The roles of risk model based on the 3-XRCC genes in lung adenocarcinoma progression
Introduction
The deoxyribonucleic acid (DNA) repair system plays a vital role in protecting the human genome from carcinogens. The abnormal expression of DNA repair genes might be the cause of tumor development and resistance of malignant cells to chemotherapeutic drugs (1-6). For example, hydroxycamptothecin (HCPT) could increase the expression of the DNA repair gene, XPF, in bladder cancer and promote apoptosis in T24 and 5637 cells. The increased expression of XPF could reduce the sensitivity of bladder cancer cells, while interfering with the expression of XPF could reduce the resistance of bladder cancer cells to chemotherapy (5). Likewise, interfering with the expression of BRCA1 interacting protein C-terminal helicase 1 (BRIP1), which regulates DNA repair and cell proliferation could induce cell cycle arrest and reduce the proliferation of breast cancer (BC) cells, and promote the invasion of BC cells (6). These examples highlight the important role of the DNA repair system in cancer progression.
The X-ray repair of cross-complementary (XRCC) genes are common components of the DNA repair system and are related to cancer progression. For example, XRCC1 is essential for DNA base excision repair, single strand break repair, and nucleotide excision repair. In ovarian cancer, XRCC1 is positive in 48% of tumor patients, which is related to advanced stage, platinum resistance, disease progression, and so on. The expression level of XRCC1 is an independent risk factor for cancer specificity and progression-free survival. Compared with XRCC1-positive cells, XRCC1-negative cells are sensitive to cisplatin, which is related to DNA double-strand breaks and cell cycle arrest of G2/M (7). XRCC2 overexpression has been found in rectal cancer tissues without preoperative radiotherapy (PRT). Compared with XRCC2-positive patients treated with PRT, XRCC2-negative patients with locally advanced rectal cancer (LARC) have improved overall survival (OS). The level of XRCC2 expression is related to the increase of radiation resistance of LARC, while cancer cells without XRCC2 expression are more sensitive to radiation in vitro, which is related to the arrest and apoptosis of cells in the G2/M phase. When the expression of XRCC2 is interfered with, the repair ability of DNA double strand breaks caused is impaired via radiation (8).
The Cancer Genome Atlas (TCGA) database aims to apply high-throughput genome analysis technology to improve people’s ability to prevent, diagnose, and treat cancer. It has multiple cancer types and groups of data, including gene expression data, microRNA (miRNA) expression data, copy number variation, DNA methylation, and so on (9,10). However, the role of XRCC genes in the progression of lung adenocarcinoma (LUAD) has not been fully elucidated. In recent years, risk models have also been commonly used to assess the prognosis of cancer patients (11,12). In this study, the expression levels, diagnostic value, and prognostic value of XRCC genes in LUAD were evaluated using the Oncomine and TCGA databases, and a risk model was constructed to evaluate the clinical predictive value for the progression of LUAD patients. The following article was presented in accordance with the TRIPOD reporting checklist (available at https://dx.doi.org/10.21037/tcr-21-1431).
Methods
The study was conducted in accordance with the Declaration of Helsinki (as revised in 2013).
Oncomine database
The Oncomine 3.0 (https://www.oncomine) database is used for the study of tumor-related genes, with a wide range of data sources and high reliability (13). The expression of XRCC genes in pan-cancer tissues was analyzed in the Oncomine database. The XRCC genes included the following: XRCC1, XRCC2, XRCC3, XRCC4, XRCC5, XRCC6, FANCG, and PRKDC. The screening criteria were as follows: (I) genes: XRCC1/2/3/4/5/6, FANCG, and PRKDC; (II) analysis type: cancer versus normal analysis; (III) data type: messenger RNA (mRNA); (IV) P<0.05; and (V) fold change: ALL.
Visualization analysis of TCGA data
The gene expression data of HTSeq-FPKM tissue, including 59 cases of lung tissues and 535 cases of LUAD tissues, and the clinical data of 522 cancer patients were downloaded from the official TCGA (https://portal.gdc.cancer.gov/projects/TCGA-LUAD (HTSeq-FPKM) website. Among them, 57 lung tissues and 57 LUAD tissues were derived from the same LUAD patients. The expressions of XRCC1/2/3/4/5/6, FANCG, and PRKDC were identified in lung and LUAD tissues, and the correlation between XRCC genes was analyzed. Principal component analysis (PCA), gene set enrichment analysis (GSEA), and clinical correlation analysis were performed in the 535 cases of LUAD issues.
Consensus clustering and survival analysis
According to the expression levels of XRCC genes, the 535 cases of LUAD tissues in TCGA database were divided into two groups using the “Consensus-ClusterPlus” in R, and PCA was performed (14,15). Kaplan-Meier survival analysis and correlation analysis were performed to evaluate the OS and clinicopathological characteristics (age, sex, clinical stage, T stage, N stage, M stage, and survival status) in both groups.
Construction of the risk model in LUAD
Univariate Cox regression analysis was used to filter the prognostic factors in patients with LUAD. The independent risk factors for poor prognosis of LUAD patients were screened by multivariate Cox regression analysis and the Akaike information criterion (AIC) (16). LUAD patients were divided into high- and low-risk groups according to the gene expression levels. Kaplan-Meier survival analysis evaluated the risk of death in two groups of LUAD patients. The relationship between risk and clinicopathological features (including age, sex, clinical stage, T stage, N stage, M stage) was assessed in patients with LUAD via correlation analysis.
The value of risk model in the prognosis of LUAD
Univariate and multivariate Cox regression analyses were used to assess the effects of the risk model, age, sex, clinical stage, T stage, N stage, and M stage on the prognosis of LUAD patients, and to evaluate the role of the risk model in the prognosis of LUAD patients (17).
Biological processes and signaling mechanisms
The XRCC genes were entered into the String (https://string-db.org) database to conduct Gene Ontology (GO), Kyoto Encyclopedia of Genes and Genomes (KEGG), and protein-protein interaction (PPI) analyses. GSEA was used to explore the biological functions and regulatory mechanisms that the influencing factors might be involved in (18-20). The LUAD tissue gene expression data from TCGA database were divided into high- and low-risk groups according to the median value of the risk model score to explore the effects of two groups on each gene. GO [biological process (BP)] and KEGG analyses were carried out using the GSEA software. The screening criteria was as follows: nominal (NOM) P<0.05.
Correlation analysis of LUAD immune cell markers
The relationship between risk model factors and LUAD immune infiltrating cell markers were analyzed in 535 cases of LUAD via correlation analysis. One-to-one correspondence between the risk score and LUAD samples was conducted. The expression level of LUAD immune infiltrating cell markers were explored in the high- and low-risk groups.
Statistical analysis
Cox regression and Kaplan-Meier survival analysis were used to analyze the risk factors associated with OS in patients with LUAD. The univariate and multivariate Cox regression analyses and AIC were used to screen the prognostic factors in patients with LUAD. Correlation analysis was used to analyze the relationship between the risk factors and LUAD immune cell infiltration markers. GraphPrism 5.0 and R (Version 3.6.1) ggplot package were plotted. P<0.05 was regarded as statistically significant.
Results
The expression level of XRCC genes was significantly increased in LUAD tissues
In the Oncomine database, XRCC1, XRCC2, XRCC3, XRCC4, XRCC5, XRCC6, FANCG, and PRKDC were abnormally expressed in pan-cancer tissues, and the expression levels of XRCC genes were mainly increased in pan-cancer tissues (Figure S1). Based on our screening criteria, most of the datasets showed that XRCC genes were predominantly higher in lung cancer tissues. Specifically, the datasets related to the expression of XRCC1, XRCC6, XRCC2, XRCC3, FANCG, XRCC4, XRCC5, and PRKDC were 4 vs. 1, 9 vs. 5, 5 vs. 4, 13 vs. 0, 14 vs. 3, 8 vs. 0, 13 vs. 4, and 18 vs. 2, respectively.
In addition, the expression levels of XRCC1, XRCC6, XRCC3, XRCC2, FANCG, XRCC4, XRCC5, and PRKDC increased in LUAD tissues in the TCGA database, and the difference was statistically significant (Figure 1). In addition, we sorted the data obtained from the TCGA database and matched the tissues one-to-one to show that the expression levels of XRCC1, XRCC6, XRCC3, XRCC2, FANCG, XRCC4, XRCC5, and PRKDC increased in LUAD tissues (Figure 2).
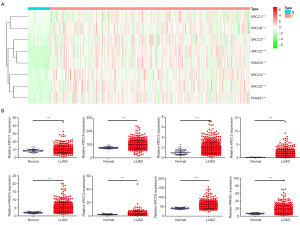
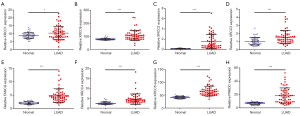
Diagnostic value of XRCC genes in LUAD
The diagnostic value of XRCC genes in LUAD was evaluated via receiver operator characteristic (ROC) analysis. The results showed that the area under the curve (AUC) of XRCC1, XRCC6, XRCC2, XRCC3, FANCG, XRCC4, XRCC5, and PRKDC were all between 0.5 and 1, which was statistically significant (Figure 3). Specifically, the AUCs of XRCC1, XRCC6, XRCC2, XRCC3, FANCG, XRCC4, XRCC5, and PRKDC were 0.6628 (Figure 3A), 0.7785 (Figure 3B), 0.9841 (Figure 3C), 0.7913 (Figure 3D), 0.9943 (Figure 3E), 0.8425 (Figure 3F), 0.8743 (Figure 3G), and 0.8732 (Figure 3H), respectively.
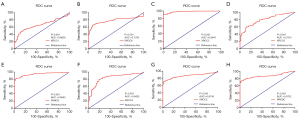
The biological functions of XRCC genes
In LUAD tissues, we observed significant correlations between the expression levels of the following genes: (I) XRCC1 and XRCC3, and FANCG and XRCC4; (II) XRCC3 and FANCG and XRCC2; (III) FANCG and XRCC5, and XRCC2 and PRKDC; (IV) XRCC5 and XRCC6, and XRCC2 and PRKDC; and (V) XRCC2 and PRKDC (Figure S2A). Using the String database, we found that XRCC genes were involved in biological processes such as DNA repair, DNA recombination, response to radiation, response to X-ray, mitotic recombination, and so on, and were also involved in the regulation of non-homologous end-joining and homologous recombination signaling mechanisms (Tables 1-3 and Table S1). In the PPI network, there was a strong functional relationship among the XRCC genes (Figure S2B).
Table 1
GO: BP | Description | P |
---|---|---|
GO:0006302 | Double-strand break repair | 2.94E-11 |
GO:0006281 | DNA repair | 3.49E-11 |
GO:0006310 | DNA recombination | 3.49E-11 |
GO:0010212 | Response to ionizing radiation | 7.62E-10 |
GO:0009314 | Response to radiation | 1.68E-09 |
GO:0006303 | Double-strand break repair via nonhomologous end joining | 2.33E-09 |
GO:0009628 | Response to abiotic stimulus | 4.44E-09 |
GO:0000723 | Telomere maintenance | 1.31E-08 |
GO:0010165 | Response to X-ray | 3.56E-08 |
GO:0010332 | Response to gamma radiation | 2.12E-07 |
GO:0075713 | Establishment of integrated proviral latency | 2.84E-07 |
GO:0006266 | DNA ligation | 1.33E-06 |
GO:0006312 | Mitotic recombination | 2.32E-06 |
GO:0071475 | Cellular hyperosmotic salinity response | 3.91E-05 |
GO:0032481 | Positive regulation of type I interferon production | 5.69E-05 |
GO:0000707 | Meiotic DNA recombinase assembly | 0.00012 |
GO:0000724 | Double-strand break repair via homologous recombination | 0.00012 |
GO:0051351 | Positive regulation of ligase activity | 0.00012 |
GO:0042148 | Strand invasion | 0.00013 |
GO:0000722 | Telomere maintenance via recombination | 0.00019 |
GO:0051103 | DNA ligation involved in DNA repair | 0.00019 |
GO:0071481 | Cellular response to X-ray | 0.00019 |
GO:0048660 | regulation of smooth muscle cell proliferation | 0.00021 |
GO:0006996 | Organelle organization | 0.00024 |
GO:0002218 | Activation of innate immune response | 0.00057 |
GO:0071480 | Cellular response to gamma radiation | 0.00069 |
GO:0007420 | Brain development | 0.00087 |
GO:0032205 | Negative regulation of telomere maintenance | 0.0012 |
GO:0007131 | Reciprocal meiotic recombination | 0.0017 |
GO:0036297 | Interstrand cross-link repair | 0.0017 |
GO:0032508 | DNA duplex unwinding | 0.002 |
GO:0033044 | Regulation of chromosome organization | 0.002 |
GO:0001756 | Somitogenesis | 0.0027 |
GO:0043902 | Positive regulation of multi-organism process | 0.0035 |
GO:0002244 | Hematopoietic progenitor cell differentiation | 0.0037 |
GO:0007399 | Nervous system development | 0.0054 |
GO:0080134 | Regulation of response to stress | 0.0074 |
GO:0022414 | Reproductive process | 0.0083 |
GO:0043085 | Positive regulation of catalytic activity | 0.0086 |
GO:0051704 | Multi-organism process | 0.0086 |
XRCC, X-ray repair of cross-complementary; GO, Gene Ontology; BP, biological process.
Table 2
GO: MF | Description | P |
---|---|---|
GO:0140097 | Catalytic activity, acting on DNA | 2.35E-07 |
GO:0003684 | Damaged DNA binding | 3.37E-07 |
GO:0008094 | DNA-dependent ATPase activity | 3.37E-07 |
GO:0003677 | DNA binding | 6.46E-05 |
GO:0000150 | Recombinase activity | 0.0001 |
GO:0003690 | Double-stranded DNA binding | 0.0001 |
GO:0008022 | Protein C-terminus binding | 0.00044 |
GO:0005524 | ATP binding | 0.00074 |
GO:0042162 | Telomeric DNA binding | 0.00074 |
GO:0003678 | DNA helicase activity | 0.00078 |
GO:0008144 | Drug binding | 0.00088 |
GO:0003697 | Single-stranded DNA binding | 0.0019 |
GO:0016787 | Hydrolase activity | 0.003 |
XRCC, X-ray repair of cross-complementary; GO, Gene Ontology; MF, molecular function.
Table 3
GO: CC | Description | P |
---|---|---|
GO:1990391 | DNA repair complex | 6.40E-11 |
GO:0070419 | Nonhomologous end joining complex | 2.57E-10 |
GO:0000784 | Nuclear chromosome, telomeric region | 8.13E-08 |
GO:0005654 | Nucleoplasm | 1.10E-05 |
GO:0043564 | Ku70:Ku80 complex | 1.10E-05 |
GO:0005958 | DNA-dependent protein kinase-DNA ligase 4 complex | 1.97E-05 |
GO:0033063 | Rad51B-Rad51C-Rad51D-XRCC2 complex | 2.58E-05 |
GO:0005730 | Nucleolus | 6.28E-05 |
GO:0005694 | Chromosome | 6.31E-05 |
GO:0032991 | Protein-containing complex | 6.31E-05 |
GO:0000783 | Nuclear telomere cap complex | 6.43E-05 |
GO:0032993 | Protein-DNA complex | 0.00015 |
GO:0043232 | Intracellular non-membrane-bounded organelle | 0.00028 |
GO:0005657 | Replication fork | 0.00056 |
XRCC, X-ray repair of cross-complementary; GO, Gene Ontology; CC, cellular component.
Consensus clustering of XRCC genes identified two clusters of LUAD with different clinical outcomes
With the evolution of clustering from k=2 to 9, k=2 might be the best choice with the least interference in our clustering (Figure 4A-4C). Therefore, we used k=2 for consensus clustering analysis, and defined it as Cluster1 and Cluster2 groups. PCA was performed in the 535 cases of LUAD from the TCGA database, and the results showed that there was a significant difference between the Cluster1 and Cluster2 groups (Figure 4D). Survival analysis showed that the OS of LUAD patients in Cluster1 was better than that of LUAD patients in Cluster2 (Figure 4E). Correlation analysis showed that there was a significant correlation between T stage and survival status of patients in the Cluster1 and Cluster2 groups (Figure 4F).
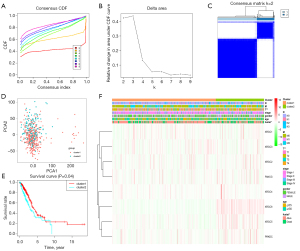
The prognostic value of XRCC genes in patients with LUAD
The value of XRCC genes in the prognosis of LUAD was explored via univariate Cox regression analysis. We found that XRCC4, XRCC5, XRCC6, and PRKDC might be the risk factors affecting the prognosis of LUAD patients (Figure 5A). On this basis, the risk model was constructed under the conditions of multivariate Cox regression analysis and AIC optimization. The results showed that XRCC4, XRCC5, and XRCC6 were independent risk factors affecting the prognosis of patients with LUAD. Kaplan-Meier survival analysis showed that the prognosis of LUAD patients in the high-risk group was worse (Figure 5B). Correlation analysis showed that high- and low-risk were significantly correlated with the gender, clinical stage, T stage, N stage, M stage, and survival status of LUAD patients (Figure 5C). The univariate and multivariate Cox regression analyses showed that the clinical stage and risk score were independent risk factors for poor prognosis in patients with LUAD (Figure 6).
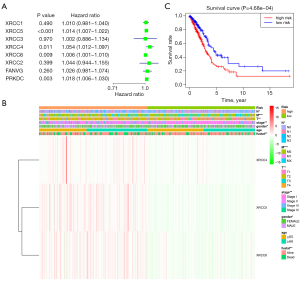
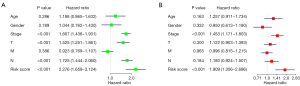
The biological functions and signaling pathways involved in the risk model
According to the median risk score, we divided the gene expression data of the 535 cases of LUAD from the TCGA into high- and low-risk groups to explore the influence of genes in two groups. The GSEA results showed that increased risk might involve biological processes such as regulation of DNA replication, mitotic metaphase plate congression, cell cycle DNA replication (Figure S3), as well as signaling systems such as RNA degradation, cell cycle, oocyte meiosis, basal transcription factors, and DNA replication (Figure S4 and Table 4).
Table 4
Name | Size | NES | NOM P value |
---|---|---|---|
RNA_degradation | 57 | 2.1544359 | 0 |
Cell_cycle | 124 | 2.139343 | 0 |
Nucleotide_excision_repair | 44 | 2.1303906 | 0.001964637 |
OOCYTE_MEIOSIS | 112 | 2.0731578 | 0.001996008 |
Mismatch_repair | 23 | 2.0708838 | 0 |
Basal_transcription_factors | 35 | 2.0075533 | 0 |
DNA_replication | 36 | 1.98874 | 0 |
Proteasome | 44 | 1.9682256 | 0.001972387 |
Ubiquitin_mediated_proteolysis | 133 | 1.9554849 | 0 |
Protein_export | 23 | 1.9503225 | 0 |
pathogenic_escherichia_coli_infection | 55 | 1.9071776 | 0.005825243 |
Citrate_cycle_tca_cycle | 30 | 1.8967364 | 0.004056795 |
Spliceosome | 126 | 1.890303 | 0.004032258 |
Pyrimidine_metabolism | 98 | 1.8573432 | 0.00204499 |
Purine_metabolism | 157 | 1.8310792 | 0.002159827 |
Cysteine_and_methionine_metabolism | 34 | 1.7912648 | 0.003861004 |
P53_signaling_pathway | 68 | 1.733961 | 0.007843138 |
One_carbon_pool_by_folate | 17 | 1.7224773 | 0.016129032 |
RNA_polymerase | 29 | 1.7176231 | 0.018367346 |
Homologous_recombination | 28 | 1.6963832 | 0.034274194 |
Biosynthesis_of_unsaturated_fatty_acids | 22 | 1.6282122 | 0.018072288 |
Riboflavin_metabolism | 15 | 1.620054 | 0.036538463 |
Aminoacyl_trna_biosynthesis | 22 | 1.5583364 | 0.049701788 |
Progesterone_mediated_oocyte_maturation | 85 | 1.4684261 | 0.07370518 |
Amyotrophic_lateral_sclerosis_als | 52 | 1.4148762 | 0.047244094 |
Glyoxylate_and_dicarboxylate_metabolism | 16 | 1.3979565 | 0.115686275 |
Glycolysis_gluconeogenesis | 62 | 1.388122 | 0.082 |
Huntingtons_disease | 177 | 1.3863257 | 0.14256199 |
Terpenoid_backbone_biosynthesis | 15 | 1.378746 | 0.14141414 |
Pentose_phosphate_pathway | 27 | 1.3762755 | 0.1097561 |
Thyroid_cancer | 29 | 1.3680532 | 0.091617934 |
Pancreatic_cancer | 70 | 1.3660588 | 0.11133201 |
Adherens_junction | 73 | 1.3573757 | 0.11576846 |
Base_excision_repair | 33 | 1.34626 | 0.17886178 |
Alzheimers_disease | 163 | 1.3419145 | 0.15352698 |
Tgf_beta_signaling_pathway | 85 | 1.3412957 | 0.11025145 |
N_glycan_biosynthesis | 46 | 1.3321104 | 0.1482966 |
Colorectal_cancer | 62 | 1.3087744 | 0.14705883 |
Propanoate_metabolism | 31 | 1.2553445 | 0.2300195 |
Glycosylphosphatidylinositol_gpi_anchor_biosynthesis | 25 | 1.2523328 | 0.22113504 |
WNT_signaling_pathway | 150 | 1.2449616 | 0.14 |
Chronic_myeloid_leukemia | 73 | 1.227265 | 0.21370968 |
Small_cell_lung_cancer | 84 | 1.197284 | 0.23203285 |
Epithelial_cell_signaling_in_helicobacter_pylori_infection | 68 | 1.1845227 | 0.20315582 |
Prostate_cancer | 89 | 1.1837994 | 0.23883495 |
Pathways_in_cancer | 325 | 1.1785803 | 0.20081967 |
Renal_cell_carcinoma | 70 | 1.164982 | 0.24055666 |
Long_term_potentiation | 70 | 1.1516585 | 0.23943663 |
Cytosolic_dna_sensing_pathway | 54 | 1.1250238 | 0.31769723 |
Ribosome | 87 | 1.1220671 | 0.43037975 |
Nicotinate_and_nicotinamide_metabolism | 24 | 1.1175917 | 0.28849903 |
Regulation_of_actin_cytoskeleton | 212 | 1.1145742 | 0.27991885 |
Parkinsons_disease | 125 | 1.089907 | 0.39793813 |
Vasopressin_regulated_water_reabsorption | 44 | 1.0887667 | 0.33466136 |
Glutathione_metabolism | 47 | 1.0859108 | 0.36452243 |
Lysine_degradation | 44 | 1.0843796 | 0.34068137 |
Snare_interactions_in_vesicular_transport | 38 | 1.0831342 | 0.33714285 |
Valine_leucine_and_isoleucine_degradation | 43 | 1.0764002 | 0.38446215 |
Pyruvate_metabolism | 40 | 1.0609999 | 0.3767821 |
Pentose_and_glucuronate_interconversions | 28 | 1.0568165 | 0.41497976 |
Selenoamino_acid_metabolism | 25 | 1.0436656 | 0.38202247 |
Rig_i_like_receptor_signaling_pathway | 70 | 1.0409458 | 0.3732535 |
Regulation_of_autophagy | 35 | 1.0247588 | 0.4329502 |
Amino_sugar_and_nucleotide_sugar_metabolism | 43 | 1.022107 | 0.4027778 |
Peroxisome | 78 | 1.0060785 | 0.4322709 |
Melanoma | 71 | 1.003775 | 0.44466403 |
Nod_like_receptor_signaling_pathway | 62 | 1.0017914 | 0.46601942 |
Alanine_aspartate_and_glutamate_metabolism | 30 | 0.98454267 | 0.47233203 |
Endocytosis | 181 | 0.9828535 | 0.45691383 |
Fructose_and_mannose_metabolism | 33 | 0.9741695 | 0.46626985 |
Glioma | 65 | 0.9564347 | 0.49203187 |
GSEA, gene set enrichment analysis; NES, normalized enrichment score; NOM, nominal.
The risk model based on XRCC4, XRCC5, and XRCC6 was related to the LUAD immunity
The correlation analysis showed that XRCC4, XRCC5, XRCC6, and their risk model were significantly correlated with the levels of immune factors (Figures 7,8). Specifically, the expression level of XRCC4 was positively correlated with the expression levels of TNFSF4, CD80, PDCD1LG2, CXCL8, etc. (Figure 7A and Table S2), and negatively correlated with the expression levels of CXCL17, IL6R, TAPBP, CXCL16, etc. (Figure 7B and Table S2). The expression level of XRCC5 was positively correlated with the expression levels of PVR, TGFBR1, CXCL8, XCL1, etc. (Figure 7C and Table S2), and negatively correlated with the expression levels of TNFRSF14, HLA-DMA, TMEM173, HLA-DPB1, etc. (Figure 7D and Table S2). The expression level of XRCC6 was positively correlated with the expression levels of CD276, TNFSF13, CXCL16, TNFSF9, etc. (Figure 7E and Table S2), and negatively correlated with the expression levels of CD160, KLRK1, BTLA, CCL16, etc. (Figure 7F and Table S2).
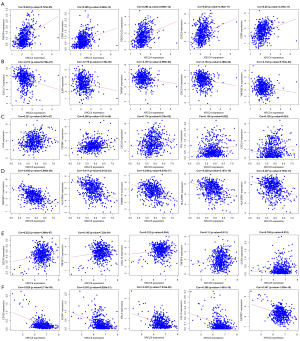
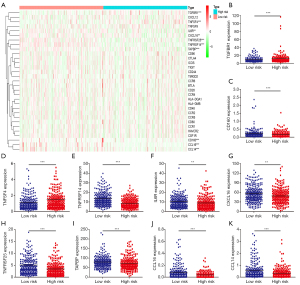
The immune factors associated with the intersection of XRCC4, XRCC5, and XRCC6 in both high- and low-risk groups were validated (Figure 8A). Specifically, the expression levels of TGFBR1, CD160, TNFSF4, TNFRSF14, IL6R, CXCL16, TNFRSF25, TAPBP, CCL16, and CCL14 were significantly associated with high- and low-risk scores (Figure 8B-8K).
Discussion
Persistent failure to repair DNA damage might lead to cell cycle arrest, apoptosis, and genomic instability, which leads to the development of many diseases (21). The XRCC genes are important components of the DNA damage repair mechanism and play important biological roles in cancer progression (21-24). At present, numerous studies have confirmed that polymorphisms of DNA damage repair genes such as XRCC1, XRCC3, and XRCC4 were associated with the survival of patients with lung cancer (25-27). However, the role of XRCC genes in the progression of LUAD has not been fully elucidated. In this study, we observed that the expression levels of XRCC1, XRCC6, XRCC3, XRCC2, FANCG, XRCC3, XRCC4, and PRKDC increased in unpaired and paired LUAD tissues. ROC analysis showed that the AUCs of XRCC1, XRCC6, XRCC2, XRCC3, FANCG, XRCC4, XRCC5, and PRKDC were all between 0.5 and 1. Cox regression analysis demonstrated that XRCC4, XRCC5 and XRCC6 were independent risk factors affecting the prognosis of LUAD patients. Kaplan-Meier survival analysis showed that the prognosis of LUAD patients in the high-risk group was worse, and a high-risk score was significantly correlated with the gender, clinical stage, T stage, N stage, M stage, and survival status of LUAD patients. These results indicated that XRCC4, XRCC5, and XRCC6 play an important role in the progression of LUAD and are expected to become biomarkers for the diagnosis and prognosis of LUAD. Muylaert et al. reported that DNA ligase IV/XRCC4 plays a crucial role in the herpesvirus replication cycle. Reducing DNA ligase IV/XRCC4 could inhibit herpes simplex virus type I DNA replication (28). The expression of Ku86 (XRCC5) is significantly increased in serous ovarian cancer (SOC), and down-regulation of Ku86 expression could promote increased γ-H2AX expression, resulting in the inhibition of cell proliferation, cell cycle block in G2 phase, and the increase of G2/G1. X-ray irradiation could also reduce the expression of Ku86 to promote the above biological effects, and increase the expression of γ-H2AX (29). XRCC6 is overexpressed in human osteosarcoma tissues and cells. The high expression of XRCC6 is related to the clinical stage and tumor size of patients with osteosarcoma. The decreased expression of XRCC6 could inhibit the proliferation of osteosarcoma cells through G2/M phase arrest, which might regulate the growth of osteosarcoma through β-catenin/Wnt signaling pathway (30). The XRCC genes were related factors of DNA damage repair, and the risk model based on XRCC4, XRCC5, and XRCC6 could involve mitotic metaphase plate congression, DNA replication, RNA degradation, the cell cycle, oocyte meiosis, basal transcription factors, DNA replication, and so on. This indicates that XRCC4, XRCC5, and XRCC6 are related to cell cycle, DNA damage and DNA replication; however, further confirmation by basic research is needed.
It is well known that the progression of cancer is related to factors in the immune microenvironment. For example, C-X-C motif chemokine ligand 8 (CXCL8) is associated with a high tumor burden in LUAD and is negatively correlated with DACH1 expression. High DACH1 expression and low CXCL8 expression has been found to prolong the time of death and tumor recurrence of patients. DACH1 can inhibit the activity of the CXCL8 promoter and reduce the level of CXCL8 expression through transcription at the sites of activating protein-1 (AP-1) and nuclear factor-κB (NF-κB) (31). We found that the expression level of XRCC4 was correlated with the expression levels of TNFSF4, CD80, PDCD1LG2, CXCL8, CXCL17, IL6R, TAPBP, CXCL16, and so on; the expression level of XRCC5 was correlated with the expression levels of PVR, TGFBR1, CXCL8, XCL1, TNFRSF14, HLA-DMA, TMEM173, HLA-DPB1, and so on; and the expression level of XRCC6 was correlated with the expression level of CD276, TNFSF13, CXCL16, TNFSF9, CD160, KLRK1, BTLA, CCL16, and so on. In the high- and low-risk groups, it was found that the expression levels of TGFBR1, CD160, TNFSF4, TNFRSF14, IL6R, CXCL16, TNFRSF25, TAPBP, CCL16, and CCL14 were significantly correlated with a high risk. Meanwhile, Jiang et al. reported that TGFBR1, TNFSF4, and IL6R were associated with lung cancer progression (32-35), which provided some evidence for our research.
The risk model based on TCGA data has good prognostic value. However, clinical tissue samples should be collected to verify the expression of XRCC4/5/6 in LUAD tissues via the RT-PCR and western-blot, and the value of XRCC4/5/6 in the diagnosis and prognosis of LUAD was analyzed. In addition, we need to build cell models in the future to explore the cell growth, migration and singaling mechanisms of XRCC4/5/6 in the progression of LUAD. Generally speaking, the XRCC genes played an important role in the diagnosis and prognosis of LUAD. XRCC4, XRCC5, and XRCC6 were independent risk factors affecting the prognosis of LUAD patients. There were significant differences in prognosis, sex, clinical stage, T stage, N stage, M stage, and survival status of LUAD patients in the high- and low-risk groups. The clinical stage and risk score were independent risk factors for poor prognosis in patients with LUAD. The risk model was involved in mitotic metaphase plate congression, RNA degradation, cell cycle, oocyte meiosis, basal transcription factors, DNA replication, and other processes. XRCC4, XRCC5, XRCC6, and the risk scores were significantly correlated with the expression levels of immune factors of TGFBR1, CD160, TNFSF4, TNFRSF14, IL6R, CXCL16, TNFRSF25, TAPBP, CCL16, and CCL14.
Conclusions
In this study, the risk model based on XRCC4, XRCC5, and XRCC6 could predict the progression of LUAD patients.
Acknowledgments
Funding:
Footnote
Reporting Checklist: The authors have completed the TRIPOD reporting checklist. Available at https://dx.doi.org/10.21037/tcr-21-1431
Conflicts of Interest: All authors have completed the ICMJE uniform disclosure form (available at https://dx.doi.org/10.21037/tcr-21-1431). The authors have no conflicts of interest to declare.
Ethical Statement: The authors are accountable for all aspects of the work in ensuring that questions related to the accuracy or integrity of any part of the work are appropriately investigated and resolved. The study was conducted in accordance with the Declaration of Helsinki (as revised in 2013). Institutional ethical approval and informed consent were waived.
Open Access Statement: This is an Open Access article distributed in accordance with the Creative Commons Attribution-NonCommercial-NoDerivs 4.0 International License (CC BY-NC-ND 4.0), which permits the non-commercial replication and distribution of the article with the strict proviso that no changes or edits are made and the original work is properly cited (including links to both the formal publication through the relevant DOI and the license). See: https://creativecommons.org/licenses/by-nc-nd/4.0/.
References
- Na R, Wu Y, Jiang G, et al. Germline mutations in DNA repair genes are associated with bladder cancer risk and unfavourable prognosis. BJU Int 2018;122:808-13. [Crossref] [PubMed]
- De Gregoriis G, Ramos JA, Fernandes PV, et al. DNA repair genes PAXIP1 and TP53BP1 expression is associated with breast cancer prognosis. Cancer Biol Ther 2017;18:439-49. [Crossref] [PubMed]
- Jhuraney A, Woods NT, Wright G, et al. PAXIP1 Potentiates the Combination of WEE1 Inhibitor AZD1775 and Platinum Agents in Lung Cancer. Mol Cancer Ther 2016;15:1669-81. [Crossref] [PubMed]
- Crowe DL, Lee MK. New role for nuclear hormone receptors and coactivators in regulation of BRCA1-mediated DNA repair in breast cancer cell lines. Breast Cancer Res 2006;8:R1. [Crossref] [PubMed]
- Li J, Zhang J, Liu Y, et al. Increased expression of DNA repair gene XPF enhances resistance to hydroxycamptothecin in bladder cancer. Med Sci Monit 2012;18:BR156-62. [Crossref] [PubMed]
- Rizeq B, Sif S, Nasrallah GK, et al. Novel role of BRCA1 interacting C-terminal helicase 1 (BRIP1) in breast tumour cell invasion. J Cell Mol Med 2020;24:11477-88. [Crossref] [PubMed]
- Abdel-Fatah T, Sultana R, Abbotts R, et al. Clinicopathological and functional significance of XRCC1 expression in ovarian cancer. Int J Cancer 2013;132:2778-86. [Crossref] [PubMed]
- Qin CJ, Song XM, Chen ZH, et al. XRCC2 as a predictive biomarker for radioresistance in locally advanced rectal cancer patients undergoing preoperative radiotherapy. Oncotarget 2015;6:32193-204. [Crossref] [PubMed]
- Ke D, Guo Q, Fan TY, et al. Analysis of the Role and Regulation Mechanism of hsa-miR-147b in Lung Squamous Cell Carcinoma Based on The Cancer Genome Atlas Database. Cancer Biother Radiopharm 2021;36:280-91. [Crossref] [PubMed]
- Liu H, Qiu C, Wang B, et al. Evaluating DNA Methylation, Gene Expression, Somatic Mutation, and Their Combinations in Inferring Tumor Tissue-of-Origin. Front Cell Dev Biol 2021;9:619330. [Crossref] [PubMed]
- Chen H, Luo J, Guo J. Development and validation of a five-immune gene prognostic risk model in colon cancer. BMC Cancer 2020;20:395. [Crossref] [PubMed]
- Lyu Z, Li N, Chen S, et al. Risk prediction model for lung cancer incorporating metabolic markers: Development and internal validation in a Chinese population. Cancer Med 2020;9:3983-94. [Crossref] [PubMed]
- Rhodes DR, Kalyana-Sundaram S, Mahavisno V, et al. Oncomine 3.0: genes, pathways, and networks in a collection of 18,000 cancer gene expression profiles. Neoplasia 2007;9:166-80. [Crossref] [PubMed]
- Wang J, Zhang C, He W, et al. Effect of m6A RNA Methylation Regulators on Malignant Progression and Prognosis in Renal Clear Cell Carcinoma. Front Oncol 2020;10:3. [Crossref] [PubMed]
- Zhu J, Wang M, Hu D. Deciphering N6-Methyladenosine-Related Genes Signature to Predict Survival in Lung Adenocarcinoma. Biomed Res Int 2020;2020:2514230. [Crossref] [PubMed]
- Zhuang Z, Li Y, Hong Y, et al. A novel prognostic score based on systemic inflammatory biomarkers for patients with oral squamous cell carcinoma. Oral Dis 2021; [Epub ahead of print]. [Crossref] [PubMed]
- Guo Q, Li D, Luo X, et al. The Regulatory Network and Potential Role of LINC00973-miRNA-mRNA ceRNA in the Progression of Non-Small-Cell Lung Cancer. Front Immunol 2021;12:684807. [Crossref] [PubMed]
- Ma X, Tao R, Li L, et al. Identification of a 5-microRNA signature and hub miRNA-mRNA interactions associated with pancreatic cancer. Oncol Rep 2019;41:292-300. [PubMed]
- Li D, Ji YM, Guo JL, et al. Upregulated expression of MTFR2 as a novel biomarker predicts poor prognosis in hepatocellular carcinoma by bioinformatics analysis. Future Oncol 2021;17:3187-201. [Crossref] [PubMed]
- Guo Q, Ke XX, Fang SX, et al. PAQR3 Inhibits Non-small Cell Lung Cancer Growth by Regulating the NF-κB/p53/Bax Axis. Front Cell Dev Biol 2020;8:581919. [Crossref] [PubMed]
- Santana T, Sá MC, de Moura Santos E, et al. DNA base excision repair proteins APE-1 and XRCC-1 are overexpressed in oral tongue squamous cell carcinoma. J Oral Pathol Med 2017;46:496-503. [Crossref] [PubMed]
- Liu ZH, Wang N, Wang FQ, et al. High expression of XRCC5 is associated with metastasis through Wnt signaling pathway and predicts poor prognosis in patients with hepatocellular carcinoma. Eur Rev Med Pharmacol Sci 2019;23:7835-47. [PubMed]
- Niu Y, Zhang X, Zheng Y, et al. XRCC1 deficiency increased the DNA damage induced by γ-ray in HepG2 cell: Involvement of DSB repair and cell cycle arrest. Environ Toxicol Pharmacol 2013;36:311-9. [Crossref] [PubMed]
- Gao Y, Ferguson DO, Xie W, et al. Interplay of p53 and DNA-repair protein XRCC4 in tumorigenesis, genomic stability and development. Nature 2000;404:897-900. [Crossref] [PubMed]
- Singh A, Singh N, Behera D, et al. Polymorphism in XRCC1 gene modulates survival and clinical outcomes of advanced North Indian lung cancer patients treated with platinum-based doublet chemotherapy. Med Oncol 2017;34:64. [Crossref] [PubMed]
- Shao N, Jiang WY, Qiao D, et al. An updated meta-analysis of XRCC4 polymorphisms and cancer risk based on 31 case-control studies. Cancer Biomark 2012-2013;12:37-47. [Crossref] [PubMed]
- Osawa K, Nakarai C, Uchino K, et al. XRCC3 gene polymorphism is associated with survival in Japanese lung cancer patients. Int J Mol Sci 2012;13:16658-67. [Crossref] [PubMed]
- Muylaert I, Elias P. Knockdown of DNA ligase IV/XRCC4 by RNA interference inhibits herpes simplex virus type I DNA replication. J Biol Chem 2007;282:10865-72. [Crossref] [PubMed]
- Ma Q, Kai J, Liu Y, et al. Targeting Ku86 enhances X-ray-induced radiotherapy sensitivity in serous ovarian cancer cells. Int J Biochem Cell Biol 2020;121:105705. [Crossref] [PubMed]
- Zhu B, Cheng D, Li S, et al. High Expression of XRCC6 Promotes Human Osteosarcoma Cell Proliferation through the β-Catenin/Wnt Signaling Pathway and Is Associated with Poor Prognosis. Int J Mol Sci 2016;17:1188. [Crossref] [PubMed]
- Liu Q, Li A, Yu S, et al. DACH1 antagonizes CXCL8 to repress tumorigenesis of lung adenocarcinoma and improve prognosis. J Hematol Oncol 2018;11:53. [Crossref] [PubMed]
- Jiang F, Yu Q, Chu Y, et al. MicroRNA-98-5p inhibits proliferation and metastasis in non-small cell lung cancer by targeting TGFBR1. Int J Oncol 2019;54:128-38. [PubMed]
- Li Y, Chen Y, Miao L, et al. Stress-induced upregulation of TNFSF4 in cancer-associated fibroblast facilitates chemoresistance of lung adenocarcinoma through inhibiting apoptosis of tumor cells. Cancer Lett 2021;497:212-20. [Crossref] [PubMed]
- Lei Y, Zang R, Lu Z, et al. ERO1L promotes IL6/sIL6R signaling and regulates MUC16 expression to promote CA125 secretion and the metastasis of lung cancer cells. Cell Death Dis 2020;11:853. [Crossref] [PubMed]
- Liang K, Liu Y, Eer D, et al. High CXC Chemokine Ligand 16 (CXCL16) Expression Promotes Proliferation and Metastasis of Lung Cancer via Regulating the NF-κB Pathway. Med Sci Monit 2018;24:405-11. [Crossref] [PubMed]
(English Language Editor: A. Kassem)