor leading to metastasis and recurrence of malignant tumors. Studies have confirmed that the breast cancer susceptibility genes, BRCA2, PALB2, and BRIP1, are the FANCD1, FANCN, and FANCJ genes of the Fanconi anemia pathway, respectively, and that their encoded proteins act downstream of this pathway. Although BRCA1 is not a Fanconi anemia gene, its product can interact with BRCA2, PALB2, and others to participate in the Fanconi anemia pathway (38); RAD51C has also been confirmed to be involved in the Fanconi anemia pathway and its biallelic mutation is associated with the corresponding subtype of Fanconi anemia (39). Studies of these genes have revealed a strong genetic link between Fanconi anemia and the susceptibility of hereditary breast cancer. The RP11 pathway enriched in RP11-454P21.1 includes the alcoholism and PPAR signaling pathways; alcohol intake at more than 10 g per day is significantly related to breast carcinoma in menopausal women (40). Alcohol causes chromosomal instability, leading to cancer-related aneuploidy events. Further, oxidative damage, DNA injury, cross-linking, and DNA strand breakage can lead to the production of reactive oxygen species, lipid peroxidation products, and acetaldehyde (41,42). In contrast, moderate drinking can reduce the risk of breast cancer by about 30% (43,44). Previous studies have demonstrated that PPARc activation can cause autophagy in breast carcinoma cells (45). PPARc is a negative regulator of estrogen synthesis in breast adipose tissue (46). PPAR signaling pathway genes can thus be a significant predictor for breast carcinoma response to neoadjuvant chemotherapy (47).
In line with the consequences of GSVA, the jointly obtained high-risk score group of the training set and the test group includes genes related to maturity onset diabetes of the young, ascorbate and aldarate metabolism, and pentose and glucuronate interconversions. Further, in the low-risk scoring group, the gene functions include AML, colorectal carcinoma, renal cell carcinoma, ERBB signal pathway, purine metabolism, and the insulin signaling pathway; some of these biological pathways were verified to be associated with tumor development and progression (48-50). These results thus demonstrate that the seven predicted prognostic lncRNAs might take part in development and progression of breast carcinoma by interactions with protein-encoding RNAs from associated biological pathways. However, further experimental research is required to confirm the functions of these lncRNAs. Understanding the functions of these seven lncRNAs will thus help clinicians diagnose cancer in the early stage and provide clinical indications for new prognostic factors for breast cancer.
Conclusions
Generally speaking, we systematically explored the lncRNA expression profiles in breast cancer patients and their corresponding clinical information, and found a seven-lncRNA (LINC02037, MAPT-AS1, RP1-37C10.3, RP11-344E13.4, RP11-454P21.1, RP11-616M22.1, SPACA6P-AS) signature. The risk scoring model in this research provides a worthy method to classify patients with diverse survival outcomes. Further, the identified seven-lncRNA signature behaved very well in predicting 3- and 5-year survival in patients with breast carcinoma, which could be an independent predictor of survival prognosis, and could provide new insights into the molecular mechanisms of oncogenesis and breast cancer progression. Through a series of experiments, we further verified that these prognostic lncRNAs can be applied as new biomarkers in breast carcinoma and could act as possible therapeutic targets. Nevertheless, this research does have some boundedness. First, the seven-lncRNA signature was only tested and validated in TCGA. If conditions permit, other databases might be used to validate the clinical values this signature. In addition, our research only examined the biological function of predictive lncRNAs using computational methods, and should be supplemented with in vitro and in vivo experiments. Combining these data will help unravel the mechanism of lncRNA involvement in breast tumorigenesis.
Acknowledgments
Funding: This study was supported by
Footnote
Reporting Checklist: The authors have completed the REMARK reporting checklist. Available at https://dx.doi.org/10.21037/tcr-21-747
Conflicts of Interest: All authors have completed the ICMJE uniform disclosure form (available at https://dx.doi.org/10.21037/tcr-21-747). The authors have no conflicts of interest to declare.
Ethical Statement: The authors are accountable for all aspects of the work in ensuring that questions related to the accuracy or integrity of any part of the work are appropriately investigated and resolved. The study was conducted in accordance with the Declaration of Helsinki (as revised in 2013). Institutional ethical approval and informed consent were waived.
Open Access Statement: This is an Open Access article distributed in accordance with the Creative Commons Attribution-NonCommercial-NoDerivs 4.0 International License (CC BY-NC-ND 4.0), which permits the non-commercial replication and distribution of the article with the strict proviso that no changes or edits are made and the original work is properly cited (including links to both the formal publication through the relevant DOI and the license). See: https://creativecommons.org/licenses/by-nc-nd/4.0/.
References
- DeSantis CE, Ma J, Goding Sauer A, et al. Breast cancer statistics, 2017, racial disparity in mortality by state. CA Cancer J Clin 2017;67:439-48. [Crossref] [PubMed]
- Jemal A, Bray F, Center MM, et al. Global cancer statistics. CA Cancer J Clin 2011;61:69-90. [Crossref] [PubMed]
- Sung H, Ferlay J, Siegel RL, et al. Global Cancer Statistics 2020: GLOBOCAN Estimates of Incidence and Mortality Worldwide for 36 Cancers in 185 Countries. CA Cancer J Clin 2021;71:209-49. [Crossref] [PubMed]
- Donepudi MS, Kondapalli K, Amos SJ, et al. Breast cancer statistics and markers. J Cancer Res Ther 2014;10:506-11. [PubMed]
- Arshi A, Sharifi FS, Khorramian Ghahfarokhi M, et al. Expression Analysis of MALAT1, GAS5, SRA, and NEAT1 lncRNAs in Breast Cancer Tissues from Young Women and Women over 45 Years of Age. Mol Ther Nucleic Acids 2018;12:751-7. [Crossref] [PubMed]
- Li J, Wang W, Xia P, et al. Identification of a five-lncRNA signature for predicting the risk of tumor recurrence in patients with breast cancer. Int J Cancer 2018;143:2150-60. [Crossref] [PubMed]
- Shi X, Sun M, Liu H, et al. Long non-coding RNAs: a new frontier in the study of human diseases. Cancer Lett 2013;339:159-66. [Crossref] [PubMed]
- Spizzo R, Almeida MI, Colombatti A, et al. Long non-coding RNAs and cancer: a new frontier of translational research? Oncogene 2012;31:4577-87. [Crossref] [PubMed]
- Zeng JH, Liang L, He RQ, et al. Comprehensive investigation of a novel differentially expressed lncRNA expression profile signature to assess the survival of patients with colorectal adenocarcinoma. Oncotarget 2017;8:16811-28. [Crossref] [PubMed]
- Zhou M, Xu W, Yue X, et al. Relapse-related long non-coding RNA signature to improve prognosis prediction of lung adenocarcinoma. Oncotarget 2016;7:29720-38. [Crossref] [PubMed]
- Sørensen KP, Thomassen M, Tan Q, et al. Long non-coding RNA expression profiles predict metastasis in lymph node-negative breast cancer independently of traditional prognostic markers. Breast Cancer Res 2015;17:55. [Crossref] [PubMed]
- Meng J, Li P, Zhang Q, et al. A four-long non-coding RNA signature in predicting breast cancer survival. J Exp Clin Cancer Res 2014;33:84. [Crossref] [PubMed]
- Zhou M, Zhong L, Xu W, et al. Discovery of potential prognostic long non-coding RNA biomarkers for predicting the risk of tumor recurrence of breast cancer patients. Sci Rep 2016;6:31038. [Crossref] [PubMed]
- Harrow J, Frankish A, Gonzalez JM, et al. GENCODE: the reference human genome annotation for The ENCODE Project. Genome Res 2012;22:1760-74. [Crossref] [PubMed]
- Robinson MD, McCarthy DJ, Smyth GK. edgeR: a Bioconductor package for differential expression analysis of digital gene expression data. Bioinformatics 2010;26:139-40. [Crossref] [PubMed]
- Zhou M, Zhao H, Wang Z, et al. Identification and validation of potential prognostic lncRNA biomarkers for predicting survival in patients with multiple myeloma. J Exp Clin Cancer Res 2015;34:102. [Crossref] [PubMed]
- Heagerty PJ, Lumley T, Pepe MS. Time-dependent ROC curves for censored survival data and a diagnostic marker. Biometrics 2000;56:337-44. [Crossref] [PubMed]
- Langfelder P, Horvath S. WGCNA: an R package for weighted correlation network analysis. BMC Bioinformatics 2008;9:559. [Crossref] [PubMed]
- Hänzelmann S, Castelo R, Guinney J. GSVA: gene set variation analysis for microarray and RNA-seq data. BMC Bioinformatics 2013;14:7. [Crossref] [PubMed]
- Zhang XQ, Sun S, Lam KF, et al. A long non-coding RNA signature in glioblastoma multiforme predicts survival. Neurobiol Dis 2013;58:123-31. [Crossref] [PubMed]
- Chen X, You ZH, Yan GY, et al. IRWRLDA: improved random walk with restart for lncRNA-disease association prediction. Oncotarget 2016;7:57919-31. [Crossref] [PubMed]
- Fu XL, Liu DJ, Yan TT, et al. Analysis of long non-coding RNA expression profiles in pancreatic ductal adenocarcinoma. Sci Rep 2016;6:33535. [Crossref] [PubMed]
- He Z, Dang J, Song A, et al. Identification of LINC01234 and MIR210HG as novel prognostic signature for colorectal adenocarcinoma. J Cell Physiol 2019;234:6769-77. [Crossref] [PubMed]
- Yang Y, Qian J, Xiang Y, et al. The prognostic value of long noncoding RNA HOTTIP on clinical outcomes in breast cancer. Oncotarget 2017;8:6833-44. [Crossref] [PubMed]
- Milevskiy MJ, Al-Ejeh F, Saunus JM, et al. Long-range regulators of the lncRNA HOTAIR enhance its prognostic potential in breast cancer. Hum Mol Genet 2016;25:3269-83. [Crossref] [PubMed]
- Deng LL, Chi YY, Liu L, et al. LINC00978 predicts poor prognosis in breast cancer patients. Sci Rep 2016;6:37936. [Crossref] [PubMed]
- Győrffy B, Hatzis C, Sanft T, et al. Multigene prognostic tests in breast cancer: past, present, future. Breast Cancer Res 2015;17:11. [Crossref] [PubMed]
- Habel LA, Sakoda LC, Achacoso N, et al. HOXB13:IL17BR and molecular grade index and risk of breast cancer death among patients with lymph node-negative invasive disease. Breast Cancer Res 2013;15:R24. [Crossref] [PubMed]
- King TA, Lyman JP, Gonen M, et al. Prognostic Impact of 21-Gene Recurrence Score in Patients With Stage IV Breast Cancer: TBCRC 013. J Clin Oncol 2016;34:2359-65. [Crossref] [PubMed]
- Kim SY, Kawaguchi T, Yan L, et al. Clinical Relevance of microRNA Expressions in Breast Cancer Validated Using the Cancer Genome Atlas (TCGA). Ann Surg Oncol 2017;24:2943-9. [Crossref] [PubMed]
- Liao Q, Liu C, Yuan X, et al. Large-scale prediction of long non-coding RNA functions in a coding-non-coding gene co-expression network. Nucleic Acids Res 2011;39:3864-78. [Crossref] [PubMed]
- Ma H, Hao Y, Dong X, et al. Molecular mechanisms and function prediction of long noncoding RNA. ScientificWorldJournal 2012;2012:541786. [Crossref] [PubMed]
- Robles AI, Harris CC. Clinical outcomes and correlates of TP53 mutations and cancer. Cold Spring Harb Perspect Biol 2010;2:a001016. [Crossref] [PubMed]
- Scheffner M, Werness BA, Huibregtse JM, et al. The E6 oncoprotein encoded by human papillomavirus types 16 and 18 promotes the degradation of p53. Cell 1990;63:1129-36. [Crossref] [PubMed]
- Clinical practice guidelines for the use of tumor markers in breast and colorectal cancer. Adopted on May 17, 1996 by the American Society of Clinical Oncology. J Clin Oncol 1996;14:2843-77. [Crossref] [PubMed]
- Gasco M, Shami S, Crook T. The p53 pathway in breast cancer. Breast Cancer Res 2002;4:70-6. [Crossref] [PubMed]
- Mirchandani KD, D'Andrea AD. The Fanconi anemia/BRCA pathway: a coordinator of cross-link repair. Exp Cell Res 2006;312:2647-53. [Crossref] [PubMed]
- O'Donovan PJ, Livingston DM. BRCA1 and BRCA2: breast/ovarian cancer susceptibility gene products and participants in DNA double-strand break repair. Carcinogenesis 2010;31:961-7. [Crossref] [PubMed]
- French CA, Tambini CE, Thacker J. Identification of functional domains in the RAD51L2 (RAD51C) protein and its requirement for gene conversion. J Biol Chem 2003;278:45445-50. [Crossref] [PubMed]
- Lew JQ, Freedman ND, Leitzmann MF, et al. Alcohol and risk of breast cancer by histologic type and hormone receptor status in postmenopausal women: the NIH-AARP Diet and Health Study. Am J Epidemiol 2009;170:308-17. [Crossref] [PubMed]
- Bonassi S, El-Zein R, Bolognesi C, et al. Micronuclei frequency in peripheral blood lymphocytes and cancer risk: evidence from human studies. Mutagenesis 2011;26:93-100. [Crossref] [PubMed]
- Fenech M, Bonassi S. The effect of age, gender, diet and lifestyle on DNA damage measured using micronucleus frequency in human peripheral blood lymphocytes. Mutagenesis 2011;26:43-9. [Crossref] [PubMed]
- Colditz GA, Bohlke K. Priorities for the primary prevention of breast cancer. CA Cancer J Clin 2014;64:186-94. [Crossref] [PubMed]
- Howell A, Anderson AS, Clarke RB, et al. Risk determination and prevention of breast cancer. Breast Cancer Res 2014;16:446. [Crossref] [PubMed]
- Zhou J, Zhang W, Liang B, et al. PPARgamma activation induces autophagy in breast cancer cells. Int J Biochem Cell Biol 2009;41:2334-42. [Crossref] [PubMed]
- Rubin GL, Zhao Y, Kalus AM, et al. Peroxisome proliferator-activated receptor gamma ligands inhibit estrogen biosynthesis in human breast adipose tissue: possible implications for breast cancer therapy. Cancer Res 2000;60:1604-8. [PubMed]
- Chen YZ, Xue JY, Chen CM, et al. PPAR signaling pathway may be an important predictor of breast cancer response to neoadjuvant chemotherapy. Cancer Chemother Pharmacol 2012;70:637-44. [Crossref] [PubMed]
- Harryvan TJ, Tushuizen ME. A Young Patient With Diabetes and Liver Tumors. Gastroenterology 2018;155:25-6. [Crossref] [PubMed]
- Verhagen FH, Stigter ECA, Pras-Raves ML, et al. Aqueous Humor Analysis Identifies Higher Branched Chain Amino Acid Metabolism as a Marker for Human Leukocyte Antigen-B27 Acute Anterior Uveitis and Disease Activity. Am J Ophthalmol 2019;198:97-110. [Crossref] [PubMed]
- DiGiovanna MP, Stern DF, Edgerton SM, et al. Relationship of epidermal growth factor receptor expression to ErbB-2 signaling activity and prognosis in breast cancer patients. J Clin Oncol 2005;23:1152-60. [Crossref] [PubMed]