A novel signature based on autophagy-related lncRNA for prognostic prediction and candidate drugs for lung adenocarcinoma
Introduction
Lung cancer is one of the world’s leading causes of cancer mortality (1), with adenocarcinoma being the most common accounting for about 40% of all cases (2). With the widespread use of low-dose computed tomography (CT), lung cancer can be diagnosed at an early stage, greatly reducing the mortality rate (3). Although the therapeutic measures for advanced lung adenocarcinoma (LUAD) have been improved and more individualised, including chemotherapy, radiotherapy, and immunotherapy (4), the prognosis remains poor. Therefore, the construction of a reliable prognostic signature is essential for the prognosis of LUAD patients and to develop effective treatment strategies.
Long non-coding RNAs (lncRNAs) are a series of transcript RNAs longer than 200 nucleotides without the functions of protein translation (5). Several studies have revealed that lncRNAs play important roles in a variety of tumour biological processes, such as neoplastic progression (6), cellular proliferation, migration, and DNA damage (7). Several lncRNAs are associated with LUAD, including FAM83A−AS1, LINC01116, MALAT1 and HOTAIR (8,9). Overexpression of FAM83A−AS1 confers tumour cells with epidermal growth factor receptor-tyrosine kinase inhibitors (EGFR-TKIs) resistance (10). Similarly, upregulation of LINC01116 markedly promoted cell proliferation and migration, inhibited cell apoptosis, and accelerated progression through the cell cycle (11). Taken together, FAM83A−AS1 and LINC01116 are involved in the development and metastasis of LUAD and may be promising biomarkers for LUAD diagnosis, prognosis, and therapy. Compared with the TNM stage, a direct factor associated with the prognosis of LUAD, lncRNAs can be extracted from serum causing no additional trauma to the patients. Thus, a better understanding of lncRNAs in LUAD may provide additional evidence for prognostic biomarkers and therapeutic targets for LUAD.
Autophagy is the degradation of intracellular lysosomes and the circulation of proteins and organelles (12) controlled by autophagy-related genes (ARGs). It has been implicated in several disease processes, including cancer, neurodegenerative disorders, autoimmune diseases, cell death, and cardiovascular diseases (13-16). In cancer development, autophagy is a double-edged sword, functioning as a tumour suppressor in the early stages, while promoting tumorigenesis and causing resistance to therapeutic agents in the advanced stages (17). Autophagy is regulated by several cellular molecules and signalling pathways, including ARGs and lncRNAs. Therefore, this study aimed to construct a risk model based on autophagy-related lncRNAs that could be utilised for clinical prediction and drug selection.
We present the following article in accordance with the TRIPOD reporting checklist (available at https://tcr.amegroups.com/article/view/10.21037/tcr-21-1554/rc).
Methods
Data acquisition and processing
The LUAD RNA-seq (FPKM) data, tumour mutation burden (TMB), and corresponding clinical information were downloaded from the TCGA database (https://portal.gdc.cancer.gov/). The cohort contained 497 tumour tissues and 54 normal tissues, with the complete clinical information of 486 patients (tumour =439, normal =47) extracted as an entire set for further analysis. To increase the study reliability, the entire set (only tumour tissues) was randomly divided into a training set (accounting for 70%, n=307) and a validation set (accounting for 30%, n=132). The “rtracklayer” and “dplyr” R packages were applied to convert gene names from Ensemble IDs to a profile of gene symbols with the Ensemble database.
Identification of autophagy-related lncRNAs
Two hundred and thirty-two ARGs were extracted from the human autophagy database (HADb) analysis. Pearson’s correlation analysis of the expression of lncRNAs and ARGs was performed through the “limma” R package and 312 lncRNAs with high correlation (|R| >0.3, P<0.001) with ARGs were identified as autophagy-related lncRNAs (ARlncRNAs).
Identification of an ARlncRNAs prognostic signature
The tumour and normal tissues were screened for the differentially expressed ARlncRNAs via the “limma” R package with thresholds set at |logFC| >1 and P<0.05. Then, to identify ARlncRNAs associated with survival, univariate cox regression analysis was performed according to the criteria of P<0.01 using the “survival” R package. Subsequently, multivariate cox regression analysis was conducted to construct the prognostic signature based on the lowest Akaike information criterion (AIC). The risk score formula is as follows: Risk score = ∑Coef (ARlncRNAs) * Exp (ARlncRNAs). The Coef (ARlncRNAs) represent the coefficient of each ARlncRNA and Exp (ARlncRNA) is the expression of each ARlncRNA. Based on the median risk score, the patients were divided into high-risk and low-risk groups, with Kaplan-Meier survival analysis performed to estimate the survival rate of both groups using the “survival” and “survminer” R packages. The receiver operating characteristic (ROC) curve was used to assess the prognostic performance through the “timeROC” R package.
Independent prognostic analysis and ROC curve
To assess the relationship of survival prognosis with clinicopathological factors (age, gender, AJCC stage, TNM stage, tobacco history, and anatomical location) and risk score, univariate and multivariate cox regression analyses were performed, respectively, using the “survival” R package. Furthermore, a time-dependent ROC curve was used to evaluate the predictive accuracy for survival time by different clinicopathological factors and risk scores using the “survivalROC” R package.
Construction and assessment of a nomogram
A nomogram incorporating the lncRNA signature and clinical factors was created using the “rms” R package to simplify the predictive model. Calibration curves were also plotted to assess whether the predicted survival in the nomogram agreed with the actual survival.
Principal components analysis (PCA) and gene set enrichment analysis (GSEA)
PCA was used to test the differentiation of patients into low- and high-risk groups, with GSEA performed to evaluate different functional phenotypes between low- and high-risk groups.
Construction of the lncRNA-mRNA co-expression network and functional enrichment analysis
A co-expression network of the autophagy-related lncRNA-mRNAs with prognostic value was established according to the criteria of |R| >0.3 and P<0.001 by Pearson correlation analysis using the “limma” R package. To further explore the functional annotation and pathway analysis of the target mRNAs, Gene Ontology (GO) and Kyoto Encyclopedia of Genes and Genomes (KEGG) enrichment analyses were performed using the “clusterProfiler” R package with the criteria of P<0.05 and FDR <0.05.
Identification of potential immunotherapy
To explore the value of our signature for predicting the efficacy of immunotherapy in LUAD, immune cell infiltration was first estimated using CIBERSORT. Then, we assessed the TMB of LUAD patients in the high-risk and low-risk groups. The Cmap database (18) uses gene-expression signatures to predict small molecular compounds for a specific disease. In the present study, the target mRNAs were divided into two groups, up- and downregulated target genes, which were uploaded from the lncRNA-mRNAs network to Cmap. A connectivity score ranging from −1 to 1 was used to reflect the degree of closeness between the expression spectrums. Drugs with negative scores were potential therapeutic molecules, so were further investigated in the PubChem database (19).
Statistical analysis
All statistical analyses and plotting were performed in the R language (Version 3.6.3). Univariate and multivariate cox regression analyses were used to identify independent prognostic factors for LUAD, through the criteria of P<0.05 and the lowest AIC respectively. Kaplan-Meier survival analysis was performed in the high-risk and low-risk groups and evaluated using a log-rank test. A two-sided P value <0.05 was considered statistically significant.
Ethical statement
The study was conducted in accordance with the Declaration of Helsinki (as revised in 2013).
Results
Identification of ARlncRNAs with significant prognostic value in LUAD
The flow chart is shown in Figure 1. First, a total of 14,064 lncRNAs were extracted from the TCGA dataset, 1,639 of which were identified as ARlncRNAs by Pearson correlation analysis (|R| >0.3, P<0.001). After differential expression analysis of the entire set, 312 ARlncRNAs were selected as shown in Figure 2. Among them, 10 ARlncRNAs were significantly associated with the survival of LUAD patients from the TCGA (P<0.01) by univariate cox regression analysis, namely, ILF3-DT, EBLN3P, AL161785.1, FAM83A-AS1, AL049555.1, CRNDE, CH17-340M24.3, LINC01116, AC092279.1, and AC026355.2. Subsequently, multivariate cox regression analysis further identified seven lncRNAs with prognostic significance based on the lowest AIC (1,037.67) (Figure 2B). Among them, FAM83A−AS1 and LINC01116 were considered as risk factors with a hazard ratio (HR) greater than 1, whereas ILF3-DT, EBLN3P, AL161785.1, AC092279.1, and AC026355.2 were considered as protective factors with HR values less than 1 (Figure 2C). The risk score for each patient was calculated using the following formula for the ARlncRNAs signature: risk score = (–0.039 * expression level of ILF3-DT) + (–0.038 * expression level of EBLN3P) + (–0.079 * expression level of AL161785.1) + (0.033 * expression level of FAM83A-AS1) + (0.069 * expression level of LINC01116) + (–0.165 * expression level of AC092279.1) + (–0.225 * expression level of AC026355.2). Next, based on the risk score formula and the calculated median risk score, the patients in the training cohort (n=307) and validation cohort (n=132) were divided into high-risk and low-risk groups. The risk distribution, survival status, and gene expression pattern of the two cohorts are shown in Figure 3. The heatmap (Figure 3A,3B) showed that two ARlncRNAs are highly expressed in the high-risk group, with the other five ARlncRNAs highly expressed in the low-risk group. The risk curve and scatterplot were applied to illustrate the risk score and the relevant survival status, indicating that the mortality occurrence depended on the risk score (Figure 3A,3B). The Kaplan-Meier curve demonstrated that the OS of the high-risk group was significantly poorer than that of the low-risk group (P=3.283e−05 in the training cohort and P=1.044e-03 in the validation cohort; Figure 3C,3D), indicating that the risk score has prognostic value. PCA was employed to demonstrate the distribution difference between the high- and low-risk groups based on the risk model (Figure 3D,3H), with satisfactory results. Time-dependent ROC curves revealed that the signature performed well in predicting OS. The AUC at 1-, 3-, and 5-year in the training cohort was 0.744, 0.679, and 0.698, and 0.671, 0.82, and 0.857 in the validation cohort (Figure 3E,3G).
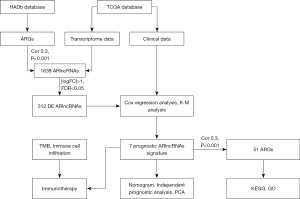
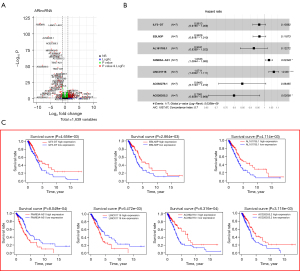

Evaluation of the risk model of seven ARlncRNAs as an independent prognostic factor
Univariate and multivariate cox regression analyses were performed in the training set to determine whether the signature was an independent prognostic factor, showing that AJCC stage (P<0.001), T stage (P=0.002), N stage (P<0.001), and risk score (P<0.001) were significantly related to OS in univariate cox regression analysis (Figure 4A), with only the AJCC stage (P<0.001) and risk score (P<0.001) significantly related to OS in multivariate cox regression analysis (Figure 4B). As shown in Figure 4C, the AUC of risk score was 0.721, which was higher than the AUC of the AJCC stage, T stage, and N stage, indicating that the prognostic risk model was relatively reliable. Taken together, the risk model of seven ARlncRNAs is an independent prognostic factor for LUAD patients.
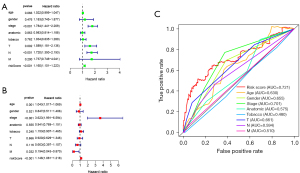
Correlation of the ARlncRNAs prognosis signature to other clinicopathological characteristics
To further evaluate whether the seven ARlncRNAs were involved in the occurrence and development of LUAD, we explored the relationship between the risk score and clinicopathological factors (Table 1), showing that patients without lymphatic metastasis had a lower risk than those with lymphatic metastasis (P<0.05).
Table 1
Clinical | Group | n | Mean | SD | t | P |
---|---|---|---|---|---|---|
Age | ≤65 | 138 | 1.452 | 2.518 | 1.525013 | 0.129 |
>65 | 149 | 1.168 | 0.793 | |||
Gender | Female | 164 | 1.168 | 0.977 | −1.5607 | 0.12 |
Male | 123 | 1.479 | 2.548 | |||
Stage | Stage I-II | 223 | 1.167 | 0.835 | −1.67197 | 0.098 |
Stage III-IV | 64 | 1.85 | 3.721 | |||
Anatomic | Left | 122 | 1.467 | 2.646 | 1.219104 | 0.224 |
Right | 165 | 1.202 | 1.041 | |||
Tobacco | Never | 46 | 1.153 | 0.53 | −1.4186 | 0.157 |
Smoke | 241 | 1.333 | 1.992 | |||
T | T1-2 | 256 | 1.189 | 0.833 | −1.4276 | 0.16 |
T3-4 | 31 | 2.175 | 4.82 | |||
N | N0 | 195 | 1.106 | 0.788 | −2.33317 | 0.021 |
N1-3 | 92 | 1.749 | 3.069 | |||
M | M0 | 273 | 1.284 | 1.812 | −0.84766 | 0.409 |
M1 | 92 | 1.848 | 2.716 |
Evaluation of the prognostic prediction nomogram
The nomogram visualises the regression analyses results and is commonly used by clinicians to predict the risk of disease or survival (20). A nomogram was constructed to accurately evaluate 1-, 3-, and 5-year survival probabilities using the risk score calculated from the ARlncRNAs prognostic signature and other clinicopathological characteristics, including age, gender, anatomical location, AJCC stage, tobacco history, T stage, N stage, and M stage (Figure 5A). The calibration plot analysis showed a high degree of consistency between the actual and the predicted 1-, 3-, and 5-year OS when compared to the reference line (Figure 5B-5D), suggesting that the nomogram was accurate and reliable.
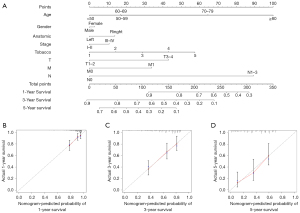
Gene set enrichment analysis
GSEA was used to conduct the functional annotation revealing that several autophagy-related signalling pathways were significantly enriched, such as “endocytosis”, the “ErbB signalling pathway”, “Notch signalling pathway”, “p53 signalling pathway”, “pathway in cancer”, “TGF-β signalling pathway” etc. (Figure 6).
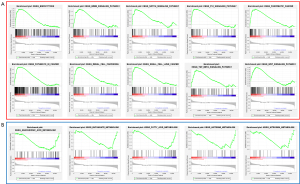
Construction of the lncRNA-mRNA co-expression network and functional enrichment analysis
To explore the regulation of the ARlncRNAs, a co-expression network consisting of seven ARlncRNAs and 51 ARGs was constructed using the Cytoscape software (Figure 7A). The Sankey diagram showed the correlation between the 51 ARGs and 7 ARlncRNAs (Figure 7B). To further investigate the function of the network, GO and KEGG analyses were performed. The top GO terms were “autophagy” in biological process (BP), “plasma membrane-bounded cell projection cytoplasm” in cellular component (CC), and “cysteine-type peptidase activity” in molecular function (MF), respectively (Figure 7C). According to KEGG analysis, “autophagy”, the “longevity regulating pathway”, “FoxO signalling pathway”, “HIF-1 signalling pathway”, “NOD-like receptor signalling pathway”, “PD-L1 expression and PD-1 checkpoint pathway” were the main pathways (Figure 7D). Taken together, the regulatory network may lead to the pathogenesis of LUAD through multiple signalling pathways.
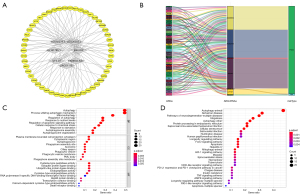
Identification of potential immunotherapy
Immune cell infiltration was estimated in LUAD patients using CIBERSORT to explore the possible relationship between the signature and potential immunotherapy, showing that among the 22 types of immune cells, plasma cells, resting dendritic cells, and resting mast cells had significant negative correlations with the risk score, while M0 macrophages and activated mast cells were positively correlated with the risk score (Figure 8A), implying the potential of the signature to reflect the tumour immune microenvironment (TIME). Moreover, there was a significant difference in the expression of TMB between the high- and low-risk groups (Figure 8B), indicating that immunotherapy may be a potentially effective treatment based on the signature. The 31 upregulated and 20 downregulated ARGs were analysed in Cmap and the results with P<0.05 were regarded as potential small molecule drugs (Figure 8C). The chemical structures of the top six agents, namely, MS-275 (C21H20N4O3), methotrexate (C20H22N8O5), desipramine (C18H22N2), benzbromarone (C17H12Br2O3), rifampicin (C43H58N4O12), and doxazosin (C23H25N5O5) are shown in Figure 8D.
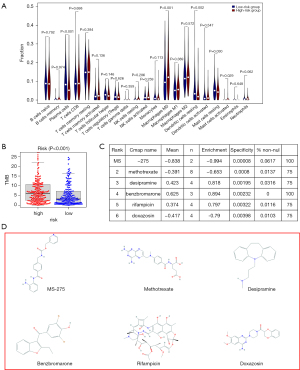
Discussion
Lung cancer is the leading cause of cancer morbidity and mortality in the world (1). For early stage LUAD patients, surgery is the recommended treatment (21), whereas chemotherapy, radiotherapy, immunotherapy, and targeted therapy are recommended for advanced LUAD patients (22). Several studies have demonstrated a strong association between TMB and the clinical benefits of receiving immunotherapy (23,24). Moreover, TIME is closely correlated to the efficacy of immunotherapy (25,26), suggesting that immunotherapy may be more effective for high-risk patients based on the risk model.
Autophagy plays a dual role at different phases of cancer development (17). Recently, regulating autophagy to improve cancer therapeutic effects has attracted great interest (27), thus, an autophagy-related signature could be used as a prognostic biomarker and to predict potential small molecular medicine for LUAD patients.
In the present study, we constructed an ARlncRNAs prognostic signature consisting of seven ARlncRNAs, in which, FAM83A−AS1 was upregulated in LUAD tumour tissues and could promote LUAD cell migration and invasion (10) and LINC01116 was associated with gefitinib and cisplatin resistance in LUAD (28,29), indicating that they could be novel biomarkers for LUAD diagnosis, prognosis, and therapy. The risk score was used to classify the training and validation cohorts into high- and low-risk groups, with the low-risk group having a better survival outcome than the high-risk group. The signature was shown to be an independent prognostic factor and more powerful in survival prediction than other clinical indicators, thus having great potential for future clinical application.
Cancer initiation and progression is regulated by multiple signalling pathways, including the ErbB signalling pathway. The erbB family of receptor tyrosine kinases include HER1 (EGFR), HER2 (erbB2), HER3 (erbB3), and HER4 (erbB4). The HER2 gene is associated with breast cancer and EGFR is highly expressed in more than 60% of NSCLCs, regulating the proliferation, survival, and motility of the tumour cells. Thus, inhibitors that target the kinase domain of EGFR have been developed and are clinically active (30). The GSEA results showed that the signature was strongly associated with the “erbB signalling pathway”, which could guide the follow-up treatment of LUAD patients.
There are usually co-expression relationships between genes, so a lncRNA-mRNA co-expression network was established for functional enrichment analysis to explore the biological mechanisms of the seven selected ARlncRNAs. Cellular senescence was enriched in the network and has been shown to restrain the cell proliferation and transformation induced by oncogene activation (31). Autophagy was also demonstrated to be necessary for the establishment of cellular senescence, so, it might be a promising therapy for LUAD by targeting the regulation of autophagy.
Importantly, we confirmed that the signature could potentially reflect the TIME and there was a significant difference in the expression of TMB between the two groups. Six small molecular drugs, namely, MS-275, methotrexate, desipramine, benzbromarone, rifampicin and doxazosin were identified from the prediction of Cmap based on the up- and downregulated ARGs. MS-275, also called entinostat, the histone deacetylase inhibitor (HDACi), is a promising drug for the treatment of breast cancer in different clinical settings. Specifically, entinostat may offer better safety through targeting class I HDACs (HDAC 1 and 3) than those nonselective broad-spectrum HDAC inhibitors. So far, there have no HDAC inhibitors been approved for NSCLC, however, entinostat alone or in combination with other molecular targeted therapies for NSCLC may be an option (32-34). Methotrexate (MTX), an anti-folate agent, is one of the most widely used and effective drugs for treating cancer, including lung cancer. However, high doses of MTX usually lead to serious side effects. Nowadays, some carriers have been loaded with MTX to improve drug safety (35-37). Desipramine, an antidepressant, is commonly used in combination regimens, not only helping to relieve depression but also enhancing the cytotoxicity of the platinum agents (38). Benzbromarone, a nonpurine xanthine oxidase inhibitor for the treatment of gout, is promising for the treatment of tumour metastasis, angiogenesis, and angiopathy (39,40). Rifampicin, a common antibiotic used for tuberculosis exerts an anti-tumour effect through direct antitumor activities when highly concentrated and antiangiogenic properties (41). Doxazosin is an α1-adrenoceptor antagonist used to treat hypertension or benign prostatic hyperplasia. Moreover, the potential of doxazosin as an osimertinib sensitiser affecting many cancers via the upregulation of autophagy was also demonstrated (42,43). Taken together, these findings may provide potential therapeutic options for LUAD patients.
Recently, the prognostic signatures of ARlncRNAs were established in several cancers (44-46), however, the role of ARlncRNAs in the prognosis of LUAD remains unclear. Thus, we focused on the essential role of ARlncRNAs in tumour malignancy to construct an ARlncRNA signature for the LUAD cohort, demonstrating that it has independent prognostic value, as well as exploring the clinical utility in potential immunotherapy and targeted therapy for LUAD patients.
Nonetheless, the study has several limitations. First, the ARlncRNAs prognosis signature was constructed based on the publicly available TCGA database. Secondly, our findings need to be proved in other independent cohorts to improve the predictive efficacy. Moreover, biochemical experiments such as immunohistochemistry, quantitative real-time PCR, and flow cytometry need to be conducted to confirm the findings.
Conclusions
A prognostic signature of seven ARlncRNAs was constructed for the LUAD cohort to explore the role of autophagy in tumour malignancy, with various potential therapeutic regimens proposed based on the immune cell infiltration and differential expression of TMB.
Acknowledgments
Funding: None.
Footnote
Reporting Checklist: The authors have completed the TRIPOD reporting checklist. Available at https://tcr.amegroups.com/article/view/10.21037/tcr-21-1554/rc
Conflicts of Interest: All authors have completed the ICMJE uniform disclosure form (available at https://tcr.amegroups.com/article/view/10.21037/tcr-21-1554/coif). The authors have no conflicts of interest to declare.
Ethical Statement: The authors are accountable for all aspects of the work in ensuring that questions related to the accuracy or integrity of any part of the work are appropriately investigated and resolved. The study was conducted in accordance with the Declaration of Helsinki (as revised in 2013).
Open Access Statement: This is an Open Access article distributed in accordance with the Creative Commons Attribution-NonCommercial-NoDerivs 4.0 International License (CC BY-NC-ND 4.0), which permits the non-commercial replication and distribution of the article with the strict proviso that no changes or edits are made and the original work is properly cited (including links to both the formal publication through the relevant DOI and the license). See: https://creativecommons.org/licenses/by-nc-nd/4.0/.
References
- Nasim F, Sabath BF, Eapen GA. Lung Cancer. Med Clin North Am 2019;103:463-73. [Crossref] [PubMed]
- Denisenko TV, Budkevich IN, Zhivotovsky B. Cell death-based treatment of lung adenocarcinoma. Cell Death Dis 2018;9:117. [Crossref] [PubMed]
- Nawa T. Low-dose CT screening for lung cancer reduced lung cancer mortality in Hitachi City. Int J Radiat Biol 2019;95:1441-6. [Crossref] [PubMed]
- Hamann HA, Ver Hoeve ES, Carter-Harris L, et al. Multilevel Opportunities to Address Lung Cancer Stigma across the Cancer Control Continuum. J Thorac Oncol 2018;13:1062-75. [Crossref] [PubMed]
- Qian X, Zhao J, Yeung PY, et al. Revealing lncRNA Structures and Interactions by Sequencing-Based Approaches. Trends Biochem Sci 2019;44:33-52. [Crossref] [PubMed]
- Rathinasamy B, Velmurugan BK. Role of lncRNAs in the cancer development and progression and their regulation by various phytochemicals. Biomed Pharmacother 2018;102:242-8. [Crossref] [PubMed]
- Huarte M, Guttman M, Feldser D, et al. A large intergenic noncoding RNA induced by p53 mediates global gene repression in the p53 response. Cell 2010;142:409-19. [Crossref] [PubMed]
- Feng C, Zhao Y, Li Y, et al. LncRNA MALAT1 Promotes Lung Cancer Proliferation and Gefitinib Resistance by Acting as a miR-200a Sponge. Arch Bronconeumol 2019;55:627-33. (Engl Ed). [PubMed]
- Loewen G, Jayawickramarajah J, Zhuo Y, et al. Functions of lncRNA HOTAIR in lung cancer. J Hematol Oncol 2014;7:90. [Crossref] [PubMed]
- Xiao G, Wang P, Zheng X, et al. FAM83A-AS1 promotes lung adenocarcinoma cell migration and invasion by targeting miR-150-5p and modifying MMP14. Cell Cycle 2019;18:2972-85. [Crossref] [PubMed]
- Zeng L, Lyu X, Yuan J, et al. Long non-coding RNA LINC01116 is overexpressed in lung adenocarcinoma and promotes tumor proliferation and metastasis. Am J Transl Res 2020;12:4302-13. [PubMed]
- White E, Mehnert JM, Chan CS. Autophagy, Metabolism, and Cancer. Clin Cancer Res 2015;21:5037-46. [Crossref] [PubMed]
- Bialik S, Dasari SK, Kimchi A. Autophagy-dependent cell death - where, how and why a cell eats itself to death. J Cell Sci 2018;131:jcs215152. [Crossref] [PubMed]
- Bravo-San Pedro JM, Kroemer G, Galluzzi L. Autophagy and Mitophagy in Cardiovascular Disease. Circ Res 2017;120:1812-24. [Crossref] [PubMed]
- Ghavami S, Shojaei S, Yeganeh B, et al. Autophagy and apoptosis dysfunction in neurodegenerative disorders. Prog Neurobiol 2014;112:24-49. [Crossref] [PubMed]
- Yin H, Wu H, Chen Y, et al. The Therapeutic and Pathogenic Role of Autophagy in Autoimmune Diseases. Front Immunol 2018;9:1512. [Crossref] [PubMed]
- Li X, He S, Ma B. Autophagy and autophagy-related proteins in cancer. Mol Cancer 2020;19:12. [Crossref] [PubMed]
- Lamb J, Crawford ED, Peck D, et al. The Connectivity Map: using gene-expression signatures to connect small molecules, genes, and disease. Science 2006;313:1929-35. [Crossref] [PubMed]
- Wang Y, Xiao J, Suzek TO, et al. PubChem's BioAssay Database. Nucleic Acids Res 2012;40:D400-12. [Crossref] [PubMed]
- Wang S, Yang L, Ci B, et al. Development and Validation of a Nomogram Prognostic Model for SCLC Patients. J Thorac Oncol 2018;13:1338-48. [Crossref] [PubMed]
- Vansteenkiste J, Crinò L, Dooms C, et al. 2nd ESMO Consensus Conference on Lung Cancer: early-stage non-small-cell lung cancer consensus on diagnosis, treatment and follow-up. Ann Oncol 2014;25:1462-74. [Crossref] [PubMed]
- Hirsch FR, Scagliotti GV, Mulshine JL, et al. Lung cancer: current therapies and new targeted treatments. Lancet 2017;389:299-311. [Crossref] [PubMed]
- Samstein RM, Lee CH, Shoushtari AN, et al. Tumor mutational load predicts survival after immunotherapy across multiple cancer types. Nat Genet 2019;51:202-6. [Crossref] [PubMed]
- Proto C, Ferrara R, Signorelli D, et al. Choosing wisely first line immunotherapy in non-small cell lung cancer (NSCLC): what to add and what to leave out. Cancer Treat Rev 2019;75:39-51. [Crossref] [PubMed]
- Frankel T, Lanfranca MP, Zou W. The Role of Tumor Microenvironment in Cancer Immunotherapy. Adv Exp Med Biol 2017;1036:51-64. [Crossref] [PubMed]
- Lei X, Lei Y, Li JK, et al. Immune cells within the tumor microenvironment: Biological functions and roles in cancer immunotherapy. Cancer Lett 2020;470:126-33. [Crossref] [PubMed]
- White E. The role for autophagy in cancer. J Clin Invest 2015;125:42-6. [Crossref] [PubMed]
- Wang H, Lu B, Ren S, et al. Long Noncoding RNA LINC01116 Contributes to Gefitinib Resistance in Non-small Cell Lung Cancer through Regulating IFI44. Mol Ther Nucleic Acids 2020;19:218-27. [Crossref] [PubMed]
- Wang J, Gao J, Chen Q, et al. LncRNA LINC01116 Contributes to Cisplatin Resistance in Lung Adenocarcinoma. Onco Targets Ther 2020;13:9333-48. [Crossref] [PubMed]
- Aran V, Omerovic J. Current Approaches in NSCLC Targeting K-RAS and EGFR. Int J Mol Sci 2019;20:5701. [Crossref] [PubMed]
- Di Micco R, Fumagalli M, Cicalese A, et al. Oncogene-induced senescence is a DNA damage response triggered by DNA hyper-replication. Nature 2006;444:638-42. [Crossref] [PubMed]
- Hicks KC, Knudson KM, Lee KL, et al. Cooperative Immune-Mediated Mechanisms of the HDAC Inhibitor Entinostat, an IL15 Superagonist, and a Cancer Vaccine Effectively Synergize as a Novel Cancer Therapy. Clin Cancer Res 2020;26:704-16. [Crossref] [PubMed]
- Ruiz R, Raez LE, Rolfo C. Entinostat (SNDX-275) for the treatment of non-small cell lung cancer. Expert Opin Investig Drugs 2015;24:1101-9. [Crossref] [PubMed]
- Trapani D, Esposito A, Criscitiello C, et al. Entinostat for the treatment of breast cancer. Expert Opin Investig Drugs 2017;26:965-71. [Crossref] [PubMed]
- Álvarez-González B, Rozalen M, Fernández-Perales M, et al. Methotrexate Gold Nanocarriers: Loading and Release Study: Its Activity in Colon and Lung Cancer Cells. Molecules 2020;25:6049. [Crossref] [PubMed]
- Koźmiński P, Halik PK, Chesori R, et al. Overview of Dual-Acting Drug Methotrexate in Different Neurological Diseases, Autoimmune Pathologies and Cancers. Int J Mol Sci 2020;21:3483. [Crossref] [PubMed]
- Yang W, Xia Y, Fang Y, et al. Selective Cell Penetrating Peptide-Functionalized Polymersomes Mediate Efficient and Targeted Delivery of Methotrexate Disodium to Human Lung Cancer In Vivo. Adv Healthc Mater 2018;7:e1701135. [Crossref] [PubMed]
- Kabolizadeh P, Engelmann BJ, Pullen N, et al. Platinum anticancer agents and antidepressants: desipramine enhances platinum-based cytotoxicity in human colon cancer cells. J Biol Inorg Chem 2012;17:123-32. [Crossref] [PubMed]
- Tadjuidje E, Wang TS, Pandey RN, et al. The EYA tyrosine phosphatase activity is pro-angiogenic and is inhibited by benzbromarone. PLoS One 2012;7:e34806. [Crossref] [PubMed]
- Azevedo VF, Kos IA, Vargas-Santos AB, et al. Benzbromarone in the treatment of gout. Adv Rheumatol 2019;59:37. [Crossref] [PubMed]
- Shichiri M, Tanaka Y. Inhibition of cancer progression by rifampicin: involvement of antiangiogenic and anti-tumor effects. Cell Cycle 2010;9:64-8. [Crossref] [PubMed]
- McConnell JD, Roehrborn CG, Bautista OM, et al. The long-term effect of doxazosin, finasteride, and combination therapy on the clinical progression of benign prostatic hyperplasia. N Engl J Med 2003;349:2387-98. [Crossref] [PubMed]
- Suzuki S, Yamamoto M, Sanomachi T, et al. Doxazosin, a Classic Alpha 1-Adrenoceptor Antagonist, Overcomes Osimertinib Resistance in Cancer Cells via the Upregulation of Autophagy as Drug Repurposing. Biomedicines 2020;8:273. [Crossref] [PubMed]
- Li X, Jin F, Li Y. A novel autophagy-related lncRNA prognostic risk model for breast cancer. J Cell Mol Med 2021;25:4-14. [Crossref] [PubMed]
- Luan F, Chen W, Chen M, et al. An autophagy-related long non-coding RNA signature for glioma. FEBS Open Bio 2019;9:653-67. [Crossref] [PubMed]
- Sun Z, Jing C, Xiao C, et al. An autophagy-related long non-coding RNA prognostic signature accurately predicts survival outcomes in bladder urothelial carcinoma patients. Aging (Albany NY) 2020;12:15624-37. [Crossref] [PubMed]