Ferroptosis related genes are regulated by methylation and predict the prognosis of glioblastoma patients
Introduction
Glioma is the most common primary tumor in central nervous system, accounting for 80% of all intracranial tumors (1). The World Health Organization (WHO) divides diffuse gliomas into WHO grade II–III astrocytoma and oligodendroglioma, grade IV glioblastoma, and childhood-related diffuse glioma (2). Different grades of gliomas differ greatly in tumor pathology, malignant potential, treatment response and survival (3). Glioblastoma multiforme (GBM) (WHO IV), accounts for 50% of all gliomas, is the most aggressive type of primary brain tumor (4). The median overall survival of GBM patients is only 12 to 14 months, 3% to 5% of patients can survive for more than 3 years, is called the long-term survivor (5-7). However, the clinical and molecular factors related to long-term survival are still scarce. Exploring changes in the genome and transcriptome and identifying aberrantly functioning molecular pathways of GBM may deepen our understanding of GBM. Currently, only isocitrate dehydrogenases (IDH) mutation status, O6-methylguanine-DNA methyltransferase (MGMT) gene promoter methylation and joint deletion of chromosomes 1p and 19q are widely recognized (3,8,9). The differences in expression levels or genomic changes of tumor driver gene also reflect survival time of GBM patients.
The ferroptotic pathway is regulated by the complex mechanisms of epigenetic, transcription, post-transcriptional, and post-translation, including DNA methylation (10,11). DNA methylation can be found in about 80–90% of CpG islands in human genome (12) and participates in the regulation of various cell activities such as proliferation, apoptosis, invasion and differentiation. DNA methylation abnormalities exist in almost all forms of human cancer (13). Abnormal methylation in gene promoter might lead to the silencing of tumor suppressor genes or the activation of oncogenes, affecting signal transduction pathways and participating in cancer development (14). Heterogeneity of DNA methylation in tumors has been shown to be a feature of GBM (15). Researchers have found that methylated genes can be used as potential biomarkers to assess the malignancy of GBM (16,17).
Ferroptosis, proposed by Stockwell in 2012, is a newly programmed cell death modalities, achieved by phospholipid peroxidation, which relies on transition metal iron, reactive oxygen species and phospholipids containing polyunsaturated fatty acid chains (18,19). The proliferation ability of tumor cells is significantly stronger than that of non-malignant cells, which greatly increases their iron requirements. This strong iron dependence makes tumor cells more susceptible to ferroptosis (20). Although there have been researches on ferroptosis in tumor, there is no systematic report on the expression, prognostic value, and genomic and epigenetic regulatory characteristics of ferroptosis related genes in GBM at the same time. In this study, we performed survival analysis of ferroptosis related genes in the Cancer Genome Atlas (TCGA) and Chinese Glioma Cooperative Group (CGCG) cohort, and built a risk-gene signature act as an independent prognostic factor. Moreover, we verified the expression of these 16 genes in GBM tumor and paired normal tissues with real-time polymerase chain reaction (RT-qPCR), and explored the reasons for regulating these genes at the level of genomic and epigenetics. We present the following article in accordance with the STREGA reporting checklist (available at https://tcr.amegroups.com/article/view/10.21037/tcr-21-2470/rc).
Methods
Specimen collection
The study protocol was approved by the Beijing Chaoyang Hospital, Capital Medical University, Beijing, China. All 30 pairs of GBM tumor tissues and matched non-tumor tissues used in this study were recruited in the Beijing Chaoyang Hospital. All cases were diagnosed as GBM built on histopathology, confirmed by more than two pathologists according to the WHO guidelines. Surgically resected tissue samples were quickly placed in liquid nitrogen and then stored in a −80 ℃ freezer. One sample from each of the four regions was taken from each surgically resected specimen and performed frozen sectioning and hematoxylin and eosin (H&E) staining to assess the percentage of tumor cells and to exclude specimens with less than 50% tumor cells. Multiple tumor tissues from the same patient were pooled for RNA extraction to avoid intratumor heterogeneity.
Data collection
mRNA-seq data, DNA methylation data by 450K BeadChip, copy number variation (CNV) annotation by GISTIC and corresponding clinical data of TCGA cohort were collection from the UCSC Xena (https://xena.ucsc.edu/). mRNA-seq data and corresponding clinical data of CGCG cohort were collection from CGCG database (http://www.cgga.org.cn/). A total of 335 ferroptosis related genes were collection from FerrDb database (http://www.zhounan.org/ferrdb; table available at https://cdn.amegroups.cn/static/public/tcr-21-2470-1.pdf).
Identification of survival related signatures and construction of risk gene signature
We first used univariate Cox regression analysis to identify ferroptosis related genes related to the survival of GBM patients. Then, the most suitable ferroptosis related genes was selected through least absolute shrinkage and selection operator (LASSO) analysis to avoid overfitting, and these genes were included in the multivariate Cox analysis to construct a prognostic model. By linearly combining the expression level of the selected ferroptosis related genes and the regression coefficient of the multivariate Cox regression analysis, the risk score model is established as risk score = Ʃ(bi * Expi), where bi represents the weight of the respective signature and Expi represents the expression value.
Tumor immune infiltration analysis
We used ESTIMATE to calculate the stroma and immune score in each GBM sample to predict the level of infiltrating stroma and immune cells. Moreover, we performed CIBERSORT to assess the degree of tumor immune infiltration of 22 immune cells and combine these immune cells into several major cell types, including CD4+ T cells, CD8+ T cells, B cells, macrophages and neutrophils. The correlation between immune infiltration score and risk score was calculated by Pearson’s correlation coefficient.
Differential expression analysis and enrichment analysis
We used Limma package (3.48.3) to estimate differential expression genes between higher and lower risk group in GBM. Genes with false discovery rate (FDR) <0.05 and absolute value of fold change >1 were identified as differential expression gene. Gene Ontology and Kyoto Encyclopedia of Genes and Genomes analysis was used to annotation the biological function enrichment of the differential expression genes and FDR <0.05 was considered as statistically significant.
RNA preparation and RT-qPCR analysis
Total RNA was isolated from tissue samples and cell lines using TRIzol (Invitrogen). First-strand cDNA synthesis of total RNA was performed using the PrimeScriptTM RT reagent Kit with gDNA Eraser (TaKaRa). qRT-PCR was accomplished on the ABI Prism 7900 system (Applied Biosystems) using SYBR Green (TaKaRa) method with the gene specific primers showed in Table S1. Measurement of individual mRNA expression was determined relative to the levels of GAPDH transcript. Three independent transfection experiments were performed, and each had three replicates.
Statistical analysis
Paired student t-test was used to compare statistically significant differences between the gene expression of tumor and normal tissues. All assays were performed using R (4.0.2) and SPSS 22.0 software, P<0.05 being the statistically significant difference criterion.
Ethical statement
The study was conducted in accordance with the Declaration of Helsinki (as revised in 2013).
Results
Prognostic related ferroptosis related genes
For ferroptosis related genes, we first identified 187 genes that are differentially expressed in GBM tumors and normal tissues (84 up-regulated and 103 down-regulated) in TCGA cohorts, as shown in Figure 1A. These differentially expressed genes can distinguish tumors from matched normal tissues (Figure 1B,1C).
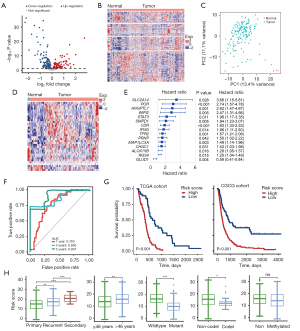
In order to explore the correlation between ferroptosis related genes and the survival of GBM patients, we found that 39 of the 335 ferroptosis related genes were significantly related to the overall survival of GBM through univariate Cox-regression analysis. Among them, 36 genes had a hazard ratio (HR) >1, which was considered risk genes, and the remaining 3 genes with HR <1, were considered protective genes. Similar to the total ferroptosis related genes, these survival-related genes showed different expression patterns in tumors and normal tissues (Figure 1D). Next, we used the LASSO Cox regression algorithm to further analyze the 39 survival-related genes in the TCGA data set to avoid data overfitting. Fifteen ferroptosis related genes were selected as prognostic factors for GBM patients to construct risk characteristics, and the risk score was obtained by combining the risk coefficients and expression values of these genes (Figure 1E). We used the receiver operating characteristic (ROC) curve to examine whether the risk score could provide early predictions for the prognosis of GBM patients. In the TCGA cohort, the 1-, 3-, and 5-year survival areas under the curve (AUCs) of risk score were 0.763, 0.856, and 0.907, respectively, indicating a well effective prediction accuracy of the risk score for the prognosis of GBM (Figure 1F). The median of the risk score was used as a cut-off value to divide patients into high-risk and low-risk groups. Regardless of the TCGA cohort or the CGCG cohort, the overall survival of patients in the low-risk group was significantly longer than in the high-risk group (Figure 1G). Patients with recurrent/secondary, advanced age, IDH wild type and 1p/19q noncodel had higher risk scores. The status of the MGMT promoter did not affect the patient’s risk score. In univariate Cox regression analysis, we found that risk score, radiotherapy, chemotherapy, histology, IDH, and 1p/19q status were significantly related to the survival of GBM patients, and multivariate Cox regression analysis indicated that risk score was independently related to patients’ survival (Table S2). Furthermore, histology, age, IDH status and 1p/19q status were all significant different between high- and low-risk patients. Risk scores were higher in patients whom were recurrent, older, IDH wild-type or 1p/19q non-codel (Figure 1H). Therefore, we can conclude that the risk score is an independent prognostic indicator of GBM patients.
Differential expression analysis between higher- and lower-risk groups
Herein, we performed gene set variation analysis (GSVA) analysis both in TCGA and CGCG dataset by the differential expressed genes to research enriched functional signaling pathway between high- and low-risk group. Furthermore, we performed GSEA analysis both in TCGA and CGCG dataset by the differential expressed genes to research enriched functional signaling pathway between high- and low-risk group. The results showed that genes highly expressed in high-risk group were mainly enriched in the biological processes that regulated adaptive immune response, chemokine signaling pathway and B cell activation. Corresponding pathways could also be observed in enrichment pathway analysis on CGCG data set (Figure 2A-2C).
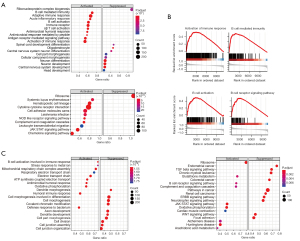
The correlation of tumor immune infiltration and ferroptosis related genes
In order to further explore the correlation between risk score and immune response, we first analyzed the differential expression of 1,040 immune-related genes (table available at https://cdn.amegroups.cn/static/public/tcr-21-2470-1.pdf) collected from the InnateDB database between the higher- and lower-risk groups, and found 308 of the 386 differentially expressed genes were significantly highly expressed in the higher-risk group (Figure 3A). As shown in the Figure 3B, the cytokine and chemokine C-C motif chemokine ligand 2 (CCL2), C-C motif chemokine receptor 4 (CCR4), C-X-C motif chemokine ligand 14 (CXCL14), C-X-C motif chemokine ligand 2 (CXCL2), C-X-C motif chemokine receptor 1 (CXCR1), C-X-C motif chemokine receptor 3 (CXCR3), interleukin (IL)-10, IL-6 and IL-7R and inflammatory response gene IKBKB, NFKB1, NFKB2, S100A8, S100A9, SPP1, TNFRSF1A, TNFRSF1B and TNFRSF9 were increased in the higher-risk group (Figure 3B). Next, we calculated the stromal scores and immune scores of immune cell subgroups through the Estimation of Stromal and Immune cells in MAlignant Tumour tissues using Expression data (ESTIMATE) and CIBERSORT algorithms. By ESTIMATE analysis, we found that high-risk group showed higher immune score compared with low-risk group in TCGA cohort, and the immune score was significantly correlated with the risk score. There was no significant difference of the stromal scores between the two groups, and there was no correlation with the risk score (Figure 3C,3D). We also calculated the infiltration ratio of 22 immune cells through CIBERSORT, and found that the scores of Treg, natural killer (NK) cells resting, mast cells activated and neutrophils were significantly up-regulation in high-risk group, while M2 Macrophage and mast cells resting were down-regulated (Figure 3E). In addition, there was a positive correlation between the B cells infiltration and the risk score, which was consistent with the results of the GSEA analysis (Figure 3F).
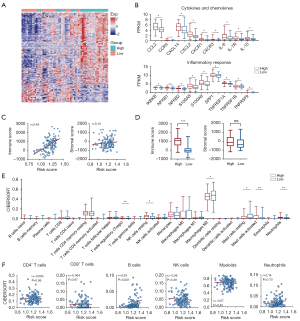
Methylation analysis of ferroptosis related genes
In order to clarify the regulation of the expression of these 15 risk genes at the genomic and epigenetic level, we performed CNV and methylation analysis on the TCGA data. Although mutation was the most common event in the tumor genome, the correlation between mutation and expression change was weak, and we did not analyze the mutation here. We have not observed which of these 15 genes had frequent copy number amplification or deletion (Figure 4A and Figure S1). However, there were 11 genes with methylation sites that significantly negatively correlated with gene expression. For example, in WIPI2 gene, the methylation values of four sites were negatively correlated with the expression, and the maximum correlation coefficient reached −0.45 (Figure 4B). These results indicate that most of the expression alterations of these might be explained by methylation.
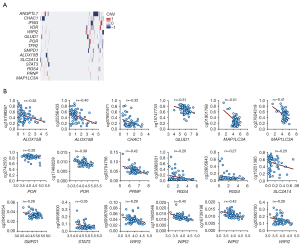
Verify the expression of ferroptosis related genes in paired GBM tumor and normal tissues
Since there was little expression data of tumor paired normal tissues regardless of TCGA or CGCG data, we collected 30 pairs of GBM and adjacent normal tissue samples to investigate the expression of risk score related 15 genes by RT-qPCR. ALOX15B, POR, STAT3 and VDR expresses higher in tumor tissues compared with normal tissues, while CHAC1, MAP1LC3A and RGS4 expressed lower (Figure 5A). In order to further analyze the expression of these four high expressed genes between tumor and adjacent normal tissues in a variety of tumors, the differential expression results from the TIMER database to illustrate that these four genes were significantly increased in several TCGA tumors tissues compared with normal tissues. ALOX15B and VDR was up-regulated in ten types of tumors tissues, POR was up-regulated in nine and STAT3 was up-regulated in six, and all these four genes expressed significantly higher in GBM tumors tissues compared with normal tissues (Figure 5B).
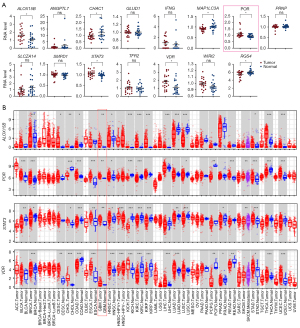
Discussion
In this study, by analyzing survival-related ferroptosis related genes in GBM, a risk score model was constructed to predict the prognosis of GBM patients, and good prediction results were achieved. We downloaded the mRNA expression profiles of 613 and 364 patients from the TCGA database and CGCG database, respectively, and obtained 15 survival-related ferroptosis related genes through univariate and multivariate Cox regression analysis. We verified the expression of these 15 genes in 30 pairs of tumors and adjacent normal tissues, and found that ALOX15B, POR, STAT3 and VDR were mainly enriched in tumor tissues. The survival time of patients in the high-risk group was significantly lower than that of the low-risk group. Multivariate Cox regression analysis showed that this risk score was an independent risk factor for the prognosis of GBM. The results of pathway enrichment analysis of genes that differ between high and low risks suggested that there were significant differences in the activity of immune-related pathways between the two groups. Next, we estimated the immune score through the ESTIMATE algorithm and the infiltration ratio of 22 immune cells through CIBERSORT algorithm, and further explored the differences in immune infiltrating cells between the two groups. In order to identify the factors that regulated the expression of risk genes in GBM, we analyzed the effects of CNV and methylation on gene expression in the TCGA data, and found that 11 of the genes were regulated by methylation sites.
In our study, 14 of the 15 survival-related genes used to construct the risk model are risk factors. These genes are also involved in the carcinogenesis and development of various tumors too and affect the prognosis of tumor patients. For example, STAT3 is extensively over-activated in cancer and non-cancer cells in the tumor microenvironment and plays a central role in regulating the anti-tumor immune response. STAT3 is involved in many biological processes, including cell proliferation, survival, differentiation, and angiogenesis, and is usually associated with poor clinical prognosis. Excessive activation of STAT3 in tumor-infiltrating lymphocytes leads to immunosuppression by suppressing innate and adaptive immune responses (21-24).
Studies have reported that innate immune cells such as NK cells were enriched in groups with high ferroptosis-related gene expression in GBM (25), which was consistent with our study. Furthermore, the researchers have demonstrated that in mouse model of GBM, neutrophil-mediated tumor cell necrosis was achieved through ferroptosis (26). Our results also showed a higher proportion of neutrophil infiltration in the patient with a higher ferroptosis risk score.
Gene methylation silencing is a common epigenetic event in tumors. Overall hypomethylation is commonly observed in malignant cells (27). Studies have shown that methylation of the MGMT promoter region in GBM has important clinical significance, which indicates the increased benefit of temozolomide chemotherapy (28). In this study, we first explored the regulatory factors of ferroptosis related genes expression in GBM at the genomic and epigenetic levels, and found that these genes are mainly affected by methylation rather than CNV. Abnormal methylation of these genes can also be observed in other tumors. In gastric cancer, Helicobacter pylori infection induced methylation silencing of the tumor suppressor gene MAP1LC3A through impairing autophagy, and facilitated gastric carcinogenesis (29). In undifferentiated embryonic carcinoma P19C6 cells, demethylation of the nucleotide region between −599 and −238 at the transcription initiation site can be observed, and the expression of PRNP was significantly increased (30). SLC2A14 had a difference in methylation status between all patients and controls (31). Although there is abnormal methylation of these genes in a variety of tumors, the regulatory relationship between specific methylation sites and expression is often tumor type specific. For example, the methylation level of cg16739198 site and the mRNA value of WIPI2 are significantly negatively correlated in GBM (r=−0.42), but they are not correlated in colon adenocarcinoma (r=−0.066), esophageal carcinoma (r=−0.095), lung adenocarcinoma (r=−0.010), or even brain lower grade glioma (r=−0.064).
It is undeniable that our research still has some flaws. It would be better if we can use experiments, such as pyrosequencing, to verify the regulation between methylation and risk genes in an independent tumor and normal sample paired verification cohort.
Conclusions
We analyzed the correlation between the expression of ferroptosis related genes in GBM and patient survival, obtained 15 survival-related events and constructed a prognostic risk prediction model. We found that the expression of these risk genes in GBM is mainly regulated by methylation rather than CNV.
Acknowledgments
We thank all the participants and their families.
Funding: This research project was supported by grants from the National Natural Science Foundation of China (No. 81960330).
Footnote
Reporting Checklist: The authors have completed the STREGA reporting checklist. Available at https://tcr.amegroups.com/article/view/10.21037/tcr-21-2470/rc
Data Sharing Statement: Available at https://tcr.amegroups.com/article/view/10.21037/tcr-21-2470/dss
Conflicts of Interest: All authors have completed the ICMJE uniform disclosure form (available at https://tcr.amegroups.com/article/view/10.21037/tcr-21-2470/coif). The authors have no conflicts of interest to declare.
Ethical Statement: The authors are accountable for all aspects of the work in ensuring that questions related to the accuracy or integrity of any part of the work are appropriately investigated and resolved. The study was conducted in accordance with the Declaration of Helsinki (as revised in 2013).
Open Access Statement: This is an Open Access article distributed in accordance with the Creative Commons Attribution-NonCommercial-NoDerivs 4.0 International License (CC BY-NC-ND 4.0), which permits the non-commercial replication and distribution of the article with the strict proviso that no changes or edits are made and the original work is properly cited (including links to both the formal publication through the relevant DOI and the license). See: https://creativecommons.org/licenses/by-nc-nd/4.0/.
References
- Schwartzbaum JA, Fisher JL, Aldape KD, et al. Epidemiology and molecular pathology of glioma. Nat Clin Pract Neurol 2006;2:494-503; quiz 1 p following 516.
- Louis DN, Perry A, Wesseling P, et al. The 2021 WHO Classification of Tumors of the Central Nervous System: a summary. Neuro Oncol 2021;23:1231-51. [Crossref] [PubMed]
- Parsons DW, Jones S, Zhang X, et al. An integrated genomic analysis of human glioblastoma multiforme. Science 2008;321:1807-12. [Crossref] [PubMed]
- Adamson C, Kanu OO, Mehta AI, et al. Glioblastoma multiforme: a review of where we have been and where we are going. Expert Opin Investig Drugs 2009;18:1061-83. [Crossref] [PubMed]
- Brandes AA, Ermani M, Basso U, et al. Temozolomide as a second-line systemic regimen in recurrent high-grade glioma: a phase II study. Ann Oncol 2001;12:255-7. [Crossref] [PubMed]
- Krex D, Klink B, Hartmann C, et al. Long-term survival with glioblastoma multiforme. Brain 2007;130:2596-606. [Crossref] [PubMed]
- Omuro A, DeAngelis LM. Glioblastoma and other malignant gliomas: a clinical review. JAMA 2013;310:1842-50. [Crossref] [PubMed]
- Brennan CW, Verhaak RG, McKenna A, et al. The somatic genomic landscape of glioblastoma. Cell 2013;155:462-77. [Crossref] [PubMed]
- Noushmehr H, Weisenberger DJ, Diefes K, et al. Identification of a CpG island methylator phenotype that defines a distinct subgroup of glioma. Cancer Cell 2010;17:510-22. [Crossref] [PubMed]
- Luo P, Yan H, Du J, et al. PLK1 (polo like kinase 1)-dependent autophagy facilitates gefitinib-induced hepatotoxicity by degrading COX6A1 (cytochrome c oxidase subunit 6A1). Autophagy 2021;17:3221-37. [Crossref] [PubMed]
- Tang D, Chen X, Kang R, et al. Ferroptosis: molecular mechanisms and health implications. Cell Res 2021;31:107-25. [Crossref] [PubMed]
- Craig JM, Bickmore WA. The distribution of CpG islands in mammalian chromosomes. Nat Genet 1994;7:376-82. [Crossref] [PubMed]
- Klutstein M, Nejman D, Greenfield R, et al. DNA Methylation in Cancer and Aging. Cancer Res 2016;76:3446-50. [Crossref] [PubMed]
- Pan Y, Liu G, Zhou F, et al. DNA methylation profiles in cancer diagnosis and therapeutics. Clin Exp Med 2018;18:1-14. [Crossref] [PubMed]
- Wenger A, Ferreyra Vega S, Kling T, et al. Intratumor DNA methylation heterogeneity in glioblastoma: implications for DNA methylation-based classification. Neuro Oncol 2019;21:616-27. [Crossref] [PubMed]
- Wang W, Zhang L, Wang Z, et al. A three-gene signature for prognosis in patients with MGMT promoter-methylated glioblastoma. Oncotarget 2016;7:69991-9. [Crossref] [PubMed]
- Wen WS, Hu SL, Ai Z, et al. Methylated of genes behaving as potential biomarkers in evaluating malignant degree of glioblastoma. J Cell Physiol 2017;232:3622-30. [Crossref] [PubMed]
- Jiang X, Stockwell BR, Conrad M. Ferroptosis: mechanisms, biology and role in disease. Nat Rev Mol Cell Biol 2021;22:266-82. [Crossref] [PubMed]
- Dixon SJ, Lemberg KM, Lamprecht MR, et al. Ferroptosis: an iron-dependent form of nonapoptotic cell death. Cell 2012;149:1060-72. [Crossref] [PubMed]
- Chen X, Kang R, Kroemer G, et al. Broadening horizons: the role of ferroptosis in cancer. Nat Rev Clin Oncol 2021;18:280-96. [Crossref] [PubMed]
- Wang T, Niu G, Kortylewski M, et al. Regulation of the innate and adaptive immune responses by Stat-3 signaling in tumor cells. Nat Med 2004;10:48-54. [Crossref] [PubMed]
- Fujiwara Y, Takeya M, Komohara Y. A novel strategy for inducing the antitumor effects of triterpenoid compounds: blocking the protumoral functions of tumor-associated macrophages via STAT3 inhibition. Biomed Res Int 2014;2014:348539. [Crossref] [PubMed]
- Hanlon MM, Rakovich T, Cunningham CC, et al. STAT3 Mediates the Differential Effects of Oncostatin M and TNFα on RA Synovial Fibroblast and Endothelial Cell Function. Front Immunol 2019;10:2056. [Crossref] [PubMed]
- Johnson DE, O'Keefe RA, Grandis JR. Targeting the IL-6/JAK/STAT3 signalling axis in cancer. Nat Rev Clin Oncol 2018;15:234-48. [Crossref] [PubMed]
- Wan RJ, Peng W, Xia QX, et al. Ferroptosis-related gene signature predicts prognosis and immunotherapy in glioma. CNS Neurosci Ther 2021;27:973-86. [Crossref] [PubMed]
- Yee PP, Wei Y, Kim SY, et al. Neutrophil-induced ferroptosis promotes tumor necrosis in glioblastoma progression. Nat Commun 2020;11:5424. [Crossref] [PubMed]
- Dawson MA, Kouzarides T. Cancer epigenetics: from mechanism to therapy. Cell 2012;150:12-27. [Crossref] [PubMed]
- Hegi ME, Diserens AC, Gorlia T, et al. MGMT gene silencing and benefit from temozolomide in glioblastoma. N Engl J Med 2005;352:997-1003. [Crossref] [PubMed]
- Muhammad JS, Nanjo S, Ando T, et al. Autophagy impairment by Helicobacter pylori-induced methylation silencing of MAP1LC3Av1 promotes gastric carcinogenesis. Int J Cancer 2017;140:2272-83. [Crossref] [PubMed]
- Dalai W, Matsuo E, Takeyama N, et al. Increased expression of prion protein gene is accompanied by demethylation of CpG sites in a mouse embryonal carcinoma cell line, P19C6. J Vet Med Sci 2017;79:644-8. [Crossref] [PubMed]
- Taylor KH, Pena-Hernandez KE, Davis JW, et al. Large-scale CpG methylation analysis identifies novel candidate genes and reveals methylation hotspots in acute lymphoblastic leukemia. Cancer Res 2007;67:2617-25. [Crossref] [PubMed]