Systematic analysis of the long noncoding RNA (lncRNA)-miRNA-mRNA competing endogenous RNA network to identify prognostic biomarkers and the potential regulatory axis in glioblastoma multiforme
Introduction
Glioblastoma (GBM), an intracranial brain tumor with a high final lethality rate and recurrence rate, maintains a poor prognosis because of the heterogeneity of its molecular and cellular profile and limited available therapies (1). At present, the GBM therapy is still limited to surgery, radiotherapy, and chemotherapy, and the 5-year survival rate is only 5.5% (2). Thus, it is still a challenge to treat this aggressive cancer. Therefore, it is crucial to uncover the underlying genetic mechanisms and identify prognostic markers of GBM to develop novel therapies.
“Competing endogenous RNA (ceRNA)”, a hypothesis intended to reveal novel interaction mechanisms between RNAs, supposedly involves competitive binding with shared microRNAs (miRNAs) to regulate downstream gene expression (3). MiRNAs, one class of small noncoding RNAs, can binding to miRNA response elements (MREs) on their target transcripts to regulate gene expression (4), thus fulfilling key roles in various diseases, including cancer (5). In recent years, large-scale studies have reported that dysregulation of ceRNAs may be involved in the pathogenesis of many cancers, such as colorectal cancer (6), and breast cancer (7). As one type of the noncoding RNAs, long noncoding RNA (lncRNA), with over 200-nucleotide length, has many structural features of mRNAs but lacks the ability to encode proteins (8,9). The lncRNA-related ceRNA network is the most commonly studied ceRNA thus far, and it has been constructed in various types of cancers, including squamous cell carcinoma (10), papillary thyroid cancer (11), and cholangiocarcinoma (12).
Such networks also have been established in GBM (13,14), and many lncRNAs have been identified to be of great clinical significance in the diagnosis and prognostication of GBM (15). For instance, HOX transcript antisense intergenic RNA (HOTAIR) is overexpressed in GBM and controls the proliferation of GBM cell (16). Moreover, during treatment of GBM patients, we can detect serum HOTAIR levels to help determine treatment response and relapse status (17). As an oncogenic lncRNA, AGAP2 antisense RNA1 (AGAP2-AS1) was related to many biological processes of GBM, such as migration, invasion and proliferation. And it is also suggested to be a prognostic biomarker and potential therapeutic target for patients with GBM (18). Based on comprehensive analysis of the functional miRNA-mRNA regulatory network in the previous studies, key hub miRNAs closely related to the GBM tumors progression were recognized (19). However, there has been limited progress in systematically understanding the underlying biological mechanisms of the ceRNA network in GBM. Therefore, more efforts should be made to explore the correlation between lncRNAs, miRNAs and mRNAs in GBM tumorigenesis and identify more potential prognostic biomarkers for GBM based on large-sample high-throughput transcriptome sequencing data.
In this study, using RNA sequence profiling data of GBM from TCGA and miRNA data from GEO database, a ceRNA network was constructed after identifying differentially expressed genes (DEGs). Furthermore, functional enrichment analysis was used to explore significant pathways associated with key genes, and univariate Cox regression followed by multivariate Cox regression was performed to validate independent factors and construct risk prediction models for GBM. Eventually, we integrated a lncRNA-miRNA-mRNA axis that was closely linked to the pathogenesis of GBM and confirmed feature sets of these core genes. Our current study will provide a novel perspective for understanding the biological mechanisms of GBM and contribute to further analysis of candidate therapeutic targets in GBM. We present the following article in accordance with the MDAR reporting checklist (available at https://dx.doi.org/10.21037/tcr-21-1162).
Methods
Data collection and preprocessing
This study was conducted as shown in the flowchart in Figure 1. The RNA-Seq row data and corresponding clinical information for cases were obtained from TCGA (https://cancergenome.nih.gov/). Due to the lack of normal controls for GBM in TCGA, a matrix file of the GSE25631 dataset containing miRNA-Seq information for GBM based on GPL8179 Illumina Human v2 MicroRNA expression beadchip from GEO database (https://www.ncbi.nlm.nih.gov/geo/) was downloaded. Afterward, data downloaded from TCGA, including 169 GBM and 5 nontumoral brain samples, were used for differentially expressed mRNAs (DEmRNAs) and differentially expressed lncRNAs (DElncRNAs) analyses. The GSE25631 dataset containing miRNA microarray data of 82 GBM tissues and 5 normal brain tissues was used to identify differentially expressed miRNAs (DEmiRNAs). RNA-Seq data were annotated based on the human whole genome (GRCh38.97), provided by the GENCODE database (https://www.gencodegenes.org).
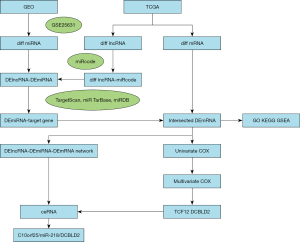
Differential expression analysis
DElncRNAs, DEmiRNAs and DEmRNAs between the GBM samples and controls were identified using the “edgeR” package (http://www.bioconductor.org/packages/release/bioc/html/edgeR.html). Fold change (FC) ≥2 and adjusted P (p.adj) <0.01 were identified to be statistically significant. Afterward, the R packages “gplots” and “pheatmap” were used in R software (version 4.0.2) to visualize differentially expressed RNAs (DERNAs) (including DEmRNAs, DElncRNAs, and DEmiRNAs).
CeRNA network construction
Based on previously obtained DERNAs, a ceRNA network comprising lncRNAs, miRNAs and mRNAs was constructed. Key DERNAs among the ceRNA network were reidentified as described in the flowchart in Figure 1. First, we predicted the miRNAs that interact with DElncRNAs through the miRcode database (http://www.mircode.org/index.php). Then, we took the intersection of predicted miRNAs and DEmiRNAs to retain the DEmiRNAs interacting with DElncRNAs for the next analysis. DElncRNA-DEmiRNA was identified in this step. Second, MiRTarBase, TargetScan, and miRDB were employed to predict target genes of DEmiRNAs retained above. Next, we took the interaction of the predicted target genes and 2,537 DEmRNAs, and 56 DEmRNAs were retained. DEmiRNA-DEmRNA was identified in this step. Finally, we retained the data of 188 DElncRNAs, 8 DEmiRNAs and 56 DEmRNAs as well as their interaction pairs for further analysis, and then a ceRNA network visualized by Cytoscape (vision 3.7.2) was generated for the analysis of interactive lncRNA-miRNA-mRNA strings.
Function and pathway analysis of DEmRNAs
The online tool Database for Annotation, Visualization and Integrated Discovery (DAVID) (https://david.ncifcrf.gov/) was downloaded to perform Gene Ontology (GO) analysis of all DEmRNAs in the ceRNA network. To display the details of the potential functions and pathways of key genes in GBM, bar plots and GO plots were depicted by the “GOplot” and “Barplot” R packages (version 4.0.2). To further identify signaling pathways associated with DEmRNAs, we used the “clusterProfiler” package to perform KEGG analysis with the significance level at an adjusted P value (p.adj) <0.01.
Construction of risk prediction model and survival analysis
To verify the correlation between key DElncRNAs or DEmRNAs in the aforementioned ceRNA network and the survival outcomes of GBM patients based on the clinical information downloaded from TCGA, we first performed univariate Cox survival regression analysis with the R language “survival” package to obtain prognosis-related factors. Then, the molecules with a P value <0.1 in the results were included in multivariate Cox regression analysis. Second, coefficients in multivariate Cox regression were used as coefficients of corresponding factors in the risk prediction model. Next, according to the median value, we divided all patients into two groups with low-risk and high-risk and depicted the survival status and risk score of each patient. Third, we performed the Kaplan-Meier analysis to identify the prognostic value of our risk model obtained above, and a log-rank P value <0.05 was considered significant. Finally, according to the area under the curve (AUC) value of the receiver operating characteristic (ROC) curve analysed using the R package “survivalROC”, we tested the accuracy of the model. During this process of model building, we depicted the Kaplan-Meier curve for each independent prognostic factor using the R package “survival” to confirm DERNAs that were significantly associated with the prognosis of GBM (P value <0.05). All statistical analyses in this study were conducted with R software (version 4.0.2).
Gene Set Enrichment Analysis (GSEA) of single genes
To perform GSEA of a single gene, all GBM transcriptional data in the TCGA database were first divided into high and low expression groups according to the median expression of the gene. Then, GSEA was conducted based on the GO database (c5.all.v7.1.symbols.gmt) using GSEA 4.1.0.jar (number of simulations=1000, false discover rate (FDR) <0.25). The study was conducted in accordance with the Declaration of Helsinki (as revised in 2013).
Statistical analysis
We have described bioinformatic analyses of databases in detail in the above method. R software (version 4.0.2) was used for data analysis and drawing. Differences between groups were compared using a two-sided Student’s t-test. The Kaplan-Meier method was performed to plot survival curves compared using the log-rank test. The level of P<0.05 was assessed as significance. Significance was denoted as *, P<0.05; **, P<0.01; ***, P<0.001.
Results
Identification of the differential expression of mRNA, lncRNA, and miRNA
According to the cutoff value described in the methods, we identified 1,668 upregulated mRNAs, 1,872 downregulated mRNAs, 869 upregulated lncRNAs, and 1,297 downregulated lncRNAs from the TCGA database in GBM. Additionally, 2 upregulated miRNAs and 64 downregulated miRNAs were found in tumor samples compared with controls based on the GSE25631 dataset. Afterward, for the obtained differential expression of RNAs, two volcano maps and a heatmap were depicted by “gplots” and “pheatmap” in the R platform, respectively (Figure 2A-2C).
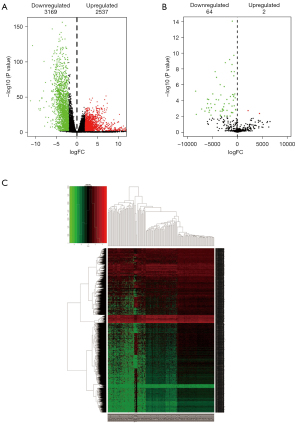
Construction of the ceRNA network in GBM
To elucidate the potential regulatory mechanism of DERNAs in GBM, a ceRNA network based on the obtained DERNAs was established. However, our DEmiRNAs and DElncRNAs/DEmRNAs were identified from two different databases. DERNAs for the ceRNA network were reidentified based on DERNAs identified from the TCGA and GEO databases as described in the flowchart in Figure 1, and the detailed selection criteria are described in the methodology section. Finally, a total of 56 DEmRNAs, 188 DElncRNAs and 8 DEmiRNAs identified in the TCGA and GEO databases were included to construct the ceRNA network using Cytoscape, which included 410 lncRNA-miRNA interactions and 56 miRNA-mRNA interactions, as shown in Figure 3.
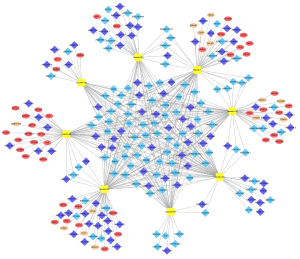
GO and KEGG functional enrichment analysis
To further elucidate the potential biological functions and major signaling pathways of key genes in the ceRNA network, 56 DEmRNAs were subjected to GO function and KEGG pathway analysis. Based on the annotation file downloaded from DAVID, the top 5 GO terms, including protein binding, translation regulator activity, mRNA 5’-UTR binding, G2/M transition of mitotic cell cycle, and platelet-derived growth factor receptor binding, were significantly enriched in these DEmRNAs with a threshold of FDR <0.05 (Table 1 and Figure 4A,4B). They are both closely associated with the occurrence and progression of cancers. Additionally, the results of KEGG pathway analysis using the “clusterProfiler” package (p.adj <0.01) revealed that these DEmRNAs had a closely relationship with various tumor-associated pathways, such as miRNAs in cancer, human cytomegalovirus and human papillomavirus infection, PI3K-Akt signaling pathway, Rap1 signaling pathway, and cell cycle (Table 2 and Figure 4C,4D). Taken together, these results indicated that 56 DEmRNAs in our ceRNA network play a vital role in the tumorigenesis of GBM.
Table 1
Category | ID | Term | Genes | P.adj |
---|---|---|---|---|
MF | GO:0005515 | protein binding | FBN2, IGSF8, ATP8A1, ITGB3, EGFR, RAP1B, ADAMTS5, SOCS1, GNG5, IGF2BP1, E2F2, NEK2, IGF2BP3, TPPP, IGF2BP2, RAB11FIP4, SNCA, ZNF365, KIRREL3, ABCA1, PDGFRA, HELLS, RRM2, CEP135, H3F3C, TCF12, HMGA2, PDIA5, CDC25A, DCBLD2, VEGFA, WEE1, CDK6, NR5A2, ESPL1, COL4A1, IRF1, DCX, CALU, BIRC5, FAS, CALM3, HOXB5 | 0.0031987 |
MF | GO:0045182 | Translation regulator activity | WEE1, CEP135, BIRC5, CALM3, NEK2, CDC25A | 0.013850364 |
MF | GO:0048027 | mRNA 5’-UTR binding | IGF2BP1, IGF2BP3, IGF2BP2 | 0.022029683 |
BP | GO:0000086 | G2/M transition of mitotic cell cycle | IGF2BP1, IGF2BP3, IGF2BP2 | 0.042209642 |
MF | GO:0005161 | Platelet-derived growth factor receptor binding | IGF2BP1, IGF2BP3, IGF2BP2 | 0.047622832 |
P.adj, adjusted P value; BP, biological process; ceRNA, competitive endogenous RNA; DEmRNAs, differentially expressed mRNAs; MF, molecular function; GO, Gene Ontology; UTR, untranslated region.
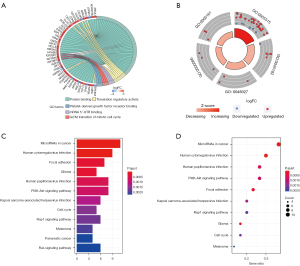
Table 2
ID | Description | P.adj |
---|---|---|
hsa05206 | MicroRNAs in cancer | 9.87e−07 |
hsa05163 | Human cytomegalovirus infection | 5.72e−06 |
hsa04510 | Focal adhesion | 0.000273514 |
hsa05214 | Glioma | 0.000273514 |
hsa05165 | Human papillomavirus infection | 0.000596577 |
hsa04151 | PI3K-Akt signaling pathway | 0.000807859 |
hsa05167 | Kaposi sarcoma-associated herpesvirus infection | 0.001231445 |
hsa04110 | Cell cycle | 0.001570567 |
hsa04015 | Rap1 signaling pathway | 0.001720824 |
hsa05218 | Melanoma | 0.002029455 |
hsa05212 | Pancreatic cancer | 0.002231835 |
hsa04014 | Ras signaling pathway | 0.002231835 |
hsa05219 | Bladder cancer | 0.005223791 |
P.adj, adjusted P value; ceRNA, competitive endogenous RNA; DEmRNAs, differentially expressed mRNAs; KEGG, Kyoto Encyclopedia of Genes and Genome.
Determination of prognostic markers
It has been verified that DEmRNAs are associated with the biological process of GBM (20). To identify key DElncRNAs and DEmRNAs related to the overall survival (OS) of patients with GBM based on the clinical information downloaded from TCGA, batch survival analysis was conducted to investigate Kaplan-Meier curves for GBM patients with 188 DElncRNAs and 56 DEmRNAs in the ceRNA network. Two DElncRNAs (C10orf25 and LINC00336) and three DEmRNAs [transcription factor 12 (TCF12), integrin β3 (ITGB3) and high mobility group AT-hook 2 (HMGA2)] exhibited obvious relationships with prognosis based on their respective optimal cutoffs (P <0.05) (Figure 5A,5B). However, due to the lack of clinical information on miRNAs in the GEO database, the prognostic relevance of miRNAs in GBM could not been verified.
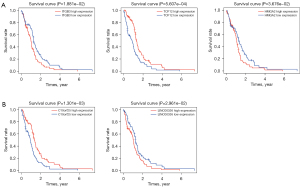
Prognostic risk model of lncRNAs
Using univariate Cox regression, we performed survival analysis of all DElncRNAs in the ceRNA network and then selected effective genes with a P value <0.1. Eventually, 13 lncRNAs were included in subsequent multivariate Cox regression analysis (Table 3). Through multivariate Cox proportional hazards regression analysis with the cutoff criterion of P <0.05, we identified a 5-lncRNA prognostic risk model to predict OS in GBM. Among these lncRNAs, one (C10orf25) was identified to be significantly associated with good survival in patients, while four (LINC00343, HOXA transcript antisense RNA, myeloid-specific 1 (HOTAIRM1), FGF12 antisense RNA 2 (FGF12-AS2) and H19) were significantly associated with poor patient survival (Figure 6A). Afterward, patients from TCGA were divided into low- and high-risk groups according to the multivariate Cox risk score, as shown in Figure 6B. We then estimated the accuracy of the 5-lncRNA model in predicting survival. As shown in the Kaplan-Meier survival curves in Figure 6C, patients with predicted high risk (n=75) had significantly shorter OS than those with low risk (n=76, P=0.00). Finally, we performed the ROC analysis to verify the sensitivity and specificity of the survival prediction of our models. As shown in Figure 6D, the area under the ROC curve (AUC) of the 5-lncRNA model was 0.837. Thus, it is reasonable to believe that this prognostic risk model is helpful to predict OS in GBM. Furthermore, survival analysis determined by the online tool UALCAN (http://ualcan.path.uab.edu) confirmed that H19 was highly expressed in GBM and a significant prognosis-associated lncRNA in this model (P=0.0028) (Figure 6E).
Table 3
Gene | Univariate | Multivariate | |||
---|---|---|---|---|---|
HR | P value | HR | P value | ||
TCF12 | 0.749212792 | 0.002468296 | 0.732609398 | 0.000866173*** | |
HMGA2 | 1.091285431 | 0.036833565 | – | – | |
SOCS1 | 1.119531829 | 0.053373083 | – | – | |
DCBLD2 | 1.180937274 | 0.060760008 | 1.229415971 | 0.018320324* | |
ITGB3 | 1.164797163 | 0.081420074 | – | – | |
HOXB5 | 1.078557228 | 0.082149925 | – | – | |
FAS | 1.156038567 | 0.082301809 | – | – | |
PDIA5 | 1.234966511 | 0.083760846 | – | – | |
C10orf25 | 0.730099975 | 0.005468958 | 0.691707148 | 0.002812926** | |
TLR8-AS1 | 1.232500084 | 0.010232995 | 1.147551853 | 0.116262752 | |
LINC00343 | 1.541331308 | 0.01029754 | 2.123514584 | 0.000174555*** | |
HOTAIRM1 | 1.175602015 | 0.0114416 | 1.172328594 | 0.024638037* | |
FGF12-AS2 | 1.235316997 | 0.019268628 | 1.22307768 | 0.038887302* | |
AC006305.1 | 0.817953561 | 0.026993957 | – | – | |
ZNF32-AS3 | 0.715346757 | 0.031182416 | – | – | |
H19 | 1.068992214 | 0.038723871 | 1.109398048 | 0.003874683** | |
ARMCX3-AS1 | 0.824965104 | 0.050530719 | – | – | |
AC002511.1 | 0.86220575 | 0.052885852 | 0.862193418 | 0.075240461 | |
AC009107.1 | 0.746697154 | 0.078678208 | 0.748349405 | 0.137853981 | |
LINC00336 | 1.204543835 | 0.08360395 | – | – | |
LINC00379 | 1.243795385 | 0.089360322 | – | – |
The top 8 genes are DEmRNAs, the last 13 genes are DElncRNAs. *, P<0.05; **, P<0.01; ***, P<0.001. DEmRNAs, differentially expressed mRNAs; DElncRNAs, differentially expressed long non-coding RNAs; HR, hazard ratio; OS, overall survival.
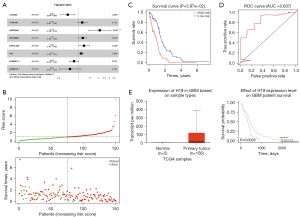
Prognostic risk model of mRNAs
A univariate Cox regression method was used to analyze the survival of all DEmRNAs in the ceRNA network, and 5 effective genes with a P value <0.1 were screened out for subsequent multivariate Cox regression analysis (Table 3). Furthermore, we identified a 2-mRNA prognostic risk model to predict OS in GBM where P<0.05 was considered significant. Among them, TCF12 was related to good survival with a P<0.001, while discoidin, CUB and LCCL domain containing 2 (DCBLD2) was related to poor survival with a P<0.05 (Figure 7A). According to the results of multivariate analysis in Figure 7B, GBM patients were divided into a low-risk group and a high-risk group. Then, a Kaplan-Meier survival curve, used to assess the accuracy of this 2-mRNA model in predicting survival, showed that patients with predicted high risk (n=75) had significantly shorter OS than those with low risk (n=76, P=0.00, Figure 7C). Finally, ROC analysis was further conducted, and the AUC was 0.699, showing a high predictive value of the 2-mRNA prognostic risk model (Figure 7D). Similarly, survival analysis using the online tool Gene Expression Profiling Interactive Analysis (GEPIA2, http://gepia2.cancer-pku.cn/#index) confirmed that DCBLD2 was highly expressed in GBM and a significant prognosis-associated mRNA in this model (P=0.032) (Figure 7E).
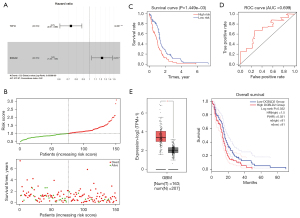
The lncRNA-miRNA-mRNA regulatory axis of the hub gene in GBM
To further understand the ceRNA regulatory mechanism of the core molecules (TCF12, DCBLD2) identified above, we reconstructed another ceRNA network based on interactions between the two core molecules and the corresponding lncRNA and miRNA from the first ceRNA. The results revealed that a total of 77 DElncRNAs and 2 DEmiRNAs formed 86 lncRNA-miRNA interaction pairs, while 2 DEmRNAs and 2 miRNAs formed 2 miRNA-mRNA interaction pairs (Figure 8). Afterward, we combined the reconstructed network with the results of previous survival analysis and found that the C10orf25/hsa-miR-218/DCBLD2 axis was of interest. C10orf25 was also a significant prognostic marker identified above. To identify the underlying functions of candidate genes in the axis, GSEA (Version: 4.1.0; https://www.gsea-msigdb.org/gsea/index.jsp) was conducted on C10orf25 and DCBLD2. With the cutoff criteria of FDR <0.25, we consequently obtained one significant gene set in the C10orf25 high expression group and 966 significant gene sets in the DCBLD2 high expression group. As shown in Figure 9, GBM samples in the C10orf25 high expression group were most significantly enriched in “gene silencing” [normalized enrichment score (NES) =1.875, FDR =0.002], and the top 5 gene sets in the DCBLD2 high-expression GBM sample group contained “cell substrate adhesion” (NES =2.249, FDR =0.012), “cell adhesion molecule binding” (NES =2.183, FDR =0.030), “extracellular structure organization” (NES =2.179, FDR =0.021), “cell matrix adhesion” (NES =2.174, FDR =0.018) and “regulation of epithelial cell migration” (NES =2.172, FDR =0.015). The results show that these two molecules were closely related to progression of GBM, which was consistent with the above results. In addition, previous studies have illustrated that miR-218 is tumor-suppressive in GBM (21). Normally, most lncRNAs are overexpressed in cancer, and they can promote tumor progression by upregulating mRNA expression via downregulated miRNAs. These data remind us that C10orf25/miR-218/DCBLD2 may be an important regulatory pathway in GBM, although many in vivo and in vitro experiments are needed for confirmation.
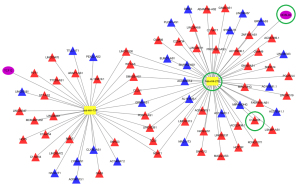
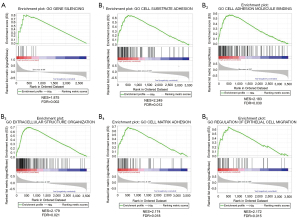
Discussion
GBM is heterogeneous in its molecular subtypes based on transcriptomic classification (22). To date, few molecules associated with the prognosis and mechanisms underlying GBM have been found. Among the established molecular markers of GBM, only the methylation of O6-methylguanine-DNA methyltransferase (MGMT) (23), mutations of isocitrate dehydrogenase (IDH) (24), and the codeletion of the long arm of chromosome 19 (1p/19q) and the short arm of chromosome 1 codeletion (25) are widely applied in the clinic. Given the heterogeneity and limited biomarkers of GBM, it is of great importance to explore new therapeutic targets and regulatory mechanisms in a timely manner. In addition, ceRNAs have recently played an important role in regulating gene expression and are a novel way to elucidate the molecular mechanisms and processes underlying cancers, including GBM (3). However, to the best of our knowledge, limited studies have focused on predicting GBM prognosis according to ceRNAs and it is important to do this work.
In the present study, based on a large cohort from the TCGA and GEO databases, DElncRNAs, DEmiRNAs, and DEmRNAs identified between GBM group and normal group were constructed into a lncRNA-miRNA-mRNA ceRNA network, which is the result of comprehensive integration of target RNA and DERNA data. Considering the interaction of DERNAs in the ceRNA network constructed above, GO and KEGG analyses of 56 DEmRNAs in the network were performed to determine which pathways and biological functions of mRNAs affect the occurrence and development of GBM, thus indirectly uncovering the functions of lncRNAs in the network. Consequently, our results confirmed the functions of these mRNAs in GBM pathogenesis, such as translation regulator activity, mRNA 5'-UTR binding, and G2/M transition of the mitotic cell cycle. The KEGG enrichment analysis of these genes also identified some vital pathways related to carcinogenesis, including miRNAs in cancer, the PI3K-Akt signaling pathway, the Rap1 signaling pathway and the cell cycle.
In our risk model constructed by univariate and multivariate Cox regression, 2-mRNA (TCF12 and DCBLD2) and 5-lncRNA (C10orf25, LINC00343, HOTAIRM1, FGF12-AS2 and H19) were identified as two holistic models to identify hub DEGs associated with GBM prognosis. Their AUC values were 0.699 and 0.837, proving the validity of these two models. Then, we verified the survival curves of genes in these models by using online tools. Our results suggest that DCBLD2 is overexpressed in GBM compared to normal brain tissues, and its overexpression represents poor survival in GBM. Previous studies have also verified that DCBLD2 may act as a survival marker gene in several cancers, including colorectal cancer (26), pancreatic cancer (27) and even GBM (28). LncRNA H19, one of the first reported long noncoding RNAs, has been shown to be a candidate for the development of promising therapeutic and diagnostic modalities for several cancers (29), especially GBM (30-32). Our results also indicate that overexpression of H19 might be a promising therapeutic target in GBM.
In addition, through batch survival analysis of DEGs in the network based on TCGA clinical information, we revealed that the expression of DEmRNAs TCF12, ITGB3 and HMGA2, and DElncRNAs C10orf25 and LINC00336 is closely related to the prognosis of GBM. TCF12, known as transcription factor 12 (HEB), has been shown to have a role in neuron differentiation and is upregulated in some tumors (33). Another study also demonstrated that targeting HEB can inhibit the proliferation of U87MG GBM cells (34), which is consistent with our finding that overexpression of TCF12 represents a good prognosis in GBM. Previous studies have suggested that ITGB3 possesses expression levels twofold higher in cancer stem cells (CSCs) than in differentiated GBM cells (35), and EGFRvIII/ITGB3 complexes can promote GBM progression and metastasis within the tumor microenvironment (36), thus serving as promising therapeutic targets. Multiple studies have validated that targeting HMGA2 in GBM may be therapeutically beneficial. High expression of HMGA2 in GBM has been confirmed to promote GBM cell viability, invasion, migration, stemness and tumor growth, inhibit cell apoptosis and autophagy (37,38), and be involved in the double-strand break repair pathway (39). LINC00336, despite the lack of any study in GBM, has been identified to be significantly correlated with renal cell carcinoma OS (40), and its overexpression may promote lung cancer cell growth, colony formation, and tumor formation, and inhibit ferroptosis (41). Regarding C10orf25, nothing is known about its functions, but our results strongly indicate that it has potential in survival prediction and may provide a new direction for further investigation in GBM.
According to two hub genes (TCF12 and DCBLD2) in our constructed 2-mRNA risk model, lncRNAs and mRNAs associated with them were selected from the first ceRNA network to construct a new core network. Combined with survival analysis, we focused on the regulatory axis of C10orf25/miR-218/DCBLD2. Furthermore, we performed GSEA for each molecule in the axis according to their expression data from the TCGA and GEO databases. The results revealed that the gene set “gene silencing” was enriched in the C10orf25 high expression phenotype and that the top 5 gene sets in the DCBLD2 high-expression GBM sample group were basically functions associated with matrix adhesion and cell migration. However, no gene set associated with miR-218 was found. MiR-218 has been explored in many studies on GBM. For instance, published studies have revealed that miR-218 can downregulated the expression of lymphoid enhancer-binding factor 1 (LEF1) and matrix metalloproteinase 9 (MMP-9), which are related to the invasive phenotype of GBM cells, thus indicating its utility for developing potential clinical strategies for GBM (42). Additionally, high expression of miR-218 in U87 and U251 cell lines may arrest cell cycle progression in G1 phase and inhibit cell proliferation (43). According to a functional analysis, we further confirmed that molecules in our model constructed above were closely related to the progression of GBM. In addition, we hypothesized that C10orf25 may weaken the downregulation effect of miR-218 on the target gene DCBLD2, which leads to the overexpression of DCBLD2, thus participating in the occurrence and development of GBM. However, many in vivo and in vitro experiments are warranted to verify its authenticity and underlying mechanism in the future.
In summary, we constructed two effective prediction models that include 2 mRNAs (TCF12 and DCBLD2) and 5 lncRNAs (C10orf25, LINC00343, HOTAIRM1, FGF12-AS2 and H19) through integrated bioinformatic analyses. Additionally, we identified several key molecules (TCF12, ITGB3, HMGA2, C10orf25 and LINC00336) closely associated with GBM prognosis using a large cohort from the TCGA and GEO databases. C10orf25/miR-218/DCBLD2 may be an important regulatory pathway in GBM. Overall, our findings contribute to further understanding the pathogenesis of GBM and finding possible candidate genes for prognostic and therapeutic usage with GBM. Of course, despite these meaningful findings, the present study had some limitations. Further validation will be needed with in vivo and in vitro experiments and clinical practice.
Acknowledgments
The results of our study are based on data from TCGA (https://portal.gdc.cancer.gov/) and the GEO platform (https://www.ncbi.nlm.nih.gov/geo/).
Funding: This study was supported by
Footnote
Reporting Checklist: The authors have completed the MDAR reporting checklist. Available at https://dx.doi.org/10.21037/tcr-21-1162
Peer Review File: Available at https://dx.doi.org/10.21037/tcr-21-1162
Conflicts of Interest: All authors have completed the ICMJE uniform disclosure form (available at https://dx.doi.org/10.21037/tcr-21-1162). The authors have no conflicts of interest to declare.
Ethical Statement: The authors are accountable for all aspects of the work in ensuring that questions related to the accuracy or integrity of any part of the work are appropriately investigated and resolved. The study was conducted in accordance with the Declaration of Helsinki (as revised in 2013).
Open Access Statement: This is an Open Access article distributed in accordance with the Creative Commons Attribution-NonCommercial-NoDerivs 4.0 International License (CC BY-NC-ND 4.0), which permits the non-commercial replication and distribution of the article with the strict proviso that no changes or edits are made and the original work is properly cited (including links to both the formal publication through the relevant DOI and the license). See: https://creativecommons.org/licenses/by-nc-nd/4.0/.
References
- Aum DJ, Kim DH, Beaumont TL, et al. Molecular and cellular heterogeneity: the hallmark of glioblastoma. Neurosurg Focus 2014;37:E11. [Crossref] [PubMed]
- Anjum K, Shagufta BI, Abbas SQ, et al. Current status and future therapeutic perspectives of glioblastoma multiforme (GBM) therapy: A review. Biomed Pharmacother 2017;92:681-9. Erratum in: Biomed Pharmacother 2018 Mar 8;101:820. PMID: 28582760. [Crossref] [PubMed]
- Salmena L, Poliseno L, Tay Y, et al. A ceRNA hypothesis: the Rosetta Stone of a hidden RNA language? Cell 2011;146:353-8. [Crossref] [PubMed]
- Bartel DP. MicroRNAs: target recognition and regulatory functions. Cell 2009;136:215-33. [Crossref] [PubMed]
- Abba ML, Patil N, Leupold JH, et al. MicroRNAs as novel targets and tools in cancer therapy. Cancer Lett 2017;387:84-94. [Crossref] [PubMed]
- Fan Q, Liu B. Comprehensive analysis of a long noncoding RNA-associated competing endogenous RNA network in colorectal cancer. Onco Targets Ther 2018;11:2453-66. [Crossref] [PubMed]
- Abdollahzadeh R, Daraei A, Mansoori Y, et al. Competing endogenous RNA (ceRNA) cross talk and language in ceRNA regulatory networks: A new look at hallmarks of breast cancer. J Cell Physiol 2019;234:10080-100. [Crossref] [PubMed]
- Ulitsky I, Bartel DP. lincRNAs: genomics, evolution, and mechanisms. Cell 2013;154:26-46. [Crossref] [PubMed]
- Ransohoff JD, Wei Y, Khavari PA. The functions and unique features of long intergenic non-coding RNA. Nat Rev Mol Cell Biol 2018;19:143-57. [Crossref] [PubMed]
- Zhou RS, Zhang EX, Sun QF, et al. Integrated analysis of lncRNA-miRNA-mRNA ceRNA network in squamous cell carcinoma of tongue. BMC Cancer 2019;19:779. [Crossref] [PubMed]
- Zhao Y, Wang H, Wu C, et al. Construction and investigation of lncRNA-associated ceRNA regulatory network in papillary thyroid cancer. Oncol Rep 2018;39:1197-206. [Crossref] [PubMed]
- Long J, Xiong J, Bai Y, et al. Construction and Investigation of a lncRNA-Associated ceRNA Regulatory Network in Cholangiocarcinoma. Front Oncol 2019;9:649. [Crossref] [PubMed]
- Liu Z, Wang X, Yang G, et al. Construction of lncRNA-associated ceRNA networks to identify prognostic lncRNA biomarkers for glioblastoma. J Cell Biochem 2020;121:3502-15. [Crossref] [PubMed]
- Zhu X, Jiang L, Yang H, et al. Analyzing the lncRNA, miRNA, and mRNA-associated ceRNA networks to reveal potential prognostic biomarkers for glioblastoma multiforme. Cancer Cell Int 2020;20:393. [Crossref] [PubMed]
- Li Y, Guo D. Identification of Novel lncRNA Markers in Glioblastoma Multiforme and Their Clinical Significance: A Study Based on Multiple Sequencing Data. Onco Targets Ther 2020;13:1087-98. [Crossref] [PubMed]
- Pastori C, Kapranov P, Penas C, et al. The Bromodomain protein BRD4 controls HOTAIR, a long noncoding RNA essential for glioblastoma proliferation. Proc Natl Acad Sci U S A 2015;112:8326-31. [Crossref] [PubMed]
- Tan SK, Pastori C, Penas C, et al. Serum long noncoding RNA HOTAIR as a novel diagnostic and prognostic biomarker in glioblastoma multiforme. Mol Cancer 2018;17:74. [Crossref] [PubMed]
- Tian Y, Zheng Y, Dong X. AGAP2-AS1 serves as an oncogenic lncRNA and prognostic biomarker in glioblastoma multiforme. J Cell Biochem 2019;120:9056-62. [Crossref] [PubMed]
- Li Y, Xu J, Chen H, et al. Comprehensive analysis of the functional microRNA-mRNA regulatory network identifies miRNA signatures associated with glioma malignant progression. Nucleic Acids Res 2013;41:e203. [Crossref] [PubMed]
- Yan Y, Zhang L, Jiang Y, et al. LncRNA and mRNA interaction study based on transcriptome profiles reveals potential core genes in the pathogenesis of human glioblastoma multiforme. J Cancer Res Clin Oncol 2015;141:827-38. [Crossref] [PubMed]
- Gao X, Jin W. The emerging role of tumor-suppressive microRNA-218 in targeting glioblastoma stemness. Cancer Lett 2014;353:25-31. [Crossref] [PubMed]
- Wu S, Mischel PS. Same Script, Different Cast: Different Cell Origins Shape Molecular Features and Therapeutic Response in Glioblastoma. Cancer Cell 2020;38:311-3. [Crossref] [PubMed]
- Hegi ME, Diserens AC, Gorlia T, et al. MGMT gene silencing and benefit from temozolomide in glioblastoma. N Engl J Med 2005;352:997-1003. [Crossref] [PubMed]
- Ichimura K, Pearson DM, Kocialkowski S, et al. IDH1 mutations are present in the majority of common adult gliomas but rare in primary glioblastomas. Neuro Oncol 2009;11:341-7. [Crossref] [PubMed]
- Riemenschneider MJ, Jeuken JW, Wesseling P, et al. Molecular diagnostics of gliomas: state of the art. Acta Neuropathol 2010;120:567-84. [Crossref] [PubMed]
- Martinez-Romero J, Bueno-Fortes S, Martín-Merino M, et al. Survival marker genes of colorectal cancer derived from consistent transcriptomic profiling. BMC Genomics 2018;19:857. [Crossref] [PubMed]
- Raman P, Maddipati R, Lim KH, et al. Pancreatic cancer survival analysis defines a signature that predicts outcome. PLoS One 2018;13:e0201751. [Crossref] [PubMed]
- Feng H, Lopez GY, Kim CK, et al. EGFR phosphorylation of DCBLD2 recruits TRAF6 and stimulates AKT-promoted tumorigenesis. J Clin Invest 2014;124:3741-56. [Crossref] [PubMed]
- Yoshimura H, Matsuda Y, Yamamoto M, et al. Expression and role of long non-coding RNA H19 in carcinogenesis. Front Biosci (Landmark Ed) 2018;23:614-25. [Crossref] [PubMed]
- Jiang X, Yan Y, Hu M, et al. Increased level of H19 long noncoding RNA promotes invasion, angiogenesis, and stemness of glioblastoma cells. J Neurosurg 2016;124:129-36. [Crossref] [PubMed]
- Wu W, Hu Q, Nie E, et al. Hypoxia induces H19 expression through direct and indirect Hif-1α activity, promoting oncogenic effects in glioblastoma. Sci Rep 2017;7:45029. [Crossref] [PubMed]
- Xiao Y, Zhu Z, Li J, et al. Expression and prognostic value of long non-coding RNA H19 in glioma via integrated bioinformatics analyses. Aging (Albany NY) 2020;12:3407-30. [Crossref] [PubMed]
- Wu K, Li S, Bodhinathan K, et al. Enhanced expression of Pctk1, Tcf12 and Ccnd1 in hippocampus of rats: Impact on cognitive function, synaptic plasticity and pathology. Neurobiol Learn Mem 2012;97:69-80. [Crossref] [PubMed]
- Godoy PR, Montaldi AP, Sakamoto-Hojo ET. HEB silencing induces anti-proliferative effects on U87MG cells cultured as neurospheres and monolayers. Mol Med Rep 2016;14:5253-60. [Crossref] [PubMed]
- Shevchenko V, Arnotskaya N, Pak O, et al. Molecular determinants of the interaction between glioblastoma CD133+ cancer stem cells and the extracellular matrix. Int Rev Neurobiol 2020;151:155-69. [Crossref] [PubMed]
- Liu Z, Han L, Dong Y, et al. EGFRvIII/integrin β3 interaction in hypoxic and vitronectinenriching microenvironment promote GBM progression and metastasis. Oncotarget 2016;7:4680-94. [Crossref] [PubMed]
- Kaur H, Ali SZ, Huey L, et al. The transcriptional modulator HMGA2 promotes stemness and tumorigenicity in glioblastoma. Cancer Lett 2016;377:55-64. [Crossref] [PubMed]
- Jia WQ, Zhu JW, Yang CY, et al. Verbascoside inhibits progression of glioblastoma cells by promoting Let-7g-5p and down-regulating HMGA2 via Wnt/beta-catenin signalling blockade. J Cell Mol Med 2020;24:2901-16. [Crossref] [PubMed]
- Liu Y, Shete S, Etzel CJ, et al. Polymorphisms of LIG4, BTBD2, HMGA2, and RTEL1 genes involved in the double-strand break repair pathway predict glioblastoma survival. J Clin Oncol 2010;28:2467-74. [Crossref] [PubMed]
- He HT, Xu M, Kuang Y, et al. Biomarker and competing endogenous RNA potential of tumor-specific long noncoding RNA in chromophobe renal cell carcinoma. Onco Targets Ther 2016;9:6399-406. [Crossref] [PubMed]
- Wang M, Mao C, Ouyang L, et al. Long noncoding RNA LINC00336 inhibits ferroptosis in lung cancer by functioning as a competing endogenous RNA. Cell Death Differ 2019;26:2329-43. [Crossref] [PubMed]
- Liu Y, Yan W, Zhang W, et al. MiR-218 reverses high invasiveness of glioblastoma cells by targeting the oncogenic transcription factor LEF1. Oncol Rep 2012;28:1013-21. [Crossref] [PubMed]
- Zhang Y, Han D, Wei W, et al. MiR-218 Inhibited Growth and Metabolism of Human Glioblastoma Cells by Directly Targeting E2F2. Cell Mol Neurobiol 2015;35:1165-73. [Crossref] [PubMed]