The role of TRIM51 as a multipurpose biomarker in melanoma
Introduction
Advances in emerging high-throughput technologies, including next-generation sequencing (NGS), have led to tremendous yields of multiomics data, including genome, transcriptome, epigenome, and proteome (1). Since comprehensive multiomics-driven molecular landscapes reflect the origin of an individual’s pathogenesis, deciphering multiomics data is a prerequisite for the implementation of precision medicine (2,3). In the cancer field, multiomics data have been accumulated from thousands of cancer cell lines (CCLs) [e.g., Cancer Cell Line Encyclopedia (CCLE)], which can promote significant research with clinical implications (4). Moreover, when therapeutic response data derived from CCLs are integrated with multiomics data, it can facilitate the functional interpretation of drug targets, investigation of unknown mechanism of action (MoA), and discovery of predictive biomarkers (5,6).
Systematic pharmacogenomics/pharmacotranscriptomic research has characterized the MoAs of small molecules and suggested novel therapeutic hypotheses that support the discovery of patient-matched drugs and predictive biomarkers. Iorio et al. uncovered clinically relevant oncogenic alterations across 1,001 CCLs that predict differential sensitivity to 265 anticancer drugs (7). The Genomics of Drug Sensitivity in Cancer (GDSC) has identified therapeutic genomic biomarkers that are associated with responses to 130 drugs under clinical and preclinical investigations (8). The Cancer Therapeutics Response Portal (CTRP) quantitatively measured the sensitivity profile of 860 CCLs to 481 small molecules and correlated chemical sensitivity to basal gene expression, revealing cellular targets and MoAs of small molecules (9).
A recent study showed that genome-wide cancer dependency is explained as much as 82% by gene expression level (10). Based on the finding, we hypothesized that transcriptome-based approaches can provide opportunities to identify molecular determinants that predict drug responses. Therefore, the aim of this study was to identify transcriptome-based biomarkers associated with drug sensitivity in cancer. To that aim, we carried out a comprehensive integrative analysis of multiple independent datasets to examine the pharmacotranscriptomic characteristics of genes associated with drug sensitivity. As a result, we found a novel relationship between TRIM51 expression levels and sensitivity to multiple BRAF-MEK inhibitors. Furthermore, we demonstrate that TRIM51 mRNA level may be used as a multiuse biomarker to determine the pharmacological activity of BRAF-MEK inhibitors and the prognosis of melanoma patients. We present the following article in accordance with the REMARK reporting checklist (available at https://dx.doi.org/10.21037/tcr-21-1102).
Methods
Data analysis
In this study, we utilized several databases as follows: the CCLE for multiomics data analysis of CCLs, the Cancer Therapeutics Response Portal (CTRP) and Profiling Relative Inhibition Simultaneously in Mixtures (PRISM) for drug sensitivity profile data of CCLs, and The Cancer Genome Atlas (TCGA) and cBioPortal (11,12) for clinical data of melanoma patients. The CTRP data quantifies the effect of each of 481 compounds by calculating the area under a 16-point concentration-response curve (AUC) across 827 CCLs. The PRISM data estimates fold-changes (log2) of chemical-perturbed viability of pooled 578 CCLs relative to negative control at a single concentration. To identify genes associated with drug sensitivity, we examined the Pearson’s correlation coefficient between log2-transformed gene expression levels (from the CCLE data) and AUCs (from the CTRP data) or sensitivity fold-changes (from the PRISM data).
In addition, we used multiple GEO datasets to examine the mRNA level of TRIM51 upon treatment with BRAF-MEK inhibitors and according to the melanoma disease status: (I) GSE127988 (13): transcriptomes of A375 melanoma cells treated with 7 doses of vemurafenib (0.001–10 uM) for 24 h. (II) GSE127948 (14): transcriptomes of 92.1 uveal melanoma cells treated with trametinib (10 nM) for 24 h. (III) GSE33655 (15): transcriptomes of uveal melanoma cells treated with selumetinib for 8 h. (IV) GSE65185 (16): transcriptomes of melanoma tumors (n=19) biopsied before therapy (vemurafenib or dabrafenib) and during disease progression. (V) GSE99898 (17): transcriptomes of melanoma tumors biopsied before (n=17) and after (n=8) therapy (vemurafenib, dabrafenib, or dabrafenib + trametinib) and during disease progression (n=13). (VI) GSE15605 (18): transcriptomes of normal skin (n=16), primary (n=46), and metastatic melanoma (n=12). (VII) GSE112509 (19): transcriptomes of microdissected melanocytic nevi (n=23) and primary melanomas (n=57). (VIII) GSE83583 (20): transcriptomes of melanocytes (n=3) and primary melanomas (n=32). (IX) GSE12391 (21): transcriptomes of common melanocytic nevi (n=18), primary radial growth phase melanomas (n=8), primary vertical growth phase melanomas (n=15), and melanoma metastases (n=5). The study was conducted in accordance with the Declaration of Helsinki (as revised in 2013).
Statistical analysis
We used GraphPad Prism (version 9.0; GraphPad Software, San Diego, CA, USA) to create the figures and perform statistical analyses. We performed a t-test to compare the means between two groups and calculated the Pearson’s correlation coefficient to assess correlated associations. For Kaplan-Meier survival analysis, patients were classified as TRIM51-high and TRIM51-low based on z-score (≥2) or median expression level and statistical significance between the two groups was examined by the log-rank test. Statistical significance was set at P<0.05.
Results
Drug response score (DRS)
To assess the pharmacotranscriptomic characteristics of genes, we analyzed well-defined CTRP data, which included the sensitivity profiles of 860 CCLs to 481 small molecules. To quantitatively measure the relative transcriptional propensity of each gene toward resistance or sensitivity to the total of 481 small molecules examined, we adopted a scoring metric, namely DRS, which evaluates each gene on the basis of correlations between gene expression and drug sensitivity.
X: an absolute value of correlation coefficient X.
NT: total number of drugs examined (481 drugs in the CTRP data).
NR: the number of drugs with a positive correlation greater than X between the expression level of “gene A” and drug sensitivity.
NS: the number of drugs with a negative correlation lower than −X between the expression level of “gene A” and drug sensitivity.
For example, “DRS(0.3)_ gene A” is the number of drugs that have a correlation >0.3 between the expression level of “gene A” and drug sensitivity minus the number of drugs that have a correlation <−0.3 between the expression level of “gene A” and drug sensitivity, divided by the 481 drugs. Accordingly, a given gene with high DRS can be classified as a predominant resistance-associated gene against the 481 small molecules, whereas a gene with low DRS can be classified as a predominant sensitivity-associated gene. According to the ordered DRS, a gene with a relatively high DRS may have a high probability of being resistant to most small molecules examined, irrespective of developmental status (e.g., FDA approval, clinical investigation, and molecular probe) and target class (e.g., kinases) (Figure 1A,1B).
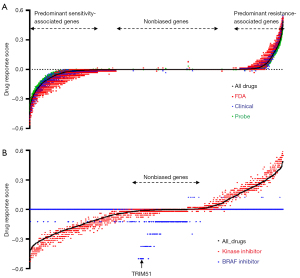
In addition to the broad propensity of genes toward sensitivity or resistance, we focused on DRS to selective inhibitors that target specific proteins. As shown in Figure 1A, genes with high DRS to most small molecules also showed high DRS to selective inhibitors against PI3Kβ, ALK, or MET (Figure S1). However, the DRS to BRAF inhibitors displayed a different behavior; genes with high DRS to BRAF inhibitors were not biased toward resistance or sensitivity to most drugs (Figure 1B). This suggests a high probability of identifying BRAF inhibitor-specific sensitivity-associated genes.
The expression level of TRIM51 gene is associated with sensitivity to BRAF-MEK inhibitors
Among the genes with high DRS to BRAF inhibitors (Figure 1B), we focused on the novel gene TRIM51 due to its exclusive highest expression in CCLs derived from melanoma, for which BRAF inhibitors are primarily indicated (Figure 2). In 201 CCLs with TRIM51 transcript detection, elevated TRIM51 levels showed a clear association with enhanced efficacy of multiple BRAF and MEK inhibitors (Figure 3A), as indicated by the decreased AUC.
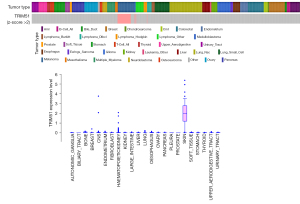
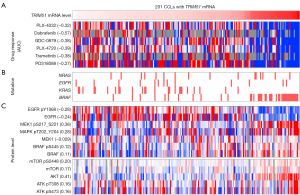
BRAF inhibitors (e.g., vemurafenib and dabrafenib) and MEK inhibitors (e.g., trametinib) have been approved for the treatment of metastatic melanoma harboring BRAF mutations (22). TRIM51-high CCLs in which BRAF-MEK inhibitors were highly efficacious (Figure 3A) co-occurred with a relatively high enrichment of BRAF mutations (Figure 3B) and increased phosphorylation levels of BRAF, MEK, and ERK1/2 (Figure 3C). Therefore, to rule out the possibility that the high efficacy could be attributed to the dependency of BRAF mutations, we examined the association of TRIM51 expression levels with sensitivity to BRAF-MEK inhibitors using the CCLs restricted to BRAF-mutant melanoma. This analysis showed a strong correlation between the expression level of TRIM51 and sensitivity to multiple BRAF (e.g., dabrafenib and GDC-0879) and MEK inhibitors (e.g., PD318088 and trametinib) (Figure 4A).
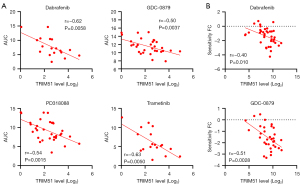
Then, we validated the result using an independent dataset from the PRISM, which examined the sensitivity profiles of 4,518 drugs across 578 CCLs. This independent analysis also revealed a significant correlation between the expression level of TRIM51 and sensitivity to BRAF inhibitors in BRAF-mutant melanoma (Figure 4B). Accordingly, these results suggest that the expression level of TRIM51 can be utilized for the detailed classification of responders to BRAF-MEK inhibitors, even in BRAF-mutant melanoma patients.
The expression level of TRIM51 gene is associated with the pharmacoclinical activity of BRAF-MEK inhibitors
The fact that the basal expression level of TRIM51 was associated with sensitivity to multiple inhibitors against both BRAF and MEK (Figures 3,4) indicated that the expression level of TRIM51 may be involved in the BRAF-MEK signaling pathway. Supporting this hypothesis, treatment with the BRAF inhibitor vemurafenib dramatically decreased the expression level of TRIM51 in a dose-dependent manner in the melanoma CCL, A375, even at concentrations lower than 100 nM (Figure 5A). Similarly, treatment with the MEK inhibitors, trametinib or selumetinib, significantly reduced the expression level of TRIM51 in various uveal melanoma CCLs (Figure 5B,5C). These data revealed that the BRAF-MEK pathway may positively regulate the mRNA levels of TRIM51 in melanoma.
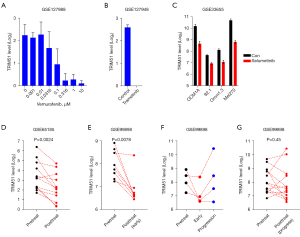
To demonstrate the clinical relevance of the BRAF-MEK pathway-dependent transcriptional regulation of TRIM51, we compared the mRNA levels of TRIM51 between paired melanoma biopsies before and after treatment with BRAF inhibitors. Intriguingly, this analysis revealed that treatment consistently reduced the expression level of TRIM51 in 11 out of 12 patients examined (Figure 5D). Similarly, early treatment with BRAF-MEK inhibitors (vemurafenib, dabrafenib, or combination therapy with dabrafenib and trametinib) also reliably decreased the expression level of TRIM51 in six out of eight paired melanoma biopsy samples examined independently (Figure 5E). Importantly, the mRNA levels of TRIM51 were restored or even increased in three out of four paired melanoma biopsies after disease progression on the inhibitors (Figure 5F). After progression, the mRNA level of TRIM51 was increased in three patients, not changed in four patients, and decreased in six patients among 13 paired samples (Figure 5G). Collectively, these results demonstrated the positive regulation of TRIM51 mRNA levels by the BRAF-MEK pathway and suggested that the expression level of TRIM51 can faithfully represent the degree of post-treatment activity and resistance to BRAF-MEK inhibitors both in vitro and in clinical situations.
The expression level of TRIM51 gene is associated with patient survival and immuno-resistance in melanoma
We then assessed whether the expression level of TRIM51 was associated with disease progression and prognosis in melanoma. The datasets from independent studies commonly showed that the expression level of TRIM51 is upregulated during disease progression; the mRNA level of TRIM51 was significantly increased in both primary and metastatic melanomas compared with normal nevi (Figure 6A). Strikingly, the upregulation of TRIM51 mRNA level was significantly associated with poor survival in both cutaneous and uveal melanoma; TRIM51-high patients showed lower survival rates than TRIM51-low patients in terms of both overall and progression-free survival (Figure 6B,6C).
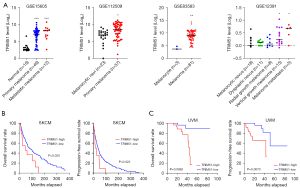
To rule out the possibility that the significant prognostic effect of TRIM51 is attributed to a standard prognostic variable, cancer staging, we assessed the mRNA levels of TRIM51 according to the American Joint Committee on Cancer (AJCC) cancer staging system. Indeed, although cancer staging was significantly associated with patient survival in cutaneous melanoma, the TRIM51 mRNA levels were not significantly changed with cancer staging (Figure S2A) as well as metastasis subgroups (Figure S2B). However, cancer staging was not tightly associated with survival of patients in uveal melanoma (Figure S2C). In addition, the TRIM51 mRNA levels were comparable according to the absence or presence of ulceration as another prognostic feature (Figure S2D). These results indicate that the expression level of TRIM51 may be a valid prognostic marker independent of several prognostic features in melanoma.
Next, we hypothesized that the lower survival rates of TRIM51-high patients may be associated with worse tumor microenvironment, especially immune resistance. In addition, the importance of immunotherapy for melanoma prompted us to explore the association between the expression level of TRIM51 and immuno-resistance related features. Indeed, TRIM51-high patients showed reduced expression levels of genes encoding granzymes (Figure 7A), T cell effector molecules (23). Moreover, TRIM51-high patients also presented the low mRNA level of LCK (Figure 7A), a T-cell predominant marker (24), suggesting low infiltration of T cells. Supporting the notion, gene set enrichment analysis (GSEA) revealed that TRIM51-high patients tended to have the low level of a CD8 T cell signature (Figure 7B). Furthermore, the expression pattern of TRIM51-high patients showed a transcriptional skewing toward resistance to nivolumab (Figure 7B). These results suggest that TRIM51-high patients may have a high probability of being resistant to immunotherapy and their lower survival rates may be, in part, attributed to the immuno-resistance hallmarks.
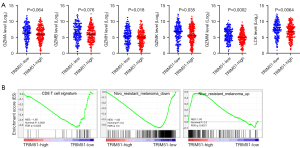
Discussion
Given that genome-wide cancer dependency is explained as much as 82% by transcriptomes (10), pharmacotranscriptomic analyses can provide great opportunities to identify molecular determinants that can predict drug responses. In this study, we carried out a comprehensive integrative analysis of multiple datasets to examine the pharmacotranscriptomic characteristics of genes associated with drug sensitivity in cancer. As a result, we identified a novel gene, TRIM51, as a biomarker for melanoma. First, it can be used as a predictive biomarker to predict drug sensitivity against BRAF-MEK inhibitors, based on the positive association of its expression level with sensitivity to multiple BRAF-MEK inhibitors. Specifically, since the predictive association was preserved even when the analysis was limited to melanoma CCLs harboring the previously known biomarker BRAF V600 mutation, the expression level of TRIM51, as an additive covariate, may reinforce the predictive power of BRAF V600 mutations to select patients for whom BRAF-MEK inhibitor treatment will be most beneficial. Although these findings were validated using multiple independent cell line-based datasets, validations based on clinical data or functional approaches have not yet been performed. Therefore, functional validations through loss-of-function or gain-of-function studies of TRIM51 are required.
Second, the expression level of TRIM51 can be utilized as a surrogate marker to determine the post-treatment activity of BRAF-MEK inhibitors. As shown in Figure 5, even low doses and short treatment durations were sufficient to decrease the expression level of TRIM51 both in vitro and in clinical cases from multiple independent studies. Thus, the expression level of TRIM51 may be sensitive and consistent enough to represent the pharmacological activity of BRAF-MEK inhibitors and resistance against the inhibitors. Lastly, the expression level of TRIM51 may be a clinically relevant prognostic marker in cutaneous and uveal melanoma. In particular, the use of TRIM51 as a biomarker in uveal melanoma, a relatively rare but fatal cancer that lacks biomarkers (25), would be of great clinical significance.
Although many biomarkers are utilized merely as predictors irrespective of their biological functions, several circumstantial evidences suggest that TRIM51 may have a functional link with the BRAF-MEK-MAPK signaling pathway in melanoma: (I) the predominant mRNA expression of TRIM51 in melanoma CCLs; (II) the transcriptional suppression of TRIM51 by both BRAF and MEK inhibitors; (III) the discovery of SPRY2, a negative regulator of the BRAF-MEK-MAPK pathway (26), as a putative binding partner of TRIM51 by a high-throughput yeast two-hybrid system (Figure S3A) (27); (IV) the expression correlation between SPRY2 and TRIM51 in melanoma (Figure S3B), and (V) the similar subcellular localization pattern of both proteins (Figure S3C) (28). Thus, the functional roles of TRIM51 should be further investigated to determine whether TRIM51 is regulated by the BRAF-MEK pathway at the transcriptional level or whether TRIM51 can actively regulate the pathway in melanoma.
In conclusion, the development of TRIM51 mRNA level as a biomarker may be effective in three aspects: patient selection for treatment with BRAF-MEK inhibitors in melanoma, determination of the pharmacological activity of BRAF-MEK inhibitors under development, and determination of prognosis after disease diagnosis in melanoma. Our study is a good example that presents the potential for implementation of precision medicine simplified by a single multiuse biomarker. Simultaneously, our research strategy based on the reanalysis of public data will motivate the development of other clinically relevant biomarkers.
Acknowledgments
Funding: This study was supported by grants from
Footnote
Reporting Checklist: The author has completed the REMARK reporting checklist. Available at https://dx.doi.org/10.21037/tcr-21-1102
Conflicts of Interest: The author has completed the ICMJE uniform disclosure form (available at https://dx.doi.org/10.21037/tcr-21-1102). The author reports that this study was supported by grants from the Korea Research Institute of Chemical Technology (KK2131-30). The author has no other conflicts of interest to declare.
Ethical Statement: The author is accountable for all aspects of the work in ensuring that questions related to the accuracy or integrity of any part of the work are appropriately investigated and resolved. The study was conducted in accordance with the Declaration of Helsinki (as revised in 2013). Institutional ethical approval and informed consent were waived.
Open Access Statement: This is an Open Access article distributed in accordance with the Creative Commons Attribution-NonCommercial-NoDerivs 4.0 International License (CC BY-NC-ND 4.0), which permits the non-commercial replication and distribution of the article with the strict proviso that no changes or edits are made and the original work is properly cited (including links to both the formal publication through the relevant DOI and the license). See: https://creativecommons.org/licenses/by-nc-nd/4.0/.
References
- Subramanian I, Verma S, Kumar S, et al. Multi-omics Data Integration, Interpretation, and Its Application. Bioinform Biol Insights 2020;14:1177932219899051. [Crossref] [PubMed]
- Mun J, Choi G, Lim B. A guide for bioinformaticians: 'omics-based drug discovery for precision oncology. Drug Discov Today 2020; Epub ahead of print. [Crossref] [PubMed]
- Pavlovic S, Kotur N, Stankovic B, et al. Pharmacogenomic and Pharmacotranscriptomic Profiling of Childhood Acute Lymphoblastic Leukemia: Paving the Way to Personalized Treatment. Genes (Basel) 2019;10:191. [Crossref] [PubMed]
- Ghandi M, Huang FW, Jané-Valbuena J, et al. Next-generation characterization of the Cancer Cell Line Encyclopedia. Nature 2019;569:503-8. [Crossref] [PubMed]
- Chiu YC, Chen HH, Gorthi A, et al. Deep learning of pharmacogenomics resources: moving towards precision oncology. Brief Bioinform 2020;21:2066-83. [Crossref] [PubMed]
- Barretina J, Caponigro G, Stransky N, et al. The Cancer Cell Line Encyclopedia enables predictive modelling of anticancer drug sensitivity. Nature 2012;483:603-7. [Crossref] [PubMed]
- Iorio F, Knijnenburg TA, Vis DJ, et al. A Landscape of Pharmacogenomic Interactions in Cancer. Cell 2016;166:740-54. [Crossref] [PubMed]
- Garnett MJ, Edelman EJ, Heidorn SJ, et al. Systematic identification of genomic markers of drug sensitivity in cancer cells. Nature 2012;483:570-5. [Crossref] [PubMed]
- Rees MG, Seashore-Ludlow B, Cheah JH, et al. Correlating chemical sensitivity and basal gene expression reveals mechanism of action. Nat Chem Biol 2016;12:109-16. [Crossref] [PubMed]
- Tsherniak A, Vazquez F, Montgomery PG, et al. Defining a Cancer Dependency Map. Cell 2017;170:564-576.e16. [Crossref] [PubMed]
- Gao J, Aksoy BA, Dogrusoz U, et al. Integrative analysis of complex cancer genomics and clinical profiles using the cBioPortal. Sci Signal 2013;6:pl1. [Crossref] [PubMed]
- Cerami E, Gao J, Dogrusoz U, et al. The cBio cancer genomics portal: an open platform for exploring multidimensional cancer genomics data. Cancer Discov 2012;2:401-4. [Crossref] [PubMed]
- Gerosa L, Chidley C, Fröhlich F, et al. Receptor-Driven ERK Pulses Reconfigure MAPK Signaling and Enable Persistence of Drug-Adapted BRAF-Mutant Melanoma Cells. Cell Syst 2020;11:478-494.e9. [Crossref] [PubMed]
- Faião-Flores F, Emmons MF, Durante MA, et al. HDAC Inhibition Enhances the In Vivo Efficacy of MEK Inhibitor Therapy in Uveal Melanoma. Clin Cancer Res 2019;25:5686-701. [Crossref] [PubMed]
- Ambrosini G, Pratilas CA, Qin LX, et al. Identification of unique MEK-dependent genes in GNAQ mutant uveal melanoma involved in cell growth, tumor cell invasion, and MEK resistance. Clin Cancer Res 2012;18:3552-61. [Crossref] [PubMed]
- Hugo W, Shi H, Sun L, et al. Non-genomic and Immune Evolution of Melanoma Acquiring MAPKi Resistance. Cell 2015;162:1271-85. [Crossref] [PubMed]
- Kakavand H, Rawson RV, Pupo GM, et al. PD-L1 Expression and Immune Escape in Melanoma Resistance to MAPK Inhibitors. Clin Cancer Res 2017;23:6054-61. [Crossref] [PubMed]
- Raskin L, Fullen DR, Giordano TJ, et al. Transcriptome profiling identifies HMGA2 as a biomarker of melanoma progression and prognosis. J Invest Dermatol 2013;133:2585-92. [Crossref] [PubMed]
- Kunz M, Löffler-Wirth H, Dannemann M, et al. RNA-seq analysis identifies different transcriptomic types and developmental trajectories of primary melanomas. Oncogene 2018;37:6136-51. [Crossref] [PubMed]
- Eskiocak U, Ramesh V, Gill JG, et al. Synergistic effects of ion transporter and MAP kinase pathway inhibitors in melanoma. Nat Commun 2016;7:12336. [Crossref] [PubMed]
- Scatolini M, Grand MM, Grosso E, et al. Altered molecular pathways in melanocytic lesions. Int J Cancer 2010;126:1869-81. [Crossref] [PubMed]
- Sanchez JN, Wang T, Cohen MS. BRAF and MEK Inhibitors: Use and Resistance in BRAF-Mutated Cancers. Drugs 2018;78:549-66. [Crossref] [PubMed]
- Sandberg JK, Fast NM, Nixon DF. Functional heterogeneity of cytokines and cytolytic effector molecules in human CD8+ T lymphocytes. J Immunol 2001;167:181-7. [Crossref] [PubMed]
- Peng W, Chen JQ, Liu C, et al. Loss of PTEN Promotes Resistance to T Cell-Mediated Immunotherapy. Cancer Discov 2016;6:202-16. [Crossref] [PubMed]
- Kaliki S, Shields CL. Uveal melanoma: relatively rare but deadly cancer. Eye (Lond) 2017;31:241-57. [Crossref] [PubMed]
- Tsavachidou D, Coleman ML, Athanasiadis G, et al. SPRY2 is an inhibitor of the ras/extracellular signal-regulated kinase pathway in melanocytes and melanoma cells with wild-type BRAF but not with the V599E mutant. Cancer Res 2004;64:5556-9. [Crossref] [PubMed]
- Rual JF, Venkatesan K, Hao T, et al. Towards a proteome-scale map of the human protein-protein interaction network. Nature 2005;437:1173-8. [Crossref] [PubMed]
- Uhlén M, Fagerberg L, Hallström BM, et al. Proteomics. Tissue-based map of the human proteome. Science 2015;347:1260419. [Crossref] [PubMed]