GABRP is a potential prognostic biomarker and correlated with immune infiltration and tumor microenvironment in pancreatic cancer
Introduction
Pancreatic cancer, primarily pancreatic ductal adenocarcinoma (PDAC), is one of the most commonly diagnosed malignancies which ranks 14th among all cancers and the 7th leading cause of cancer-related death worldwide. Global cancer statistics reveal that there were 495,773 new cases and 466,003 deaths of pancreatic cancer in 2020 (1). Pancreatic cancer is a fatal malignancy with a 5-year survival of about 5% when diagnosed and a median survival of <11 months (2). Surgical resection is the only potential curative treatment for pancreatic cancer at present and adjuvant chemotherapies have improved patient outcomes, but at the cost of a higher incidence of adverse effect(3,4). However, just a minority of pancreatic cancer patients are eligible for surgical therapy since the disease lacks early symptoms until developed into advanced stages (5). In recent years, targeted therapy and immunotherapy have brought therapeutic benefit in many solid tumors. Several targeted therapies such as VEGF inhibitors and KRAS inhibitors have been evaluated in pancreatic cancer, but received minimal effect. The same can be seen in immunotherapy, which shows promising efficacy in several solid tumor especially melanoma including PD-1 inhibitors and CTLA-4 inhibitors while the response rate is unsatisfactory in pancreatic cancer (6-8). Thus, it remains imperative to identify potential prognostic biomarkers and therapeutic targets to facilitate the clinical treatment and discovery of clinical candidate compounds and to exploit possible strategies of immunotherapy for pancreatic cancer.
GABRP is the π subunit of the gamma-aminobutyric acid (GABA) A receptor, which is a hetero-pentameric and ligand-gated chloride channel composed of five subunits. These subunits are encoded by 19 genes in 8 subclasses based on sequence homology including α1–6, β1–3, γ1–3, δ, ε, θ, π and ρ1–3. GABAA receptors mainly distributed in the central nervous system which frequently consists of at least two α, two β subunits and one γ subunit. The γ subunit can be replaced by a δ, ε, π subunit, but the function of the π subunit is still unclear(9-11). Unlike other GABAA receptors, GABRP is detectable in multiple non-neuronal normal human tissues including uterus, prostate, ovaries, small intestine and lung. It is reported that GABRP may play an important role in endometrial receptivity and control receptor sensitivity to endogenous steroids and pregnenolone (12-14).
Although the function of GABRP in normal tissues has not been fully elucidated, several studies have revealed dysregulation of GABRP expression in solid tumors including breast, ovarian, cervical and pancreatic cancer(15-18). Jiang et al. proposed that GABRP promoted pancreatic tumorigenesis via KCNN4-mediated Ca2+ signaling to regulate chemokine and macrophage recruitment, which indicated the significance and potential immunomodulatory role of GABRP in pancreatic cancer(18). However, there has been no study to comprehensively explore the expression, prognostic value and regulatory role of GABRP in pancreatic cancer and whether GABRP was associated with immune infiltration or could affect the tumor microenvironment. Here, we integrated multiple ‘omics’ data about pancreatic cancer from multiple authoritative databases and used bioinformatics analysis to comprehensively and systematically analyze the expression profile, clinicopathological characteristics and potential molecular mechanism of GABRP in pancreatic cancer. For the first time, we elucidated the potential mechanism of GABRP dysregulation and showed how GABRP might participate in regulating pancreatic tumor infiltrating cells and tumor microenvironment, which further confirmed the important role of GABRP as a prognostic biomarker and therapeutic target in pancreatic cancer as well as a possible regulator of tumor microenvironment affecting the efficacy of immunotherapy (Figure 1A). We present the following article in accordance with the REMARK reporting checklist (available at https://tcr.amegroups.com/article/view/10.21037/tcr-21-2021/rc).
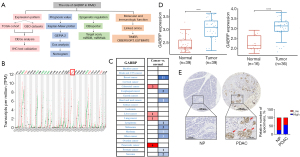
Methods
Data collection
The data on GABRP mRNA expression in pancreatic adenocarcinoma (PAAD) tissues and normal tissues and corresponding clinical data were retrieved from the TCGA database (https://portal.gdc.cancer.gov/). A total of 178 tumor tissues and 4 normal tissues obtained from The Cancer Genome Atlas (TCGA) database were included in the data analysis. The GABRP mRNA expression profiles of two pancreatic cancer datasets from the gene expression omnibus (GEO) database (https://www.ncbi.nlm.nih.gov/geo/) were also included in the data analysis, which consisted of microarrays with accession number GSE15471 (GEO platform GPL570) which contained 39 tumor samples and 39 normal samples and the GSE16515 (GEO platform GPL570) which contained 36 tumor samples and 16 normal samples(19,20). The GABRP mRNA expression profiles of three pancreatitis datasets from the GEO database were also included in the data analysis including microarrays with the accession number, GSE15471 (GEO platform GPL6246), which contained six pancreatitis samples and five normal samples, the GSE65146 (GEO platform GPL6246), which contained 64 pancreatitis samples and 9 normal samples, and the GSE41418 (GEO platform GPL1261) which contained 6 pancreatitis samples and 6 normal samples.
Differential expression analysis
Oncomine database analysis
The Oncomine database (http://www.oncomine.org) incorporated 86,733 samples and 715 gene expression datasets into a single comprehensive database allowing various analytical methods(21). We used the Oncomine database to evaluate the mRNA expression level of the GABRP gene among various cancer types. The threshold significance was determined by a P value of 0.001, fold-change of 2, gene rank of ‘all’ and data type of ‘mRNA’.
GEPIA2 database analysis
The gene expression profiling interactive analysis 2 (GEPIA2) database (http://GEPIA2.cancer-pku.cn/index.html) was utilized to evaluate the expression of GABRP in different cancer types. Featuring 198,619 isoforms and 84 cancer subtypes, GEPIA2 (22) is an updated and enhanced version of GEPIA, a web resource for gene expression analysis based on tumor and normal samples from the TCGA and the GTEx databases. The gene expression profiles of GABRP among all tumor samples paired normal tissues were shown in a dot-plot.
Analysis of GEO microarray datasets
Two datasets, GSE15471 and GSE16515, were used to analyze the expression of GABRP in pancreatic cancer between normal tissues and tumor tissues. The data was processed by R software v4.0.3 and the plot was constructed using the R software package, ggplot2.
Survival analysis and construction of nomogram
The Kaplan-Meier plotter (www.kmplot.com) is a web-based tool for exploring the impact of 54,000 genes on patient survival covering 21 cancer types(23). The database is based on gene expression data, relapse-free and overall survival information downloaded from TCGA, GEO and the European Genome-Phenome Archive (EGA) database. The Kaplan-Meier plotter was used to perform prognostic analysis of GABRP and hsa-miR-3655 expression levels in PAAD, and the association between GABRP expression and survival outcome based on different immune cell contents with auto-selected cutoff values. GEPIA2 was used to perform prognostic analysis of GABRP expression levels in PAAD based on GABRP expression with a specific cutoff value using the R software package ‘survival’. The log rank P value, the hazard ratio (HR), and survival curves were calculated and annotated for each plot.
Univariate and multivariate Cox regression analysis was performed using the prognosis model from the Assistant for Clinical Bioinformatics (https://www.aclbi.com/static/index.html#/prognosis) to identify the appropriate terms to build the nomogram. The Forest was used to show the P value, HR and 95% CI of each variable through ‘forestplot’ in the R package. The variable that shows significance in multivariate Cox proportional analysis shows that it should be an independent indicator of prognosis. Based on the results of multivariate Cox proportional hazards analysis, a nomogram was developed to predict the X-year overall recurrence through the ‘rms’ R package.
Hypermethylation of GABRP promoter and prediction of GABRP regulatory miRNAs
The cBioPortal for cancer genomics was used to explore the relationship between GABRP DNA methylation and GABRP expression in TCGA-PAAD(24). TargetScan, miRDB and miRwalk were used to predict the regulatory miRNAs of GABRP(25-27). The common miRNAs predicted by the above three databases were included in the prediction cohort. The common miRNAs significantly downregulated in PAAD in the three cohorts were then regarded as potential regulatory miRNAs of PARPBP in HCC. UALCAN (28) (http://ualcan.path.uab.edu/index.html) is an interactive web-portal web resource to perform comprehensive analysis of TCGA gene expression data. We used UALCAN to assess the methylation levels of GABRP and the expression of miR-3655 in PAAD and its effect on prognosis.
LinkedOmics database analysis
LinkedOmics is a publicly available portal that includes multi-omics data from all 32 TCGA cancer types and provides a unique platform for molecular biologists and clinicians to access, analyze and compare multi-omics cancer data within and across tumor types(29). We searched for GABRP co-expressed genes in the PAAD dataset (n=178) based on the Pearson correlation coefficient. In the LinkInterpreter module, gene set enrichment analysis (GSEA) module was used to assess the biological processes (BPs), cell components (CCs), molecular function (MFs), and KEGG pathways based on co-expressed genes. The GSEA program was run with 500 simulations. If the P value and false discovery rate (FDR) were both less than 0.05, the gene set was considered significantly enriched.
TIMER database analysis
The tumor immune estimation resource (TIMER, https://cistrome.shinyapps.io/timer/) is a web server designed for comprehensive analysis of tumor-infiltrating immune cells in 32 cancer types from TCGA(30). The TIMER database was used to analyze the relationship between the level of GABRP expression and six tumor-infiltrating immune cells (TIICs) and tumor purity in PAAD. The six TIICs include B cells, CD4+ T cells, CD8+ T cells, macrophages, neutrophils, and dendritic cells. The SCNA (somatic copy number alteration) module was used to compare tumor infiltration levels in PAAD with different somatic copy number alterations for GABRP. Then the correlation between GABRP and several immune cell markers were assessed by using the correlation module(31).
CIBERSORT database analysis
Cell type identification by estimating relative subsets of RNA transcripts (CIBERSORT, https://cibersort.stanford.edu/) is a deconvolution algorithm developed to characterize 22 types of immune cells composition in complex tissues(32). By integrating TCGA-PAAD datasets, GSE15471 and GSE16515 datasets, we analyzed the integrated mRNA expression matrix using the CIBERSORT R script acquired from the CIBERSORT website. After filtering samples with P≥0.05, 59 normal samples and 205 tumor samples were included in the study.
Estimation of tumor microenvironment components in PAAD
The ESTIMATE algorithm(33) uses gene expression signatures to estimate the fraction of stromal cells and immune cells and calculates the immune score and stromal score of PAAD tumor samples. The algorithm was implemented using the ‘estimate’ package by R software. Two subtypes of PAAD were classified based on GABRP expression (high vs. low) and compared using the Kruskal-Wallis test.
Immunohistochemistry (IHC) analysis
The PAAD chip (Cat. No. HPanA060CS03-M-109) was purchased from SuperChip in Shanghai, China. The chip contained two normal pancreatic tissues and 29 PAAD tumor and adjacent tumor tissues, which included phases I (3 cases), II (23 cases) and III (3 cases) PAAD tumor tissues. After routinely dewaxing and hydration, antigen retrieval was performed in 10 mM saline sodium citrate at sub-boiling temperature for 90 s. Then, the activity of endogenous peroxidases in specimens was blocked with 3% hydrogen peroxide. After permeabilization with 0.1% Triton X-100 and blocking with 5% bovine serum albumin (BSA), the chip was incubated with primary antibody against rabbit anti-GABRP antibody (1:200 dilution, PA5-46830, Invitrogen) at 4 ℃ overnight. The chip was balanced at room temperature for 30 min, washed with PBS and incubated with horseradish peroxidase-conjugated goat anti-mouse IgG (DAKO, Wuhan, China) for 60 min at room temperature. GABRP expression was visualized by DAB (DAKO, Wuhan, China) staining. The chip was scanned by Pannoramic Scan (3DHISTECH Ltd., Hungary).
Statistical analysis
The Wilcoxon rank sum test was employed to explore the different expression pattern between PAAD tumor samples and normal samples. The PAAD cohorts were divided into two groups using the optimal threshold evaluated by X-tile 3.6.1 on the basis of GABRP expression levels(34). The relationship between GABRP expression and clinicopathological parameters was analyzed using Chi-squared test. The correlation between GABRP and TIICs was calculated by Spearman correlation. The Cox proportional hazards model was used to identify the independent indicators related to OS, and the hazard ratios (HRs) with 95% confidence intervals (CI) were also calculated. Nomograms were constructed based on the results of multivariate Cox analysis on the basis of OS to predict the X-year overall recurrence using the ‘rms’ R package. All statistical analyses were performed using R software (Version 4.0.2), and the threshold of statistical significance was set at P<0.05.
Ethical statement
The study was conducted in accordance with the Declaration of Helsinki (as revised in 2013). Institutional ethical approval and informed consent were waived.
Results
GABRP expression was upregulated in pancreatic cancer
The mRNA expression of GABRP in different cancers and normal tissues was evaluated by analyzing the GEPIA2 and Oncomine databases (Figure 1). In GEPIA2, the expression of GABRP was obviously elevated in PAAD compared to normal tissues (Figure 1B). In the Oncomine database, the expression of GABRP was significantly higher in PAAD than normal tissues (Figure 1C). Next, the expression of GABRP in pancreatic cancer was further validated by analyzing two GEO cohorts (GSE15471 and GSE16515) (19,20,35,36). In GEO cohort, GSE15471 and GSE16515 (Figure 1D), GABRP was significantly overexpressed in tumor samples compared to normal pancreas tissues. To further check the expression pattern of GABRP protein in PDAC, immunohistochemical (IHC) analysis was carried out in tissue microarrays of human PDAC. It was shown in Figure 1E that GABRP expression is higher in PDAC than in normal pancreas, while commonly detected in the PDAC cells (Figure 1E). However, there was no significant change in the expression of GABRP in pancreatitis (Table S1). In summary, GABRP was highly expressed in pancreatic cancer.
Association between GABRP expression, clinicopathological characteristics and survival outcomes
The clinicopathological data of 178 PAAD patients were obtained from the TCGA database and separated into low or high GABRP expression based on the optimal threshold of OS. As shown in Table 1, there was no significance in age, gender, TNM stage and living status while grade was significantly associated with the expression level of GABRP (P<0.05). The correlation between GABRP expression and survival outcome of PAAD was next investigated using the Kaplan-Meier plotter. Results from Kaplan-Meier (KM) survival analysis showed that elevated expression of GABRP was linked to poor prognosis in PAAD. Patients with higher GABRP expression had shorter overall survival than those with lower GABRP expression (Figure 2A, HR =1.91, P=0.0024). Similarly, statistically significant lower disease-free survival (DFS) was observed in patients with higher GABRP expression (Figure 2B, HR =5, P=0.00073). GEPIA2 was used to confirm the effect of GABRP expression on pancreatic cancer prognosis (Figure 2C,2D). Similar results from GEPIA2 disclosed that patients with high GABRP expression had worse prognosis in TCGA PAAD cohorts.
Table 1
Variables | Total (N=178) | GABRP expression | P value | |
---|---|---|---|---|
High (N=111) | Low (N=67) | |||
Age (years), n (%) | 1 | |||
<65 | 82 (46.1) | 51 (45.9) | 31 (46.3) | |
≥65 | 96 (53.9) | 60 (54.1) | 36 (53.7) | |
Gender, n (%) | 0.0809 | |||
Male | 98 (55.1) | 55 (49.5) | 43 (64.2) | |
Female | 80 (44.9) | 56 (50.5) | 24 (35.8) | |
Grade, n (%) | 0.01938* | |||
G1 | 31 (17.4) | 14 (12.6) | 17 (25.4) | |
G2 | 95 (53.4) | 61 (55.0) | 34 (50.7) | |
G3 | 48 (27.0) | 35 (31.5) | 13 (19.4) | |
G4 | 2 (1.1) | 0 (0) | 2 (3.0) | |
Unknown | 2 (1.1) | 1 (0.9) | 1 (1.5) | |
TNM stage, n (%) | 0.4281 | |||
I | 21 (11.8) | 10 (9.0) | 11 (16.4) | |
II | 147 (82.6) | 96 (86.5) | 51 (76.1) | |
III | 3 (1.7) | 2 (1.8) | 1 (1.5) | |
IV | 4 (2.2) | 3 (2.7) | 1 (1.5) | |
Unknown | 3 (1.7) | 0 (0) | 3 (4.5) | |
Living status, n (%) | 1 | |||
Alive | 71 (39.9) | 36 (32.4) | 35 (52.2) | |
Dead | 107 (60.1) | 75 (67.6) | 32 (47.8) |
*, P<0.05. PAAD, pancreatic adenocarcinoma; TCGA, The Cancer Genome Atlas.
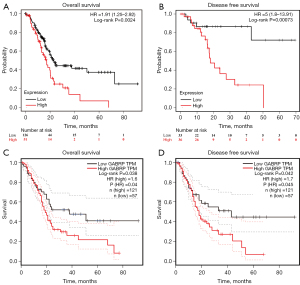
Expression of GABRP is an independent prognostic indicator of PAAD
The prognostic value of GABRP in PAAD was first assessed by using the Kaplan-Meier plotter and GEPIA2. In the next step, Cox regression analysis was performed to explore independent risk factors of OS by using data from the TCGA PAAD cohort. In the univariate model, GABRP expression, age and grade were significantly related to OS in PAAD (Figure 3A, all P<0.05). Multivariate analysis further confirmed that GABRP expression was an independent risk factor of OS in PAAD [Figure 3B, HR =1.09879 (1.00705, 1.19888), P=0.03419]. Finally, a prognostic nomogram for PAAD was constructed to further predict the prognostic value of GABRP in PAAD based on mRNA expression and GABRP expression was identified as an independent indicator of OS. As shown in Figure 3C, a nomogram was generated to assess the performance of the risk score in combination with the GABRP expression of PAAD patients in predicting the OS. The concordance index (c-index) value was 0.541 for PAAD, which measures the level of agreement between prediction by nomogram and the observed survival status. The calibration curves for 1-, 2-, 3- and 5-year survival were also plotted for PAAD (Figure 3D).
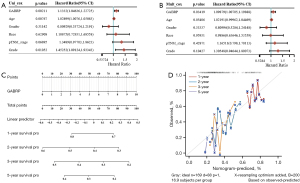
Promoter hypomethylation and hsa-miR-3655 downregulation may contribute to GABRP upregulation in PAAD
The potential mechanism of GABRP overexpression in PAAD was explored from the perspective of epigenetic regulation. Increasingly, studies have revealed abnormal DNA methylation in cancers and many non-neoplasm diseases. Promoters with cancer-associated hypomethylation are always linked to the activation of genes that were silent in normal tissues (37). The analysis via cBioportal revealed a significant negative correlation between GABRP methylation and GABRP expression (Figure 4A, Spearman’s r=−0.77, P=6.52e-37, Pearson’s r=−0.66, P=1.40e-23). In addition, UALCAN was used to verify the promoter methylation of GABRP in PAAD, and the level of GABRP promoter methylation in PAAD was significantly lower than in normal tissues (Figure 4B). Patients with PAAD were stratified based on the stage, grade, nodal metastasis status and TP53 mutant status. The results showed that lower levels of GABRP promoter methylation were associated with higher-grade tumors, advanced individual cancer stages, lymph node metastasis and TP53 mutant status (Figure S1A-S1D).
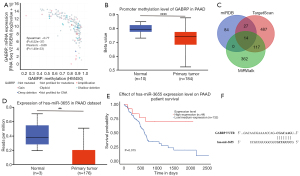
We next explored the potential regulatory miRNAs of GABRP in PAAD using miRDB, TargetScan and MirWalk. Seventeen common miRNAs were selected as candidates containing GABRP-binding sequences (Figure 4; Table S2). After evaluation with UALCAN, hsa-miR-3655 was identified as a potential regulatory miRNA of GABRP. Hsa-miR-3655 was significantly downregulated in PAAD compared with normal tissues in the TCGA-PAAD dataset (Figure 4D, P=0.0067). KM analysis revealed that low expression of hsa-miR-3655 was associated with worse survival (Figure 4E, P=0.015).
Analysis of co-expressed genes of GABRP in PAAD
LinkedOmics was used to explore the co-expressed genes of GABRP in PAAD which should shed light on the biological meaning of GABRP in the process of tumorigenesis and development. A total of 6,647 genes had co-occurrences in PAAD (false discovery rate, FDR <0.01). A total of 4,100 genes (red dots) were significantly positively correlated with GABRP expression and 2,547 genes (green dots) were significantly negatively correlated with GABRP expression in PAAD (Figure S2A). The top 50 ranked genes significantly correlated with GABRP expression were visualized in a heatmap (Figure S2B,S2C). GABRP expression showed a strong negative association with expression of PEMT (r=−0.5772, P=3.34E-17), ATP6AP1 (r=−0.5402, P=7.08E-15), HDAC5 (r=−0.5217, P=8.17E-14), etc. (Figure S2B). GABRP expression also showed a strong positive association with expression of GTB4 (r=0.6364, P=1.33E-21), MMP7 (r=0.6193, P=3.14E-20), LOC284578 (r=0.6085, P=2.06E-19), etc. (Figure S2C). GSEA of co-expressed genes of GABRP was performed to explore the underlying biological functions of GABRP overexpression in PAAD. Gene ontology (GO) term annotation and Kyoto Encyclopedia of Genes and Genomes (KEGG) pathway analysis by GSEA are presented in Figure 5. In biological processes (BPs), GABRP co-expressed genes were correlated with regulation of leukocyte activation, immune response-regulating signaling pathway, adaptive immune response, etc. (Figure 5A). In cellular components (CCs), GABRP co-expressed genes were related to immunological synapse, cell substrate junction, MHC protein complex, etc. (Figure 5B). In molecular functions (MFs), GABRP co-expressed genes were associated with collagen binding, cytokine receptor activity, cytokine binding, etc. (Figure 5C). KEGG pathway analysis showed enrichment in the NOD-like receptor signaling pathway, NF-kappa B signaling pathway, cytokine-cytokine receptor interaction, etc. (Figure 5D). The GO analysis and KEGG analysis results revealed that GABRP co-expressed genes had a widespread impact on immune responses.
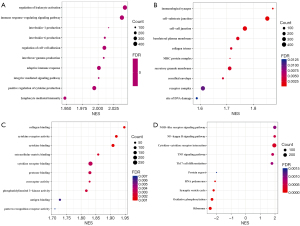
Differential immune infiltration landscape of pancreatic cancer and normal tissues
We systematically depicted the pattern of immune cells in pancreatic cancer by extracting and processing the signature gene expression profiles from TCGA-PAAD and GEO cohorts (GSE15471 and GSE16515) performed by CIBERSORT. After filtering the samples for P≥0.05, 59 normal samples and 205 tumor samples were included in the analysis. The differential immune infiltration landscape of pancreatic cancer and normal tissues was displayed in Figure 6. There was a different pattern of infiltrating immune cells between normal tissues and pancreatic cancer tissues (Figure 6A). Moreover, different correlation patterns among 22 immune cells were found in pancreatic cancer and normal tissues (Figure 6B). In the study cohort, the fractions of 22 immune cells were correlated with each other in different degrees suggesting a complex tumor infiltrating environment in pancreatic cancer. The violin plot of 22 immune cells showed that the proportion of different infiltrating immune cells significantly varied between tumor and normal tissues except for macrophages M1 and M2 (Figure 6C). Compared to normal tissues, the fractions of naïve B cells, CD4+ T cells and macrophage M0 were relatively higher in tumor tissues while CD8+ T cells and NK cells were significantly lower in tumor tissues.
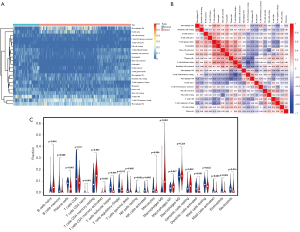
Correlation between GABRP expression and immune infiltration in pancreatic cancer
Based on the results of GSEA analysis, we first assessed the relationship between GABRP and six types of infiltrating immune cells including B cells, CD8+T cells, CD4+T cells, macrophages, neutrophils and dendritic cells by accessing the TIMER database (Figure 7A). The results suggested that the expression level of GABRP was positively correlated with the infiltration degree of B cells (r=0.22, P=3.86E-03), CD8+ T cells (r=0.298, P=7.73E-05), macrophages (r=0.194, P=1.10E-02), neutrophils (r=0.277, P=2.41E-04) and dendritic cells (r=0.264, P=4.94E-04) in PAAD. In addition, a higher GABRP expression level was associated with lower tumor purity (r=−0.295, P=8.63E-05), which may contribute to worse clinical features and outcomes(38). Genes with somatic copy number alterations (sCNAs) could influence tumorigenesis, progression and immunotherapy response(39). GABRP copy number alterations were related to immune cell infiltration by B cells, CD4+ T cells, macrophages, and neutrophils in PAAD (Figure 7B).
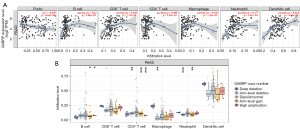
Correlation between GABRP expression and immune marker expression in pancreatic cancer
In order to clarify the reason why GABRP was associated with immune infiltration, we further explored the correlations between GABRP expression and a series of immune markers related to various types of immune cells using the TIMER database. Several immune cells were characterized by immune markers including B-cells, CD8+ T cells, neutrophils, macrophages, dendritic cells, Th1 cells, Th2 cells and Treg cells, and their correlation with GABRP expression was determined using the TIMER database (40,41). As shown in Table 2, GABRP expression had a significantly positive correlation with immune makers of B cells, CD8+ T cells, neutrophils, dendritic cells, Th1 cells, Th2 cells and Treg cells, without regard to age or tumor purity. The macrophage markers were significantly correlated with GABRP expression, except for CD84 and CD163 in the condition adjusted by tumor purity optionally. The above analysis laid the foundation for the important role of GABRP in immune infiltration.
Table 2
Cell type | Gene markers | PAAD | |||||||
---|---|---|---|---|---|---|---|---|---|
None | Purity | Age | |||||||
Cor | P | Cor | P | Cor | P | ||||
B cells | CD19 | 0.263 | 3.73E-04 | 0.214 | 4.94E-03 | 0.264 | 3.76E-04 | ||
CD79A | 0.26 | 4.60E-04 | 0.201 | 8.24E-03 | 0.262 | 4.12E-04 | |||
CD8+ T cells | CD8A | 0.325 | 1.02E-05 | 0.279 | 2.15E-04 | 0.323 | 1.10E-05 | ||
CD8B | 0.332 | 6.45E-06 | 0.282 | 1.87E-04 | 0.331 | 6.44E-06 | |||
Neutrophils | CEACAM3 | 0.372 | 3.00E-07 | 0.311 | 3.50E-05 | 0.37 | 3.72E-07 | ||
CSF3R | 0.295 | 6.59E-05 | 0.202 | 7.99E-03 | 0.293 | 7.26E-05 | |||
FCGR3B | 0.24 | 1.26E-03 | 0.158 | 3.85E-02 | 0.238 | 1.37E-03 | |||
CCR7 | 0.236 | 1.54E-03 | 0.19 | 1.28E-02 | 0.225 | 2.51E-03 | |||
Macrophages | CD68 | 0.268 | 3.01E-04 | 0.182 | 1.72E-04 | 0.26 | 4.58E-04 | ||
CD84 | 0.2 | 7.45E-03 | 0.108 | 1.58E-01 | 0.2 | 7.59E-03 | |||
CD163 | 0.233 | 1.73E-03 | 0,13 | 8.91E-02 | 0.229 | 2.03E-03 | |||
MS4A4A | 0.256 | 5.60E-04 | 0.153 | 4.53E-02 | 0.225 | 5.87E-04 | |||
Dendritic cells | CD1C | 0.271 | 2.47E-04 | 0.221 | 3.60E-03 | 0.258 | 4.95E-04 | ||
ITGAX | 0.297 | 5.89E-05 | 0.222 | 3.51E-03 | 0.291 | 7.96E-05 | |||
NRP1 | 0.265 | 3.62E-04 | 0.201 | 8.36E-03 | 0.248 | 8.60E-04 | |||
Th1 cells | STAT1 | 0.393 | 6.66E-08 | 0.336 | 6.99E-06 | 0.391 | 6.73E-08 | ||
TBX21 | 0.226 | 2.39E-03 | 0.184 | 1.60E-02 | 0.226 | 2.38E-03 | |||
Th2 cells | GATA3 | 0.27 | 2.72E-04 | 0.248 | 1.09E-03 | 0.263 | 3.97E-04 | ||
STAT6 | 0.42 | 6.59E-09 | 0.413 | 2.02E-08 | 0.417 | 6.81E-09 | |||
Treg | CCR8 | 0.3 | 4.56E-05 | 0.24 | 1.58E-03 | 0.291 | 8.20E-05 | ||
FOXP3 | 0.296 | 6.36E-05 | 0.229 | 2.64E-03 | 0.287 | 1.03E-04 |
PAAD, pancreatic adenocarcinoma.
Prognostic analysis of GABRP based on different immune cell contents in pancreatic cancer
Given the effect of GABRP on prognosis and immune cell infiltration in PAAD, we determined whether GABRP expression could affect the prognosis of pancreatic cancer due to immune cell infiltration. We performed a prognostic analysis of GABRP based on different immune cell contents in PAAD using the Kaplan-Meier plotter. The results indicated that higher GABRP expression related to worse prognosis in terms of enriched B cells, decreased CD4+ T cells, enriched CD8+ T cells, decreased macrophages, enriched NK cells, and decreased Treg cells compared to decreased B cells, enriched CD4+ T cells, decreased CD8+ T cells, enriched macrophages, decreased NK cells, and enriched Treg cells (Figure 8). In addition, the different profiles of CD4+ T cells and macrophages could considerably alter the effect of GABRP expression on survival outcome of PAAD (Figure 8C,8D,8G,8H). The above results suggested that higher expression of GABRP may affect survival of pancreatic cancer partly due to immune infiltration.
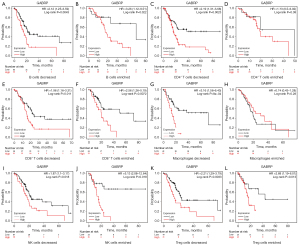
Evaluation of tumor microenvironment in pancreatic cancer based on GABRP expression
As one of the most lethal solid tumors worldwide, pancreatic cancer is characterized by an immunosuppressive and obstructive tumor microenvironment as well as a highly malignant phenotype(42). Thus, gaining insight into the tumor environment is critical to improving immunotherapy. Given the impact of GABRP expression on immune infiltration in pancreatic cancer, we speculated whether the expression level of GABRP was associated with the status of the tumor microenvironment. ESTIMATE was used to evaluate the composition and infiltration of immune cells and stromal cells in the tumor microenvironment using gene expression signatures based on GABRP expression. Compared to the cohorts with lower GABRP expression, higher GABRP expression was significantly correlated with higher immune score, stromal score and lower tumor purity (Figure 9A-9D). Furthermore, we examined the expression of six immune checkpoint genes including PDCD1, CD274, CTLA4, PDCD1LG2, TIGHT and TIM3), which participated in immune escape and affected immunotherapy response. The results showed that expression of the above immune checkpoint genes was significantly upregulated in the cohort with higher GABRP expression (Figure 9E-9J, P<0.05).
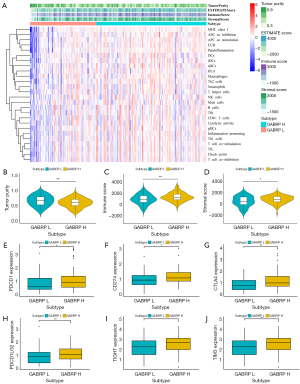
Discussion
Pancreatic cancer is one of the most fatal malignancies worldwide with the worst survival of all carcinomas(43). In recent years, the incorporation of immunotherapy has greatly revitalized and achieved great therapeutic advances in the treatment of many solid tumors(44). However, pancreatic cancer remains almost completely resistant to immunotherapy and the majority of clinical trials related to immunotherapy failed to prove clinical efficacy in pancreatic cancer (7). Thus, it is worthwhile to identify novel prognostic biomarker and therapeutic targets as well as to understand the factors affecting the efficacy of immunotherapy for pancreatic cancer. In this study, we performed a systematic bioinformatics analysis of GABRP in pancreatic cancer integrating the mRNA expression profiles from TCGA-PAAD and GEO cohorts. In pancreatic cancer, enhanced GABRP expression was correlated with a worse prognosis and was an independent predictor of survival. We found that the expression level of GABRP was associated with the number of infiltrating immune cells and several immune cell markers indicating the role of GABRP in immunological interactions. We found a potential link between GABRP expression and the tumor microenvironment, highlighting the need for further research to elucidate the immunological role of GABRP in pancreatic cancer.
We assessed GABRP expression in pancreatic cancer compared with normal tissues and found that GABRP was significantly upregulated in pancreatic cancer using the independent Oncomine and GEPIA2 databases, which was further validated in two GEO datasets (GSE15471 and GSE16515). We observed a remarkable correlation between GABRP and relatively poor prognosis in pancreatic cancer related to OS and DFS. Multivariate analysis revealed that the expression level of GABRP was an independent risk factor for unfavorable survival in pancreatic cancer. However, GABRP expression was not correlated with TNM stage, but grade, in the TCGA cohort, which may be due to the limited number of cases with metastasis. To further validate the prognostic value of GABRP in pancreatic cancer, nomograms were constructed based on GABRP expression. Even if the nomograms reached a moderate prognostic accuracy (C index =0.541), they may not be precise enough due to the lack of enough independent risk factors identified in multivariate Cox analysis. However, the above results suggested that GABRP holds promise as a valuable prognostic biomarker for pancreatic cancer to improve clinical decisions.
We further explored the potential mechanism of GABRP overexpression in pancreatic cancer from the perspective of epigenetics. DNA methylation plays an important role in regulating gene expression and aberrant DNA methylation is involved in cancer progression(45). DNA hypomethylation is frequently observed in human tumors and contributes to the aberrant activation of specific genes related to cancer progression(46). In pancreatic cancer, there exists a significantly negative correlation between GABRP methylation and GABRP mRNA expression. Moreover, methylation of the GABRP promoter was significantly upregulated in pancreatic tumor tissues. The above analysis implied that the enhanced expression of GABRP might be partially attributed to DNA hypomethylation. Micro-RNAs (miRNAs) can regulate the expression of target genes in many processes and have been identified as significant participants in the progression of pancreatic cancer(47). In the present study, hsa-miR-3655 was identified as a candidate regulatory miRNA for GABRP, and we showed that the expression of hsa-miR-3655 was significantly downregulated in pancreatic cancer compared with normal controls, and its downregulation was related to poor prognosis. The putative binding between the GABRP 3’-UTR site and miR-3655 further confirmed that miR-3655 could be a potential upstream regulator of GABRP in pancreatic cancer. The above findings suggested that epigenetic regulation might contribute to the dysregulation of GABRP.
To further clarify the underlying biological function of GABRP in pancreatic cancer, GSEA was performed to determine GABRP co-expressed genes. GO analysis showed consistent enrichment of genes in several immunologically relevant biological processes and pathways, which indicated that GABRP might participate in immune responses. An inflammatory microenvironment is now recognized to be an integral factor contributing to tumorigenesis(48). KEGG pathway analysis showed that several inflammation-related pathways were enriched such as the NOD-like receptor pathway, NF kappa B pathway, and TNF pathway, all of which are crucial inflammatory regulators involved in tumor initiation and progression(49-51). The results of GSEA analysis suggested that the role of GABRP in pancreatic cancer was closely connected with an inflammatory immune response.
Immune checkpoint therapy (ICT) has demonstrated great clinical benefit in several cancer treatments in recent years, although pancreatic cancer studies reported limited clinical response to ICT (52-54). Thus, a comprehensive and in depth understanding of tumor immune environment and immune infiltration in pancreatic cancer could greatly assist in the development of effective immunotherapeutic strategies for pancreatic cancer. We compared the immune infiltration landscape between pancreatic cancer and normal controls and found an obvious increase in macrophages, especially M0 macrophages, and CD4+ T cells, and a decrease in CD8+ T cells. The infiltration of macrophages into solid tumors was associated with poor survival outcomes while the infiltration of CD8+ T cells was correlated with better prognosis (55,56). We further explored this subject and found a relationship between GABRP expression and immune infiltration. The expression of GABRP was positively correlated with the infiltration of B cells, CD8+ T cells, macrophages, neutrophils and dendritic cells, and GABRP copy number alteration was associated with B cells, CD4+ T cells, macrophages, and neutrophil infiltration in PAAD. In addition, GABRP expression was associated with different immune cell markers, and the differences in numbers of infiltrating immune cells could significantly affect the prognosis of pancreatic cancer patients. All the above results indicated that GABRP was correlated with immune infiltration which affected the prognosis of pancreatic cancer.
The immune cells in the pancreatic cancer microenvironment play a significant role in tumorigenesis and progression (57). Given the impact of GABRP on immune infiltration, we further explored the relationship between GABRP expression and the components of the pancreatic cancer microenvironment using ESTIMATE to evaluate 22 types of immune cells and stromal cells in tumor samples. We found that higher GABRP expression was significantly correlated with higher immune score and stromal score and lower tumor purity, which was in accordance with the analysis of immune infiltration via TIMER. Inflammation is intimately associated with immune responses, and inflammatory infiltration into the pancreatic cancer microenvironment contributed to tumor growth and metastasis(58). Pancreatic cancer is characterized by a pathological hallmark of stroma desmoplasia, which creates a mechanical barrier that reduces chemotherapy efficacy and results in low immune cell infiltration(57). According to our results, higher GABRP expression was correlated with increased density of immune cells and stromal cells, which resulted in lower tumor purity and complexity of the tumor microenvironment. The above results indicated an underlying connection between GABRP and tumor microenvironment. Higher GABRP expression was markedly associated with higher expression of six immune checkpoint genes, indicating that GABRP expression might potentially affect the efficacy of immunotherapy(59). The above correlation might provide new insights into immunological treatment for pancreatic cancer patients who received limited benefit from immune checkpoint inhibitors.
In summary, GABRP is a potential prognostic marker for pancreatic cancer and correlated with immune infiltration and tumor microenvironment. However, the present study still has some limitations. While the conclusions of this study were based on GABRP mRNA and protein expression, our results only indicated an overall relationship between GABRP and immune infiltration and tumor microenvironment. Further studies are needed to verify the epigenetics regulation of GABRP expression level and the underlying mechanism of the specific interactions between GABRP, immune infiltration and tumor environment.
Conclusions
In the present study, we demonstrated that GABRP was overexpressed in pancreatic cancer and that enhanced GABRP expression correlated with poor prognosis. Promoter hypomethylation and the regulation of hsa-miR-3655 might contribute to the overexpression of GABRP in pancreatic cancer. GABRP expression was correlated with immune infiltration and microenvironment. Further studies are needed to elucidate the molecular mechanism of the regulatory role of GABRP on the immune response.
Acknowledgments
We would like to thank Gary Bentley for his help in polishing our paper.
Funding: This research was funded by Beijing Natural Science Foundation (7212157), CAMS Innovation Fund for Medical Sciences (2016-I2M-3-007), National Natural Science Foundation of China (81803584, 81703536), Technology Major Projects for “Major New Drugs Innovation and Development” (2018ZX09711001-005-025, 2018ZX09711001-012).
Footnote
Reporting Checklist: The authors have completed the REMARK reporting checklist. Available at https://tcr.amegroups.com/article/view/10.21037/tcr-21-2021/rc
Data Sharing Statement: Available at https://tcr.amegroups.com/article/view/10.21037/tcr-21-2021/dss
Peer Review File: Available at https://tcr.amegroups.com/article/view/10.21037/tcr-21-2021/prf
Conflicts of Interest: All authors have completed the ICMJE uniform disclosure form (available at https://tcr.amegroups.com/article/view/10.21037/tcr-21-2021/coif). The authors have no conflicts of interest to declare.
Ethical Statement: The authors are accountable for all aspects of the work in ensuring that questions related to the accuracy or integrity of any part of the work are appropriately investigated and resolved. The study was conducted in accordance with the Declaration of Helsinki (as revised in 2013). Institutional ethical approval and informed consent were waived.
Open Access Statement: This is an Open Access article distributed in accordance with the Creative Commons Attribution-NonCommercial-NoDerivs 4.0 International License (CC BY-NC-ND 4.0), which permits the non-commercial replication and distribution of the article with the strict proviso that no changes or edits are made and the original work is properly cited (including links to both the formal publication through the relevant DOI and the license). See: https://creativecommons.org/licenses/by-nc-nd/4.0/.
References
- Sung H, Ferlay J, Siegel RL, et al. Global Cancer Statistics 2020: GLOBOCAN Estimates of Incidence and Mortality Worldwide for 36 Cancers in 185 Countries. CA Cancer J Clin 2021;71:209-49. [Crossref] [PubMed]
- Collisson EA, Bailey P, Chang DK, et al. Molecular subtypes of pancreatic cancer. Nat Rev Gastroenterol Hepatol 2019;16:207-20. [Crossref] [PubMed]
- McGuigan A, Kelly P, Turkington RC, et al. Pancreatic cancer: A review of clinical diagnosis, epidemiology, treatment and outcomes. World J Gastroenterol 2018;24:4846-61. [Crossref] [PubMed]
- Conroy T, Hammel P, Hebbar M, et al. FOLFIRINOX or Gemcitabine as Adjuvant Therapy for Pancreatic Cancer. N Engl J Med 2018;379:2395-406. [Crossref] [PubMed]
- Mizrahi JD, Surana R, Valle JW, et al. Pancreatic cancer. Lancet 2020;395:2008-20. [Crossref] [PubMed]
- Wainberg ZA, Hochster HS, Kim EJ, et al. Open-label, Phase I Study of Nivolumab Combined with nab-Paclitaxel Plus Gemcitabine in Advanced Pancreatic Cancer. Clin Cancer Res 2020;26:4814-22. [Crossref] [PubMed]
- Schizas D, Charalampakis N, Kole C, et al. Immunotherapy for pancreatic cancer: A 2020 update. Cancer Treat Rev 2020;86:102016. [Crossref] [PubMed]
- Neoptolemos JP, Kleeff J, Michl P, et al. Therapeutic developments in pancreatic cancer: current and future perspectives. Nat Rev Gastroenterol Hepatol 2018;15:333-48. [Crossref] [PubMed]
- Miller PS, Scott S, Masiulis S, et al. Structural basis for GABAA receptor potentiation by neurosteroids. Nat Struct Mol Biol 2017;24:986-92. [Crossref] [PubMed]
- Zhang X, Du Z, Liu J, et al. Γ-aminobutyric acid receptors affect the progression and migration of tumor cells. J Recept Signal Transduct Res 2014;34:431-9. [Crossref] [PubMed]
- Sigel E, Steinmann ME. Structure, function, and modulation of GABA(A) receptors. J Biol Chem 2012;287:40224-31. [Crossref] [PubMed]
- Zafrakas M, Chorovicer M, Klaman I, et al. Systematic characterisation of GABRP expression in sporadic breast cancer and normal breast tissue. Int J Cancer 2006;118:1453-9. [Crossref] [PubMed]
- Hedblom E, Kirkness EF. A novel class of GABAA receptor subunit in tissues of the reproductive system. J Biol Chem 1997;272:15346-50. [Crossref] [PubMed]
- Sadeghi H, Taylor HS. HOXA10 regulates endometrial GABAA {pi} receptor expression and membrane translocation. Am J Physiol Endocrinol Metab 2010;298:E889-93. [Crossref] [PubMed]
- Wali VB, Patwardhan GA, Pelekanou V, et al. Identification and Validation of a Novel Biologics Target in Triple Negative Breast Cancer. Sci Rep 2019;9:14934. [Crossref] [PubMed]
- Sung HY, Yang SD, Ju W, et al. Aberrant epigenetic regulation of GABRP associates with aggressive phenotype of ovarian cancer. Exp Mol Med 2017;49:e335. [Crossref] [PubMed]
- Li Y, Huang Y, Zhou C, et al. MiR-320c prevents the malignant development of cervical cancer by regulating GABRP level. Eur Rev Med Pharmacol Sci 2020;24:8731-9. [PubMed]
- Jiang SH, Zhu LL, Zhang M, et al. GABRP regulates chemokine signalling, macrophage recruitment and tumour progression in pancreatic cancer through tuning KCNN4-mediated Ca2+ signalling in a GABA-independent manner. Gut 2019;68:1994-2006. [Crossref] [PubMed]
- Badea L, Herlea V, Dima SO, et al. Combined gene expression analysis of whole-tissue and microdissected pancreatic ductal adenocarcinoma identifies genes specifically overexpressed in tumor epithelia. Hepatogastroenterology 2008;55:2016-27. [PubMed]
- Li L, Zhang JW, Jenkins G, et al. Genetic variations associated with gemcitabine treatment outcome in pancreatic cancer. Pharmacogenet Genomics 2016;26:527-37. [Crossref] [PubMed]
- Rhodes DR, Kalyana-Sundaram S, Mahavisno V, et al. Oncomine 3.0: genes, pathways, and networks in a collection of 18,000 cancer gene expression profiles. Neoplasia 2007;9:166-80. [Crossref] [PubMed]
- Tang Z, Kang B, Li C, et al. GEPIA2: an enhanced web server for large-scale expression profiling and interactive analysis. Nucleic Acids Res 2019;47:W556-60. [Crossref] [PubMed]
- Nagy Á, Lánczky A, Menyhárt O, et al. Validation of miRNA prognostic power in hepatocellular carcinoma using expression data of independent datasets. Sci Rep 2018;8:9227. [Crossref] [PubMed]
- Cerami E, Gao J, Dogrusoz U, et al. The cBio cancer genomics portal: an open platform for exploring multidimensional cancer genomics data. Cancer Discov 2012;2:401-4. [Crossref] [PubMed]
- Agarwal V, Bell GW, Nam JW, et al. Predicting effective microRNA target sites in mammalian mRNAs. Elife 2015; [PubMed]
- Chen Y, Wang X. miRDB: an online database for prediction of functional microRNA targets. Nucleic Acids Res 2020;48:D127-31. [Crossref] [PubMed]
- Sticht C, De La Torre C, Parveen A, et al. miRWalk: An online resource for prediction of microRNA binding sites. PLoS One 2018;13:e0206239. [Crossref] [PubMed]
- Chandrashekar DS, Bashel B, Balasubramanya SAH, et al. UALCAN: A Portal for Facilitating Tumor Subgroup Gene Expression and Survival Analyses. Neoplasia 2017;19:649-58. [Crossref] [PubMed]
- Vasaikar SV, Straub P, Wang J, et al. LinkedOmics: analyzing multi-omics data within and across 32 cancer types. Nucleic Acids Res 2018;46:D956-63. [Crossref] [PubMed]
- Li T, Fan J, Wang B, et al. TIMER: A Web Server for Comprehensive Analysis of Tumor-Infiltrating Immune Cells. Cancer Res 2017;77:e108-10. [Crossref] [PubMed]
- Danaher P, Warren S, Dennis L, et al. Gene expression markers of Tumor Infiltrating Leukocytes. J Immunother Cancer 2017;5:18. [Crossref] [PubMed]
- Newman AM, Liu CL, Green MR, et al. Robust enumeration of cell subsets from tissue expression profiles. Nat Methods 2015;12:453-7. [Crossref] [PubMed]
- Yoshihara K, Shahmoradgoli M, Martínez E, et al. Inferring tumour purity and stromal and immune cell admixture from expression data. Nat Commun 2013;4:2612. [Crossref] [PubMed]
- Camp RL, Dolled-Filhart M, Rimm DL. X-tile: a new bio-informatics tool for biomarker assessment and outcome-based cut-point optimization. Clin Cancer Res 2004;10:7252-9. [Crossref] [PubMed]
- Pei H, Li L, Fridley BL, et al. FKBP51 affects cancer cell response to chemotherapy by negatively regulating Akt. Cancer Cell 2009;16:259-66. [Crossref] [PubMed]
- Ellsworth KA, Eckloff BW, Li L, et al. Contribution of FKBP5 genetic variation to gemcitabine treatment and survival in pancreatic adenocarcinoma. PLoS One 2013;8:e70216. [Crossref] [PubMed]
- Ehrlich M. DNA hypermethylation in disease: mechanisms and clinical relevance. Epigenetics 2019;14:1141-63. [Crossref] [PubMed]
- Aran D, Sirota M, Butte AJ. Systematic pan-cancer analysis of tumour purity. Nat Commun 2015;6:8971. [Crossref] [PubMed]
- Li T, Fu J, Zeng Z, et al. TIMER2.0 for analysis of tumor-infiltrating immune cells. Nucleic Acids Res 2020;48:W509-14. [Crossref] [PubMed]
- Yang J, Li H, Hu S, et al. ACE2 correlated with immune infiltration serves as a prognostic biomarker in endometrial carcinoma and renal papillary cell carcinoma: implication for COVID-19. Aging (Albany NY) 2020;12:6518-35. [Crossref] [PubMed]
- Xiao Z, Hu L, Yang L, et al. TGFβ2 is a prognostic-related biomarker and correlated with immune infiltrates in gastric cancer. J Cell Mol Med 2020;24:7151-62. [Crossref] [PubMed]
- Bazzichetto C, Conciatori F, Luchini C, et al. From Genetic Alterations to Tumor Microenvironment: The Ariadne's String in Pancreatic Cancer. Cells 2020;9:309. [Crossref] [PubMed]
- Leinwand J, Miller G. Regulation and modulation of antitumor immunity in pancreatic cancer. Nat Immunol 2020;21:1152-9. [Crossref] [PubMed]
- Hegde PS, Chen DS. Top 10 Challenges in Cancer Immunotherapy. Immunity 2020;52:17-35. [Crossref] [PubMed]
- Choudhury JH, Das R, Laskar S, et al. Detection of p16 Promoter Hypermethylation by Methylation-Specific PCR. Methods Mol Biol 2018;1726:111-22. [Crossref] [PubMed]
- Van Tongelen A, Loriot A, De Smet C. Oncogenic roles of DNA hypomethylation through the activation of cancer-germline genes. Cancer Lett 2017;396:130-7. [Crossref] [PubMed]
- Qadir MI, Faheem A. miRNA: A Diagnostic and Therapeutic Tool for Pancreatic Cancer. Crit Rev Eukaryot Gene Expr 2017;27:197-204. [Crossref] [PubMed]
- Hanahan D, Weinberg RA. Hallmarks of cancer: the next generation. Cell 2011;144:646-74. [Crossref] [PubMed]
- Liu P, Lu Z, Liu L, et al. NOD-like receptor signaling in inflammation-associated cancers: From functions to targeted therapies. Phytomedicine 2019;64:152925. [Crossref] [PubMed]
- Balkwill F. TNF-alpha in promotion and progression of cancer. Cancer Metastasis Rev 2006;25:409-16. [Crossref] [PubMed]
- Hoesel B, Schmid JA. The complexity of NF-κB signaling in inflammation and cancer. Mol Cancer 2013;12:86. [Crossref] [PubMed]
- Demaria O, Cornen S, Daëron M, et al. Harnessing innate immunity in cancer therapy. Nature 2019;574:45-56. [Crossref] [PubMed]
- Blando J, Sharma A, Higa MG, et al. Comparison of immune infiltrates in melanoma and pancreatic cancer highlights VISTA as a potential target in pancreatic cancer. Proc Natl Acad Sci U S A 2019;116:1692-7. [Crossref] [PubMed]
- Jiang J, Zhou H, Ni C, et al. Immunotherapy in pancreatic cancer: New hope or mission impossible? Cancer Lett 2019;445:57-64. [Crossref] [PubMed]
- Cassetta L, Pollard JW. Targeting macrophages: therapeutic approaches in cancer. Nat Rev Drug Discov 2018;17:887-904. [Crossref] [PubMed]
- Hou YC, Chao YJ, Hsieh MH, et al. Low CD8+ T Cell Infiltration and High PD-L1 Expression Are Associated with Level of CD44+/CD133+ Cancer Stem Cells and Predict an Unfavorable Prognosis in Pancreatic Cancer. Cancers (Basel) 2019;11:541. [Crossref] [PubMed]
- Ho WJ, Jaffee EM, Zheng L. The tumour microenvironment in pancreatic cancer - clinical challenges and opportunities. Nat Rev Clin Oncol 2020;17:527-40. [Crossref] [PubMed]
- Padoan A, Plebani M, Basso D. Inflammation and Pancreatic Cancer: Focus on Metabolism, Cytokines, and Immunity. Int J Mol Sci 2019;20:676. [Crossref] [PubMed]
- Tang C, Ma J, Liu X, et al. Identification of Four Immune Subtypes in Bladder Cancer Based on Immune Gene Sets. Front Oncol 2020;10:544610. [Crossref] [PubMed]