Bibliometrics research on radiomics of lung cancer
Introduction
Lung cancer is the cancer with the highest morbidity and mortality in China and worldwide (1). In China, the incidence and mortality of lung cancer have seen a clear upward trend in recent years. According to the statistics of the National Cancer Center of China, the incidence and mortality of lung cancer in China ranked first among malignant tumors in 2014, with about 781,000 new cases and 626,000 deaths. The incident and mortality rates are higher in males than in females, and higher in cities than in rural areas. There are also regional differences in the rate of morbidity and deaths, from high to low in the eastern, central and western regions (2). Most early-stage lung cancer has no obvious symptoms, and most patients are in the advanced stage when they show symptoms, resulting in a low overall 5-year survival rate for advanced-stage lung cancer (3). Thin-slice CT scanning has become an effective method for screening high-risk groups. In recent years, due to the popularization of health examinations and thin-slice CT examinations, the detection rate of early lung cancer has increased (4). After Lambin et al. first proposed the concept of radiomics in 2012, the technology has been widely used in clinical practice and research such as tumor diagnosis and prognosis (5-7). After nearly 10 years of development, the research of radiomics in the field of lung cancer has developed rapidly (8,9). This study is based on the Science Citation Index Expanded (SCI-E) database to evaluate lung cancer radiomics literature since 2012, and analyze the current status of related research and forecast the future development directions.
Methods
Data source
The literature was obtained from the SCI-E database in the Web of Science Core Collection (WOSCC).
Search strategy
The search was performed based on the SCI-expanded index. The first search formula is topic = Lung cancer OR Lung neoplasms (#1), the second search formula is topic = Radiomics (#2), and the third search formula is #1 and #2, that is, literature that meets both the first and second search results. The search time range is from 1900 to March 18, 2021.
Analysis method
The full record of the search results and the cited references was exported in plain text format, and CiteSpace software was used to analyze the annual publication status, country and institution distribution, journal distribution, author distribution, and frequency of keywords used in publications of radiomics of lung cancer. HistCite software was used to visualize the citation chronology of lung cancer radiomics literature, and Pajek software was used to analyze the main path of the citation chronology. The bibliometric online analysis platform developed by the National Science Library of the Chinese Academy of Sciences was used to visualize analysis of historical keywords, number of articles in the past years, and national partnerships.
Statistical processing
This study mainly analyzes the distribution of lung cancer radiomics literature in different years, countries, institutions, authors. Therefore, statistical processing mainly uses absolute numbers and percentages (n, %) without comparison between groups.
Results
Literature search results
There are a total of 749 publications on lung cancer radiomics, including 529 original articles (70.63%), 109 reviews (14.55%), 93 meeting abstracts (12.4%), 15 editorial materials (2.00%), 14 articles of early access (1.87%), 8 proceeding papers (1.07%), 3 corrections, 2 books, and 1 data paper (Table 1). Although the search scope is from 1900, the first relevant document appeared in 2012 (Table 2, Figure 1). The citation frequency is 21,676 times, the h-index count is 66, and the average number of citations per item is 28.94 (Figure 2). There has been an almost exponential increase in the publication of related papers and the citation of references (Figures 1,2). The HistCite software was used to visualize the citation chronology of lung cancer radiomics literature, and the Pajek software was used to analyze the main path of the citation chronology. The 30 documents with the highest frequency of citations are selected to make the citation chronology, as shown in Figure 3. We exported the citation relationship matrix of the current citation chronicle in HistCite, and imported the matrix file into Pajek for main path analysis. An in-depth analysis found that these 14 documents (Figure 4) represent the development stage of lung cancer radiomics and related research, from the initial image analysis to the clinical significance to the recent standardization, which basically reflects the development process of related fields (Table 3).
Table 1
Literatures | Records | % of 749 |
---|---|---|
Articles | 529 | 70.63 |
Reviews | 109 | 14.55 |
Meeting abstracts | 93 | 12.42 |
Editorial materials | 15 | 2.00 |
Early access | 14 | 1.87 |
Proceeding papers | 8 | 1.07 |
Corrections | 3 | 0.40 |
Book chapters | 2 | 0.27 |
Data paper | 1 | 0.13 |
Table 2
Years | Records | % of 749 |
---|---|---|
2021 | 27 | 3.60 |
2020 | 241 | 32.18 |
2019 | 173 | 23.10 |
2018 | 143 | 19.09 |
2017 | 89 | 11.88 |
2016 | 42 | 5.61 |
2015 | 21 | 2.80 |
2014 | 6 | 0.80 |
2013 | 5 | 0.67 |
2012 | 2 | 0.27 |
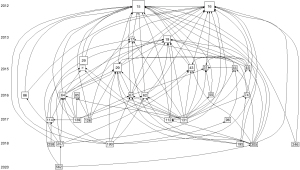
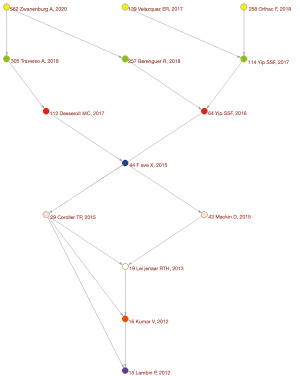
Table 3
No. | Articles | Citation | Reference |
---|---|---|---|
1 | Lambin P, Rios-Velazquez E, Leijenaar R, et al. Radiomics: extracting more information from medical images using advanced feature analysis | 280 | (5) |
2 | Kumar V, Gu Y, Basu S, et al. Radiomics: the process and the challenges | 178 | (10) |
3 | Leijenaar RT, Carvalho S, Velazquez ER, et al. Stability of FDG-PET radiomics features: an integrated analysis of test-retest and inter-observer variability | 94 | (11) |
4 | Coroller TP, Grossmann P, Hou Y, et al. CT-based radiomic signature predicts distant metastasis in lung adenocarcinoma | 122 | (12) |
5 | Mackin D, Fave X, Zhang L, et al. Measuring computed tomography scanner variability of radiomics features | 80 | (13) |
6 | Fave X, Mackin D, Yang J, et al. Can radiomics features be reproducibly measured from CBCT images for patients with non-small cell lung cancer? | 42 | (14) |
7 | Yip SS, Aerts HJ. Applications and limitations of radiomics | 62 | (15) |
8 | Desseroit MC, Tixier F, Weber WA, et al. Reliability of PET/CT shape and heterogeneity features in functional and morphologic components of non-small cell lung cancer tumors: a repeatability analysis in a prospective multicenter cohort | 37 | (16) |
9 | Yip SS, Kim J, Coroller TP, et al. Associations between somatic mutations and metabolic imaging phenotypes in non-small cell lung cancer | 38 | (17) |
10 | Rios Velazquez E, Parmar C, Liu Y, et al. Somatic mutations drive distinct imaging phenotypes in lung cancer | 59 | (18) |
11 | Berenguer R, Pastor-Juan MDR, Canales-Vazquez J, et al. Radiomics of CT features may be nonreproducible and redundant: influence of CT acquisition parameters | 34 | (19) |
12 | Orlhac F, Boughdad S, Philippe C, et al. A postreconstruction harmonization method for multicenter radiomic studies in PET | 27 | (20) |
13 | Traverso A, Wee L, Dekker A, et al. Repeatability and reproducibility of radiomic features: a systematic review | 27 | (21) |
14 | Zwanenburg A, Vallieres M, Abdalah MA, et al. The image biomarker standardization initiative: standardized quantitative radiomics for high-throughput image-based phenotyping | 31 | (22) |
Distribution of countries and institutions
The country visualization map (Figures 5,6) and the list (Table 4) show the top 5 countries with the number of publications: the United States, China, the Netherlands, Italy, and France. The top five centrally ranked countries are the United States, China, France, Canada, and Germany. In the visualization view, the number of network nodes (N=70), and the number of connections (E=187) were both small, indicating that although a large number of countries have participated in the research of lung cancer radiomics, it is still dominated by a few countries (Table 5). The visualization of papers published in different countries over the years (Figure 7) shows that the number of papers published by major countries and the overall number are on the rise. Institutional visualization map (Figure 8) and list (Tables 6,7) show similar results. Related studies are mainly concentrated in medical centers such as Moffitt Cancer Center, Maastricht University, and Harvard Medical School, resulting in cooperation mainly between authors from these institutions (Figure 9).
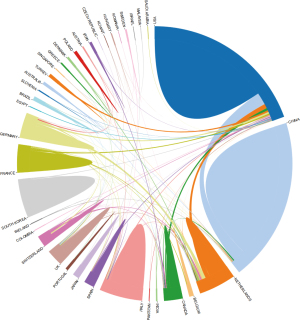
Table 4
Rank | Countries | Frequency |
---|---|---|
1 | USA | 296 |
2 | China | 236 |
3 | Netherlands | 66 |
4 | Italy | 63 |
5 | France | 59 |
6 | Canada | 42 |
7 | Germany | 40 |
8 | South Korea | 40 |
9 | England | 31 |
10 | Switzerland | 26 |
Table 5
Rank | Countries | Centrality |
---|---|---|
1 | USA | 0.73 |
2 | China | 0.28 |
3 | France | 0.26 |
4 | Canada | 0.13 |
5 | Germany | 0.12 |
6 | Netherlands | 0.10 |
7 | Italy | 0.09 |
8 | England | 0.06 |
9 | Singapore | 0.05 |
10 | Switzerland | 0.03 |
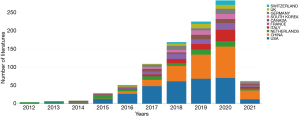
Table 6
Rank | Institutions | Frequency |
---|---|---|
1 | H. Lee Moffitt Canc Ctr & Res Inst | 43 |
2 | Maastricht Univ | 40 |
3 | Harvard Med Sch | 29 |
4 | Univ Texas MD Anderson Canc Ctr | 28 |
5 | Stanford Univ | 26 |
6 | Univ S. Florida | 25 |
7 | Shanghai Jiao Tong Univ | 21 |
8 | Sungkyunkwan Univ | 19 |
9 | Shandong Univ | 19 |
10 | Fudan Univ | 17 |
Table 7
Rank | Institutions | Centrality |
---|---|---|
1 | Stanford Univ | 0.36 |
2 | H. Lee Moffitt Canc Ctr & Res Inst | 0.21 |
3 | Maastricht Univ | 0.12 |
4 | Harvard Med Sch | 0.12 |
5 | GE Healthcare | 0.11 |
6 | Univ Texas MD Anderson Canc Ctr | 0.10 |
7 | Univ Groningen | 0.10 |
8 | Univ Toronto | 0.09 |
9 | Shanghai Jiao Tong Univ | 0.08 |
10 | Chinese Acad Sci | 0.08 |
Authors distribution
The top-ranked authors in terms of distribution have published a lot of work, and their papers are often published in the more authoritative journals. Further analysis reveals that these authors come from major institutions. Philippe Lambin and Ralph T. H. Leijenaar are from Maastricht Univ, while Hugo J. W. L. Aerts, Raymond H. Mak, and Chintan Parmar are all from Harvard Medical School. Robert J. Gillies, Matthew B. Schabath, and Yoganand Balagurunathan are all from Moffitt Cancer Center, and these authors often cooperate in important articles. Therefore, we can see in the centrality index that these authors have high centrality rankings (Tables 8,9). Similarly, due to the quality of their work, these authors are also cited more than other authors (Tables 10,11, Figure 10).
Table 8
Rank | Authors | Records |
---|---|---|
1 | Lambin P | 32 |
2 | Aerts HJWL | 31 |
3 | Gillies RJ | 29 |
4 | Schabath MB | 22 |
5 | Leijenaar RTH | 19 |
6 | Balagurunathan Y | 17 |
7 | Mak RH | 16 |
8 | Lee HY | 15 |
9 | Parmar C | 14 |
10 | Tian J | 14 |
Table 9
Rank | Authors | Centrality |
---|---|---|
1 | Gillies RJ | 0.12 |
2 | Mackin D | 0.08 |
3 | Zhang GG | 0.08 |
4 | Tian J | 0.05 |
5 | Leijenaar RTH | 0.05 |
6 | Latifi K | 0.04 |
7 | Schabath MB | 0.04 |
8 | Lambin P | 0.04 |
9 | Napel S | 0.04 |
10 | Moros EG | 0.03 |
Table 10
Rank | Authors | Frequency |
---|---|---|
1 | Lambin P | 354 |
2 | Aerts HJWL | 347 |
3 | Gillies RJ | 277 |
4 | Parmar C | 207 |
5 | Kumar V | 179 |
6 | Coroller TP | 160 |
7 | Leijenaar RTH | 153 |
8 | Ganeshan B | 142 |
9 | Haralick RM | 139 |
10 | Hatt M | 128 |
Table 11
Rank | Authors | Centrality |
---|---|---|
1 | Basu S | 0.64 |
2 | Amadasun M | 0.30 |
3 | Aerts HJWL | 0.22 |
4 | Gevaert O | 0.12 |
5 | Armato SG | 0.12 |
6 | Boellaard R | 0.10 |
7 | Alic L | 0.10 |
8 | Coroller TP | 0.09 |
9 | Cook GJR | 0.08 |
10 | Balagurunathan Y | 0.08 |
Journals
This study includes 749 radiomics articles that were published in 184 journals, of which 18 journals have more than 10 articles (Table 12). These journals have published 402 publications, accounting for 53.67% of the total literature (Table 8). The citation frequency and centrality ranking of these journals show that the top 10 journals are mainly imaging and oncology journals (Tables 13,14). The results also show that journals such as Medical Physics, Frontiers in Oncology, and European Radiology have great influence on lung cancer radiomics.
Table 12
Rank | Journals | Records | % of 749 |
---|---|---|---|
1 | Medical Physics | 50 | 6.68 |
2 | Frontiers in Oncology | 39 | 5.21 |
3 | European Radiology | 31 | 4.14 |
4 | Scientific Reports | 30 | 4.01 |
5 | European Journal of Nuclear Medicine and Molecular Imaging | 28 | 3.74 |
6 | International Journal of Radiation Oncology, Biology, Physics | 28 | 3.74 |
7 | Physics in Medicine and Biology | 28 | 3.74 |
8 | Journal of Thoracic Oncology | 25 | 3.34 |
9 | Radiotherapy and Oncology | 21 | 2.80 |
10 | PLoS One | 20 | 2.67 |
11 | Journal of Nuclear Medicine | 18 | 2.40 |
12 | Translational Lung Cancer Research | 15 | 2.00 |
13 | European Journal of Radiology | 14 | 1.87 |
14 | Cancers | 12 | 1.60 |
15 | Lung Cancer | 12 | 1.60 |
16 | Radiology | 11 | 1.47 |
17 | Journal of Thoracic Disease | 10 | 1.34 |
18 | Physica Medica: European Journal of Medical Physics | 10 | 1.34 |
Table 13
Rank | Journals | Frequency |
---|---|---|
1 | Radiology | 537 |
2 | Sci Rep-UK | 407 |
3 | PLoS One | 390 |
4 | Nat Commun | 353 |
5 | Med Phys | 343 |
6 | Eur Radiol | 340 |
7 | Radiother Oncol | 335 |
8 | Eur J Cancer | 331 |
9 | J Nucl Med | 282 |
10 | Int J Radiat Oncol | 277 |
Table 14
Rank | Journals | Centrality |
---|---|---|
1 | Algorithms | 0.22 |
2 | Algorithms Cluster D | 0.22 |
3 | Aaai Spring S Series | 0.22 |
4 | Acad Radiol | 0.19 |
5 | Am J Roentgenol | 0.14 |
6 | Clin Cancer Res | 0.12 |
7 | Lung Cancer | 0.11 |
8 | Acta Oncol | 0.11 |
9 | CA Cancer J Clin | 0.11 |
10 | Brit J Cancer | 0.10 |
Keywords
CiteSpace V was used to construct a keyword co-occurrence map (Figure 11: N=355, E=1,877, Tables 15,16), which shows that radiomics, lung cancer, survival, characteristics, and CT are keywords in the literature. Further, using CiteSpace for burst detection for high-frequency keywords (Figure 12) shows that the focus of research is gradually shifting from imaging feature analysis to deep learning and artificial intelligence. Therefore, the current and future research hotspots are not about extracting image features from the image itself in the past, but turning to how to use artificial intelligence to more accurately judge lesions. In other words, it is necessary to further integrate radiomics with clinical practice and prognosis analysis, and use the accumulated big data to make more accurate diagnostic and prognostic judgments, and even provide reasonable treatment plans. The keyword visualization view over time shows that the frequency of use of the main keywords is increasing year by year, which is consistent with the increase in the number of related publications (Figure 13).
Table 15
Rank | Keywords | Frequency |
---|---|---|
1 | Radiomics | 445 |
2 | Lung cancer | 178 |
3 | Feature | 152 |
4 | Cell lung cancer | 139 |
5 | Image | 136 |
6 | Texture analysis | 116 |
7 | Computed tomography | 110 |
8 | Survival | 107 |
9 | Cancer | 104 |
10 | CT | 101 |
Table 16
Rank | Keywords | Centrality |
---|---|---|
1 | Chemotherapy | 0.18 |
2 | Radiomics | 0.17 |
3 | Carcinoma | 0.13 |
4 | Cancer | 0.12 |
5 | CT | 0.12 |
6 | NSCLC | 0.12 |
7 | Immunotherapy | 0.10 |
8 | Texture analysis | 0.09 |
9 | Heterogeneity | 0.09 |
10 | Diagnosis | 0.09 |
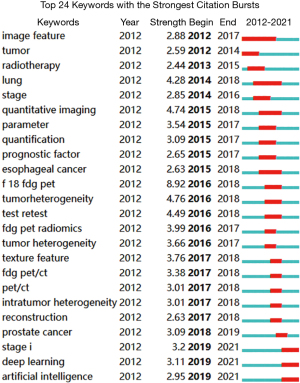
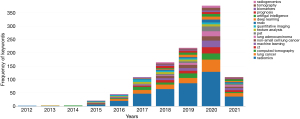
Discussion
This study analyzed the research literature related to lung cancer since the first introduction of radiomics in 2012, and found that radiomics research in the field of lung cancer has developed rapidly. The number of related publications and the citation frequency have increased almost exponentially, especially in recent years. The recent traction of computer deep learning and artificial intelligence has seen the focus of research has gradually shift from the initial image feature data extraction, classification, and mining to the current deep learning based on big data. On this basis, artificial intelligence applied to practical work has greatly improved the accuracy and efficiency of diagnosis. In terms of the distribution of research, the data shows that lung cancer radiomics research is mainly concentrated in the United States, China, the Netherlands and other countries. Some of these countries (such as the United States and the Netherlands) have done a lot of pioneering work based on theoretical and technological leadership. Some countries (such as China) generate abundant clinical data based on a large number of cases, combined with advanced computer technology, and contribute important practical application results. Therefore, the current related research is relatively concentrated in the aforementioned countries and research institutions, but the cooperative research in this field is relatively extensive, and many authors have participated in international cooperation.
Radiomics refers to the use of computer technology to screen and analyze image features extracted from regions of interest such as CT, magnetic resonance imaging, and PET through quantitative methods, to quantitatively describe the biological characteristics and heterogeneity of tumors. This high-throughput quantitative analysis method avoids the bias caused by the subjectivity of imaging physicians (23,24). Radiomics usually includes five processes including standardized image acquisition and reconstruction, lesion image segmentation, feature extraction and selection, prediction model construction and verification, and model classification and prediction (25). Currently, in clinical work, doctors’ image analysis is mainly limited to the preliminary analysis of tumor morphology and geometric characteristics, including roughly measuring the size of the lesion, and a simple description of CT values, PET-CT, and MRI signal levels. Radiomics is based on conventional imaging diagnosis, in-depth mining of image data, and looking for systemic imaging diagnosis technology that reflects the objective characteristics of the disease. In recent years, it has also been combined with the pathophysiological changes at the tissue, cell, and gene level to develop radiomics that can better reflect the biological characteristics of tumors (26-28).
Due to the popularity of thin-slice CT scans, a large number of lung nodules have been discovered. Distinguishing between benign and malignant nodules is a huge challenge for radiologists and clinicians. Since applying radiomics in the diagnosis of lung cancer, significant progress has been made in the identification of lung masses (29,30). Based on the previous research, Alahmari et al. found that combining delta radiomics features with conventional radiomics features can further improve the ability to identify lung tumors, that is to say that the dynamic changes of radiomic characteristics can better distinguish between benign and malignant lung tumors, but the authors did not conduct follow-up studies on the prognostic images yielded by this method (31). Dynamic changes mean that a certain period of observation is required, and time is an important cost for cancer patients. Sun et al. performed radiomics analysis on lung adenocarcinoma with simple ground-glass nodules and found that radiomic-based nomograms combined with RAD scores, edge morphology, acupuncture signs, and tumor size can be used to evaluate whether simple ground-glass nodules are biomarkers of aggressiveness. Similar studies are abundant (32,33). Differentiating between benign and malignant tumors determines the course of treatment, and the specific treatment plan requires more individualized information, especially the pathological type and genotype of the tumor tissue. The results of radiomics combined with histopathology and gene phenotype showed that the appearance and imaging features of tumors are closely related to pathological changes, and changes in gene, protein, and molecular expression, that is, imaging features are closely related to lung tumor histology (34-36). In addition to differential diagnosis and pathological gene prediction, radiomics can also predict the prognosis of lung cancer patients. Hosny et al. conducted a retrospective radiomics study on patients with non-small cell lung cancer (NSCLC), and the results showed that based on standard CT images from NSCLC patients, deep learning networks can be used to stratify the risk of death in these patients (37). It is evident that radiomics as a diagnostic evaluation technique has been applied to all aspects of lung cancer. The quantitative analysis of relevant literature shows that radiomics has good prospects in the field of lung cancer. But at present, it is relatively limited to a few countries and research institutions. The potential regional differences in these results and differences in environmental factors need to be further analyzed based on big data, combined with artificial intelligence and deep learning. However, such a huge amount of work is difficult to complete by humans, thus radiomics will have greater value in the future.
Limitations
Due to the large number of documents retrieved, we only conducted in-depth analysis of the top-ranked institutions, authors, and journals, and were unable to analyze specific documents one by one, and did not sufficiently explore the associations between research details, especially in the lack of further analysis of important documents. Future research should focus on the related work of some important investigators and research institutions based on the results of this research.
Acknowledgments
Funding: None.
Footnote
Conflicts of Interest: All authors have completed the ICMJE uniform disclosure form (available at https://dx.doi.org/10.21037/tcr-21-1277). The authors have no conflicts of interest to declare.
Ethical Statement: The authors are accountable for all aspects of the work in ensuring that questions related to the accuracy or integrity of any part of the work are appropriately investigated and resolved.
Open Access Statement: This is an Open Access article distributed in accordance with the Creative Commons Attribution-NonCommercial-NoDerivs 4.0 International License (CC BY-NC-ND 4.0), which permits the non-commercial replication and distribution of the article with the strict proviso that no changes or edits are made and the original work is properly cited (including links to both the formal publication through the relevant DOI and the license). See: https://creativecommons.org/licenses/by-nc-nd/4.0/.
References
- Bray F, Ferlay J, Soerjomataram I, et al. Global cancer statistics 2018: GLOBOCAN estimates of incidence and mortality worldwide for 36 cancers in 185 countries. CA Cancer J Clin 2018;68:394-424. [Crossref] [PubMed]
- Chen WQ, Li H, Sun KX, et al. Report of cancer incidence and mortality in China, 2014. Zhonghua Zhong Liu Za Zhi 2018;40:5-13. [PubMed]
- Richards TB, Henley SJ, Puckett MC, et al. Lung cancer survival in the United States by race and stage (2001-2009): findings from the CONCORD-2 study. Cancer 2017;123:5079-99. [Crossref] [PubMed]
- Vaidya P, Bera K, Gupta A, et al. CT derived radiomic score for predicting the added benefit of adjuvant chemotherapy following surgery in stage I, II resectable non-small cell lung cancer: a retrospective multicohort study for outcome prediction. Lancet Digit Health 2020;2:e116-28. [Crossref] [PubMed]
- Lambin P, Rios-Velazquez E, Leijenaar R, et al. Radiomics: extracting more information from medical images using advanced feature analysis. Eur J Cancer 2012;48:441-6. [Crossref] [PubMed]
- Hatt M, Tixier F, Visvikis D, et al. Radiomics in PET/CT: more than meets the eye? J Nucl Med 2017;58:365-6. [Crossref] [PubMed]
- Chen B, Yang L, Zhang R, et al. Radiomics: an overview in lung cancer management-a narrative review. Ann Transl Med 2020;8:1191. [Crossref] [PubMed]
- Kim G, Kim J, Cha H, et al. Metabolic radiogenomics in lung cancer: associations between FDG PET image features and oncogenic signaling pathway alterations. Sci Rep 2020;10:13231. [Crossref] [PubMed]
- Wong CW, Chaudhry A. Radiogenomics of lung cancer. J Thorac Dis 2020;12:5104-9. [Crossref] [PubMed]
- Kumar V, Gu Y, Basu S, et al. Radiomics: the process and the challenges. Magn Reson Imaging 2012;30:1234-48. [Crossref] [PubMed]
- Leijenaar RT, Carvalho S, Velazquez ER, et al. Stability of FDG-PET radiomics features: an integrated analysis of test-retest and inter-observer variability. Acta Oncol 2013;52:1391-7. [Crossref] [PubMed]
- Coroller TP, Grossmann P, Hou Y, et al. CT-based radiomic signature predicts distant metastasis in lung adenocarcinoma. Radiother Oncol 2015;114:345-50. [Crossref] [PubMed]
- Mackin D, Fave X, Zhang L, et al. Measuring computed tomography scanner variability of radiomics features. Invest Radiol 2015;50:757-65. [Crossref] [PubMed]
- Fave X, Mackin D, Yang J, et al. Can radiomics features be reproducibly measured from CBCT images for patients with non-small cell lung cancer? Med Phys 2015;42:6784-97. [Crossref] [PubMed]
- Yip SS, Aerts HJ. Applications and limitations of radiomics. Phys Med Biol 2016;61:R150-66. [Crossref] [PubMed]
- Desseroit MC, Tixier F, Weber WA, et al. Reliability of PET/CT shape and heterogeneity features in functional and morphologic components of non-small cell lung cancer tumors: a repeatability analysis in a prospective multicenter cohort. J Nucl Med 2017;58:406-11. [Crossref] [PubMed]
- Yip SS, Kim J, Coroller TP, et al. Associations between somatic mutations and metabolic imaging phenotypes in non-small cell lung cancer. J Nucl Med 2017;58:569-76. [Crossref] [PubMed]
- Rios Velazquez E, Parmar C, Liu Y, et al. Somatic mutations drive distinct imaging phenotypes in lung cancer. Cancer Res 2017;77:3922-30. [Crossref] [PubMed]
- Berenguer R, Pastor-Juan MDR, Canales-Vazquez J, et al. Radiomics of CT features may be nonreproducible and redundant: influence of CT acquisition parameters. Radiology 2018;288:407-15. [Crossref] [PubMed]
- Orlhac F, Boughdad S, Philippe C, et al. A Postreconstruction harmonization method for multicenter radiomic studies in PET. J Nucl Med 2018;59:1321-8. [Crossref] [PubMed]
- Traverso A, Wee L, Dekker A, et al. Repeatability and reproducibility of radiomic features: a systematic review. Int J Radiat Oncol Biol Phys 2018;102:1143-58. [Crossref] [PubMed]
- Zwanenburg A, Vallieres M, Abdalah MA, et al. The image biomarker standardization initiative: standardized quantitative radiomics for high-throughput image-based phenotyping. Radiology 2020;295:328-38. [Crossref] [PubMed]
- Gampala S, Vankeshwaram V, Gadula SSP. Is artificial intelligence the new friend for radiologists? A review article. Cureus 2020;12:e11137. [Crossref] [PubMed]
- Pesapane F, Codari M, Sardanelli F. Artificial intelligence in medical imaging: threat or opportunity? Radiologists again at the forefront of innovation in medicine. Eur Radiol Exp 2018;2:35. [Crossref] [PubMed]
- Levy MA, Freymann JB, Kirby JS, et al. Informatics methods to enable sharing of quantitative imaging research data. Magn Reson Imaging 2012;30:1249-56. [Crossref] [PubMed]
- Castiglioni I, Gallivanone F, Losio C. Frontiers from radiomics in molecular imaging. Contrast Media Mol Imaging 2019;2019:7919545. [Crossref] [PubMed]
- Gillies RJ, Kinahan PE, Hricak H. Radiomics: images are more than pictures, they are data. Radiology 2016;278:563-77. [Crossref] [PubMed]
- van Griethuysen JJM, Fedorov A, Parmar C, et al. Computational radiomics system to decode the radiographic phenotype. Cancer Res 2017;77:e104-7. [Crossref] [PubMed]
- Khawaja A, Bartholmai BJ, Rajagopalan S, et al. Do we need to see to believe?-radiomics for lung nodule classification and lung cancer risk stratification. J Thorac Dis 2020;12:3303-16. [Crossref] [PubMed]
- Ather S, Kadir T, Gleeson F. Artificial intelligence and radiomics in pulmonary nodule management: current status and future applications. Clin Radiol 2020;75:13-9. [Crossref] [PubMed]
- Alahmari SS, Cherezov D, Goldgof D, et al. Delta radiomics improves pulmonary nodule malignancy prediction in lung cancer screening. IEEE Access 2018;6:77796-806.
- Fan L, Fang M, Li Z, et al. Radiomics signature: a biomarker for the preoperative discrimination of lung invasive adenocarcinoma manifesting as a ground-glass nodule. Eur Radiol 2019;29:889-97. [Crossref] [PubMed]
- Sun Y, Li C, Jin L, et al. Radiomics for lung adenocarcinoma manifesting as pure ground-glass nodules: invasive prediction. Eur Radiol 2020;30:3650-9. [Crossref] [PubMed]
- Yoon J, Suh YJ, Han K, et al. Utility of CT radiomics for prediction of PD-L1 expression in advanced lung adenocarcinomas. Thorac Cancer 2020;11:993-1004. [Crossref] [PubMed]
- Aerts HJ, Velazquez ER, Leijenaar RT, et al. Decoding tumour phenotype by noninvasive imaging using a quantitative radiomics approach. Nat Commun 2014;5:4006. Erratum in: Nat Commun 2014;5:4644. [Crossref] [PubMed]
- Gu Q, Feng Z, Liang Q, et al. Machine learning-based radiomics strategy for prediction of cell proliferation in non-small cell lung cancer. Eur J Radiol 2019;118:32-7. [Crossref] [PubMed]
- Hosny A, Parmar C, Coroller TP, et al. Deep learning for lung cancer prognostication: a retrospective multi-cohort radiomics study. PLoS Med 2018;15:e1002711. [Crossref] [PubMed]
(English Language Editor: G. Stone)