Screening key prognostic factors and constructing survival prognostic risk prediction model based on ceRNA network in early lung adenocarcinoma
Introduction
Lung cancer is among the malignant tumors with the highest fatality rate. Since the 1970s, lung adenocarcinoma (LUAD) has become the most common pathological type of lung cancer (1). Radical surgery is the primary treatment for LUAD, but patients still have a high risk of metastasis and recurrence postoperatively. Moreover, the 5-year survival rate is still low (2). Therefore, it is urgent to study the mechanism of occurrence and development of LUAD and find potential key prognostic markers.
Aberrations in the RNA regulatory network are an essential factor in cancer occurrence and development. RNA regulations include signal transduction pathways at the mRNA transcription level and noncoding gene regulation at the post-transcriptional level (3).
Studies associated abnormal miRNA expression in all basic cell processes with carcinogenesis, such as proliferation, differentiation and death (4). miRNAs may be potential markers for cancer diagnosis, prognostic evaluation and treatment targets. Studies have shown that some specific miRNAs can predict the clinical outcome of patients with LUAD, such as miR-432, miR-210, miR-145 and miR-31 (5). Accumulating evidence indicates that lncRNAs play vital roles in the regulation of RNAs. In 2011, Salmena et al. (6) put forward the competitive endogenous hypothesis. mRNAs, LncRNAs and other noncoding RNAs can be regulated through the microRNA response elements (MRE). This competitive endogenous RNA (ceRNA) forms a huge RNA regulatory network.
High-throughput sequencing technology has led to the discovery of various new prognostic markers based on the gene level. Therefore, it is essential for patients and clinicians to establish an integrated RNA model related to LUAD prognoses. In our research, sequence data from 238 early LUAD samples and 18 normal samples were downloaded from The Cancer Genome Atlas (TCGA), including mRNAs, miRNAs and lncRNAs data. Using these, we explored early LUAD-specific miRNA and lncRNA expression. Then we constructed a ceRNA co-expression network of early LUAD. This ceRNA network can help clarify the functions of lncRNAs in early LUAD. With clinical data, we finally screened out optimized independent prognostic-related RNAs and constructed a prognostic risk prediction model for early-stage LUAD. We present the following article in accordance with the MDAR reporting checklist (available at https://dx.doi.org/10.21037/tcr-20-3273).
Methods
Data collection and preprocessing
We searched LUAD samples in the TCGA dataset (https://portal.gdc.cancer.gov/) and selected 256 samples. The study was conducted in accordance with the Declaration of Helsinki (as revised in 2013). These samples simultaneously detected mRNA and miRNA expression levels with the Illumina HiSeq 2000 RNA sequencing platform. The pathologic stage factors indicated they were all early-stage (Stage I) samples. We also downloaded each sample’s clinical data. In this dataset (training dataset), there are 18 normal samples and 238 tumor samples.
To verify our results, we used “lung adenocarcinoma, Homo sapiens” as keywords to filter a validation dataset in the NCBI GEO (7) (https://www.ncbi.nlm.nih.gov/) database. The criteria were as follows: (I) the samples should be early-stage samples; (II) they all detected lncRNA, miRNA, and Mrna; (III) the samples have clinical data. A total of 32 samples in datasets GSE63459 and GSE63805 met the criteria. Additionally, the samples were detected by the Illumina HumanRef-8 v3.0 Expression BeadChip and NanoString nCounter Human miRNA assay.
Screening of significantly differentially expressed RNA (DERs)
First, we downloaded detailed annotation information from the Ensembl genome browser 96-database (8) (http://asia.ensembl.org/index.html), including probes, gene symbols, RNA types and other information. Then we re-annotated the data in the training and validation data set. Finally, we obtained the expression levels of lncRNA, miRNA and mRNA of the paired samples.
According to the sample source, the training data set samples were divided into two groups, LUAD and control. The expression data were analyzed with the limma package of R (9). |log2 foldchange (FC)| >0.5 and false discovery rate (FDR) <0.05 were considered significant. We generated a heatmap using the pheatmap package (10).
Construction and functional analysis of ceRNA
Gene regulation at the post-transcriptional level is not a simple microRNA-mRNA silencing mechanism but a complex network. Many noncoding RNAs have microRNA binding sites and can act as miRNA sponges in cells (6,11). They can release miRNA’s regulatory effect on its target genes and change their expression levels. lncRNA is the most typical one. First, we searched the connection between DElncRNAs and DEmiRNAs through the DIANA-LncBasev2 database (12) (http://carolina.imis.athena-innovation.gr/diana_tools/web/index.php?r=lncbasev2%2Findex-experimental). We constructed a DElncRNA-DEmiRNA connection network according to the connection pairs whose expression differences were opposite to each other. Then we searched the target genes regulated by DEmiRNA in the starBase Version 2.0 database (13) (http://starbase.sysu.edu.cn/). We still used negatively correlated pairs to construct a DEmiRNA-DEmRNA connection network.
Finally, we sorted the results of DElncRNA-DEmiRNA and DEmiRNA-DEmRNA and then constructed a ceRNA regulatory network composed of DElncRNA-DEmiRNA-DEmRNA. We visualized the network through Cytoscape v.3.6.1 (14). Next, we used DAVID v.6.8 (15,16) to analyze the Gene Ontology (GO) biological process and Kyoto Encyclopedia of Genes and Genomes (KEGG) pathway enrichment analysis of the DERs in the ceRNA network. A p-value less than 0.05 was considered statistically significant for enrichment screening.
Construction of prognostic score model
Screening of DERs related to prognosis
There are 232 LUAD samples in the training data set. Combining the clinical data of these samples, we performed univariate Cox regression analysis on RNAs in the ceRNA network using the R package of survival (17). First, we screened out DERs (including lncRNA, miRNA and mRNA) significantly related to overall survival prognosis; then, for these DERs, we performed a multivariate Cox regression analysis to screen DERs significantly related to independent prognosis. We selected Plog-rank<0.05 as the threshold for significant correlation.
Screening the optimal DER combination and constructing a prognostic score model
Based on the expression of the DERs that were significantly related to independent prognosis, the R package of penalized (18) screened out the optimal prognosis-related signature DERs by the Cox-Proportional Hazards model (19).
According to the prognostic coefficient and the expression level of the optimized signature DERs, we constructed the following prognostic score (PS) model:
βDERs represents the prognosis coefficient of signature DERs, and Exp DERs represents the expression level of signature DERs in the training data set.
Effectiveness evaluation of the PS model
We calculated each sample’s PS value in the training data set and divided the samples into high-risk and low-risk groups according to the median value of PS. Then, we used Kaplan-Meier (KM) analysis to evaluate the association between high-risk and low-risk samples and actual survival prognostic information using the R survival package (17). Finally, we evaluated the association in the validation dataset.
Construction and evaluation of survival rate models
This study used the R survival package (17) to perform univariate and multivariate Cox regression analysis on the LUAD samples in the training set. After screening, we obtained independent survival prognostic clinical factors. We selected Plog-rank<0.05 as the threshold for significant correlation. To further investigate the relationship between independent prognostic factors and risk groups, we conducted a risk stratification analysis on these independent prognostic clinical factors, dividing the samples into different groups according to the clinical factors. Then, correlation analyses of risk prognosis models were performed in other groups.
To further study the relationship between independent prognostic factors and survival prognosis, we used the independent prognostic factors and the prognostic score model to construct a nomogram for three and five years of survival rate prediction model.
We used the area under the ROC curve (AUC) and C-index to evaluate the different types of models. (I) AUC. It is one of the main indicators for evaluating the two-class model. AUROC is a quantitative indicator of the ROC curve. The value is distributed between 0.5 and 1. The closer to 1, the better the classifier performance (20). (II) C-index: C-index >0.70 indicates a good model (21).
Results
Screening of DERs
In our research, 16,205 mRNA, 150 lncRNA, and 761 miRNA were obtained after annotation. According to the sample source, we divided the samples in the training data set into two groups: LUAD and control. Through the limma package, we filtered 2,909 DERs, including 2,701 mRNAs (728 down- and 1,973 up-regulated), 47 lncRNAs (5 down- and 42 up-regulated), and 161 miRNAs (20 down and 141 up-regulation). As shown in Figure 1, the samples cluster into two types.
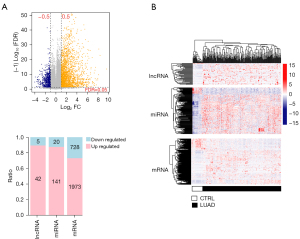
Construction and functional analysis of ceRNA
Through the DIANA-LncBasev2 database, we obtained 150 pairs of lncRNAs and DEmiRNAs with opposite expressions. Using starBase Version 2.0 database, we obtained 150 pairs of lncRNAs and DEmiRNAs with opposite expressions. Based on these results, we constructed a ceRNA regulatory network composed of lncRNA-miRNA-mRNA (Figure 2). There were 293 nodes in the network, including 32 lncRNAs, 87 miRNAs and 174 mRNAs.
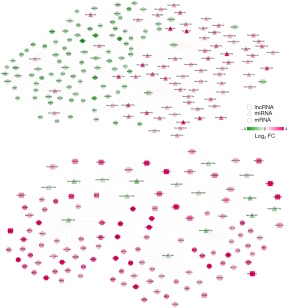
To further elucidate the underlying biological functions of genes and the main signaling pathways in the ceRNA network, we performed functional enrichment analyses, including GO and KEGG analysis, for the mRNAs in the ceRNA. As shown in Figure 3, we obtained 20 significantly related GO biological processes and 15 KEGG signaling pathways. DEmRNA in the ceRNA regulatory network were significantly related to the cell cycle biological processes, and subsequently, significantly participates in the KEGG signal pathway of the cell cycle.
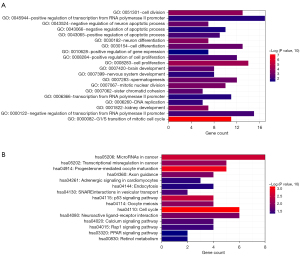
Construction of prognostic score model
Based on the DERNAs in the ceRNA network, univariate Cox regression analysis was used to screen out DERs significantly related to survival prognosis by the R package of survival. On this basis, we performed multivariate cox regression analysis and obtained 16 independently significant prognostic DERs, including ten mRNAs, four lncRNAs and two miRNAs. Based on the 16 DERs, we used the Cox-PH model of the regularization regression algorithm to screen the optimal combination of signature DERs and finally obtained 12 DERs. As shown in Table 1, there were two lncRNAs, two miRNAs and eight mRNAs.
Table 1
Symbol | Type | Multi-variate Cox regression analysis | LASSO coef | ||
---|---|---|---|---|---|
HR | 95% CI | P value | |||
MIR99AHG | lncRNA | 0.313 | 0.0859–0.911 | 4.78E−02 | −0.0519 |
MEG8 | 3.869 | 1.327–11.283 | 1.32E−02 | 0.4764 | |
hsa-miR-490-3p | miRNA | 0.309 | 0.130–0.732 | 7.64E−03 | −0.7347 |
hsa-miR-542-5p | 1.770 | 1.201–2.609 | 3.91E−03 | 0.3371 | |
MFAP3L | mRNA | 1.463 | 1.184–2.491 | 1.62E−02 | 0.2376 |
RIMS4 | 2.544 | 1.454–4.451 | 1.07E−03 | 0.5450 | |
KIF23 | 0.420 | 0.205–0.860 | 1.77E−02 | −0.2931 | |
SLC38A3 | 0.342 | 0.104–0.623 | 4.77E−02 | −0.6480 | |
ANLN | 1.573 | 1.025–2.742 | 1.10E−02 | 0.3258 | |
E2F7 | 2.340 | 1.114–4.915 | 2.48E−02 | 0.2904 | |
HMGA2 | 1.178 | 1.033–1.633 | 3.24E−02 | 0.1643 | |
POU4F1 | 1.057 | 1.051–1.701 | 4.95E−02 | 0.0531 |
A PS model was constructed based on the prognostic coefficient and their expression level of the 12 signature DERs in the TCGA training set samples.
We calculated the risk score based on the PS model of each sample in the TCGA training data set. Then, according to their median, we divided all the samples into the high-risk and low-risk groups. The prognostic value of these signature DERs was demonstrated by K-M plots (Figure 4A). Finally, we performed the same analysis in the validation data set (Figure 4B). The results showed that in the training data set and the validation data set, and there was a significant correlation between the different risk groups obtained by dividing the samples based on the PS model prediction and the actual prognosis.
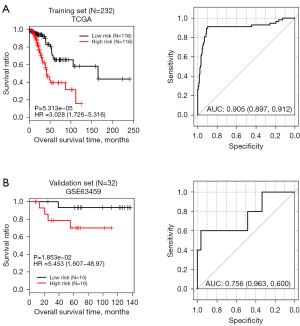
Construction of survival rate model
Combined with the clinical data of LUAD samples in the training data set, we used univariate and multivariate Cox regression analyses to select independent prognostic clinical factors (Table 2). The results significantly associated three clinical factors with independent prognosis: age, tumor recurrence, and PS model status. Figure 5 showed the KM curves related to the survival prognosis of age and tumor recurrence. It can be seen from the figure that samples with low age and no tumor recurrence have a better survival prognosis. This conclusion is consistent with the actual situation. Additionally, we grouped the samples according to age (>65 and ≤65) and tumor recurrence (recurrence and without recurrence). Finally, we analyzed the correlation between the PS model’s prediction results and the actual prognosis in each group (Figure 5, middle and right).
Table 2
Clinical characteristics | TCGA (n=232) | Uni-variable cox | Multi-variable cox | |||
---|---|---|---|---|---|---|
HR (95% CI) | P value | HR (95% CI) | P value | |||
Age (years), mean ± SD | 65.96±9.90 | 1.041 (1.011–1.072) | 4.968E−03 | 1.046 (1.011–1.082) | 1.011E-02 | |
Gender (male/female) | 93/139 | 0.617 (0.355–1.073) | 8.399E−02 | – | – | |
Pathologic M (M0/M1/–) | 145/0/87 | – | – | – | – | |
Pathologic N (N0/N1/N2N3/–) | 228/0/0/0/4 | – | – | – | – | |
Pathologic T (T1/T2) | 117/115 | 0.919 (0.543–1.555) | 7.534E−01 | – | – | |
Radiation therapy (yes/no/–) | 16/204/12 | 2.118 (0.992–4.523) | 5.258E−02 | – | – | |
Smoking history (never/reform/current/–) | 10/54/32/152 | 0.971 (0.499–1.889) | 9.301E−01 | – | – | |
Tumor recurrence (yes/no/–) | 58/151/23 | 2.141 (1.289–4.522) | 4.563E−03 | 2.185 (1.159–4.117) | 1.566E-02 | |
PS status (high/low) | 116/116 | 3.029 (1.726–5.316) | 5.313E−05 | 3.443 (1.655–7.166) | 9.450E-04 | |
Vital status (death/alive) | 57/175 | – | – | – | – | |
Overall survival time (months), mean ± SD | 34.59±34.67 | – | – | – | – |
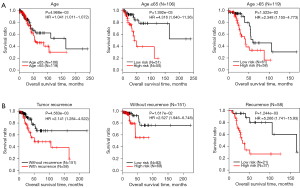
Construction of survival rate model with independent prognostic clinical factors
To further analyze the correlation between age, tumor recurrence, PS model status and survival prognosis, we constructed a nomogram (Figure 6A). The nomogram uses the “Total points” axis in the first row to integrate various clinical indicators and predict the sample’s survival. The C-index for 3-year and 5-year survival were 0.775 and 0.715, respectively, suggesting an excellent prediction performance (Figure 6B).
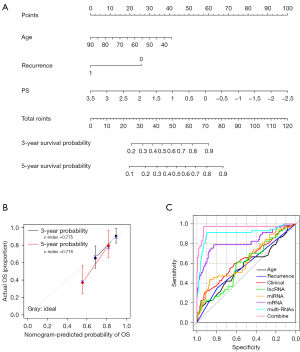
Additionally, based on independent prognostic clinical factors, we constructed separate prognostic clinical models of clinical factors. Then we compared them with the previously-constructed PS prognostic model (Figure 6C, Table 3).
Table 3
Type | AUROC (specificity and sensitivity) | C-index | P value |
---|---|---|---|
Age | 0.597 (0.516, 0.683) | 0.596 | 7.462E−02 |
Recurrence | 0.573 (0.596, 0.549) | 0.686 | 9.545E−03 |
Clinical | 0.636 (0.719, 0.631) | 0.675 | 1.496E−05 |
lncRNA | 0.591 (0.594, 0.614) | 0.611 | 3.327E−02 |
miRNA | 0.634 (0.669, 0.539) | 0.596 | 7.904E−02 |
mRNA | 0.817 (0.829, 0.789) | 0.689 | 1.045E−04 |
multi-RNAs | 0.905 (0.897, 0.912) | 0.785 | 1.32E−10 |
Combine | 0.963 (0.932, 0.976) | 0.805 | 2.07E−14 |
Discussion
LUAD is a subtype of lung cancer with a high incidence and complex pathogenesis. Advances in molecular biology helped us better understand the molecular mechanism of LUAD occurrence and development to discover potential molecular markers. In recent years, the ceRNA hypothesis has received increasing attention in cancer research. The mutual regulation of various types of RNA provides new clues for cancer research.
In our research, we used LUAD samples from patients in early-stage from the TCGA database. We constructed a ceRNA regulatory network composed of lncRNA, miRNA, and mRNA. In the ceRNA network, there are 32 lncRNAs, 87 miRNAs, and 174 mRNAs. It reveals the interrelationship between lncRNA, miRNA, and mRNA. By GO and KEGG pathway analyses, mRNAs in the ceRNA network were enriched in 20 biological processes and 15 signal pathways. They are significantly related to the biological process “cell cycle” and signal pathway “cell cycle”. Combined with clinical data, we screened 16 RNAs that are independently significantly related to prognosis.
Many of these RNAs play a regulatory role in cancer. Abnormal LncRNA expression will affect cancer patients’ prognoses. LncRNA MIR99AHG plays an essential role in gastric cancer progression by inhibiting apoptosis via miR577/FOXP1 axis (22). The overexpression of lncRNA MEG8 can inhibit the expression of microRNA-34a and microRNA-203. It is essential for TGF-β-induced epithelial-mesenchymal transition in lung cancer cells (23). miRNA is a type of noncoding RNA molecule and plays a vital role at the post-transcriptional level. Li et al. (24) proved that the overexpression of miRNA miR-490-3p will promote lung cancer metastasis. The expression of miRNA hsa-miR-542-5p is significantly different between non-small-cell lung cancer and normal tissues (25). All biological activities in cells are ultimately manifested through the expression and biological functions of proteins. ANLN, SLC38A3, and HMGA2 played important roles in metastases in lung cancer (26-28). They may act as potential therapeutic targets. MFAP3L amplification is related to poor prognosis by promoting cell invasion and metastasis in colorectal cancer (29). KIF23 has been identified as a prognostic signature for ovarian cancer (30).
Using the Cox-PH model, we obtained an optimal combination of 12 RNAs to construct a PS model to predict prognosis. Kaplan-Meier survival curves indicated that the PS model significantly correlates with poor survival among patients with early-stage LUAD. Analyzing the clinical data, age and tumor metastasis were two important factors related to LUAD prognosis. According to the PS model, age, and tumor metastasis information, we can predict patients’ 3-year and 5-year survival rates through the nomogram.
Conclusions
In this study, a ceRNA network was established for early-stage LUAD. Combined with the clinical data, we conducted prognostic risk-related and biological function analysis of RNAs in the ceRNA network. This provides reference and research directions for an in-depth study of LUAD gene regulation and offers potential biomarkers for the prognoses of patients with LUAD.
Acknowledgments
Funding: None.
Footnote
Reporting Checklist: The authors have completed the MDAR reporting checklist. Available at https://dx.doi.org/10.21037/tcr-20-3273
Conflicts of Interest: All authors have completed the ICMJE uniform disclosure form (available at https://dx.doi.org/10.21037/tcr-20-3273). The authors have no conflicts of interest to declare.
Ethical Statement: The authors are accountable for all aspects of the work in ensuring that questions related to the accuracy or integrity of any part of the work are appropriately investigated and resolved. The study was conducted in accordance with the Declaration of Helsinki (as revised in 2013).
Open Access Statement: This is an Open Access article distributed in accordance with the Creative Commons Attribution-NonCommercial-NoDerivs 4.0 International License (CC BY-NC-ND 4.0), which permits the non-commercial replication and distribution of the article with the strict proviso that no changes or edits are made and the original work is properly cited (including links to both the formal publication through the relevant DOI and the license). See: https://creativecommons.org/licenses/by-nc-nd/4.0/.
References
- Travis WD, Brambilla E, Noguchi M, et al. International association for the study of lung cancer/american thoracic society/european respiratory society international multidisciplinary classification of lung adenocarcinoma. J Thorac Oncol 2011;6:244-85. [Crossref] [PubMed]
- Zhao J, Li L, Wang Q, et al. CircRNA Expression Profile in Early-Stage Lung Adenocarcinoma Patients. Cell Physiol Biochem 2017;44:2138-46. [Crossref] [PubMed]
- Gupta RA, Shah N, Wang KC, et al. Long non-coding RNA HOTAIR reprograms chromatin state to promote cancer metastasis. Nature 2010;464:1071-6. [Crossref] [PubMed]
- Du X, Zhang J, Wang J, et al. Role of miRNA in Lung Cancer-Potential Biomarkers and Therapies. Curr Pharm Des 2018;23:5997-6010. [Crossref] [PubMed]
- Giordano M, Boldrini L, Servadio A, et al. Differential microRNA expression profiles between young and old lung adenocarcinoma patients. Am J Transl Res 2018;10:892-900. [PubMed]
- Salmena L, Poliseno L, Tay Y, et al. A ceRNA hypothesis: the Rosetta Stone of a hidden RNA language? Cell 2011;146:353-8. [Crossref] [PubMed]
- Barrett T, Wilhite SE, Ledoux P, et al. NCBI GEO: archive for functional genomics data sets--update. Nucleic Acids Res 2013;41:D991-5. [Crossref] [PubMed]
- Nicolaidou V, Papaneophytou C, Koufaris C. Detection and characterisation of novel alternative splicing variants of the mitochondrial folate enzyme MTHFD2. Mol Biol Rep 2020;47:7089-96. [Crossref] [PubMed]
- Ritchie ME, Phipson B, Wu D, et al. limma powers differential expression analyses for RNA-sequencing and microarray studies. Nucleic Acids Res 2015;43:e47. [Crossref] [PubMed]
- Wang L, Cao C, Ma Q, et al. RNA-seq analyses of multiple meristems of soybean: novel and alternative transcripts, evolutionary and functional implications. BMC Plant Biol 2014;14:169. [Crossref] [PubMed]
- Hansen TB, Jensen TI, Clausen BH, et al. Natural RNA circles function as efficient microRNA sponges. Nature 2013;495:384-8. [Crossref] [PubMed]
- Paraskevopoulou MD, Vlachos IS, Karagkouni D, et al. DIANA-LncBase v2: indexing microRNA targets on non-coding transcripts. Nucleic Acids Res 2016;44:D231-8. [Crossref] [PubMed]
- Li JH, Liu S, Zhou H, et al. starBase v2.0: decoding miRNA-ceRNA, miRNA-ncRNA and protein-RNA interaction networks from large-scale CLIP-Seq data. Nucleic Acids Res 2014;42:D92-7. [Crossref] [PubMed]
- Shannon P, Markiel A, Ozier O, et al. Cytoscape: a software environment for integrated models of biomolecular interaction networks. Genome Res 2003;13:2498-504. [Crossref] [PubMed]
- Huang da W. Sherman BT, Lempicki RA. Systematic and integrative analysis of large gene lists using DAVID bioinformatics resources. Nat Protoc 2009;4:44-57. [Crossref] [PubMed]
- Huang da W. Sherman BT, Lempicki RA. Bioinformatics enrichment tools: paths toward the comprehensive functional analysis of large gene lists. Nucleic Acids Res 2009;37:1-13. [Crossref] [PubMed]
- Wang P, Wang Y, Hang B, et al. A novel gene expression-based prognostic scoring system to predict survival in gastric cancer. Oncotarget 2016;7:55343-51. [Crossref] [PubMed]
- Goeman JJ. L1 penalized estimation in the Cox proportional hazards model. Biom J 2010;52:70-84. [PubMed]
- Tibshirani R. The lasso method for variable selection in the Cox model. Stat Med 1997;16:385-95. [Crossref] [PubMed]
- Robin X, Turck N, Hainard A, et al. pROC: an open-source package for R and S+ to analyze and compare ROC curves. BMC Bioinformatics 2011;12:77. [Crossref] [PubMed]
- Harrell FE Jr, Lee KL, Mark DB. Multivariable prognostic models: issues in developing models, evaluating assumptions and adequacy, and measuring and reducing errors. Stat Med 1996;15:361-87. [Crossref] [PubMed]
- Meng Q, Wang X, Xue T, et al. Long noncoding RNA MIR99AHG promotes gastric cancer progression by inducing EMT and inhibiting apoptosis via miR577/FOXP1 axis. Cancer Cell Int 2020;20:414. [Crossref] [PubMed]
- Terashima M, Ishimura A, Wanna-Udom S, et al. MEG8 long noncoding RNA contributes to epigenetic progression of the epithelial-mesenchymal transition of lung and pancreatic cancer cells. J Biol Chem 2018;293:18016-30. [Crossref] [PubMed]
- Li J, Feng Q, Wei X, et al. MicroRNA-490 regulates lung cancer metastasis by targeting poly r(C)-binding protein 1. Tumour Biol 2016;37:15221-8. [Crossref] [PubMed]
- Joerger M, Baty F, Früh M, et al. Circulating microRNA profiling in patients with advanced non-squamous NSCLC receiving bevacizumab/erlotinib followed by platinum-based chemotherapy at progression (SAKK 19/05). Lung Cancer 2014;85:306-13. [Crossref] [PubMed]
- Gao X, Dai M, Li Q, et al. HMGA2 regulates lung cancer proliferation and metastasis. Thorac Cancer 2017;8:501-10. [Crossref] [PubMed]
- Wang Y, Fu L, Cui M, et al. Amino acid transporter SLC38A3 promotes metastasis of non-small cell lung cancer cells by activating PDK1. Cancer Lett 2017;393:8-15. [Crossref] [PubMed]
- Xu J, Zheng H, Yuan S, et al. Overexpression of ANLN in lung adenocarcinoma is associated with metastasis. Thorac Cancer 2019;10:1702-9. [Crossref] [PubMed]
- Lou X, Kang B, Zhang J, et al. MFAP3L activation promotes colorectal cancer cell invasion and metastasis. Biochim Biophys Acta 2014;1842:1423-32. [Crossref] [PubMed]
- Hu Y, Zheng M, Wang C, et al. Identification of KIF23 as a prognostic signature for ovarian cancer based on large-scale sampling and clinical validation. Am J Transl Res 2020;12:4955-76. [PubMed]