A novel immune checkpoint-related gene signature for predicting overall survival and immune status in triple-negative breast cancer
Introduction
Triple-negative breast cancer (TNBC) is a highly aggressive subtype which is characterized by negative expression of estrogen receptor (ER), progesterone receptor (PR) and human epidermal growth factor receptor 2 (HER2). TNBC accounts for approximately 15–20% of all breast cancer patients and occurs more commonly in younger patients (1,2). Due to the lack of effective therapeutic targets, surgery combined radiotherapy and chemotherapy is the main therapeutic approach for TNBC patients. However, the resistance of the adjuvant therapy and the aggressive nature of TNBC triggers to the high postoperative recurrence rate which even reached 25–40% (2), and the median survival time was only 20 months (3,4). Therefore, it’s urgent to explore an effective therapeutic approach for TNBC patients.
In recent years, immunotherapy has made a rapid development in the treatment of several tumors such as melanoma (5), lymphoma (6), and lung cancer (7). Previous studies have also demonstrated that immunotherapy might be effective in TNBC (8,9). Immune checkpoints are considered as an important therapeutic target and immune checkpoint inhibitors (ICIs) were well-studied in different malignancies. ICIs could block immunosuppressive receptors and improve the cytotoxicity and proliferation of tumor-infiltrating lymphocytes (TILs) (10). It is worth mentioning that high level of genomic instability and tumor mutational loads results in higher tumor immunogenicity of TNBC in comparison with other subtypes (11,12). Therefore, TNBC patients are expected to benefit from immunotherapy. However, the overall remission rate for TNBC patients remained only 5–23% (13), which indicated that only a small number of the patients could benefit from the immunotherapy. Therefore, a robust biomarker is significant to identify the patients who can greatly benefit from immunotherapy.
Several published studies revealed that the expression of immune checkpoint genes (ICGs) correlates with the response of immunotherapy and prognosis outcomes in nasopharyngeal carcinoma, hepatocellular carcinoma and other tumors (14,15). However, studies for TNBC were limited to a single ICG and the findings were still under debate. Few studies have systematically investigated the expression pattern of ICGs in TNBC or attempted to establish an ICGs-based prognostic gene signature to facilitate and improve clinical decision making. In our present study, we aimed to identify the prognosis-related ICGs and construct a novel gene signature for TNBC based on the transcriptomic and clinical data from the TNBC cohort in The Cancer Genome Atlas (TCGA) database and the Molecular Taxonomy of Breast Cancer International Consortium (METABRIC) database, in order to provide references for individual prognosis and treatment guidance.
Methods
Data collection
We present the following article in accordance with the TRIPOD reporting checklist (available at https://tcr.amegroups.com/article/view/10.21037/tcr-21-1455/rc) (Figure 1). The transcriptome data and clinical characteristics of TNBC samples were obtained from TCGA (https://portal.gdc.cancer.gov/) and METABRIC database (https://molonc.bccrc.ca/aparicio-lab/research/metabric/). The inclusion criteria were: (I) TNBC samples determined by the immunochemistry results of ER, PR and HER2 status; (II) transcriptome data and clinical data were comprehensive and available; (III) the overall survival (OS) time was longer than 30 days. After screening, 113 TNBC patients in TCGA database and 286 TNBC patients in METABRIC database were included in our present study. The study was conducted in accordance with the Declaration of Helsinki (as revised in 2013). The clinical information of two cohorts was summarized in Table 1. R software (version 4.0.3) was utilized for data collection and processing.
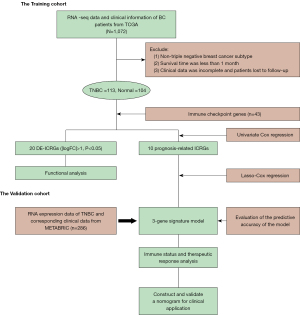
Table 1
Variables | TCGA cohort (n=113) | METABRIC cohort (n=286) |
---|---|---|
Age at diagnosis (years) | ||
<50 | 41 (36.3) | 104 (36.4) |
≥50 | 72 (63.7) | 182 (63.6) |
T stage | ||
T1–2 | 101 (89.4) | 264 (92.3) |
T3–4 | 12 (10.6) | 22 (7.7) |
N stage | ||
N0 | 96 (84.9) | 230 (80.4) |
N+ | 17 (15.1) | 56 (19.6) |
AJCC stage | ||
I–IIa | 95 (84.0) | 221 (78.1) |
IIa–IV | 18 (16.0) | 61 (21.9) |
Surgery | ||
No | 5 (4.4) | 6 (2.1) |
Yes | 108 (95.6) | 280 (97.9) |
Radiotherapy | ||
No | 51 (45.1) | 80 (28.0) |
Yes | 62 (54.9) | 206 (72.0) |
Chemotherapy | ||
No | 34 (30.1) | 132 (46.2) |
Yes | 79 (69.9) | 154 (53.8) |
TNBC, triple-negative breast cancer; TCGA, The Cancer Genome Atlas; METABRIC, Molecular Taxonomy of Breast Cancer International Consortium.
Differentially expressed ICGs (DE-ICGs) analysis
A total of 43 ICGs according to the literatures were found in TCGA transcriptome data, and most of the ICGs were ligands, receptors or important molecules in immune checkpoint pathways (Table S1). The expression data of 43 immune-checkpoint related genes (ICRGs) of TNBC tissues and normal breast tissues in the TCGA database were extracted. The R package “limma” (version 3.46.0) was used to proceed the DE-ICRGs analysis. |Log2fold change (log2FC)|>1 and P value <0.05 were the cut-off criteria for DE-ICGs.
Gene Ontology (GO) enrichment and Kyoto Encyclopedia of Genes and Genomes (KEGG) pathway analysis
R package “ClusterProfiler” (version 3.18.0) was applied to perform GO functional annotation analysis and KEGG pathway enrichment analysis for DE-ICGs. GO functional analysis includes three main categories: biological processes (BPs), cellular components (CCs), and molecular functions (MFs). The dotplots of the results of GO analysis and KEGG pathway analysis were generated.
Identification of prognosis related ICGs
We used the samples in TCGA database as the training cohort and the METABRIC database was employed as the validation cohort. The least absolute shrinkage and selection operator (LASSO) Cox regression analysis was employed to identify the prognosis related ICGs according to the OS events, OS time and the expression level of DE-ICGs. Firstly, the R package “Survival” (version 3.2-7) was used to conduct univariate cox regression analysis to screen the potential prognosis related genes. Candidate genes with statistical significance after screening by univariable Cox regression analysis (P value: entry 0.05, removal 0.10) were put into the LASSO regression analysis to reduce collinearity between genes and prevent over-fitting of the prognostic risk model. Finally, the genes identified by LASSO regression analysis were entered into the multivariate Cox regression analysis to construct a prognostic model for predicting OS in TNBC patients.
Construction and validation of ICGs-signature
The coefficients of hub genes in the multivariate Cox regression were used to calculate the risk score for each sample. The risk score = β1 × X1 + β2 × X2 + … + βn × Xn, where X represents the gene expression values and β represents the regression coefficient of each hub gene included in the ICGs-signature model. The median score was considered as the cut-off value to divide the TNBC patients into a high-risk group and a low-risk group. The Kaplan-Meier survival curves were generated by R package “Survival” (version 3.2-7) to further explore the association between the risk scores and prognostic outcomes. The receiver operating characteristic (ROC) curves of the risk score in both training and validation sets were plotted by R package “timeROC” (version 0.4) and the area under the curve (AUC) values were calculated respectively.
Immune status analysis
To further explore the correlation between the risk score and immune status of TNBC patients, a single sample gene set enrichment analysis (ssGSEA) was conducted by R software. Immunophenoscore (IPS), which is a machine learning-based algorithm, was used for the quantitative evaluation of tumor immunogenicity. It is calculated based on the Z-score of representative cell type gene expression including: immunomodulators, effector cells, immunosuppressive cells and MHC molecules. The IPS (range, 0–10) is calculated based on the gene expression in representative cell types. The IPS of patients were downloaded from The Cancer Immunome Atlas (TCIA). The IPS in the high-risk group and low-risk group was analyzed. The expression level of four ICGs (PD-1, CTLA-4, PD-L1, PD-L2) was also compared between two groups.
Statistical analysis
The statistical significance of ICGs expression level between TNBC tissues and normal breast tissues were estimated by Student’s t-test. The Mann-Whitney test was used to analyze the IPS scores in high-risk group and low-risk group. A P value of <0.05 was considered statistically significant.
Results
DE-ICGs in TNBC
Twenty ICGs were differentially expressed in TNBC tumor tissues in comparison with the normal breast tissues. Nineteen ICGs were down-regulated and one ICG was up-regulated in TNBC tissues (Figure 2A,2B). Furthermore, GO and KEGG pathway analysis were conducted. The results of GO enrichment analysis illustrated that the DE-ICGs were mostly involved in “T cell activation”, “positive regulation of lymphocyte activation”, “regulation of leukocyte cell-cell adhesion” in the BP category, “MHC protein complex” in the CC category, and “peptide antigen binding”, “antigen binding” in the MF category (Figure 2C). KEGG pathway analysis revealed that these DE-ICGs were mainly associated with cell adhesion molecules, antigen processing and presentation, PD-L1 expression and PD-1 checkpoint pathway in cancer (Figure 2D).
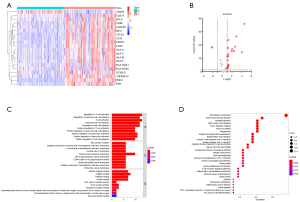
Development of prognosis related ICGs-signature
Firstly, univariate cox regression analysis was used to select candidate ICGs that were associated with OS of TNBC patients. Ten ICGs were identified as potential OS predictors, including IDO1, CD274, PDCD1LG2, PDCD1, CTLA4, ICOS, KIR3DL2, HLA-B, HLA-F, LGA3 (Figure 3A). All the 10 ICGs were favorable prognostic predictors [hazard ratio (HR) <1; P<0.05]. The correlation between 10 genes was depicted in Figure 3B. Secondly, LASSO regression analysis showed that 3 of 10 ICGs were the OS-related ICGs including PDCD1LG2, KIR3DL2 and PDCD1 (Figure 3C,3D). Eventually, the 3 OS-related ICGs were included in the multivariant Cox regression model (Table 2). The coefficients of the OS-related ICGs were shown in Figure 3E.
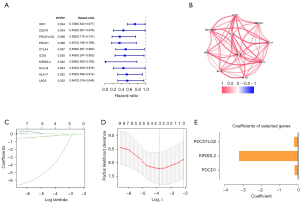
Table 2
Gene symbols | Description | Coefficients |
---|---|---|
PDCD1LG2 | Programmed cell death 1 ligand 2 | −0.199675855 |
KIR3DL2 | Killer cell immunoglobulin like receptor, three Ig domains and long cytoplasmic tail 2 | −3.200022817 |
PDCD1 | Programmed cell death 1 | −0.171493072 |
LASSO, least absolute shrinkage and selection operator.
After risk scores of each sample in training set were calculated, 113 patients in TCGA database were divided into low-risk group (n=56) and high-risk group (n=57) by the median score (median score =−0.91; Figure 4A,4B). Kaplan-Meier survival curve showed that patients in the high-risk group had worse OS than those in the low-risk group (P<0.001; Figure 4C). The predictive value of the 3-ICGs signature was assessed by ROC curve. The AUC of the ROC curve for predicting 1-, 2- and 3-year OS were 0.925, 0.822 and 0.835, respectively (Figure 4D). The risk scores of patients in METABRIC cohort were also calculated and the patients were then divided into low-risk group (n=143) and high-risk group (n=143) (Figure 4E,4F). In the validation cohort, patients in the high-risk group exhibited a worse prognosis than those in the high-risk group (Figure 4G). ROC curves also indicated a fairly good predictive value of 3-ICGs signature in the validation cohort (Figure 4H). Moreover, higher pathological stages and lower expression level of these hub genes were found in the high-risk group (Figure 5).
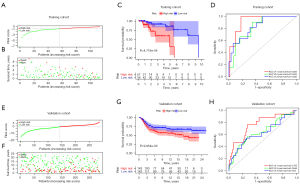
In order to further confirm the weight of 3-ICGs risk score in prognostic prediction, the univariant and multivariant Cox regression analyses were conducted. The results showed that the 3-ICGs risk score was a significant indicator for predicting the OS of TNBC patients compared with other clinical characteristics (Table 3).
Table 3
Variables | Univariate analysis | Multivariate analysis | |||||
---|---|---|---|---|---|---|---|
HR | 95% CI | P value | HR | 95% CI | P value | ||
Age (years) | |||||||
<50 | 1.000 | 1.000 | |||||
≥50 | 1.13 | 0.45–2.88 | 0.791 | 1.12 | 0.30–4.12 | 0.868 | |
Surgery | |||||||
No | 1.000 | 1.000 | |||||
Yes | 0.43 | 0.12–1.58 | 0.104 | 0.25 | 0.05–1.29 | 0.098 | |
Chemotherapy | |||||||
No | 1.000 | 1.000 | |||||
Yes | 1.85 | 0.60–5.74 | 0.285 | 2.87 | 0.81–10.17 | 0.102 | |
T stage | |||||||
T1–2 | 1.000 | 1.000 | |||||
T3–4 | 2.78 | 0.89–8.65 | 0.078 | 1.25 | 0.48–3.28 | 0.147 | |
N stage | |||||||
N0 | 1.000 | 1.000 | |||||
N+ | 3.56 | 2.29–14.98 | 0.026 | 1.27 | 0.40–4.07 | 0.685 | |
AJCC stage | |||||||
I–IIa | 1.000 | 1.000 | |||||
IIa–IV | 6.96 | 2.57–18.85 | <0.001 | 4.14 | 0.81–21.23 | 0.089 | |
Risk score | |||||||
Low risk | 1.000 | 1.000 | |||||
High risk | 8.80 | 2.12–36.45 | <0.001 | 10.82 | 1.72–68.05 | 0.011 |
HR, hazard ratio; CI, confidence interval.
Patterns of immune cell infiltration of TNBC patients
To evaluate the difference of immune status in high-risk and low-risk group, the enrichment scores of different immune cell subpopulations or immune-related pathways were evaluated by ssGSEA analysis. As expected, adaptive immunity cells and innate immunity cells were significantly abundant in the low-risk group, including B cells, CD8+ T cells, DCs, macrophages and NK cells (Figure 6A). Higher enrichment scores were observed for cytokine-cytokine receptor interaction, checkpoint, cytolytic activity and T cell co-stimulation signaling pathways in low-risk group. Furthermore, the scores of type I and II IFN response were significantly higher than that in the high-risk group (Figure 6B). Above all, these results indicated that effective immune response was more activated in the low-risk group, which may contribute to the therapeutic response difference.
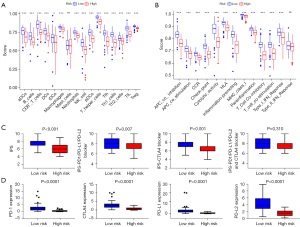
Moreover, the IPS scores were calculated to reveal the immunogenicity of patients in high-risk and low risk groups. Our results showed that low-risk patients tended to have higher IPS scores of PD-1, CTLA4, PD-L1 and PD-L2 (Figure 6C). Similarly, the expression level of immune checkpoint molecules (PD-1, CTLA4, PD-L1 and PD-L2) was higher in low-risk group (Figure 6D). We could speculate that low-risk group patients might get a favorable response to immunotherapy due to their higher immunogenicity.
Discussion
In recent years, immunotherapy for malignancies developed rapidly. Immunotherapy is expected to improve the outcomes of TNBC patients. However, TNBC patients could hardly achieved complete pathological remission from ICI therapy (16,17). Therefore, it is critical to identify biomarkers for predicting the response of immunotherapy in TNBC patients. Considering the tumor heterogeneity, the predictive value of a single biomarker is limited. Previous studies have indicated that several ICG signature models had favorable predictive value in long-term prognostic outcomes and therapeutic response in lung cancer, endometrial cancer and hepatocellular carcinoma. However, systematic and comprehensive studies are lacking in TNBC. In our present study, based on TCGA and METABRIC database, the expression pattern and prognostic value of 43 ICGs were analyzed. A novel 3-ICGs signature was finally developed for predicting the prognostic outcomes and immune status in TNBC patients.
In the 3-ICGs signature, PDCD1 (PD-1), PDCD1LG2 (PD-L2) and KIR3DL2 were significantly associated with a favorable prognosis in TNBC patients. Programmed cell death receptor (PDCD1/PD-1) is an important immunosuppressive molecule, mostly expressed on the surface of activated T cells and B cells. PD-1 can bind to its ligands (PD-L1, PD-L2) on the surface of tumor cells and contributes to the immune escape of tumor cells and promotes tumor development (18). Antibodies targeting PD-1 showed promising antitumor activity in melanoma, non-small cell lung cancer and kidney cancer. Interestingly, Wang et al. (19) found that PD-1 was also expressed on the surface of several tumor cell lines, which could contribute to anti-tumor effects by inhibiting AKT and ERK1/2 signaling pathways. In addition, PD-1 could be intrinsically expressed by tumor cells and could bind to self-expressed PD-L1 (20). Ren et al. (21) proposed that higher PD-1 expression in tumor was correlated with favorable disease-free survival and OS of TNBC patients, which was consistent with our findings. In addition, PD-L2 plays different roles in different tumors. Lin et al. (22) showed that PD-L2 might be a marker for poor prognosis in some digestive system tumors, while the opposite result was found in head and neck cancer (23). However, Asano et al. (24) reported that the expression level of PD-L2 was not related to the prognosis of TNBC patients, which seems not to be consistent with the findings of our study. We considered that the sample size of previous study was relatively small and further research is necessary. Similarly, KIR3DL2 could inhibit the tumor-killing ability by suppressing the cytotoxic function of NK cells and the secretion of IFN-γ and TNF-α (25). The effect of Lacutamab, an anti-KIR3DL2 monoclonal antibody, was now evaluated by the clinical trial with encouraging results (26,27). However, the role of KIR3DL2 in TNBC has not been reported.
Immune microenvironment plays a significant role in the tumorigenicity and development of TNBC. Our findings showed that significantly abundant TILs, CD8+ T cells, B cells, NK cells and Th cells were observed in low-risk group patients identified by the 3-ICGs signature. The proportion of TILs in TNBC was related to the prognostic outcome (28). Moreover, it is generally believed that enriched CD8+ T cells, B cells, NK cells were associated with favorable prognosis in TNBC patients. Likewise, Th cells could also help the B cells to regulate the anti-tumor immune response in TNBC (29). In terms of immune signaling pathways, impaired immune activity was associated with a higher risk score, including cytolytic activity, T cell co-stimulation, type I or II IFN-response. In addition, IPS and the expression level of PD-1, CTLA-4, PD-L1 and PD-L2 were significantly higher in low-risk group. IPS has been used to evaluate the response of tumors to ICIs (30). It is suggested that low-risk patients were more likely to have higher immunogenicity and expected to greatly benefit from immunotherapy.
Since the 3-ICGs signature contains only three genes, it is cost-effective and easy-to-use in clinical practice. The score of 3-ICGs signature could guide the immunotherapy, surveillance strategy and clinical decision making. However, there were some limitations in our study. All the clinical and transcriptome data collected in our study were based on publicly available datasets, the accuracy of the model should by further verified in clinical practice. A further in vitro or in vivo experimental study is necessary to be conducted to demonstrate the result of the present study findings.
Conclusions
A novel 3-ICGs signature based on the expression pattern of PDCD1, PDCD1LG2 and KIR3DL2 was developed and validated. The 3-ICGs signature may be not only served as a robust prognostic marker, but also an indicator reflecting immunotherapy response.
Acknowledgments
We acknowledge TCGA database and METABRIC database for providing their platforms and contributors for uploading their meaningful datasets.
Funding: This study was funded by National Science Foundation for Young Scientists of China (No. 81801300).
Footnote
Reporting Checklist: The authors have completed the TRIPOD reporting checklist. Available at https://tcr.amegroups.com/article/view/10.21037/tcr-21-1455/rc
Peer Review File: Available at https://tcr.amegroups.com/article/view/10.21037/tcr-21-1455/prf
Conflicts of Interest: All authors have completed the ICMJE uniform disclosure form (available at https://tcr.amegroups.com/article/view/10.21037/tcr-21-1455/coif). The authors have no conflicts of interest to declare.
Ethical Statement: The authors are accountable for all aspects of the work in ensuring that questions related to the accuracy or integrity of any part of the work are appropriately investigated and resolved. The study was conducted in accordance with the Declaration of Helsinki (as revised in 2013).
Open Access Statement: This is an Open Access article distributed in accordance with the Creative Commons Attribution-NonCommercial-NoDerivs 4.0 International License (CC BY-NC-ND 4.0), which permits the non-commercial replication and distribution of the article with the strict proviso that no changes or edits are made and the original work is properly cited (including links to both the formal publication through the relevant DOI and the license). See: https://creativecommons.org/licenses/by-nc-nd/4.0/.
References
- Borri F, Granaglia A. Pathology of triple negative breast cancer. Semin Cancer Biol 2021;72:136-45. [Crossref] [PubMed]
- Gluz O, Liedtke C, Gottschalk N, et al. Triple-negative breast cancer--current status and future directions. Ann Oncol 2009;20:1913-27. [Crossref] [PubMed]
- Schmid P, Adams S, Rugo HS, et al. Atezolizumab and Nab-Paclitaxel in Advanced Triple-Negative Breast Cancer. N Engl J Med 2018;379:2108-21. [Crossref] [PubMed]
- Garrido-Castro AC, Lin NU, Polyak K. Insights into Molecular Classifications of Triple-Negative Breast Cancer: Improving Patient Selection for Treatment. Cancer Discov 2019;9:176-98. [Crossref] [PubMed]
- Queirolo P, Boutros A, Tanda E, et al. Immune-checkpoint inhibitors for the treatment of metastatic melanoma: a model of cancer immunotherapy. Semin Cancer Biol 2019;59:290-7. [Crossref] [PubMed]
- Ansell SM, Lin Y. Immunotherapy of lymphomas. J Clin Invest 2020;130:1576-85. [Crossref] [PubMed]
- Forde PM, Chaft JE, Smith KN, et al. Neoadjuvant PD-1 Blockade in Resectable Lung Cancer. N Engl J Med 2018;378:1976-86. [Crossref] [PubMed]
- Nanda R, Chow LQ, Dees EC, et al. Pembrolizumab in Patients With Advanced Triple-Negative Breast Cancer: Phase Ib KEYNOTE-012 Study. J Clin Oncol 2016;34:2460-7. [Crossref] [PubMed]
- Heimes AS, Schmidt M. Atezolizumab for the treatment of triple-negative breast cancer. Expert Opin Investig Drugs 2019;28:1-5. [Crossref] [PubMed]
- Santarpia M, González-Cao M, Viteri S, et al. Programmed cell death protein-1/programmed cell death ligand-1 pathway inhibition and predictive biomarkers: understanding transforming growth factor-beta role. Transl Lung Cancer Res 2015;4:728-42. [PubMed]
- Luen S, Virassamy B, Savas P, et al. The genomic landscape of breast cancer and its interaction with host immunity. Breast 2016;29:241-50. [Crossref] [PubMed]
- Schumacher TN, Schreiber RD. Neoantigens in cancer immunotherapy. Science 2015;348:69-74. [Crossref] [PubMed]
- Keenan TE, Tolaney SM. Role of Immunotherapy in Triple-Negative Breast Cancer. J Natl Compr Canc Netw 2020;18:479-89. [Crossref] [PubMed]
- Song D, Tian J, Han X, et al. A model of seven immune checkpoint-related genes predicting overall survival for head and neck squamous cell carcinoma. Eur Arch Otorhinolaryngol 2021;278:3467-77. [Crossref] [PubMed]
- Xu D, Liu X, Wang Y, et al. Identification of immune subtypes and prognosis of hepatocellular carcinoma based on immune checkpoint gene expression profile. Biomed Pharmacother 2020;126:109903. [Crossref] [PubMed]
- Loibl S, Untch M, Burchardi N, et al. A randomised phase II study investigating durvalumab in addition to an anthracycline taxane-based neoadjuvant therapy in early triple-negative breast cancer: clinical results and biomarker analysis of GeparNuevo study. Ann Oncol 2019;30:1279-88. [Crossref] [PubMed]
- Nanda R, Liu MC, Yau C, et al. Effect of Pembrolizumab Plus Neoadjuvant Chemotherapy on Pathologic Complete Response in Women With Early-Stage Breast Cancer: An Analysis of the Ongoing Phase 2 Adaptively Randomized I-SPY2 Trial. JAMA Oncol 2020;6:676-84. [Crossref] [PubMed]
- Pardoll DM. The blockade of immune checkpoints in cancer immunotherapy. Nat Rev Cancer 2012;12:252-64. [Crossref] [PubMed]
- Wang X, Yang X, Zhang C, et al. Tumor cell-intrinsic PD-1 receptor is a tumor suppressor and mediates resistance to PD-1 blockade therapy. Proc Natl Acad Sci U S A 2020;117:6640-50. [Crossref] [PubMed]
- Zhao Y, Harrison DL, Song Y, et al. Antigen-Presenting Cell-Intrinsic PD-1 Neutralizes PD-L1 in cis to Attenuate PD-1 Signaling in T Cells. Cell Rep 2018;24:379-390.e6. [Crossref] [PubMed]
- Ren X, Wu H, Lu J, et al. PD1 protein expression in tumor infiltrated lymphocytes rather than PDL1 in tumor cells predicts survival in triple-negative breast cancer. Cancer Biol Ther 2018;19:373-80. [Crossref] [PubMed]
- Lin X, Lin K, Lin C, et al. Prognostic and clinicopathological utility of PD-L2 expression in patients with digestive system cancers: A meta-analysis. Int Immunopharmacol 2020;88:106946. [Crossref] [PubMed]
- Yearley JH, Gibson C, Yu N, et al. PD-L2 Expression in Human Tumors: Relevance to Anti-PD-1 Therapy in Cancer. Clin Cancer Res 2017;23:3158-67. [Crossref] [PubMed]
- Asano Y, Kashiwagi S, Goto W, et al. Prediction of treatment responses to neoadjuvant chemotherapy in triple-negative breast cancer by analysis of immune checkpoint protein expression. J Transl Med 2018;16:87. [Crossref] [PubMed]
- Martinez XU, Di Raimondo C, Abdulla FR, et al. Leukaemic variants of cutaneous T-cell lymphoma: Erythrodermic mycosis fungoides and Sézary syndrome. Best Pract Res Clin Haematol 2019;32:239-52. [Crossref] [PubMed]
- Bagot M, Porcu P, Marie-Cardine A, et al. IPH4102, a first-in-class anti-KIR3DL2 monoclonal antibody, in patients with relapsed or refractory cutaneous T-cell lymphoma: an international, first-in-human, open-label, phase 1 trial. Lancet Oncol 2019;20:1160-70. [Crossref] [PubMed]
- Khan M, Arooj S, Wang H NK. Cell-Based Immune Checkpoint Inhibition. Front Immunol 2020;11:167. [Crossref] [PubMed]
- Loi S, Michiels S, Salgado R, et al. Tumor infiltrating lymphocytes are prognostic in triple negative breast cancer and predictive for trastuzumab benefit in early breast cancer: results from the FinHER trial. Ann Oncol 2014;25:1544-50. [Crossref] [PubMed]
- Hollern DP, Xu N, Thennavan A, et al. B Cells and T Follicular Helper Cells Mediate Response to Checkpoint Inhibitors in High Mutation Burden Mouse Models of Breast Cancer. Cell 2019;179:1191-1206.e21. [Crossref] [PubMed]
- Charoentong P, Finotello F, Angelova M, et al. Pan-cancer Immunogenomic Analyses Reveal Genotype-Immunophenotype Relationships and Predictors of Response to Checkpoint Blockade. Cell Rep 2017;18:248-62. [Crossref] [PubMed]