Transcriptome analysis of the effect of a novel human serine protease inhibitor SPINK13 on gene expression in MHCC97-H cells
Introduction
Previous studies have shown that Serine peptidase inhibitor, Kazal type 13 (SPINK13) has antitumor effects in vitro and in vivo (1,2), but the molecular mechanism is still unclear. Studying the changes of cell transcriptome caused by drug treatment can help to elucidate the process of cell responses to drugs and the biological pathway involved in the responses (3). Transcriptome sequencing based on the Illumina second generation sequencing platform has the advantages of accurate quantification, high throughput, good repeatability, wide detection range, low sequencing cost, and comprehensive study of the whole gene expression profile of samples, which has been widely used in many fields (4,5). Previous studies have not fully elucidated the molecular mechanism of SPINK13’s antitumor effects. In this study, transcriptome sequencing and bioinformatics analysis were used to analyze the differential gene expression of MHCC97-H cells treated by SPINK13 to determine the molecular mechanism for SPINK13 to inhibit tumors.
The purpose of this study was to explore the molecular mechanism for SPINK13 of inhibiting tumors by using transcriptome sequencing technology to detect the gene expression difference of tumor cells with and without SPINK13 treatment. We comprehensively studied the molecular mechanism of SPINK13 to inhibit hepatocellular carcinoma through transcriptome sequencing and bioinformatics analysis. The packages edgeR, DEseq (6), CuffDiff, and EBSeq (7) were used in the bioinformatics analysis. The functional classification and biological pathway of differential genes were enriched in the Gene Ontology (GO) (8) and Kyoto Encyclopedia of Genes and Genomes (KEGG) databases (9,10). Through transcriptome sequencing, differential expression analysis and gene enrichment analysis, we studied the effect of SPINK13 on MHCC97-H cells on the transcriptional level. We present the following article in accordance with the MDAR reporting checklist (available at https://dx.doi.org/10.21037/tcr-21-1928).
Methods
Instrument and reagents
An Agilent Bioanalyzer 2100 system (Agilent Technologies Inc., Santa Clara, CA, USA) was used for the determination of DNA concentration; Proflex (Life Technologies, Carlsbad, CA, USA) was used for polymerase chain reaction, and an Illumina Hiseq2500 platform (Illumina Inc., San Diego, USA) was used for high-throughput sequencing. TRIzol reagent was obtained from Invitrogen Corp. (Waltham, MA, USA); chloroform and 2-propanol were purchased from Sinopharm (Shanghai, China); Agarose was obtained from Biowest (Barcelona, Spain); a human Ribo-Zero Magnetic Gold Kit and NEBNext® Ultra™ RNA Library Prep Kit were purchased from Illumina Inc. (USA); Human hepatocellular carcinoma cell line, MHCC97-H cells, was donated from Dr. Feng Fan; and SPINK13 protein was synthesized by our laboratory from exogenous expression of HE239T cells. The study was conducted in accordance with the Declaration of Helsinki (as revised in 2013).
Sample preparation
The samples of MHCC97-H cells were divided into 2 groups: group BC (blank control), and group EG (experimental group, 30 µg/mL cells treated with SPINK13), with 3 parallel biological samples were set in each group. The total number of cells was about 107.
Total RNA extraction
Total RNA was extracted by TRIzol method (11-13). The sample was diluted 1:50 with RNase-free water. The concentration and the purity were detected with Thermo NanoDrop™ One Ultra-Micro Spectrophotometer (Thermo Fisher Scientific, Waltham, MA, USA). After passing the quality test, the RNA solution was stored in the refrigerator at −80 °C.
Messenger RNA purification
According to Illumina’s instructions for the Ribo-Zero Magnetic Gold Kit (Human), messenger RNA (mRNA) was enriched from total RNA samples using oligo (dT) coupled to magnetic beads.
Library preparation and detection
Library preparation and detection were performed according to the instructions for NEBNext® Ultra™ RNA Library Prep Kit for Illumina® (#E7530L, NEB).
Preliminary quantification of the achieved library was made with Qubit 3.0 (Thermo Fisher, USA) and the fragment size of the library was detected with the Agilent Bioanalyzer 2100 system. The required fragment size was 250–550 bp. The effective concentration of real-time quantitative polymerase chain reaction (qPCR) analysis was performed with Bio-Rad CFX 96 fluorescence qPCR detection system (Bio-Rad Laboratories, Hercules, CA, USA) and Bio-Rad kit iQ SYBR Green. The required effective concentration of the library was more than 10 nM.
Sequencing
The library was diluted to 2 nM and high-throughput sequencing was performed on Hiseq2500 platform (Illumina Inc., USA).
Statistical analysis
Sequencing quality analysis
SolexaQA (Illumina, USA) was used to filter out the sequences with base quality score less than 20 in the original data, that is, the longest sequences with base quality score more than 20 in the original read were retained. If the length of the filtered reads was less than 35 bp, the whole reads were discarded. Finally, 2 reads met the conditions in the reserved paired end data.
Gene transcriptional level calculation
TopHat (version 2.1.1; https://ccb.jhu.edu/software/tophat/index.shtml) was used to compare the filtered data to the human reference genome (GRCh, version 38.82) (14,15). After confirming that the samples of each group were not contaminated by other samples, the transcripts of each sample were further constructed using Cufflinks (version 2.2.1; http://cole-trapnell-lab.github.io/cufflinks/releases/v2.2.1/), and the transcriptional levels of genes and transcripts were obtained respectively, which were indicated by fragments per kilobase of exon model per million mapped fragments (FPKM).
Differential gene expression analysis
A total of 4 separate differential expression tests were implemented by DEGseq and the intersection was obtained from the results of Fisher’s exact test (FET), fold-change threshold on the MA-plot (FC), likelihood ratio test (LRT), and the MA-plot-based method with random sampling model (MARS). The significance of the differences among all genes and transcripts was calculated by t-test, and the genes whose expression changes were statistically significant (P value <0.05) and whose differences were more than 2 times (|log2 (fold change)| >1) were defined as differentially expressed genes (DEGs).
GO enrichment analysis
The identified DEGs were analyzed by GO (16). The online bioinformatics website, Functional annotation bioinformatics microarray analysis (DAVID, https://david.ncifcrf.gov/) (17) was used to carry out GO gene function annotation and enrichment analysis on important DEGs. The GO analysis was based on hypergeometric testing to calculate whether the DEGs identified in the classification of functional terms are enriched in a certain class of GO terms (18,19).
KEGG enrichment analysis
The identified differential genes were analyzed by KEGG (9,10). In the KEGG pathway database, biological metabolic pathways are divided into 6 categories: cellular processes, environmental information processing, genetic information processing, human diseases, metabolism, and organismal systems and each of them is divided into layer 2, layer 3, and layer 4. The layer 2 currently includes 43 seed pathways, the layer 3 is the metabolic pathway map, and the layer 4 is the specific annotation information of each metabolic pathway map (20-26). In this paper, DAVID online analysis was used, taking DEGs as foreground and all genes as background, and calculated the enrichment degree of foreground genes in each signal pathway (expressed by P value) based on hypergeometric distribution algorithm. Pathway analysis was used to identify the signaling pathways involved in the DEGs, the most important biochemical metabolic pathway, and signal transduction pathway.
Protein interaction analysis of DEGs
The identified DEGs were submitted to an online tool, STRING database (https://www.string-db.org/) (27), for protein-protein interaction (PPI) analysis (28,29). This analysis can provide ideas for mining candidate genes for treatment.
Results
RNA extraction and library quality inspection results
After RNA extraction, the purity of RNA was detected with NanoDrop 2000 micro spectrophotometer, and the integrity of RNA samples was detected with Agilent 2100 bioanalyzer and Agilent RNA 6000 Nano kit. The test results showed that the RNA extraction results of all samples met the following requirements: the total amount was more than 200 ng, the concentration was more than 20 ng/µL, RIN ≥7.0, 28 s/18 s ≥1.0, optical density (OD) 260/280 ≥1.8 and OD 260/230 ≥1.8, indicating that the extracted RNA had high concentration, good integrity, and no protein or polysaccharide pollution. Therefore, the total amount and quality of samples met the requirements of subsequent database construction, making it suitable for subsequent experiments.
The size of the final product was 392–422 bp, which met the requirements of 250–550 bp. The qPCR results showed that the molarity of the 7 groups of samples were over 10 nM. Thus, the constructed library fully met the requirements of subsequent sequencing and could be sequenced.
Filtration of low-quality data
After sequencing of the transcriptome library on Hiseq2500 sequencing platform was completed, about 24 megabytes of data was obtained from each sample, a 2-terminal sequence with length of 150 bp (reads). Low-quality data was filtered out from the obtained transcriptome data by comparing and analyzing the expression difference, function, localization, pathway, and so on. The analysis showed that the Q20 and Q30 of each group were more than 98% and 92%, respectively, and the sequencing quality was good. The final data for analysis (clean data) was obtained after adapter removal and quality trimming from the original sequencing results. The quality of the filtered sequencing data met the requirements of subsequent analysis, and the amount of sequencing data in each group basically showed no difference, which could be used for further analysis of DEGs and functional annotation.
Calculation of gene transcriptional level
TopHat (version 2.1.1) was used to compare the filtered data of 8 samples to the human reference genome (GRCh version 38.82). The comparison results showed that all overall read mapping rates were more than 85%, indicating that the quality of sequencing data was high and there was no contamination of other samples in each group. Thus, the transcript information of each sample was constructed, and the transcriptional levels of gene and transcripts were obtained respectively (Figures 1,2).
Identification of DEGs
In order to determine the mechanism of SPINK13, after the transcription level data was obtained, the DEGs whose expression level had changed resulting from SPINK13 treatment were identified by comparing the expression levels of genes in the EG and BC groups. The DEGs and transcripts are displayed in Figures 3,4, respectively. There were 446 genes significantly differentially expressed between the EG group and BC group, of which 347 genes were up-regulated in the EG group, while 99 genes were down regulated in the EG group.
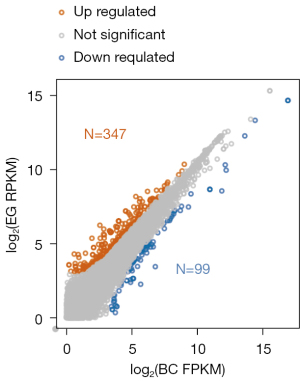
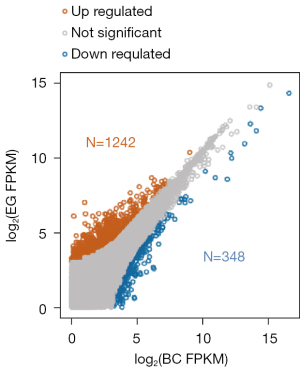
GO enrichment analysis of DEGs
The GO enrichment analysis of the DEGs treated by SPINK13 was performed with DAVID (Figures 5-7). The results of cell component enrichment showed that the most significant change in the transcription level of genes occurred in the nucleus (Figure 4). The results of molecular function enrichment showed that the transcription level of the genes related to DNA binding and chromosome changed significantly after SPINK13 treatment (Figure 5). There were many kinds of terms enriched in biological processes, among which nucleosome assembly was the strongest signal (nucleosome assembly is related to S phase of cell cycle). In addition, the terms related to cell division and proliferation were also enriched, indicating that SPINK13 treatment significantly changed the transcription level of genes related to biological processes such as nucleosome assembly and cell division, including CDC20, CDC34, CDCA8, and CDCA5, which are classical cell cycle regulatory proteins. It is worth noting that terms related to liver development were also enriched (Figure 6). Among the upregulated genes, there are a total of 32 tumor-related genes were HMGB2, NR4A2, TOP2, CEBPB, and so on, of which 12 genes in total related to liver cancer were HMGB2, CCNA2, CDCA8, HJURP, and so on. Meanwhile, a total of 2 genes were downregulated and neither of them was related to tumors.
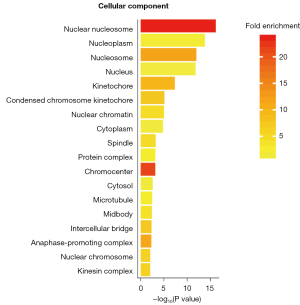
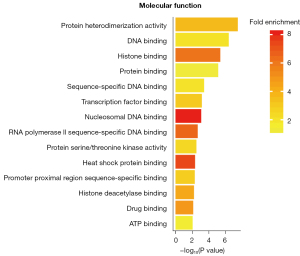
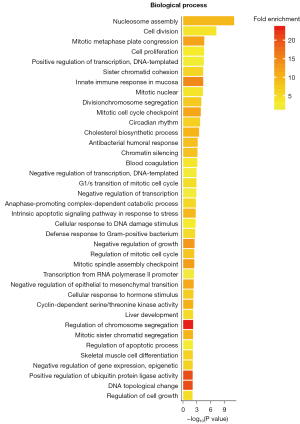
KEGG enrichment analysis of DEGs
DAVID online analysis was used to perform KEGG enrichment analysis for 446 DEGs, and pathway analysis was used to determine the signal pathways, the most important biochemical metabolic pathway and signal transduction pathway involved in the DEGs (Figure 7).
The KEGG enrichment results showed that there were 13 signaling pathways with P<0.001, of which the 3 most related signaling pathways were systemic lupus erythematosus, alcoholism, and cell cycle-related signaling pathways. The results for the enrichment of systemic lupus erythematosus and alcoholism seemed to be inconsistent with our previous understanding. After we carefully examined the DEGs that played a role in these 2 pathways, we found that these genes played a role related to nucleosome in the systemic lupus erythematosus signaling pathway and participated in the regulation of histone and MAPK signaling in the alcoholism signaling pathway. This is consistent with the enrichment results of the third pathway, cell cycle related pathway. These results suggest that SPINK13 affects the normal progress of cell cycle. It is worth noting that the enrichment results also showed the related signal pathways that inhibited the proliferation of cancer cells, such as transcriptional in cancer, p53 signal pathway, tumor necrosis factor (TNF) signal pathway, microRNAs in cancer, and MAPK signal pathway.
The KEGG enrichment analysis showed that there were 6 classical signaling pathways related to tumor in the 13 signaling pathways with P value <0.001, and a total of 58 input genes. Among the 58 genes, 8 genes, JUN, GADD45A, GADD45B, TNFRSF1A, FOS, CDKN1B, NFKBIA, and BBC3 met 2 or more classical tumor signaling pathways and were the conditional genes by which SPINK13 resulted in gene alteration and antitumor effects.
Protein interaction analysis of DEGs
Protein interaction analysis showed that there were 35 nodal proteins upregulated after SPINK13 treatment, of which 14 proteins were related to tumor. They were HIST2H2BE, HIST1H2AC, RCC1, CKS1B, UBE2C, TOP2A, CCNB2, CCNA2, CDC20, AURKB, PTTG1, BUBI, NEK2 and INCENP (30-34). Except that RCC1, AURKB, NEk2, and INCENP were not found in the KEGG pathway, the remaining 10 genes had the largest number in the cell cycle pathway. This result was consistent with the previous enrichment analysis. Protein interaction analysis showed that there were only 2 nodal proteins downregulated after SPINK13 treatment. They were ACAT2 and HMGCS1, which were not related to tumor. Cholesterol acyltransferase 2, ACAT2, was mainly expressed in the liver and small intestine (35,36); whereas HMGCS1, a key enzyme that catalyzes the condensation of acetyl CoA and the conversion of acetyl CoA to 3-hydroxy-3-methylglutaryl, was involved in the biosynthesis of cholesterol in the liver and was related to liver and adipose tissue metabolism (37-39) (Figures 8,9).
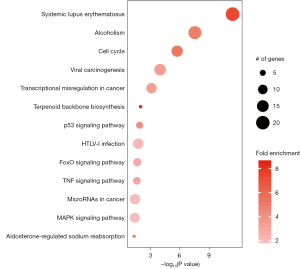
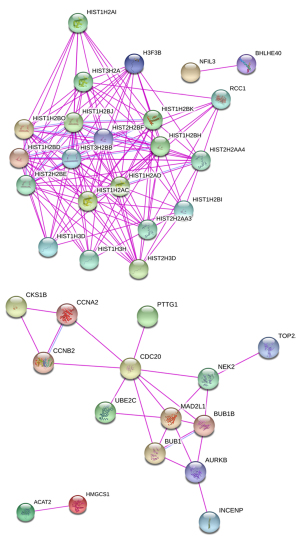
Discussion
Herein, we mainly discuss the relationship between SPINK13 and genes in tumor signaling pathways, so as to provide reference for further research exploring the molecular mechanism for SPINK13 to inhibit tumors. We studied the effect of SPINK13 on MHCC97-H cells on the transcriptional level including transcriptome sequencing, differential expression analysis and gene enrichment analysis. There were 8 genes that were present at the same time in 2 or more classical tumor signaling pathways (P<0.001 in KEGG analysis), namely, JUN, FOS, GADD45A, GADD45B, TNFRSF1A, CDKN1B, NFKBIA, and BBC3.
SPINK13 belongs to the SPINK family which has a characteristic signature that contains at least one conserved Kazal domain with six consensus cysteines forming a 1–5/2–4/3–6 disulfide bond pattern (40). The deregulation of SPINK protein can lead to serious diseases, such as acrosomal integrity and male fertility, clear cell renal cell carcinoma and ovarian cancer (41-43).
The gene JUN is a proto-oncogene, encoding c-Jun protein which is a transcription factor of activation protein 1 (AP-1) family. The increase of JUN expression level will lead to abnormal cell proliferation and division, causing carcinogenesis. High expression of JUN can be detected in a variety of cancers (44). The gene FOS is also a proto-oncogene, encoding c-Fos protein which is a transcription factor of activation protein 1 (AP-1) family (45). The c-Fos protein can form dimer AP-1 transcription factor with c-Jun protein, which is involved in the regulation of cell proliferation, differentiation, transformation, and apoptosis process (45). Omics data analysis showed that FOS and JUN were significantly upregulated in hepatoma cells, and AP-1 transcription factor formed by the combination of c-Fos and c-Jun was in the central position in the carcinogenic signal regulatory network of hepatoma cells (46). The AP-1 transcription factor is a downstream-activated transcription factor in the MAPK signal transduction pathway (47). The results of RNA-seq showed that the expression levels of JUN and FOS in hepatocarcinoma cells were significantly down-regulated after SPINK13 treatment, indicating that SPINK13 might inhibit the growth of hepatocarcinoma cells by inhibiting the JNK signaling pathway.
The GADD45 gene is a class of growth arrest and DNA damage inducible (GADD) genes. The GADD45 protein is involved in DNA damage repair, cell cycle regulation, apoptosis, and other cell life activities. It is closely related to the occurrence of cancer, and the expression level of GADD45 is downregulated in a variety of cancer cells; in other words, it is a tumor suppressor (48). In the study on hepatocarcinoma cells, it was found that the upregulated expression of GADD45 could inhibit the development of liver cancer (49). In this study, we found that 2 members of the GADD45 family, GADD45A and GADD45B, were upregulated after treatment with SPINK13, indicating that SPINK13 can inhibit hepatocarcinoma cells by increasing the expression of GADD45.
The TNF receptor encoded by TNFRSF1A is the receptor of TNF-α on the cell membrane. It can mediate the signal pathway and activate the downstream transcription factor nuclear factor kappa-B (NF-κB), thus participating in the regulation of cell proliferation, differentiation, and apoptosis. On the one hand, TNFRSF1A protein can activate NF-κB through the IKK/NF-κB signaling pathway, or activate the JNK signaling pathway, and finally induce the expression of anti-apoptosis genes. On the other hand, TNFRSF1A protein can form a death-inducing signal complex with other proteins, thus causing apoptosis. Therefore, whether the activation of TNFRSF1A can induce cell survival or death depends on the signal level of both sides and the time of action (50). In this study, the expression level of TNFRSF1A was significantly upregulated after SPINK13 treatment. Further studies are needed to confirm how the upregulated TNFRSF1A by SPINK13 works.
As an inhibitor protein encoded by NFKBIA, NF-κB is an endogenous inhibitor of transcription factor NF-κB. The NFKBIA protein, by binding with NF-κB, covers the nuclear localization signal region of NF-κB, making it unable to enter the nucleus, thus inhibiting the anti-apoptotic pathway mediated by NFκB (51). A study found that NFKBIA expression level decreased in a variety of malignant tumors (52). In patients with liver cancer who had different clinical symptoms, the expression of NFKBIA was decreased in liver cancer tissue (53). In this study, the expression level of NFKBIA was significantly upregulated after SPINK13 treatment, indicating that SPINK13 might inhibit the growth of cancer cells by inhibiting the IKK/NF-κB signal transduction pathway.
The BH3-only protein of Bcl-2 family encoded by BBC3 is an apoptosis initiation protein, which plays an important role in the process of apoptosis (54). The upstream promoter sequence of BBC3 contains the binding site of p53, a key target protein of apoptosis (55). Studies have shown that the downregulation of BBC3 is related to the occurrence of liver cancer, breast cancer, rectal cancer, and other malignant tumors (56-58). In this study, the expression level of BBC3 was significantly upregulated after SPINK13 treatment, indicating that SPINK13 can inhibit the growth of cancer cells by increasing the expression of BBC3.
The p27kip1 protein encoded by CDKN1B is a cyclin-dependent kinase (CDK) inhibitor. The binding of p27kip1 to cyclin E-CDK2 or cyclin D-CDK4 will result in the loss of cyclin kinase activity of E-CDK2 and D-CDK4 proteins, thus preventing the transition from G1 phase to S phase, and realizing the negative regulation function of cell proliferation (59). The gene CDKN1B is a tumor suppressor, which is involved in cell differentiation, proliferation, apoptosis, intercellular adhesion, growth inhibition, and other cellular functions. The decreased expression level of CDKN1B can be detected in a variety of cancers (60). In this study, the expression level of CDKN1B was significantly upregulated after SPINK13 treatment, indicating that SPINK13 can regulate the expression of CDKN1B, thus affecting the process of cell cycle and inhibiting tumor.
The results of molecular function enrichment showed that nucleosome assembly, terms related to cell division and proliferation, liver development were enriched after SPINK13 treatment. A total of 32 tumor-related genes including HMGB2, NR4A2, TOP2, CEBPB were upregulated, 12 genes including HMGB2, CCNA2, CDCA8, HJURP were related to liver cancer. In addition, 2 genes were downregulated and neither of them was related to tumors.
At present, there are many known causes of hepatocellular carcinogenesis, involving many pathways of carcinogenesis, such as p53 pathway, receptor tyrosine kinase pathway, PI3K/Akt signaling pathway, MAPK signaling pathway, IKK/NF-κB signaling pathway, transforming growth factor-β (TGF-β) signaling pathway, and so on. Under normal circumstances, most of these signaling pathways control the self-renewal of hepatocytes and prevent injuries; however, when these signaling pathways are abnormally activated, normal hepatocytes may transform into hepatoma cells (61). In previous experiments, we obtained evidence that SPINK13 can inhibit liver cancer, but the mechanism was not clear. By transcriptome sequencing, we identified 8 genes regulated by SPINK13 and involved in tumor regulation pathway. The gene CDKN1B is an inhibitor of cell cycle pathway; JUN and FOS are AP-1 transcription factors activated in JNK downstream signaling pathway in the MAPK signaling pathway; GADD45A, GADD45B, and BBC3 can be activated by p53; TNFRSF1A and NFKBIA are key elements of the IKK/NF-κB signaling pathway. These results indicate that SPINK13 inhibits the development of hepatocarcinoma cells mainly by regulating the JNK pathway, p53 pathway, and IKK/NF-κB pathway. This study provides a basis for further study on the mechanism of SPINK13.
Acknowledgments
Funding: All authors reported funding from
Footnote
Reporting Checklist: The authors have completed the MDAR reporting checklist. Available at https://dx.doi.org/10.21037/tcr-21-1928
Data Sharing Statement: Available at https://dx.doi.org/10.21037/tcr-21-1928
Conflicts of Interest: All authors have completed the ICMJE uniform disclosure form (available at https://dx.doi.org/10.21037/tcr-21-1928). All authors reported funding from 2020 Party Building-Ideological and Political Research Project, School of Medicine, Shanghai Jiaotong University (DJ2035). The authors have no other conflicts of interest to declare.
Ethical Statement: The authors are accountable for all aspects of the work in ensuring that questions related to the accuracy or integrity of any part of the work are appropriately investigated and resolved. The study was conducted in accordance with the Declaration of Helsinki (as revised in 2013). Institutional ethical approval and informed consent were waived.
Open Access Statement: This is an Open Access article distributed in accordance with the Creative Commons Attribution-NonCommercial-NoDerivs 4.0 International License (CC BY-NC-ND 4.0), which permits the non-commercial replication and distribution of the article with the strict proviso that no changes or edits are made and the original work is properly cited (including links to both the formal publication through the relevant DOI and the license). See: https://creativecommons.org/licenses/by-nc-nd/4.0/.
References
- Wang XL, Li Y, Cheng XY, et al. Inhibitory effects of the recombinant human serine protease inhibitor Hespintor on the proliferation, migration and invasion of hepatocellular carcinoma cells. Oncol Rep 2015;33:729-36. [Crossref] [PubMed]
- Wei L, Lun Y, Zhou X, et al. Novel urokinase-plasminogen activator inhibitor SPINK13 inhibits growth and metastasis of hepatocellular carcinoma in vivo. Pharmacol Res 2019;143:73-85. [Crossref] [PubMed]
- Wacker SA, Houghtaling BR, Elemento O, et al. Using transcriptome sequencing to identify mechanisms of drug action and resistance. Nat Chem Biol 2012;8:235-7. [Crossref] [PubMed]
- Mutz KO, Heilkenbrinker A, Lönne M, et al. Transcriptome analysis using next-generation sequencing. Curr Opin Biotechnol 2013;24:22-30. [Crossref] [PubMed]
- Conesa A, Madrigal P, Tarazona S, et al. A survey of best practices for RNA-seq data analysis. Genome Biol 2016;17:13. [Crossref] [PubMed]
- Soneson C, Delorenzi M. A comparison of methods for differential expression analysis of RNA-seq data. BMC Bioinformatics 2013;14:91. [Crossref] [PubMed]
- Zhang ZH, Jhaveri DJ, Marshall VM, et al. A comparative study of techniques for differential expression analysis on RNA-Seq data. PLoS One 2014;9:e103207. [Crossref] [PubMed]
- Ashburner M, Ball CA, Blake JA, et al. Gene ontology: tool for the unification of biology. The Gene Ontology Consortium. Nat Genet 2000;25:25-9. [Crossref] [PubMed]
- Kanehisa M, Goto S. KEGG: kyoto encyclopedia of genes and genomes. Nucleic Acids Res 2000;28:27-30. [Crossref] [PubMed]
- Li X, Liu Z, Li J, et al. The progress of KEGG database and its application in bioinformatics. Pharm Biotechnol 2012;19:535-9.
- Sambrook J, Russell DW. Molecular Cloning: A Laboratory Manual. Beijing: China Science Publishing & Media Ltd., 2002.
- Connolly MA, Clausen PA, Lazar JG. Purification of RNA from animal cells using trizol. CSH Protoc 2006;2006:pdb.
- Rio DC, Ares M Jr, Hannon GJ, et al. Purification of RNA using TRIzol (TRI reagent). Cold Spring Harb Protoc 2010;2010:pdb.prot5439.
- Mortazavi A, Williams BA, McCue K, et al. Mapping and quantifying mammalian transcriptomes by RNA-Seq. Nat Methods 2008;5:621-8. [Crossref] [PubMed]
- Chen EA, Souaiaia T, Herstein JS, et al. Effect of RNA integrity on uniquely mapped reads in RNA-Seq. BMC Res Notes 2014;7:753. [Crossref] [PubMed]
- Rhee SY, Wood V, Dolinski K, et al. Use and misuse of the gene ontology annotations. Nat Rev Genet 2008;9:509-15. [Crossref] [PubMed]
- Dennis G Jr, Sherman BT, Hosack DA, et al. DAVID: Database for Annotation, Visualization, and Integrated Discovery. Genome Biol 2003;4:3. [Crossref] [PubMed]
- Bauer S, Gagneur J, Robinson PN. GOing Bayesian: model-based gene set analysis of genome-scale data. Nucleic Acids Res 2010;38:3523-32. [Crossref] [PubMed]
- Carbon S, Ireland A, Mungall CJ, et al. AmiGO: online access to ontology and annotation data. Bioinformatics 2009;25:288-9. [Crossref] [PubMed]
- Kanehisa M, Goto S, Hattori M, et al. From genomics to chemical genomics: new developments in KEGG. Nucleic Acids Res 2006;34:D354-7. [Crossref] [PubMed]
- Kanehisa M, Goto S, Sato Y, et al. Data, information, knowledge and principle: back to metabolism in KEGG. Nucleic Acids Res 2014;42:D199-205. [Crossref] [PubMed]
- Goto S, Nishioka T, Kanehisa M. LIGAND database for enzymes, compounds and reactions. Nucleic Acids Res 1999;27:377-9. [Crossref] [PubMed]
- Hashimoto K, Goto S, Kawano S, et al. KEGG as a glycome informatics resource. Glycobiology 2006;16:63R-70R. [Crossref] [PubMed]
- Galperin MY, Fernández-Suárez XM. The 2012 Nucleic Acids Research Database Issue and the online Molecular Biology Database Collection. Nucleic Acids Res 2012;40:D1-8. [Crossref] [PubMed]
- Kanehisa M, Goto S, Furumichi M, et al. KEGG for representation and analysis of molecular networks involving diseases and drugs. Nucleic Acids Res 2010;38:D355-60. [Crossref] [PubMed]
- Muto A, Kotera M, Tokimatsu T, et al. Modular architecture of metabolic pathways revealed by conserved sequences of reactions. J Chem Inf Model 2013;53:613-22. [Crossref] [PubMed]
- Snel B, Lehmann G, Bork P, et al. STRING: a web-server to retrieve and display the repeatedly occurring neighbourhood of a gene. Nucleic Acids Res 2000;28:3442-4. [Crossref] [PubMed]
- Schwartz AS, Yu J, Gardenour KR, et al. Cost-effective strategies for completing the interactome. Nat Methods 2009;6:55-61. [Crossref] [PubMed]
- Franceschini A, Szklarczyk D, Frankild S, et al. STRING v9.1: protein-protein interaction networks, with increased coverage and integration. Nucleic Acids Res 2013;41:D808-15. [Crossref] [PubMed]
- Lin X, Yang Y, Guo Y, et al. PTTG1 is involved in TNF-α-related hepatocellular carcinoma via the induction of c-myc. Cancer Med 2019;8:5702-15. [Crossref] [PubMed]
- Parte S, Virant-Klun I, Patankar M, et al. PTTG1: a Unique Regulator of Stem/Cancer Stem Cells in the Ovary and Ovarian Cancer. Stem Cell Rev Rep 2019;15:866-79. [Crossref] [PubMed]
- Ma K, Sun X, Ma L, et al. Expression of Serum PTTG1 in Laryngeal Carcinoma and Its Correlation to Prognosis. Clin Exp Otorhinolaryngol 2020;13:64-8. [Crossref] [PubMed]
- Liu XL, Liu HM, Han N, et al. PCAT1 promotes the proliferative and migratory potentials of ovarian cancer via targeting NEK2. Eur Rev Med Pharmacol Sci 2019;23:8239-48. [PubMed]
- Lu H, Yao B, Wen X, et al. FBXW7 circular RNA regulates proliferation, migration and invasion of colorectal carcinoma through NEK2, mTOR, and PTEN signaling pathways in vitro and in vivo. BMC Cancer 2019;19:918. [Crossref] [PubMed]
- Kursula P, Sikkilä H, Fukao T, et al. High resolution crystal structures of human cytosolic thiolase (CT): a comparison of the active sites of human CT, bacterial thiolase, and bacterial KAS I. J Mol Biol 2005;347:189-201. [Crossref] [PubMed]
- Song XQ, Fukao T, Yamaguchi S, et al. Molecular cloning and nucleotide sequence of complementary DNA for human hepatic cytosolic acetoacetyl-coenzyme A thiolase. Biochem Biophys Res Commun 1994;201:478-85. [Crossref] [PubMed]
- Vock C, Döring F, Nitz I. Transcriptional regulation of HMG-CoA synthase and HMG-CoA reductase genes by human ACBP. Cell Physiol Biochem 2008;22:515-24. [Crossref] [PubMed]
- Lu Y, Dollé ME, Imholz S, et al. Multiple genetic variants along candidate pathways influence plasma high-density lipoprotein cholesterol concentrations. J Lipid Res 2008;49:2582-9. [Crossref] [PubMed]
- Skibola CF, Bracci PM, Halperin E, et al. Polymorphisms in the estrogen receptor 1 and vitamin C and matrix metalloproteinase gene families are associated with susceptibility to lymphoma. PLoS One 2008;3:e2816. [Crossref] [PubMed]
- Wapenaar MC, Monsuur AJ, Poell J, et al. The SPINK gene family and celiac disease susceptibility. Immunogenetics 2007;59:349-57. [Crossref] [PubMed]
- Cai S, Zhang P, Dong S, et al. Downregulation of SPINK13 Promotes Metastasis by Regulating uPA in Ovarian Cancer Cells. Cell Physiol Biochem 2018;45:1061-71. [Crossref] [PubMed]
- Ma L, Yu H, Ni Z, et al. Spink13, an epididymis-specific gene of the Kazal-type serine protease inhibitor (SPINK) family, is essential for the acrosomal integrity and male fertility. J Biol Chem 2013;288:10154-65. [Crossref] [PubMed]
- Xu WH, Shi SN, Wang J, et al. The Role of Serine Peptidase Inhibitor Kazal Type 13 (SPINK13) as a Clinicopathological and Prognostic Biomarker in Patients with Clear Cell Renal Cell Carcinoma. Med Sci Monit 2019;25:9458-70. [Crossref] [PubMed]
- Jiao S, Liu BC, Liu HF. Advance of research on role of c-Jun in tumorigenesis. Zhonghua Lao Dong Wei Sheng Zhi Ye Bing Za Zhi 2008;26:253-5. [PubMed]
- Eferl R, Wagner EF. AP-1: a double-edged sword in tumorigenesis. Nat Rev Cancer 2003;3:859-68. [Crossref] [PubMed]
- Bakiri L, Hamacher R, Graña O, et al. Liver carcinogenesis by FOS-dependent inflammation and cholesterol dysregulation. J Exp Med 2017;214:1387-409. [Crossref] [PubMed]
- Wu Q, Wu W, Fu B, et al. JNK signaling in cancer cell survival. Med Res Rev 2019;39:2082-104. [Crossref] [PubMed]
- Guo X, Guo W, Dong Z. The progress of studies on the role of GADD45 in anti-tumor treatment. Tumor 2015;35:1051-6.
- Seewoo V, Yang W, Du H, et al. The different induction mechanisms of growth arrest DNA damage inducible gene 45 β in human hepatoma cell lines. Chemotherapy 2012;58:165-74. [Crossref] [PubMed]
- Ma C, Chen H. Relationship between signal transduction of tumor necrosis factor receptor 1 and tumor. Journal of Shantou University Medical College 2008;58-60.
- Linnewiel-Hermoni K, Motro Y, Miller Y, et al. Carotenoid derivatives inhibit nuclear factor kappa B activity in bone and cancer cells by targeting key thiol groups. Free Radic Biol Med 2014;75:105-20. [Crossref] [PubMed]
- Bredel M, Scholtens DM, Yadav AK, et al. NFKBIA deletion in glioblastomas. N Engl J Med 2011;364:627-37. [Crossref] [PubMed]
- Wang W, Ren G, Qi J, et al. Expression of NFKBIA in primary liver cancer and its significance. Shandong Medical Journal 2015;55:16-9.
- Huang DC, Strasser A. BH3-Only proteins-essential initiators of apoptotic cell death. Cell 2000;103:839-42. [Crossref] [PubMed]
- Vousden KH, Lu X. Live or let die: the cell's response to p53. Nat Rev Cancer 2002;2:594-604. [Crossref] [PubMed]
- Qiu W, Wang X, Leibowitz B, et al. PUMA-mediated apoptosis drives chemical hepatocarcinogenesis in mice. Hepatology 2011;54:1249-58. [Crossref] [PubMed]
- Kim MR, Jeong EG, Chae B, et al. Pro-apoptotic PUMA and anti-apoptotic phospho-BAD are highly expressed in colorectal carcinomas. Dig Dis Sci 2007;52:2751-6. [Crossref] [PubMed]
- Wang R, Wang X, Li B, et al. Tumor-specific adenovirus-mediated PUMA gene transfer using the survivin promoter enhances radiosensitivity of breast cancer cells in vitro and in vivo. Breast Cancer Res Treat 2009;117:45-54. [Crossref] [PubMed]
- Guan X, Chen L, Wang J. Protein profiling: a possible molecular mechanism to mislocalization and down-expression of p27(Kip1) in tumor cells. Med Hypotheses 2007;69:580-3. [Crossref] [PubMed]
- Slingerland J, Pagano M. Regulation of the cdk inhibitor p27 and its deregulation in cancer. J Cell Physiol 2000;183:10-7. [Crossref] [PubMed]
- Liu Q, Liu J. The progress of studies on molecular signaling pathway related to hepatocellular carcinoma. World Chinese Journal of Digestology 2014;22:59-66. [Crossref]
(English Language Editor: J. Jones)