Characterization of diagnostic and prognostic significance of cell cycle-linked genes in hepatocellular carcinoma
Introduction
Hepatocellular carcinoma (HCC) is the most frequent histological type of liver cancer, that accounts for 75–85% of all types of primary liver cancer (1). On the basis of the 2018 global cancer statistics, HCC incidence ranks sixth, and the mortality rate ranks third (1). Multiple risk factors promoted occurrence and progression of HCC, mainly including hepatitis virus infection, aflatoxin exposure, alcohol abuse, and metabolic syndrome (2,3). At present, HCC treatment approaches include non-pharmacological and pharmacological therapies, consisting of transcatheter arterial chemoembolization (TACE), surgical resection, small molecule targeted drugs, liver transplantation, ablation, and monoclonal antibodies (4). Although the level of therapeutic approaches of HCC has advanced in recent years, long-term prognosis of individuals with HCC remains unsatisfactory due to late diagnosis and recurrence (5). Therefore, exploring the underlying mechanism contributing to tumorigenesis of HCC is mandatory to identify novel biomarkers for early diagnosis and targeted therapy.
Increasing evidence indicated that cell cycle dysregulation is strongly associated with tumor initiation and progression (6). The cell cycle is tightly regulated by cyclins and associated regulatory proteins (7,8). Currently, accumulated studies suggested that thorough investigation of biological process of cell cycle may improve survival prognosis and treatment effects for HCC patients. Xiao et al. reported that down-regulation of centromere protein M (CENPM) arrested cell cycle, as well as promoted apoptosis of HCC cells, inhibiting HCC migration and invasion (9). Liu et al. found that tanshinone (TA) I can induce G0/G1 arrest to exert anti-cancer effects in HCC through inhibiting cyclin D expression (10). Zeng et al. demonstrated that CCT6A depletion repressed HCC cell growth via repressing G1-to-S transition, as it downregulated cyclin D expression (11). Therefore, constructing a prediction model on the basis of cell cycle-linked genes may help estimate the prognosis of individuals with HCC.
Herein, we first obtained transcriptome along with the matching clinicopathological data of individuals with HCC from The Cancer Genome Atlas (TCGA) and International Cancer Genome Consortium (ICGC) data resources. Then, TCGA cohort data were employed to create an estimation model on the basis of the cell cycle-linked genes, and ICGC cohort data were employed to validate the prediction model. Furthermore, relationship of the risk signature with the biological function, as well as clinicopathological features, was analyzed.
We present the following article in accordance with the TRIPOD reporting checklist (available at https://dx.doi.org/10.21037/tcr-21-1145).
Methods
Data processing
The transcriptome, somatic mutation, along with the matching clinical data of 371 individuals with HCC were abstracted from TCGA (training cohort) (https://portal.gdc.cancer.gov/). Besides, the copy number variation (CNV) dataset was obtained from UCSC genome browser (https://xena.ucsc.edu/). Similarly, transcriptome profiling along with the matching clinicopathological information of 231 HCC samples were abstracted from the ICGC (https://dcc.icgc.org/releases) (validation cohort). A total of 1,875 cell cycle-linked genes were collected from a previous study. In this study, we further deleted 6 samples from the 371 samples from the TCGA database, including 5 samples with a survival time of 0 days and 1 sample with a missing survival time. This is because before the Lasso regression analysis, the survival time of these samples did not meet the prerequisites for the Lasso regression analysis. Therefore, a total of 365 samples from the TCGA database were used as the training cohort. The study was conducted in accordance with the Declaration of Helsinki (as revised in 2013).
Identification of cell cycle-linked genes for constructing a prediction model
First, 1,875 cell cycle-linked genes were uploaded into the Search Tool for Recurring Instances of Neighboring Genes (STRING) website (https://string-db.org/) to analyze the protein-protein interaction (PPI) network between genes. Meanwhile, according to each gene’s number of adjacent nodules from high to low, the top 50 genes were screened for subsequent analysis. Then, differentially expressed genes (DEGs) among the 50 genes between HCC and neighboring non-malignant tissues were screened with the ‘limma’ package in R. The screening criteria were |log2fold change (log2FC)| >1 along with false discovery rate (FDR) <0.05. Moreover, univariate Cox regression analyses were applied to identify genes which could independently assess the prognosis of patients (P<0.001) for the overall survival (OS) of individuals with HCC from the 50 genes, and further intersected with DEGs to obtain DEGs with independent prognostic value using ‘Venn’ package in R. Additionally, the somatic mutation and CNV information of DEGs with independent prognostic value were analyzed using R software.
Creation and verification of an estimation model using cell cycle-linked genes
DEGs with independent prognostic value were subjected to lasso regression assessment using ‘glmnet’ package to design a prediction model. Then, risk score of each sample was computed as below:
Where β designates the regression coefficient, and Exp designates the levels of genes. On the basis of the median risk score, TCGA cohort samples were stratified to two risk groups: high and low. Principal component analysis (PCA) and t-distributed stochastic neighbor embedding (t-SNE) analyses were respectively conducted using ‘Rtsne’ and ‘prcomp’ functions to assess the clustering ability of prognostic model. Furthermore, Kaplan-Meier survival curve along with the receiver operating characteristic (ROC) curve were respectively drawn using ‘survival’ and ‘survival ROC’ packages to explore the accuracy of prediction model. Besides, according to the same calculation formula of the risk score, ICGC cohort samples were employed to verify the estimation capacity of prediction model.
Assessment of independent prognostic value of cell cycle-linked risk genes
To estimate the performance of the risk signature compared to conventional clinicopathologic features, were conducted univariate along with multivariate Cox regression analyses in R software.
Single-sample gene set enrichment analysis (ssGSEA)
To investigate the relationship of the risk signature with immune status, differences in both infiltrations of 16 kinds of immune cells, as well as the functions and pathways of 13 immune-related cells between the two risk groups were compared using ‘GSVA’ package in R.
Investigating the relationship of somatic mutation with survival outcomes of HCC patients, risk signature in the TCGA database
Tumor mutation burden (TMB) is the total number of mutations present in a tumor specimen (12). In this research, Kaplan-Meier survival assessment was employed to examine the relationship of HCC patients’ survival outcomes with TMB, as well as TMB combined with risk signature. Additionally, mutation frequency differences of the top 30 genes between the two risk groups were analyzed with R software.
Exploring the differences in the expression levels of immune checkpoint molecules between the two risk groups in the TCGA database
Immune checkpoint molecules influence the efficacy of immunotherapy (13). The levels of three immune checkpoint molecules, consisting of PD-1, PD-L1, and CTLA-4, between the two risk groups were explored with R software.
Examining the relationship between subgroups of patients with different clinicopathological features and risk signature in the TCGA database
According to different clinicopathological features (age, gender, and TNM-stage), we first divided patients in TCGA cohort into different subgroups. Then, we analyzed the differences in risk scores between different subgroups. In addition, we estimated the prognostic significance of risk signature in different subgroups using Kaplan-Meier analysis.
Statistical analyses
R software (V.4.0.2) was utilized for data analysis. The significance between the two groups was identified using Wilcox test. The survival time differences between the two risk groups were estimated using Kaplan-Meier curves and log-rank test. The area under the ROC curve (AUC) was calculated to assess the risk signature’s accuracy. Independent factors of OS were determined using univariate along with multivariate Cox regression analyses. The ssGSEA scores of immune cells or functions between high- and low-risk groups were compared using Mann-Whitney test. P<0.05 was the cut-off of statistical significance.
Results
Identification of cell cycle-related genes for constructing a prediction model
Figure 1 illustrates the workflow of data analysis (14). According to PPI network, we screened the top 50 cell cycle-linked genes in the TCGA dataset (Figure 2A). The Venn diagram shows 30 cell cycle-linked genes, which showed differential expression between HCC and neighboring non-malignant tissues and the potential to be independent prognostic biomarker (Figure 2B). Meanwhile, the heatmap displayed differential expression levels of 30 cell cycle-linked genes in HCC and neighboring non-malignant tissues (Figure 2C). The forest map showed that 30 cell cycle-linked genes were independent predictors of HCC prognosis [hazard ratio (HR) >1; P<0.001] (Figure 2D). Besides, the CNV analysis indicated that CNV deletion frequency was more common among 30 cell cycle-linked genes (Figure 2E). Based on the TCGA database, the somatic mutation analysis illustrated that mutation frequency of 30 cell cycle-linked genes was very low (Figure 2F).
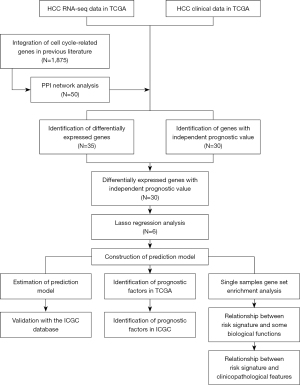
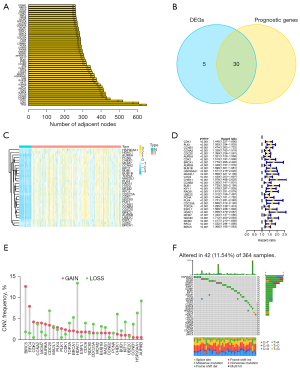
Constructing a prediction model using TCGA cohort
The 30 DEGs with independent prognostic value were conducted lasso regression analysis. Six genes (PLK1, CDC20, HSP90AA1, CHEK1, HDAC1, and NDC80) were selected to construct a prediction model. Risk scores were computed as below:
Evaluating the predictive ability of the prediction model using TCGA cohort
Based on the median risk score, all TCGA data set samples were stratified into high- (N=182) and low-risk (N=183) groups (Figure 3A). The risk plot indicated that high-risk score patients exhibited shorter survival times in contrast with those with low-risk scores (Figure 3B). Besides, PCA along with t-SNE tests uncovered that the prediction model exhibited good clustering ability (Figure 3C,3D). Additionally, Kaplan-Meier analysis demonstrated that high-risk scores correlated with poorer OS (P=7.232e-05) (Figure 3E). The AUC values were determined using ROC curve analysis (0.737 at 1 year, 0.712 at 2 years, and 0.683 at 3 years) (Figure 3F).
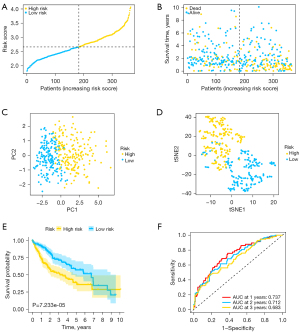
Validating the performance of the prediction model using ICGC cohort
To test the predictive ability of the estimation model, ICGC data set samples were utilized for validation. The risk score of each sample was computed on the basis of the same formula from TCGA cohort. ICGC data set samples were then stratified into high- (N=115) and low-risk (N=116) groups (Figure 4A). Similarly, patients with high-risk scores had worse outcomes in contrast with those with low-risk scores (Figure 4B). Likewise, PCA and t-SNE analyses showed that prediction model could well separate patients in different groups into two regions (Figure 4C,4D). Similarly, Kaplan-Meier data validated that patients with high-risk scores had a poorer survival prognosis in contrast with those with low-risk scores (P=1.403e-04) (Figure 4E). Besides, the AUC values were 0.742 at 1 year, 0.743 at 2 years, and 0.741 at 3 years, respectively (Figure 4F).
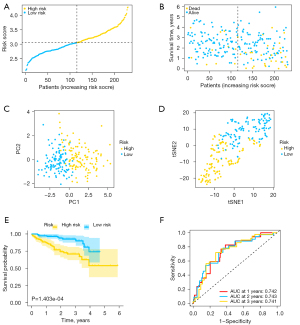
Assessment of independent predictive significance of cell cycle-linked risk score signature
Univariate along with multivariate Cox regression analyses were employed to assess the independent predictive significance of risk signature for OS in TCGA, as well as ICGC cohorts. For TCGA cohort, univariate analysis indicated that TNM stage and risk signature were independent predictors of OS (Figure 5A). Moreover, multivariate analysis confirmed that TNM stage along with risk signature remained independent predictive factors (Figure 5B). Besides, ICGC cohort analysis results also demonstrated that TNM stage along with risk signature were independent predictive indicators of OS (Figure 5C,5D).
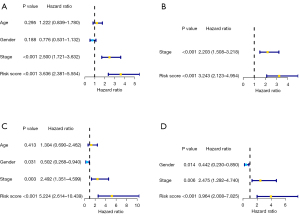
The association between the immune status and risk signature
In this study, the infiltration of 13 immune cells, as well as the activity of 16 immune-linked functions or cascades were used to represent immune status and were quantified based on ssGSEA strategy. TCGA cohort patients were employed to explore the relationship of immune status with the risk signature, and ICGC cohort patients were used for validation. The scores of immune cell invasion showed that the scores of activated dendritic cells (aDCs), inhibited dendritic cells (iDCs), macrophages, and T helper 2 (Th2) cells were higher in high-risk group in contrast with those in low-risk group, whereas natural killer (NK) cells were lower in the high-risk group in contrast with those in low-risk group (Figure 6A,6B). Furthermore, the immune-linked functions or cascades analysis indicated that type II interferon (IFN) response was lower in the high-risk group in contrast with that in the low-risk group (Figure 6C,6D). These results confirmed that risk signature could better reflect the immune status in HCC.
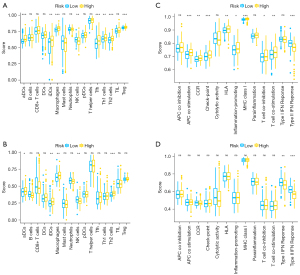
The relationship between somatic mutation and survival outcomes, risk signature in the TCGA database
Kaplan-Meier analysis illustrated that patients with high TMB exhibited shorter survival times in contrast with those with low TMB (Figure 7A). Moreover, patients with high TMB and high-risk scores had the worst survival outcomes (Figure 7B). Besides, TP53 is the most commonly mutated gene, and mutation frequency of TP53 was remarkably higher in the high-risk group (43%) in contrast with that in the low-risk group (12%) (Figure 7C,7D).
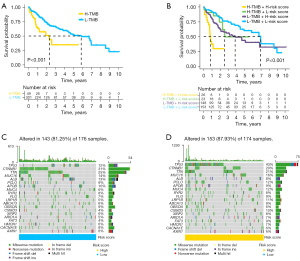
The differences in the expression of immune checkpoint molecules between high- and low-risk groups in the TCGA database
The box plots showed that expression levels of PD-1 (P=8.1e-11), PD-L1 (P=0.044), and CTLA-4 (P=1e-11) were remarkably higher in high-risk group in contrast with those in the low-risk group (Figure 8A-8C).
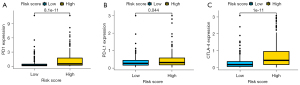
The relationship between subgroups with different clinicopathological features and risk signature in the TCGA database
In this study, we found that patients with age ≤65 or with stage III–IV exhibited remarkably higher risk score in contrast with corresponding those with age >65 or with stage I–II (Figure 9A-9C). Additionally, there was significant differences between high- and low-risk groups for patients with age >65 (P≤0.001) or male (P<0.001) or stage I–II (P=0.003) or stage III–IV (P=0.014) (Figure 10A-10C).
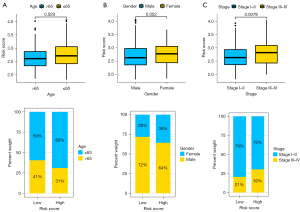
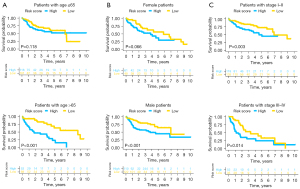
Discussion
Herein, we not only analyzed the differential expression of 50 cell cycle-linked genes in HCC and neighboring non-malignant tissues but also assessed the relationship of the expression of these genes with OS of individuals with HCC. Besides, a prediction model using six cell cycle-linked genes was built and validated based on public database. Importantly, this prediction model can not only predict the survival prognosis and biological function of HCC patients well but also provide novel biomarkers for precision treatment.
The cell cycle dysregulation is one of the most significant features of tumors, and the elucidation of the underlying mechanisms is expected to improve tumor therapy development (15). Recently, with the rapid development of high-throughput sequencing technology and the release of public databases, it is possible to systematically explore the expression and function of cell cycle-linked genes in HCC tissues. For instance, a study based on bioinformatics reported that MITD1, involved in cell cycle regulation, can be employed as an independent predictive factor for individuals with HCC, and MITD1 expression is associated with clinicopathological characteristics and natural killer cell infiltration (16). Additionally, increasing findings using bioinformatics demonstrated that DEGs between HCC and neighboring non-malignant tissues were remarkably enriched in cell cycle pathway (17-19). Therefore, the utility of bioinformatics to study the function of cell cycle-linked genes in HCC pathogenesis is necessary for improving the therapeutic effect of HCC.
Furthermore, we constructed an estimation model using six cell cycle-linked genes, including PLK1, CDC20, HSP90AA1, CHEK1, HDAC1, and NDC80. For PLK1, several studies indicated that PLK1 expression was higher in HCC tissues in contrast with that in neighboring non-malignant tissues, and PLK1 overexpression was linked to poor prognosis in individuals with HCC (20-22). Moreover, PLK1 has proven to be a promising therapeutic target, and its knockout can effectively repress the proliferation and migration of HCC cells (23,24). Deng et al. found that PLK1 inhibition induces mitotic arrest at G2/M phase, leading to HCC cell apoptosis (25). Numerous findings based on bioinformatics confirmed that CDC20 might have a critical regulatory role in the occurrence and progression of HCC (26-28). Moreover, increased CDC20 was linked to poor prognosis of individuals with HCC (29,30). Additionally, Liu et al. stated that CDC20 inhibition could activate HCC cell apoptosis and autophagy (31). For HSP90AA1, Chen et al. illustrated that HSP90AA1 expression was remarkably increased in HCC tissues, and high HSP90AA1 expression is an indicator of unfavorable prognosis in individuals with HCC (32). Shi et al. indicated that preventing HSP90AA1 degradation can activate c-MYC, leading to initiation and progression of HCC (33). Regarding CHEK1 (also known as CHK1), Gong et al. illustrated that CHEK1 was remarkably up-regulated in HCC tissues and demonstrated that CHEK1 inhibition could diminish proliferative and invasive potentials of HCC cells (34). Another study has also confirmed CHEK1 significance as a potential therapeutic target for HCC (35). They found that interferon regulatory factor 1 (IRF-1) can inhibit CHEK1 expression by inducing miR-195, contributing to apoptosis of HCC cells and infiltration of NK cells (35). For HDAC1, a previous study demonstrated that HDAC1 was up-regulated in HCC tissues, and high expression of HDAC1 was linked to poor tumor differentiation, contributing to tumor progression (36). In addition, two studies indicated that HDAC1 upregulation could facilitate HCC development by promoting epithelial-mesenchymal transition (EMT) (37,38). Besides, some findings demonstrated that HDAC1 inhibition could repress growth of HCC cells and trigger cell cycle arrest and apoptosis in HCC cells (39-42). For NDC80, Ju et al. documented that NDC80 expression is remarkably increased in HCC tissues in contrast with that in neighboring non-malignant tissues, and NDC80 silencing can inhibit proliferation and contribute to apoptosis for HCC cells (43). Liu et al. also manifested that NDC80 knockdown can inhibit growth of HCC cells (44). In general, the expression levels of these six genes (PLK1, CDC20, HSP90AA1, CHEK1, HDAC1, and NDC80) are remarkably increased in HCC tissues, and the up-regulated expression was linked to poor prognosis of individuals with HCC.
Currently, TNM staging system has been widely used to assess survival prognosis and guide treatment in HCC. However, since TNM staging system cannot consider the heterogeneity of patients at the same stage, it cannot always reliably predict a patient’s survival. The occurrence and development of tumors are closely linked to disorders of some biological functions. Therefore, exploring the function of linked genes involved in these biological processes is expected to improve prediction accuracy and therapeutic effects in the future. Herein, we constructed an estimation model using cell cycle-linked genes. We found that this cell cycle-linked risk signature can make a good prediction of prognosis and disease progression of individuals with HCC, even for subgroups of patients with different clinicopathological features. Importantly, this risk signature also can reflect some important status of biological functions, such as immune-linked function, gene mutation frequency, and expression of immune checkpoint molecules, which helps clinicians adopt more accurate and personalized treatments for individuals with HCC.
Based on ssGSEA results, we discovered that infiltration of tumor-associated macrophages in the high-risk group was dramatically higher in contrast with that in the low-risk group. In recent years, increasing basic and clinical studies have illustrated that the number and phenotype of tumor-linked macrophages infiltrated in tumor tissues are closely linked to the prognosis of tumor patients (45). As the main component of the tumor microenvironment, tumor-linked macrophages secrete numerous cytokines to repress the body’s adaptive immune response, accelerate the growth of tumor cells, and promote tumor-linked angiogenesis and tumor metastasis (46). Yao et al. found that HCC cells cultured with M2-like macrophages exhibit enhanced metastatic ability and upregulation of EMT-linked markers via TLR4/STAT3 signaling cascade (47). In addition, a recent study reported a new type of subpopulation in tumor-associated macrophages (48). These special cells can dramatically promote tumor angiogenesis because of expressing tyrosine-protein kinase receptor Tie-2 (also referred to as angiopoietin-1 receptor), which can bind to all known angiopoietins (49,50).
Additionally, we found that TP53 mutation is the most common type of mutation, and the frequency of TP53 mutation is higher in high-risk groups in contrast with that in low-risk groups. A previous study indicated that individuals with HCC with TP53 mutation are correlated with worse clinical stage and prognosis (51). Long et al. reported that TP53 mutation led to downregulation of immune response in HCC, correlating higher infiltration of immunosuppressive cells and immune checkpoint molecule expression (52). Interestingly, we also found that the expression levels of immune checkpoint molecules were higher in high-risk group in contrast with that those in low-risk group, consistent with a previous study suggesting that tumor cells can suppress T cell-mediated immune responses by up-regulating immune checkpoint molecules, thereby evading immune attacks and promoting metastasis (53). Gao et al. demonstrated that PD-L1 overexpression was dramatically associated with progression and postoperative recurrence (54). Another study revealed that adding anti-CTLA-4 antibody can further enhance antitumor effect of tumor-specific T cells (55). These findings further support that expression levels of immune checkpoint molecules are correlated with survival prognosis and therapeutic effect of HCC.
As far as we know, this is the first study to use cell cycle-linked genes to create an estimation model for prognosis of individuals with HCC. Meanwhile, the prediction model had good predictive ability. Besides, this risk signature can be utilized not only as an independent prognostic factor for OS but also to assess differences in biological function status between different groups. Notably, six cell cycle-linked genes are expected to be novel biomarkers for HCC diagnosis, as well as treatment. Nevertheless, this study has some limitations. Firstly, all findings were retrospectively obtained based on a public database, which required further prospective verification. Secondly, the underlying function of the six genes requires additional validation via experimental studies. Thirdly, identifying these six genes comes from comparing the DEGs between HCC and non-malignant tissues, and the influence of different HCC pathogenic factors on the expression of cell cycle-linked genes needs to be further explored.
Conclusions
We established a prognostic model using six cell cycle-linked genes for predicting HCC prognosis, implying good predictive ability and proved to be an independent predictor of OS. Hence, the six genes were expected to be novel biomarkers for HCC diagnosis and treatment.
Acknowledgments
We thank Home for Researchers editorial team (www.home-for-researchers.com) for editing this manuscript.
Funding:
Footnote
Reporting Checklist: The authors have completed the TRIPOD reporting checklist. Available at https://dx.doi.org/10.21037/tcr-21-1145
Peer Review File: Available at https://dx.doi.org/10.21037/tcr-21-1145
Conflicts of Interest: All authors have completed the ICMJE uniform disclosure form (available at https://dx.doi.org/10.21037/tcr-21-1145). The authors have no conflicts of interest to declare.
Ethical Statement: The authors are accountable for all aspects of the work in ensuring that questions related to the accuracy or integrity of any part of the work are appropriately investigated and resolved. The study was conducted in accordance with the Declaration of Helsinki (as revised in 2013).
Open Access Statement: This is an Open Access article distributed in accordance with the Creative Commons Attribution-NonCommercial-NoDerivs 4.0 International License (CC BY-NC-ND 4.0), which permits the non-commercial replication and distribution of the article with the strict proviso that no changes or edits are made and the original work is properly cited (including links to both the formal publication through the relevant DOI and the license). See: https://creativecommons.org/licenses/by-nc-nd/4.0/.
References
- Bray F, Ferlay J, Soerjomataram I, et al. Global cancer statistics 2018: GLOBOCAN estimates of incidence and mortality worldwide for 36 cancers in 185 countries. CA Cancer J Clin 2018;68:394-424. [Crossref] [PubMed]
- El-Serag HB, Rudolph KL. Hepatocellular carcinoma: epidemiology and molecular carcinogenesis. Gastroenterology 2007;132:2557-76. [Crossref] [PubMed]
- Welzel TM, Graubard BI, Zeuzem S, et al. Metabolic syndrome increases the risk of primary liver cancer in the United States: a study in the SEER-Medicare database. Hepatology 2011;54:463-71. [Crossref] [PubMed]
- Chen Z, Xie H, Hu M, et al. Recent progress in treatment of hepatocellular carcinoma. Am J Cancer Res 2020;10:2993-3036. [PubMed]
- Finn RS, Zhu AX, Farah W, et al. Therapies for advanced stage hepatocellular carcinoma with macrovascular invasion or metastatic disease: a systematic review and meta-analysis. Hepatology 2018;67:422-35. [Crossref] [PubMed]
- Stein GS, van Wijnen AJ, Stein JL, et al. An architectural perspective of cell-cycle control at the G1/S phase cell-cycle transition. J Cell Physiol 2006;209:706-10. [Crossref] [PubMed]
- Wang XQ, Liu YK, Qing C, et al. A review of the effectiveness of antimitotic drug injections for hypertrophic scars and keloids. Ann Plast Surg 2009;63:688-92. [Crossref] [PubMed]
- Fang JS, Coon BG, Gillis N, et al. Shear-induced Notch-Cx37-p27 axis arrests endothelial cell cycle to enable arterial specification. Nat Commun 2017;8:2149. [Crossref] [PubMed]
- Xiao Y, Najeeb RM, Ma D, et al. Upregulation of CENPM promotes hepatocarcinogenesis through mutiple mechanisms. J Exp Clin Cancer Res 2019;38:458. [Crossref] [PubMed]
- Liu X, Liu J. Tanshinone I induces cell apoptosis by reactive oxygen species-mediated endoplasmic reticulum stress and by suppressing p53/DRAM-mediated autophagy in human hepatocellular carcinoma. Artif Cells Nanomed Biotechnol 2020;48:488-97. [Crossref] [PubMed]
- Zeng G, Wang J, Huang Y, et al. Overexpressing CCT6A contributes to cancer cell growth by affecting the G1-to-S phase transition and predicts a negative prognosis in hepatocellular carcinoma. Onco Targets Ther 2019;12:10427-39. [Crossref] [PubMed]
- Shrestha R, Prithviraj P, Anaka M, et al. Monitoring immune checkpoint regulators as predictive biomarkers in hepatocellular carcinoma. Front Oncol 2018;8:269. [Crossref] [PubMed]
- Xiong Y, Xiong Z, Cao H, et al. Multi-dimensional omics characterization in glioblastoma identifies the purity-associated pattern and prognostic gene signatures. Cancer Cell Int 2020;20:37. [Crossref] [PubMed]
- Wang J, Han K, Zhang C, et al. Identification and validation of ADME genes as prognosis and therapy markers for hepatocellular carcinoma patients. Biosci Rep 2021;41:BSR20210583. [Crossref] [PubMed]
- Malumbres M, Barbacid M. To cycle or not to cycle: a critical decision in cancer. Nat Rev Cancer 2001;1:222-31. [Crossref] [PubMed]
- Shen H, Wang Z, Ren S, et al. Prognostic biomarker MITD1 and its correlation with immune infiltrates in hepatocellular carcinoma (HCC). Int Immunopharmacol 2020;81:106222. [Crossref] [PubMed]
- Craske MG, Street L, Barlow DH. Instructions to focus upon or distract from internal cues during exposure treatment of agoraphobic avoidance. Behav Res Ther 1989;27:663-72. [Crossref] [PubMed]
- Shen S, Kong J, Qiu Y, et al. Identification of core genes and outcomes in hepatocellular carcinoma by bioinformatics analysis. J Cell Biochem 2019;120:10069-81. [Crossref] [PubMed]
- Wang Z, Pan L, Guo D, et al. A novel five-gene signature predicts overall survival of patients with hepatocellular carcinoma. Cancer Med 2021;10:3808-21. [Crossref] [PubMed]
- He ZL, Zheng H, Lin H, et al. Overexpression of polo-like kinase1 predicts a poor prognosis in hepatocellular carcinoma patients. World J Gastroenterol 2009;15:4177-82. [Crossref] [PubMed]
- Sun W, Su Q, Cao X, et al. High expression of polo-like kinase 1 is associated with early development of hepatocellular carcinoma. Int J Genomics 2014;2014:312130. [Crossref] [PubMed]
- Mok WC, Wasser S, Tan T, et al. Polo-like kinase 1, a new therapeutic target in hepatocellular carcinoma. World J Gastroenterol 2012;18:3527-36. [Crossref] [PubMed]
- Xu L, Zhu Y, Shao J, et al. Dasatinib synergises with irinotecan to suppress hepatocellular carcinoma via inhibiting the protein synthesis of PLK1. Br J Cancer 2017;116:1027-36. [Crossref] [PubMed]
- Zheng DW, Xue YQ, Li Y, et al. Volasertib suppresses the growth of human hepatocellular carcinoma in vitro and in vivo. Am J Cancer Res 2016;6:2476-88. [PubMed]
- Deng Z, Chen G, Liu S, et al. Discovery of methyl 3-((2-((1-(dimethylglycyl)-5-methoxyindolin-6-yl)amino)-5-(trifluoro-methyl) pyrimidin-4-yl)amino)thiophene-2-carboxylate as a potent and selective polo-like kinase 1 (PLK1) inhibitor for combating hepatocellular carcinoma. Eur J Med Chem 2020;206:112697. [Crossref] [PubMed]
- Yang WX, Pan YY, You CG. CDK1, CCNB1, CDC20, BUB1, MAD2L1, MCM3, BUB1B, MCM2, and RFC4 may be potential therapeutic targets for hepatocellular carcinoma using integrated bioinformatic analysis. Biomed Res Int 2019;2019:1245072. [Crossref] [PubMed]
- Zhuang L, Yang Z, Meng Z. Upregulation of BUB1B, CCNB1, CDC7, CDC20, and MCM3 in tumor tissues predicted worse overall survival and disease-free survival in hepatocellular carcinoma patients. Biomed Res Int 2018;2018:7897346. [Crossref] [PubMed]
- Mi N, Cao J, Zhang J, et al. Identification of hub genes involved in the occurrence and development of hepatocellular carcinoma via bioinformatics analysis. Oncol Lett 2020;20:1695-708. [Crossref] [PubMed]
- Zhang X, Zhang X, Li X, et al. Connection between CDC20 expression and hepatocellular carcinoma prognosis. Med Sci Monit 2021;27:e926760. [Crossref] [PubMed]
- Yang G, Wang G, Xiong Y, et al. CDC20 promotes the progression of hepatocellular carcinoma by regulating epithelial-mesenchymal transition. Mol Med Rep 2021;24:483. [Crossref] [PubMed]
- Liu M, Zhang Y, Liao Y, et al. Evaluation of the antitumor efficacy of RNAi-mediated inhibition of CDC20 and heparanase in an orthotopic liver tumor model. Cancer Biother Radiopharm 2015;30:233-9. [Crossref] [PubMed]
- Chen W, Li G, Peng J, et al. Transcriptomic analysis reveals that heat shock protein 90α is a potential diagnostic and prognostic biomarker for cancer. Eur J Cancer Prev 2020;29:357-64. [Crossref] [PubMed]
- Shi W, Feng L, Dong S, et al. FBXL6 governs c-MYC to promote hepatocellular carcinoma through ubiquitination and stabilization of HSP90AA1. Cell Commun Signal 2020;18:100. [Crossref] [PubMed]
- Gong D, Feng PC, Ke XF, et al. Silencing long non-coding RNA LINC01224 inhibits hepatocellular carcinoma progression via microRNA-330-5p-induced inhibition of CHEK1. Mol Ther Nucleic Acids 2020;19:482-97. [Crossref] [PubMed]
- Yan Y, Zheng L, Du Q, et al. Interferon regulatory factor 1 (IRF-1) downregulates Checkpoint kinase 1 (CHK1) through miR-195 to upregulate apoptosis and PD-L1 expression in Hepatocellular carcinoma (HCC) cells. Br J Cancer 2021;125:101-11. [Crossref] [PubMed]
- Ler SY, Leung CH, Khin LW, et al. HDAC1 and HDAC2 independently predict mortality in hepatocellular carcinoma by a competing risk regression model in a Southeast Asian population. Oncol Rep 2015;34:2238-50. [Crossref] [PubMed]
- Zhou H, Xu J, Zhang C, et al. Aberrant histone deacetylase 1 expression upregulates vimentin expression via an NF-κB-dependent pathway in hepatocellular carcinoma. Oncol Lett 2019;18:339-47. [Crossref] [PubMed]
- Lei W, Zhang K, Pan X, et al. Histone deacetylase 1 is required for transforming growth factor-beta1-induced epithelial-mesenchymal transition. Int J Biochem Cell Biol 2010;42:1489-97. [Crossref] [PubMed]
- Zhang Y, Chen J, Wu SS, et al. HOXA10 knockdown inhibits proliferation, induces cell cycle arrest and apoptosis in hepatocellular carcinoma cells through HDAC1. Cancer Manag Res 2019;11:7065-76. [Crossref] [PubMed]
- Zhou H, Cai Y, Liu D, et al. Pharmacological or transcriptional inhibition of both HDAC1 and 2 leads to cell cycle blockage and apoptosis via p21Waf1/Cip1 and p19INK4d upregulation in hepatocellular carcinoma. Cell Prolif 2018;51:e12447. [Crossref] [PubMed]
- Sun TY, Xie HJ, Li Z, et al. miR-34a regulates HDAC1 expression to affect the proliferation and apoptosis of hepatocellular carcinoma. Am J Transl Res 2017;9:103-14. [PubMed]
- Buurman R, Sandbothe M, Schlegelberger B, et al. HDAC inhibition activates the apoptosome via Apaf1 upregulation in hepatocellular carcinoma. Eur J Med Res 2016;21:26. [Crossref] [PubMed]
- Ju LL, Chen L, Li JH, et al. Effect of NDC80 in human hepatocellular carcinoma. World J Gastroenterol 2017;23:3675-83. [Crossref] [PubMed]
- Liu B, Yao Z, Hu K, et al. ShRNA-mediated silencing of the Ndc80 gene suppress cell proliferation and affected hepatitis B virus-related hepatocellular carcinoma. Clin Res Hepatol Gastroenterol 2016;40:297-303. [Crossref] [PubMed]
- Lin Y, Xu J, Lan H. Tumor-associated macrophages in tumor metastasis: biological roles and clinical therapeutic applications. J Hematol Oncol 2019;12:76. [Crossref] [PubMed]
- Chen Y, Song Y, Du W, et al. Tumor-associated macrophages: an accomplice in solid tumor progression. J Biomed Sci 2019;26:78. [Crossref] [PubMed]
- Yao RR, Li JH, Zhang R, et al. M2-polarized tumor-associated macrophages facilitated migration and epithelial-mesenchymal transition of HCC cells via the TLR4/STAT3 signaling pathway. World J Surg Oncol 2018;16:9. [Crossref] [PubMed]
- Matsubara T, Kanto T, Kuroda S, et al. TIE2-expressing monocytes as a diagnostic marker for hepatocellular carcinoma correlates with angiogenesis. Hepatology 2013;57:1416-25. [Crossref] [PubMed]
- Lewis CE, Pollard JW. Distinct role of macrophages in different tumor microenvironments. Cancer Res 2006;66:605-12. [Crossref] [PubMed]
- Venneri MA, De Palma M, Ponzoni M, et al. Identification of proangiogenic TIE2-expressing monocytes (TEMs) in human peripheral blood and cancer. Blood 2007;109:5276-85. [Crossref] [PubMed]
- Takai A, Dang HT, Wang XW. Identification of drivers from cancer genome diversity in hepatocellular carcinoma. Int J Mol Sci 2014;15:11142-60. [Crossref] [PubMed]
- Long J, Wang A, Bai Y, et al. Development and validation of a TP53-associated immune prognostic model for hepatocellular carcinoma. EBioMedicine 2019;42:363-74. [Crossref] [PubMed]
- Xu F, Jin T, Zhu Y, et al. Immune checkpoint therapy in liver cancer. J Exp Clin Cancer Res 2018;37:110. [Crossref] [PubMed]
- Gao Q, Wang XY, Qiu SJ, et al. Overexpression of PD-L1 significantly associates with tumor aggressiveness and postoperative recurrence in human hepatocellular carcinoma. Clin Cancer Res 2009;15:971-9. [Crossref] [PubMed]
- Mizukoshi E, Nakamoto Y, Arai K, et al. Comparative analysis of various tumor-associated antigen-specific t-cell responses in patients with hepatocellular carcinoma. Hepatology 2011;53:1206-16. [Crossref] [PubMed]