Differentiating mass-like tuberculosis from lung cancer based on radiomics and CT features
Introduction
According to World Health Organization data, China has one of the highest burdens of tuberculosis (TB) in the world (1). With population aging and widespread antibiotics use, there is an increasing number of TB subtypes with irregular masses, which overlaps much phenotypes with peripheral lung cancer and can easily be confused with lung cancer. Previous studies used CT morphology combined with laboratory detection as the basis of traditional diagnosis. In clinic, although sputum detection of Mycobacterium tuberculosis is the diagnostic “gold standard” for TB, but its positivity rate is low, and sputum culture and purified protein derivative (PPD) tests are time-consuming and limited by a variety of factors. A positive auxiliary T-spot test indicates the possibility of TB infection (2). Objectively, accurately, and non-invasively improving the diagnosis and identification of TB has become the focus of imaging.
Radiomics is emerging as a research tool that allows for the extraction and quantitative analysis of the massive gray-scale data in images that cannot be recognized by the naked eye which indirectly reflects the heterogeneity of lesions. Studies on the correlation in features of radiomics with the gene phenotype, pathological type, clinical stage, and efficacy evaluation of tumors has been widely carried out (3-6). The present study aims at innovative exploration of exploring the feasibility and efficacy of combining CT morphology with radiomics texture features for differentiating mass-like TB and peripheral lung cancer, which is more objective.
We present the following article in accordance with the STARD reporting checklist (available at https://dx.doi.org/10.21037/tcr-21-1719).
Methods
General data
CT data of 37 cases of mass-like TB (the TB group) and 41 cases of peripheral lung cancer (the PLC group) were included in this retrospective analysis. The TB group comprised 27 males and 10 females aged 40–84 years, with an average age of 60.4 years old. The PLC group comprised 28 males and 15 females, aged 40–86 years old, with an average age of 63.3 years old. Among the 41 cases in the PLC group were 21 cases of squamous cell carcinoma and 20 cases of adenocarcinoma. None of the patients had a history of treatment before chest CT scan.
The criteria for inclusion in this study were as follows: (I) patients with peripheral irregular masses with a maximum diameter of ≥3.0 cm, as determined by CT; (II) complete pathological results under surgical resection; (III) needle biopsy: TB confirmed by acid-fast staining and laboratory examinations, or lung cancer diagnosed with immunohistochemistry and negative lymph node metastasis; (IV) complete clinical and imaging data. The exclusion criteria were as follows: (I) patients with a history of TB or diagnostic anti-TB treatment; (II) patients with a history of lung cancer, clear lymph nodes, or distant metastasis; (III) patients with unclear histopathology; (IV) patients with poor quality images which affected analysis and measurement. The study was approved by the Research Ethics Committee of Anhui Provincial Cancer Hospital (2021-YXK-03). All procedures performed in this study involving human participants were in accordance with the Declaration of Helsinki (as revised in 2013). Individual consent for this retrospective analysis was waived.
Equipment
All patients underwent plain and enhanced scanning using a 64-row gem energy spectrum CT scanner (GE, USA), with the scanning parameters of tube voltage (120 kVp), tube current (100–300 mAs), collimator (64 layers ×0.625 mm), rotation speed (0.5 s), field of view (350 mm ×350 mm), matrix (512×512), layer thickness (5.0 mm), and interval (5.0 mm). After undergoing a plain scan, patients were intravenously injected with the contrast agent iodophor, at a flow rate of 3.0–3.5 mL/s and a dosage of 1.5 mL/kg. Dual-phase scanning in the arterial phase (AP) and venous phase (VP) was performed, and scans were reconstructed with a slice thickness of 1.25 mm.
Evaluation of CT signs
The following CT signs were evaluated: lesion location, maximum diameter, morphological type (regular or irregular), mass substance (density, enhancement, calcification, or necrosis), edge (lobular or burr sign), surroundings (exudation or satellite focus), pleura (thickening or depressed sign), bronchial signs (truncated or cone-shaped), and vascular signs (blood vessel thickening or blood vessel clustering).
Extraction of texture features
The maximum sections of pulmonary tuberculosis and lung cancer masses were observed on the axial CT scan lung window (Figure 1A-1D), and the solid components and boundaries of the masses were observed on the soft tissue window (Figure 1B-1E) at the same level during the enhanced venous phase. The ITK-SNAP software (University of Pennsylvania, USA) was used to scan the soft tissue window on CT and manually outline the region of interest (ROI, Figure 1C-1F). Large vessels and cavities were avoided based on the vein enhancement phase at the same level, and the width and position of the window were set as 400 HU and 40 HU, respectively. The original image and ROI were imported into the Artificial Intelligence Kit version V3.2.2.R (AK, GE, USA). After voxel adjustment, resampling, noise reduction, and gray-scale discretization, standardized reconstruction and fusion were performed (Figure 2A).
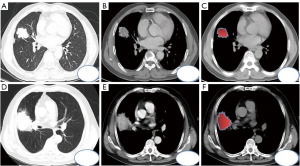
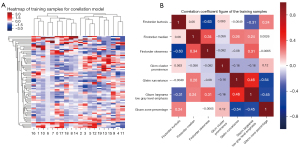
Texture features included first-order features, gray level co-occurrence matrix (GLCM) features, gray level size zone matrix (GLSZM) features, and gray level run length matrix (GLRLM) features.
Reproducibility assessment
The reproducibility assessment was carried out by two senior doctors (physician A and physician B) with rich experience in chest imaging under unknown clinical pathological results. Physician A performed CT sign evaluation, ROI delineation, and feature extraction of all the data twice, which allowed the consistency of the measurer. Physician B performed CT sign evaluation, ROI setting, and feature extraction only once, and their consistency with physician A was evaluated. An intergroup and intragroup consistency coefficient (ICC) of >0.75 indicated good consistency.
Statistical analysis
The collected data were analyzed using SPSS 20 (IBM, USA), MedCalc 16.8.4 (Beijing Reachsoft, China), and IPMs (version 1.1.1.R). First, IPMs software was used to reduce the dimensionality of all texture parameters. After dimensionality reduction, data distribution type detection was performed on the texture parameters and CT imaging features obtained. According to the data distribution type, the independent-samples t-test, Mann-Whitney U test, Pearson chi-square test, or Fisher’s exact test was performed to compare the two groups, with P<0.05 indicating a statistically significant difference. Finally, logistic regression analysis was used to establish a texture feature model, a morphology model, and a combined prediction model, and the diagnostic efficacy was evaluated using the receiver operating characteristic (ROC) curve.
Results
Comparison of morphological characteristics between the TB group and PLC group
The comparison of 11 qualitative CT imaging characteristics between the TB group and PLC group showed that 8 characteristics (lobular sign, long burr sign, exudation, pleura, necrosis, trachea, blood vessel, calcification, satellite lesion) differed significantly (P<0.05, Table 1). Meanwhile, morphology, short burr sign, and peripheral exudation were not statistically significantly different between the groups (P>0.05, Table 1).
Table 1
Sign | Category | TB group (n=37), n (%) | PLC group (n=41), n (%) | P |
---|---|---|---|---|
Morphology | Irregular | 34 (91.90) | 32 (78.00) | 0.121 |
Regular | 3 (8.10) | 9 (22.00) | ||
Lobular | None | 26 (70.27) | 12 (29.26) | 0.001a |
Shallow | 8 (21.62) | 19 (46.35) | ||
Deep | 3 (8.11) | 10 (24.39) | ||
Short burr | Without | 26 (70.27) | 30 (73.17) | 0.121 |
With | 11 (29.73) | 11 (26.83) | ||
Long burr | Without | 1 (2.70) | 8 (19.51) | 0.021a |
With | 36 (97.30) | 33 (80.49) | ||
Exudation | Without | 7 (18.92) | 6 (14.63) | 0.763 |
With | 30 (81.08) | 35 (85.37) | ||
Pleura | None | 1 (2.70) | 2 (4.88) | 0.015a |
Indentation | 7 (18.92) | 19 (46.34) | ||
Thickening | 29 (78.38) | 20 (48.78) | ||
Necrosis | None | 6 (16.22) | 10 (24.39) | 0.026a |
Smooth necrotic wall | 20 (54.05) | 10 (24.39) | ||
Necrotic wall blurring | 11 (29.73) | 21 (51.22) | ||
Trachea | None | 10 (27.03) | 7 (17.08) | 0.025a |
Truncation | 5 (13.51) | 17 (41.46) | ||
Cone | 22 (59.46) | 17 (41.46) | ||
Blood vessel | Thickening | 31 (83.78) | 24 (58.54) | 0.024a |
Bundling | 6 (16.22) | 17 (41.46) | ||
Calcification | Without | 24 (64.86) | 38 (92.68) | 0.004a |
With | 13 (35.14) | 3 (7.32) | ||
Satellite lesion | Without | 21 (56.76) | 32 (78.00) | 0.044a |
With | 16 (42.24) | 9 (22.00) |
a, indicates a statistically significant difference (P<0.05) between the two groups. Pearson’s chi-square test was selected as the testing method, and if the expected frequency of the cell was <5, Fisher’s exact test was used. TB, tuberculosis; PLC, peripheral lung cancer.
Seven quantitative CT imaging characteristics were incorporated into a comparative analysis between the two groups. The results revealed that 5 quantitative characteristics (size, plain scan CT value, arterial CT value, venous CT value, the difference between the venous and plain CT) were statistically significantly different between the two groups (P<0.05, Table 2). Meanwhile, the difference between AP and plain CT and between the VP and AP CT were not statistically significant (P>0.05, Table 2).
Table 2
Sign | TB group (n=37) | PLC group (n=41) | t/z | P |
---|---|---|---|---|
Maximum diameter | 40.22±10.67 | 46.06±14.02 | −1.904 | 0.061 |
Mean of plain CT scan value | 26.23±13.68 | 31.64±8.09 | −2.149 | 0.035a |
Mean of CT value in the AP | 33.78±16.73 | 47.87±11.21 | −4.409 | 0.041a |
Mean CT value in the VP | 36.53±16.82 | 52.77±11.22 | −5.509 | <0.001a |
CT (AP − plain scan) | 7.55±8.11 | 16.23±7.82 | −4.812 | <0.001a |
CT (VP − plain scan) | 8.4±14.85 | 21.13±8.08 | −4.658 | <0.001a |
CT (VP − AP) | 0.56±6.57 | 4.89±5.70 | −1.541 | 0.128 |
Normally distributed data are expressed as the mean ± standard deviation, and data with a skewed distribution are expressed as the median and interquartile range; a, indicates a statistically significant difference (P<0.05) between the two groups. Data conforming to a normal distribution in both groups were analyzed by independent-samples t-test; otherwise, the Mann-Whitney U test was used. TB, tuberculosis; PLC, peripheral lung cancer; VP, venous phase; AP, arterial phase.
Differences in texture characteristics between the two groups
After row dimensionality reduction, 7 texture parameters were included for comparative analysis (Figure 2B) including 3 first-order features (Kurtosis, Median, and Skewness), 1 gray-level co-occurrence matrix parameter (glcm_Cluster Prominence, CP), 1 gray-scale run length matrix parameter (glrlm_Run Variance, RV), and 2 gray-scale region size matrix parameter [glszm Large Area Low Gray Level Emphasis (LALGLE) and glszm_Zone Percentage (ZP)]. The comparison between the groups showed that the differences between the 7 texture parameters were statistically significant (P<0.05, Table 3).
Table 3
Index | BT group (n=37) | PLC group (n=41) | t/z | P |
---|---|---|---|---|
Kurtosis | −0.20, 1.15 | −0.53, 0.57 | −2.757 | 0.006a |
Median | −0.27±1.24 | 0.25±0.65 | −2.353 | 0.021a |
Skewness | −0.21±1.14 | 0.43, 0.66 | −2.852 | 0.004a |
CP | −0.19, 0.25 | −0.22, 0.02 | −4.108 | <0.001a |
RV | −0.64, 0.59 | 0.08, 1.45 | −4.868 | <0.001a |
LALGE | −0.67, 0.30 | 0.03, 1.66 | −5.228 | <0.001a |
ZP | 0.56±1.16 | −0.51±0.43 | 5.506 | <0.001a |
Normally distributed data were expressed as the mean ± standard deviation, and data with skewed distribution were expressed as the median and interquartile range; a, indicates a statistically significant difference (P<0.05) between the two groups. Data conforming to normal distribution in both groups were analyzed by independent-samples t-test; otherwise, the Mann-Whitney U test was performed. TB, tuberculosis; PLC, peripheral lung cancer; CP, cluster prominence; RV, run variance; LALGE, large area low gray level emphasis; ZP, zone percentage.
Logistic regression analysis and diagnostic efficacy evaluation
The 7 texture parameters, 4 quantitative parameters, and 8 qualitative parameters that differed significantly between the two groups were included in logistic regression analysis to establish a texture feature model, a morphological model, and a combined prediction model (P<0.05). The model equations were as follows: texture feature model = 0.283 + 1.587 × LALGE − 1.418 × ZP; morphological model = 2.084 − 0.221 × mean CT value of VP + 0.259 × CT (VP − plain scan) + 2.25 × foliar changes − 2.466 × pleural changes; combined prediction model = 2.086 + 0.228 × CT (VP − plain scan) − 4.128 × pleural changes + 2.558 × LALGE − 2.228 × ZP.
The ROC curve analysis showed that the area under the ROC curve (AUC) values of the texture feature, morphology, and combined prediction models were 0.856, 0.950, and 0.982, respectively (P<0.05, Figure 3, Table 4).
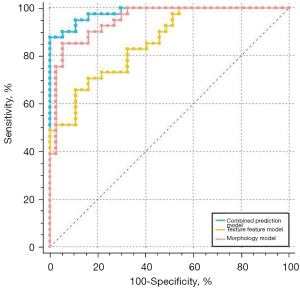
Table 4
Model | AUC (95% CI) | Sensitivity | Specificity | P |
---|---|---|---|---|
Texture feature | 0.856 (0.759–0.925) | 65.85% | 89.19% | <0.001a |
Morphology | 0.950 (0.875–0.986) | 85.37% | 94.59% | <0.001a |
Combined prediction | 0.982 (0.923–0.999) | 87.80% | 100.00% | <0.001a |
a, indicated statistically significant difference (P<0.05) between the two groups. AUC, area under the receiver operating characteristic curve; CI, confidence interval.
A comparison of the ROC curves of the 3 models showed that the AUC values of the combined prediction model and the morphology model were significantly higher than that of the texture feature model (P<0.05), while there was no significant difference between the AUC values of the combined prediction and morphology models (P>0.05).
Discussion
Conventional CT scan is the main examination method for distinguishing TB from lung cancer. Diagnosis and differentiation depend on the location, size and shape of the mass, as well as its lobes, borders, density, and enhancement characteristics. Traditional qualitative analysis of CT images involves making preliminary diagnosis based on the characteristics of the mass. However, the diagnostic accuracy is closely related to doctors’ experience, judgment of signs is also subjective and limited, lacks quantitative indicators, has poor reproducibility, and is closely related to the physician’s experience. Therefore, diagnosis mainly depends on biopsy pathology.
With the rapid development of radiomics in recent years, studies on the differentiation of granulomatous lung lesions from lung cancer have been conducted (7,8). With the deepening of research, it is possible to differentiate specific cases of atypical mass-like TB and peripheral lung cancer. In this study, CT plain scan texture feature parameters were used in combination with morphological features to evaluate the differences of parameters between patients with TB and lung cancer. Logistic regression analyses were performed to analyze the indicators with significant differences between the two groups, and the predictive factors were used to evaluate the characteristic parameters both independently and together according to the ROC curve.
Previous studies have shown that shallow lobes, long burrs, satellite lesions, calcification, and mild or circular enhancement are all the characteristic of mass-like TB (9,10). The edge of the mass was formed by the fusion of fibrous cords or protruding caseous nodules. And the superficial lobed and long burrs of cords were common to see. Tuberculosis often has bronchial spread and satellite foci around nodules or masses. Calcification is the most common complication of tuberculosis, mainly distributed around caseous necrosis and cavity wall. The enhancement showed that the central necrotic area was not enhanced or slightly enhanced, and the granulation tissue was ringed, and the inner edge of the central necrotic area was smooth (11). The pattern of enhancement is related to the lack of vascular structure in central coagulation necrosis, surrounding by epithelioid cells, giant cells unequal to Langerhans, infiltration of peripheral lymphocytes and proliferation of fibroblasts (12). Research on peripheral lung cancer with different growth speed in all directions around the block structure, reaction, and tumour fibroblasts edge is more deep lobulated, while empty and calcification re relatively rare, the density of small lump is uniform. The surrounding shows infiltrative growth and the burrs are short and soft, due to mass jostled or tumour fibrous tissue hyperplasia. Bronchovascular bundles are usually extruded, contracted or gathered. The enhancement showed uneven enhancement from mild to moderate, like marshy, with a patchy and slightly fuzzy necrotic area in the center, and the enhancement was lower than the surrounding area (13,14). However, the mechanism is related to the uneven blood supply caused by immature intratumor vessels and static and dynamic fistula, and the hypoxia caused by the lower rate of angiogenesis than tumor growth. The absolute value of standardized iodine content (dNIC) in and around the center of inflammatory mass was significantly lower than that in lung cancer (12). The results of this study showed that the AUC of quantitative CT was 0.586–0.807 and that of qualitative CT was 0.569–0.986. Furthermore, the diagnostic efficiency of the presence of necrosis was 0.986, which indirectly indicates that TB masses mainly have caseous necrosis at their center.
Multiple studies have shown that radiomics can be applied to quantitatively evaluate the difference in texture characteristics between peripheral lung cancer and inflammatory masses, with differential diagnostic efficacy (13,14). For instance, Dennie et al. (7) studied the heterogeneity of lung cancer and infective granulomas, and found differences in texture characteristics between the central area and the edge of the mass. Chen et al.’s study (8) on lung adenocarcinoma and inflammatory granuloma reported that the AUC of imaging features within nodules was 0.75, compared to that of 0.80 for the combination of nodule and surrounding histological features, indicating that the omics features around nodules carry great value in the prediction of malignant tumors. Suo et al. (15) identified sex and age, irregular edges and lobular features of the lesion, and radiomics features as the main factors for distinguishing lung adenocarcinoma from granulomatous TB, which indicates that the combination of a clinical risk factor model and an imaging features model is of great value for differentiating the two diseases. Beig et al. (16) showed that a nomogram based on imaging features from within a 4-mm peripheral radius of the nodule had the highest efficiency for distinguishing lung cancer and TB; in the training group, the AUC was 0.914 (with a sensitivity and specificity of 0.890 and 0.796, respectively), and in the verification group, the AUC was 0.900 (with a sensitivity and specificity of 0.788 and 0.907, respectively). Their observations showed that the 4-mm area around the tumor had a more potent distinguishing value than the intratumoral features.
The 7 texture parameters screened out in this study showed significant differences between the TB group and the PLC group (P<0.05). The kurtosis of the first-grade radiomics features reflected the peak apex degree of the gray distribution within the pixel. High kurtosis indicated that the gray area was further away from the mean distribution; low kurtosis indicated the opposite distribution trend; the median indicated the median gray intensity level in the pixel; and the skewness reflected the offset characteristics and symmetry of the gray distribution relative to the mean. The closer the gray distribution was to the tail, the higher the tumor heterogeneity, which indicates the possibility of progression. The co-occurrence matrix feature (glcm_Cluster Prominence, CP) describes the tumor complexity, variation, and texture thickness, as well as gray-level and contrast-related information, which reflects the degree of kurtosis and asymmetry of the gray scale matrix, and differences in image streaks and texture gully depth of the gray-scale run-length matrix (glrlm_Run Variance, RV) between histopathological types. Large area low gray level emphasis (LALGLE) reflects the proportion of the large area gray scale in the low-level grayscale, which indicates differences in tissue structure within the mass. The zone percentage (ZP) reflects the roughness of texture. In this study, the AUC of the independent texture parameter was 0.650–0.844, which shows the high heterogeneity and complexity of the internal tissue composition of lung cancer. Meanwhile, TB masses mainly consist of caseous necrosis surrounded by heterogeneous granulation tissue.
In this study, logistic regression analysis of variables and characteristics with significant differences between groups showed that kurtosis and the presence of clear-edge necrosis were independent predictive factors for distinguishing mass-like TB from peripheral lung cancer, with an AUC of 0.982 and a sensitivity and specificity of 87.8% and 100%, respectively. The sensitivity in this study is similar to that reported in the literature (8), while the specificity is higher than that reported previously. This difference may be related to the TB masses in this study consisting mostly or completely of caseous necrosis, and there being obvious differences in the deviation of gray distribution from the mean value and the symmetry of the fine structure and the gray-scale distribution in lung cancer.
With the clinical application of multimodal quantitative parameter imaging and artificial intelligence (AI), the imaging diagnosis of intrapulmonary mass has gone beyond morphological evaluation, and its accuracy has been significantly improved. It was reported that energy spectrum CT quantitative analysis has certain advantages in the differential diagnosis of lung cancer and inflammatory myofibroblastoma (17). Cui et al. (18) applied MRI combined with diffusion-weight imaging (DWI) sequence imaging to study pulmonary tuberculosis, and the features of nodular changes, nodules or masses, cavities and other features showed a high consistency with CT. T2WI can effectively distinguish caseous necrosis and liquefaction necrosis, predict different pathological stages of tuberculosis, and the apparent diffusion coefficient (ADC) can quantify the degree of limited diffusion of water molecules in different cells and tissues, and distinguish the cell density of granuloma and malignant tumor. Yu et al. (19) constructed a convolutional neural network CNN training set model based on 3d deep learning based on the data of more than 40,000 CT images of pulmonary nodular lesions based on AI computer-aided diagnosis technology. In the test set, 94.4% confidence intervals were obtained. Xing et al. (20) quantified bronchiectasis and cavitation in lung CT images of 116 patients and obtained 103 quantitative features. Linear Support Vector Machine (SVM) was used to classify and identify discriminative features, realizing quickly diagnose nontuberculous mycobacter in the lungs.
However, this study has a number of limitations. First, the sample size was small and the results might have bias. Second, manual ROI delineation was limited to two-dimensional data at the largest level of the lesion rather than three-dimensional volume data, which might have caused deviations in the structure and heterogeneity of tissue components. Third, this study was a retrospective rather than prospective study with training group models and verification. In the future, the results of this study need to be further verified by using an enlarged sample size and multicentral clinical trials.
In conclusion, the lungs tumor CT morphology performance article shallow lobe, long cable, satellite, calcification and enhance oven is mild or circular reinforcement, necrosis. Prediction model of evaluating diagnostic performance is set up by combining omics level gray-scale image (kurtosis, the median, partial degrees) and gray level co-occurrence matrix, the length of the gray-level run-length matrix and gray area size matrix characteristics and difference between lung cancer., It can predict the diagnosis of tuberculosis at the maximum level of the lesion, effectively and noninvasively improve the accuracy of diagnosis, further promote the management of pulmonary mass and the formulation of treatment plans, and improve the quality of life of patients.
Acknowledgments
Funding: None.
Footnote
Reporting Checklist: The authors have completed the STARD reporting checklist. Available at https://dx.doi.org/10.21037/tcr-21-1719
Data Sharing Statement: Available at https://dx.doi.org/10.21037/tcr-21-1719
Conflicts of Interest: All authors have completed the ICMJE uniform disclosure form (available at https://dx.doi.org/10.21037/tcr-21-1719). The authors have no conflicts of interest to declare.
Ethical Statement: The authors are accountable for all aspects of the work in ensuring that questions related to the accuracy or integrity of any part of the work are appropriately investigated and resolved. The study was approved by the Research Ethics Committee of Anhui Provincial Cancer Hospital (2021-YXK-03). All procedures performed in this study involving human participants were in accordance with the Declaration of Helsinki (as revised in 2013). Individual consent for this retrospective analysis was waived.
Open Access Statement: This is an Open Access article distributed in accordance with the Creative Commons Attribution-NonCommercial-NoDerivs 4.0 International License (CC BY-NC-ND 4.0), which permits the non-commercial replication and distribution of the article with the strict proviso that no changes or edits are made and the original work is properly cited (including links to both the formal publication through the relevant DOI and the license). See: https://creativecommons.org/licenses/by-nc-nd/4.0/.
References
- Harding E. WHO global progress report on tuberculosis elimination. Lancet Respir Med 2020;8:19. [Crossref] [PubMed]
- Takwoingi Y, Whitworth H, Rees-Roberts M, et al. Interferon gamma release assays for Diagnostic Evaluation of Active tuberculosis (IDEA): test accuracy study and economic evaluation. Health Technol Assess 2019;23:1-152. [Crossref] [PubMed]
- Tan Y, Zhang ST, Wei JW, et al. A radiomics nomogram may improve the prediction of IDH genotype for astrocytoma before surgery. Eur Radiol 2019;29:3325-37. [Crossref] [PubMed]
- Park CJ, Choi YS, Park YW, et al. Diffusion tensor imaging radiomics in lower-grade glioma: improving subtyping of isocitrate dehydrogenase mutation status. Neuroradiology 2020;62:319-26. [Crossref] [PubMed]
- Cong M, Feng H, Ren JL, et al. Development of a predictive radiomics model for lymph node metastases in pre-surgical CT-based stage IA non-small cell lung cancer. Lung Cancer 2020;139:73-9. [Crossref] [PubMed]
- Ravanelli M, Farina D, Morassi M, et al. Texture analysis of advanced non-small cell lung cancer (NSCLC) on contrast-enhanced computed tomography: prediction of the response to the first-line chemotherapy. Eur Radiol 2013;23:3450-5. [Crossref] [PubMed]
- Dennie C, Thornhill R, Sethi-Virmani V, et al. Role of quantitative computed tomography texture analysis in the differentiation of primary lung cancer and granulomatous nodules. Quant Imaging Med Surg 2016;6:6-15. [PubMed]
- Chen X, Feng B, Chen Y, et al. A CT-based radiomics nomogram for prediction of lung adenocarcinomas and granulomatous lesions in patient with solitary sub-centimeter solid nodules. Cancer Imaging 2020;20:45. [Crossref] [PubMed]
- Li BG, Ma DQ, Xian ZY, et al. The value of multislice spiral CT features of cavitary walls in differentiating between peripheral lung cancer cavities and single pulmonary tuberculous thick-walled cavities. Br J Radiol 2012;85:147-52. [Crossref] [PubMed]
- Wang XL, Shan W. Application of dynamic CT to identify lung cancer, pulmonary tuberculosis, and pulmonary inflammatory pseudotumor. Eur Rev Med Pharmacol Sci 2017;21:4804-9. [PubMed]
- Wu L, Cao G, Zhao L, et al. Spectral CT Analysis of Solitary Pulmonary Nodules for Differentiating Malignancy from Benignancy: The Value of Iodine Concentration Spatial Distribution Difference. Biomed Res Int 2018;2018:4830659. [Crossref] [PubMed]
- Zeng J, Liu Z, Shen G, et al. MRI evaluation of pulmonary lesions and lung tissue changes induced by tuberculosis. Int J Infect Dis 2019;82:138-46. [Crossref] [PubMed]
- Yang X, He J, Wang J, et al. CT-based radiomics signature for differentiating solitary granulomatous nodules from solid lung adenocarcinoma. Lung Cancer 2018;125:109-14. [Crossref] [PubMed]
- Chen S, Harmon S, Perk T, et al. Using neighborhood gray tone difference matrix texture features on dual time point PET/CT images to differentiate malignant from benign FDG-avid solitary pulmonary nodules. Cancer Imaging 2019;19:56. [Crossref] [PubMed]
- Suo S, Cheng J, Cao M, et al. Assessment of Heterogeneity Difference Between Edge and Core by Using Texture Analysis: Differentiation of Malignant From Inflammatory Pulmonary Nodules and Masses. Acad Radiol 2016;23:1115-22. [Crossref] [PubMed]
- Beig N, Khorrami M, Alilou M, et al. Perinodular and Intranodular Radiomic Features on Lung CT Images Distinguish Adenocarcinomas from Granulomas. Radiology 2019;290:783-92. [Crossref] [PubMed]
- Feng B, Chen X, Chen Y, et al. Radiomics nomogram for preoperative differentiation of lung tuberculoma from adenocarcinoma in solitary pulmonary solid nodule. Eur J Radiol 2020;128:109022. [Crossref] [PubMed]
- Cui EN, Yu T, Shang SJ, et al. Radiomics model for distinguishing tuberculosis and lung cancer on computed tomography scans. World J Clin Cases 2020;8:5203-12. [Crossref] [PubMed]
- Yu Y, Wang X, Shi C, et al. Spectral Computed Tomography Imaging in the Differential Diagnosis of Lung Cancer and Inflammatory Myofibroblastic Tumor. J Comput Assist Tomogr 2019;43:338-44. [Crossref] [PubMed]
- Xing Z, Ding W, Zhang S, et al. Machine Learning-Based Differentiation of Nontuberculous Mycobacteria Lung Disease and Pulmonary Tuberculosis Using CT Images. Biomed Res Int 2020;2020:6287545. [Crossref] [PubMed]
(English Language Editor: J. Reynolds)