A pan-cancer study of PD-1 and CTLA-4 as therapeutic targets
Introduction
In recent years, basic research in cancer immunology has paved the way for developing and approving immune checkpoint blockers (1). Pan-cancer analysis at the molecular level has provided insights into the common characteristics and heterogeneity of various human malignant tumors (2).
The immune escape mechanism of tumor cells is the target of immunotherapy (3). Cytotoxic T lymphocyte-associated protein (CTLA-4) and programmed cell death (PD-1) inhibit T cell responses. Immune checkpoints activate T cells and participate in tumor cells’ immune response after proliferation and differentiation of the T cells (4,5). Immune checkpoints include positive molecules (e.g., CD28, CD80, and CD86) and harmful molecules such as programmed cell death 1 ligand 1 (PD-L1), and CTLA-4 (6-8). The known immune checkpoints include CTLA-4 and PD-1, expressed in malignant tumors such as non-small cell lung cancer, renal cell carcinoma, and ovarian cancer (9-11). An in-depth study of CTLA-4 and PD-1 resulted in the development of ipilimumab, the first CTLA-4 inhibitor and so far the only agent approved by the US Food and Drug Administration (FDA) (12). Evidence suggests that ipilimumab improves overall survival (OS) in patients with renal cell carcinoma, advanced non-small cell lung cancer, and melanoma (13-16).
We examined similarities and differences in PD-1 and CTLA-4 expression in patients with 33 cancers using The Cancer Genome Atlas (TCGA) pan-cancer data to determine the impact of subtypes of the immune environment on tumor progression and treatment. We systematically summarized and visualized the impact of PD-1 and CTLA-4 on pan-cancer by analyzing the tumor immune microenvironment to predict the ability of patients to respond to immunotherapy. We present the following article in accordance with the MDAR reporting checklist (available at https://dx.doi.org/10.21037/tcr-21-561).
Methods
Online microarray database
We downloaded RNA-Seq data from TCGA for 33 tumours, DNA-methylation (DNAss), stemness scores based on mRNA (RNAss), and immune subtypes. Fifteen types of cancers with none or fewer than 5 related normal samples were identified, and eighteen types of cancers were used to study whether the expression of CTLA-4 and PD-1 in tumor samples differed from that of normal tissues.
The study was conducted in accordance with the Declaration of Helsinki (as revised in 2013).
Analysis of tumor microenvironment
The immune and matrix scores of 33 tumors were evaluated using ESTIMATE prediction software, and the infiltration degree of immune and stromal cells across tumors was predicted. The Spearman correlation test was used to calculate the correlation between the expression of genes and these scores. Six immune subtypes were characterized to degree resistant invasion within the tumor environment. Tumor stem highlights extricated from transcriptome and epigenetics of TCGA tumor tests were utilized to degree stem cell-like characteristics. The Spearman correlation test was used to test the correlation between cancer and the expression of PD-1 and CTLA-4.
NCI-60 analysis
The NCI-60 database was accessed using the CellMiner interface (https://discover.nci.nih.gov/cellminer/), containing data from 60 cancer cell lines from nine types of tumors. The expression of PD-1 and CTLA-4 mRNA and the scores of cell sensitivity data (GI50) were searched and analyzed using a Pearson correlation analysis to determine the pertinence between drug sensitivity and gene expression. The correlation analysis used 262 FDA-approved drug reactions or drugs in clinical trials.
Statistical analysis
A linear mixed-effect model was used to contrast the expression of genes between normal and tumor tissues in eighteen cancers. Box charts were used to illustrate gene expression of various types of cancer. A univariate Cox proportional hazard regression model was used to study the relationship between gene expression and OS. Spearman correlation was used to study the relationship between expression of genes and dryness score, matrix score, immune score, and drug sensitivity. Linear regression examined the relationship between expression of genes and clinical features, immune components, and renal clear cell subtypes. All tests were performed using R, version 4.0.0 (http://www.r-project.org/). For survival studies, we used α=0.05 as the cut-off and differences where P<0.05 are considered significant.
Results
Expression of CTLA-4 and PD-1 across cancers
To determine the differences of the expression of CTLA-4 and PD-1 between normal tissues and cancer, we analyzed 18 tumors with normal sample size ≥5 in TCGA. Expression levels of CTLA-4 and PD-1 in tumor tissues were different from those of normal tissues (Figure 1). The expression of PD-1 in some tumors (e.g., breast invasive carcinoma, BRCA) was significantly higher than in normal tissues (Figure 1A), while in kidney chromophobe (KICH) and thyroid carcinoma (THCA) was lower than normal tissues (P<0.05). The expression of CTLA-4 in KICH was significantly lower than that of normal tissues, while in most other tumors, it was significantly higher than in normal tissues (Figure 1B). According to the Spearman correlation test, there was a positive correlation between the expression of CTLA-4 and PD-1 (P<0.05), suggesting that they might possess common characteristics or functions.
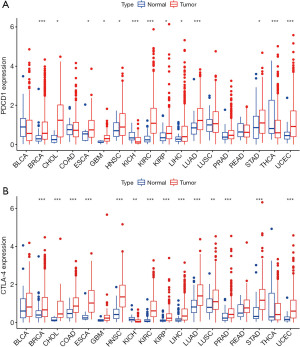
Relationship between PD-1 and CTLA-4 expression and OS
We used a univariate Cox proportional hazard regression model to analyze the relationship between the expression of PD-1 and CTLA-4 and OS in 33 tumors in TCGA and displayed results in a forest map (Figure 2). The expression of CTLA-4 and PD-1 were similar and were protective factors in cervical squamous cell carcinoma and endocervical adenocarcinoma (CSEC), head and neck squamous cell carcinoma (HNSC), skin cutaneous melanoma (SKCM), and uterine corpus endometrial carcinoma (UCEC). However, they were risk factors for kidney renal clear cell carcinoma (KIRC), uveal melanoma (UVM), and brain lower grade glioma (LGG).

PD-1 and CTLA-4 are related to immune responses and the immune microenvironment of cancer
Six types of immune infiltration were identified in human tumors: C1 (tissue healing type), C2 (IFN-γ-dominant type), C3 (inflammatory type), C4 (lymphocyte depletion type), C5 (immune silent type), and C6 (TGF-β-dominant type). We analyzed the immune infiltration in pan-cancerous TCGA data and correlated it with the expression of CTLA-4 and PD-1 (Figure 3A). There was a prominent correlation between higher expression of CTLA-4 and PD-1 and infiltration of types 2 and 6 (C2 and C6). The OS of these patients was poor because of their high proliferation rate and high levels of TGF-β.

We used ESTIMATE prediction software to score tumor immune cell infiltration and correlated it with expression of CTLA-4 and PD-1. We found that tumor immune cell infiltration scores positively correlated with the expression of CTLA-4 and PD-1 in 33 tumor types (Figure 3B).
Association of the expression CTLA-4 and PD-1 with drug sensitivity
Drug sensitivity was determined using Z-score, with higher scores indicating higher sensitivity to drug treatment. First, we found that increased PD-1 and CTLA-4 gene expression was associated with increased drug sensitivity to several drugs across several cell lines (P<0.001; Figure 4). For example, PD-1 was associated with sensitivity to treatment with XK-469 (for solid tumors and multi-drug resistant cancers). The others were bendamustine (for non-Hodgkin lymphoma and chronic lymphocytic leukemia), ifosfamide (for recurrent testicular cancer and germ cell tumors, sarcomas, and bladder cancer, small cell lung cancer, and head and neck cancer), etoposide (for small cell lung cancer and testicular cancer), dimethylaminoparthenolide (for pancreatic cancer), oxaliplatin (for rectal cancer), and alectinib (for non-small-cell lung cancer). CTLA-4 was associated with sensitivity to vemurafenib (for late-stage melanoma), dabrafenib (for late-stage melanoma, and metastatic non-small cell lung cancer with BRAF V600E or V600K mutations).
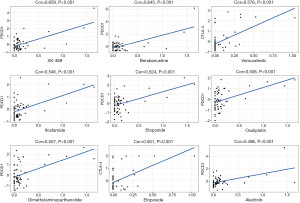
PD-1 and CTLA-4 in renal clear cell carcinoma
We analyzed the immune subtypes of renal clear cell carcinoma in TCGA (Figure 5A) and relationship with expression of CTLA-4 and PD-1 (Figure 5A). There was a high correlation between higher levels of CTLA-4 and PD-1 and type 2 infiltration. Then, we found a positive correlation between the relationship between clinical stage and expression level of PD-1 and CTLA-4 (Figure 5B).
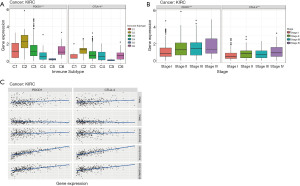
Immune and matrix scores of tumor tissues were calculated using ESTIMATE. Then, we evaluated the association between these scores and the expression of CTLA-4 and PD-1 (Figure 5C). Levels of CTLA-4 and PD-1 significantly correlated with immune, matrix, and estimated scores.
Discussion
Over the last 10 years, immune checkpoint blockade has been studied in clinical trials and showed great potential across cancers. The CTLA-4 and PD-1 pathways were particularly well-studied. PD-1 is an immune receptor which belongs to the CD28/CTLA-4 series (17), a member of the immunoglobulin super family B7, and a 55 kD type I transmembrane glycoprotein (18-20). Several lines of evidence suggest that the ligands of this protein (PD-L1, CD274, and B7-H1) are upregulated in a number of cancers. Overexpression of PDL-1 suppresses the activity of receptor PD-1 and tumor-infiltrating effector CD4/CD8 T cells to protect cancer cells (21). Unlike the direct cytotoxicity of conventional chemotherapy, the basic principle of immune checkpoint blockade is to release the suppressed immunity in the tumour and allow the host’s cytotoxic T cells to target the tumour cells (22). PD-1 and CTLA-4 are encoded by the PDCD1 and CTLA-4 genes, which are expressed on activated T cells and also constitutively on immunosuppressive Treg cells (23). When the ligands of two receptors act on each other, the cytotoxicity of T cells is inhibited. This immune escape mechanism is hijacked by many types of tumors, including renal cell carcinoma, and it is reported that the immune test axis in renal cell carcinoma is upregulated. A number of clinical trials on blocking immune checkpoints have shown encouraging results (24). A sub-group of patients reportedly achieved durable long-term responses, demonstrating the potential of this approach. Questions have been raised regarding differences in PD-1 and CTLA-4 between tumor tissues and normal tissues or paracancerous tissues; if there are differences, the nature of the connection between them should be investigated.
Our study shows a systemic pan-cancer analysis of PD-1 and CTLA-4. There is substantial heterogeneity in the expression of CTLA-4 and PD-1 in pan-cancer; however, the expression was lower in normal tissues and paracancerous tissues. PD-1 primarily showed upregulated expression in tumors such as BRCA and was downregulated in KICH and THCA. By contrast, the expression of CTLA-4 was significantly lower in KICH than in normal tissue and significantly higher in other tumors than in normal tissue.
We also found that the expression of these factors varied across immune infiltration subtypes. We analysed the immune microenvironment of pan-cancer in TCGA and correlated it with the expression levels of CTLA-4 and PD-1. We found a positive correlation between the expression of both CTLA-4 and PD-1, suggesting that they may share a common profile or function. We also found that they were expressed in most of the 33 cancer types and were strongly expressed in immune subtypes with high proliferation rates (C2 and C6). They also highly expressed TGF-β infiltrates, indicating that they promote tumor growth.
Univariate Cox proportional hazard regression model was used to analyze the relationship between expression of PD-1 and CTLA-4 and OS. We found that higher expression of PD-1 and CTLA-4 was associated with worse survival in KIRC, UVM, and LGG and was better in CSEC, HNSC, SKCM, and UCEC.
PD-1 and CTLA-4 expression also correlated with the degree of stromal and immune cell infiltrates. These factors may help predict the outcome of immune checkpoint blockers in patients with specific cancers. The expression of CTLA-4 and PD-1 gene positively correlated with the score of tumor dryness and drug resistance, suggesting that PD-1 and CTLA-4 may stimulate tumorigenesis. Interestingly, we found that the immune infiltrative subtype of renal cell carcinoma was significantly associated with PD-1 and CTLA-4 levels, where higher levels of PD-1 and CTLA-4 correlated with IFN-γ subtypes of immune infiltrates, C2, suggesting a correlation with poor prognosis.
The limitation of this study was that we did not use experiments to validate our findings; however, such experiments require the collaboration of several departments, which is a vast undertaking. We found a strong correlation between levels of CTLA-4 and PD-1 and infiltration of types 2 and 6 (C2 and C6). There was a correlation between the clinical stage of tumors and the expression of CTLA-4 and PD-1. In renal clear cell carcinoma, there was a strong correlation between clinical stage and expression of CTLA-4 and PD-1. Levels of PD-1 and CTLA-4 significantly correlated with immune scores, stromal scores, and estimated scores. Therefore, the significance of this study lies in determining the impact of various subtypes of the immune environment on tumor progression and treatment. Analysis of the tumor immune microenvironment could predict the ability of patients to respond to immunotherapy and reveal new therapeutic targets.
Conclusions
We found significant differences in CTLA-4 and PD-1 gene expression levels between pan-cancer patients and corresponding normal tissues and paracancerous tissues. The expression of both PD-1 and CTLA-4 were significantly and positively correlated. These findings suggest that these proteins may share common characteristics or functions. Further prospective studies and detection methods are needed to confirm this observation and determine whether they can direct personalized immune checkpoint blocking therapy in patients with clear cell renal cell carcinoma.
Acknowledgments
We would like to thank Dr. Robert P Lindeman for his help in polishing our paper.
Funding: This study was supported by
Footnote
Reporting Checklist: The authors have completed the MDAR reporting checklist. Available at https://dx.doi.org/10.21037/tcr-21-561
Conflicts of Interest: All authors have completed the ICMJE uniform disclosure form (available at https://dx.doi.org/10.21037/tcr-21-561). The authors have no conflicts of interest to declare.
Ethical Statement: The authors are accountable for all aspects of the work in ensuring that questions related to the accuracy or integrity of any part of the work are appropriately investigated and resolved. The study was conducted in accordance with the Declaration of Helsinki (as revised in 2013). Institutional ethical approval and informed consent were waived.
Open Access Statement: This is an Open Access article distributed in accordance with the Creative Commons Attribution-NonCommercial-NoDerivs 4.0 International License (CC BY-NC-ND 4.0), which permits the non-commercial replication and distribution of the article with the strict proviso that no changes or edits are made and the original work is properly cited (including links to both the formal publication through the relevant DOI and the license). See: https://creativecommons.org/licenses/by-nc-nd/4.0/.
References
- Charoentong P, Finotello F, Angelova M, et al. Pan-cancer Immunogenomic Analyses Reveal Genotype-Immunophenotype Relationships and Predictors of Response to Checkpoint Blockade. Cell Rep 2017;18:248-62. [Crossref] [PubMed]
- Cancer Genome Atlas Research Network. The Cancer Genome Atlas Pan-Cancer analysis project. Nat Genet 2013;45:1113-20. [Crossref] [PubMed]
- Vaddepally RK, Kharel P, Pandey R, et al. Review of Indications of FDA-Approved Immune Checkpoint Inhibitors per NCCN Guidelines with the Level of Evidence. Cancers (Basel) 2020;12:738. [Crossref] [PubMed]
- Postow MA, Sidlow R, Hellmann MD. Immune-Related Adverse Events Associated with Immune Checkpoint Blockade. N Engl J Med 2018;378:158-68. [Crossref] [PubMed]
- Li B, Chan HL, Chen P. Immune Checkpoint Inhibitors: Basics and Challenges. Curr Med Chem 2019;26:3009-25. [Crossref] [PubMed]
- Zhao Y, Lee CK, Lin CH, et al. PD-L1:CD80 Cis-Heterodimer Triggers the Co-stimulatory Receptor CD28 While Repressing the Inhibitory PD-1 and CTLA-4 Pathways. Immunity 2019;51:1059-1073.e9. [Crossref] [PubMed]
- Sugiura D, Maruhashi T, Okazaki IM, et al. Restriction of PD-1 function by cis-PD-L1/CD80 interactions is required for optimal T cell responses. Science 2019;364:558-66. [Crossref] [PubMed]
- Wei SC, Duffy CR, Allison JP. Fundamental Mechanisms of Immune Checkpoint Blockade Therapy. Cancer Discov 2018;8:1069-86. [Crossref] [PubMed]
- Shi Y. Regulatory mechanisms of PD-L1 expression in cancer cells. Cancer Immunol Immunother 2018;67:1481-9. [Crossref] [PubMed]
- Jiang Y, Chen M, Nie H, et al. PD-1 and PD-L1 in cancer immunotherapy: clinical implications and future considerations. Hum Vaccin Immunother 2019;15:1111-22. [Crossref] [PubMed]
- Rowshanravan B, Halliday N, Sansom DM. CTLA-4: a moving target in immunotherapy. Blood 2018;131:58-67. [Crossref] [PubMed]
- Schadendorf D, Hodi FS, Robert C, et al. Pooled Analysis of Long-Term Survival Data From Phase II and Phase III Trials of Ipilimumab in Unresectable or Metastatic Melanoma. J Clin Oncol 2015;33:1889-94. [Crossref] [PubMed]
- Motzer RJ, Tannir NM, McDermott DF, et al. Nivolumab plus Ipilimumab versus Sunitinib in Advanced Renal-Cell Carcinoma. N Engl J Med 2018;378:1277-90. [Crossref] [PubMed]
- Cella D, Grünwald V, Escudier B, et al. Patient-reported outcomes of patients with advanced renal cell carcinoma treated with nivolumab plus ipilimumab versus sunitinib (CheckMate 214): a randomised, phase 3 trial. Lancet Oncol 2019;20:297-310. [Crossref] [PubMed]
- Motzer RJ, Rini BI, McDermott DF, et al. Nivolumab plus ipilimumab versus sunitinib in first-line treatment for advanced renal cell carcinoma: extended follow-up of efficacy and safety results from a randomised, controlled, phase 3 trial. Lancet Oncol 2019;20:1370-85. [Crossref] [PubMed]
- Hellmann MD, Paz-Ares L, Bernabe Caro R, et al. Nivolumab plus Ipilimumab in Advanced Non-Small-Cell Lung Cancer. N Engl J Med 2019;381:2020-31. [Crossref] [PubMed]
- Okazaki T, Honjo T. PD-1 and PD-1 ligands: from discovery to clinical application. Int Immunol 2007;19:813-24. [Crossref] [PubMed]
- Hua Z, Li D, Xiang G, et al. PD-1 polymorphisms are associated with sporadic breast cancer in Chinese Han population of Northeast China. Breast Cancer Res Treat 2011;129:195-201. [Crossref] [PubMed]
- Ivansson EL, Juko-Pecirep I, Gyllensten UB. Interaction of immunological genes on chromosome 2q33 and IFNG in susceptibility to cervical cancer. Gynecol Oncol 2010;116:544-8. [Crossref] [PubMed]
- Li Z, Li N, Zhu Q, et al. Genetic variations of PD1 and TIM3 are differentially and interactively associated with the development of cirrhosis and HCC in patients with chronic HBV infection. Infect Genet Evol 2013;14:240-6. [Crossref] [PubMed]
- Mishra A, Verma M. Epigenetic and Genetic Regulation of PDCD1 Gene in Cancer Immunology. Methods Mol Biol 2018;1856:247-54. [Crossref] [PubMed]
- Mellman I, Coukos G, Dranoff G. Cancer immunotherapy comes of age. Nature 2011;480:480-9. [Crossref] [PubMed]
- Pentcheva-Hoang T, Corse E, Allison JP. Negative regulators of T-cell activation: potential targets for therapeutic intervention in cancer, autoimmune disease, and persistent infections. Immunol Rev 2009;229:67-87. [Crossref] [PubMed]
- Johnson DB, Rioth MJ, Horn L. Immune checkpoint inhibitors in NSCLC. Curr Treat Options Oncol 2014;15:658-69. [Crossref] [PubMed]