Elevated RECQL1 expression predicts poor prognosis and associates with tumor immune infiltration in low-grade glioma
Introduction
Glioma is the most prevalent and devastating primary tumor in the adult central nervous system (CNS) (1), with a high recurrence rate and short survival (2). Although the survival time of low-grade glioma (LGG) patients is relatively longer compared with glioblastoma (GBM, WHO grade IV), it is still an urgent problem that need to be solved to reduce the postoperative recurrence and mortality rate of LGG after surgery (3). Despite the tremendous progress made in adjuvant therapy and surgical procedures in the past few decades, they have not significantly improved the prognosis of patients as much as we expected.
Genetic heterogeneity remains a barrier to improving the clinical outcomes of LGG (4) and is responsible for different treatment response and prognosis of patients (3). The well-known and accepted prognostic signatures of glioma include isocitrate dehydrogenase (IDH) mutation (5), 1p/19q codeletion status (6) and telomerase reverse transcriptase (TERT) mutation (7), etc. These changes often indicate different treatment responses of patients. In the process of exploring effective treatment options, the relationship between the tumor microenvironment (TME) and immunotherapy has received increasing attention. Studies have suggested that metastasis and insensitivity to therapy of glioma are attributed to infiltration of immune cells into lesions (8,9). Immunotherapy, which altered the treatment paradigm for glioma, has been adopted to the therapy of glioma (10). Genes related to immune components of tumor microenvironment have important value as prognostic markers (11). Hence, it is crucial to explore novel prognostic signatures associated with tumor immune infiltration and synergize with the aforementioned markers to ameliorate diagnosis and predict patient prognosis more accurately, to clarify the molecular mechanism of LGG to improve treatment strategies more efficiently.
The RecQ DNA helicases unwind DNA double-strand in a 3′ to 5′ direction and maintains genome integrity by playing a pivotal role in multiple DNA repair pathways (12). Five proteins of RecQ family were found in human cells: dysregulation of RecQ protein-like 1 (RECQL1) (also known as RECQL or RECQ1), WRN (also known as RECQL2), BLM (also known as RECQL3), RECQL4 and RECQ5 (12,13). Except leading to genome instability due to its deletion (13), mutations in genes encoding RecQ DNA helicase occurs in certain rare diseases, such as Bloom’s syndrome and Werner’s syndrome (14,15). For many years, extensive literatures pay attention to the exploration of the correlation between RECQL1 and malignancies, including oral and tongue squamous cell carcinoma (16,17), ovarian cancer (18,19), head and neck squamous cell carcinoma (20), hepatocellular carcinoma (21) and glioblastoma (22). RECQL1 is primarily involved in cell proliferation and can be used to prognostic biomarker in these cancers studied. Besides, RECQL1 has been shown to correlate with the expression levels of immunosuppressive factors in tongue squamous cell carcinoma (17). Based on the aforementioned research conclusions, we infer that RECQL1 is associated with immune infiltration of LGG and has value as a potential prognostic molecule.
In the current study, we focused on the expression and prognostic significance of RECQL1 in LGG, as well as whether it is involved in tumor immune infiltration of LGG through a multi-cohort large-scale bioinformatics analysis. We present the following article in accordance with the TRIPOD reporting checklist (available at https://tcr.amegroups.com/article/view/10.21037/tcr-21-2762/rc).
Methods
Data preparation
Sequencing data of 505 LGG samples in Fragments Per Kilobase of exon model per Million mapped fragments (FPKM) format was acquired from The Cancer Genome Atlas (TCGA, https://portal.gdc.cancer.gov/) and used as the training cohort. The clinical information matched to each sample was collected from two literatures published in 2016 and 2018 (23,24). 418 samples from the Chinese Glioma Genome Atlas (CGGA, http://www.cgga.org.cn/) (25) were employed as the validation cohort. All samples in both cohorts have complete follow-up information and clinical characteristics data (Table S1). The mRNA expression profile of Gravendeel (26) and Rembrandt (27) datasets were collected from the GLIOVIS database (http://GLIOVIS.bioinfo.cnio.es/), while the microarray data of GSE4290 (28) was taken from Gene Expression Omnibus (GEO) database (https://www.ncbi.nlm.nih.gov/geo). Gene Expression Profiling Interactive Analysis 2 (GEPIA2) database, including expression profile data of tumor samples (from TCGA) and normal samples (from Genotype-Tissue Expression Project) (29), was also employed. The study was conducted in accordance with the Declaration of Helsinki (as revised in 2013). Our research is based on open-source data from public databases. The patients involved in the databases have obtained ethical approval, so there are no ethical issues.
Differential expression analysis
GSE4290, Rembrandt and Gravendeel datasets were used to initially distinguish the mRNA expression level of RecQ helicases between LGG and non-tumor samples, while GEPIA2 was used to verify the difference analysis results and analyze the expression levels of RECQL1 in different histological subtypes. Among the five genes tested, only RECQL1 meets the criteria for identifying differentially expressed gene (DEG) between low-grade glioma (LGG) and non-tumor specimens: (I) differential expression is statistically significant in all the three datasets; (II) meaningful cut-off of GEPIA2: |log2FC|>1.5, q value <0.01. The expression difference of RECQL1 among different WHO grades was based on expression profile analysis of TCGA and CGGA.
Survival analysis and construction of prediction model
Firstly, patients from TCGA and CGGA were separated into two cohorts (RECQL1high and RECQL1low) according to the median expression value of RECQL1. The Kaplan-Meier (K-M) plots was used for visualization of survival analysis to explore the correlation between the RECQL1 expression level and overall survival (OS) or progression-free survival (PFS) in LGG patients. Univariate and multivariate Cox regression analysis further evaluated whether the expression level of RECQL1 and six clinical variables [age (continuous, years), gender (ref. female), grade (ref. WHO II), IDH mutation status (ref. mutant), 1p/19q codeletion status (ref. non-codeletion) and O-6-methylguanine-DNA methyltransferase (MGMT) promoter status (ref. unmethylated)] are independent prognostic factors related to patients’ OS and PFS. The same method was used to analyze samples from CGGA cohort for validation.
A Cox proportional hazard model was constructed based on the expression of RECQL1 and the four meaningful variables (age, grade, IDH mutation status, 1p/19q codeletion status) to assess the risk scores of each patient. Subsequently, the K-M plots were plotted after grouping by the median risk score. A nomogram for clinical prediction was generated by using the R software “rms” package based on the independent prognostic factors derived from COX regression analysis before. Concordance index (C-index) and calibration curve were used to evaluate the predictive performance of the nomogram model, where C-index >0.7 was considered acceptable for the model. Data obtained from TCGA and CGGA were used for training set and external validation set respectively.
Functional enrichment analysis
Gene Set Enrichment Analysis (GSEA) can interpret gene expression data by using predefined gene sets (30,31). GSEA software (v4.1.0) was used to perform Gene Ontology (GO) and Kyoto Encyclopedia of Genes and Genomes (KEGG) analysis to screen for biological pathways with significant differences between the RECQL1high and RECQL1low groups to illuminate the potential role of RECQL1 in LGG. The terms with |NES|>2, Nom P value<0.001 and FDR q value <0.001 were considered as significant pathways.
Immune infiltration analysis
Tumor Immune Estimation Resource (TIMER) provides six analysis modules to comprehensively explore the molecular characteristics of tumor immune interactions (32), which was used to calculate the correlation between the expression of RECQL1 and the abundance of tumor infiltrating immune cells (TIICs), and to explore whether those variables are independent factors predicting the prognosis of LGG. We also used this tool to explore the correlation between RECQL1 and the genetic markers of 16 immune infiltrating cells, which were obtained from published researches (33-35). The R “GSVA” package (36) was used for single sample Gene Set Enrichment Analysis (ssGSEA) based on RNA sequencing data of TCGA to quantify tumor infiltration of 28 tumor-infiltrating lymphocytes (TILs) (Figure S1). Gene signatures of those lymphocytes were downloaded from the Tumor-Immune System Interaction Database (TISIDB) website (37).
Statistical analysis
The differences between two groups (Normal vs. Tumor, RECQL1Highvs. RECQL1Low) were compared using Wilcox test. Spearman analysis was adopted to calculate the relationship between two different variables. Survival analysis was completed by “survival” and “survminer” R packages. Statistical analysis and drawing were done in R project (version 4.1.0). Unless otherwise specified, a two-sided P value less than 0.05 was set as a statistically significant threshold.
Results
Expression level of RecQ DNA helicases in LGG
The differential expression of five genes encoding RecQ DNA helicases in non-tumor and LGG samples was conducted in Rembrandt, Gravendeel and GSE4290 datasets, and subsequently verified by the GEPIA2 database. The expression of RECQL2 between tumor group and non-tumor group in the Rembrandt, Gravendeel and GSE4290 datasets was statistically significant, while RECQL3, RECQL4 and RECQL5 were not (Figure S2).
However, the expression of the above four genes in the GEPIA2 database had no difference according to the setting criteria (Figure S2D,S2H,S2L,S2P). Obviously, the differential expression analysis of RECQL1 is not only significant in the above three datasets (Figure 1A-1C), but also meets the criteria for identifying differential genes in the GEPIA2 database (Figure 1D).
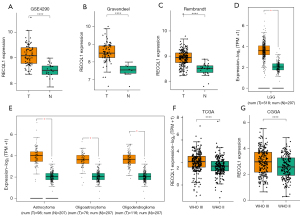
Compared with non-tumor samples, the expression level of RECQL1 in the three histological subtypes (astrocytoma, oligoastrocytoma and oligodendroglioma) of LGG was significantly up-regulated according to the GEPIA2 database (Figure 1E). In order to illuminate the potential association between the expression of RECQL1 and LGG, we also analyzed the sequencing data. Obviously, the expression level of RECQL1 is positively correlated with the WHO classification of LGG (Figure 1F,1G), which demonstrated that RECQL1 might be correlate with the development of LGG. Hence, we used RECQL1 as a candidate gene for subsequent analysis.
RECQL1 overexpression predicts a poorer prognosis of LGG patients
Using TCGA and CGGA cohorts, we investigated the prognostic potential of RECQL1 for LGG patients. Firstly, patients in the two cohorts were separated into RECQL1High and RECQL1Low groups according to the median expression value of RECQL1. From the survival status distribution of the two groups of patients, it was concluded that the survival status of RECQL1High group was more terrible than that of the RECQL1Low group (Figure 2A,2B), which indicated RECQL1 may be an accomplice of LGG. K-M plots betokened that high RECQL1 expression was dramatically associated with poor overall survival (Figure 2C,2D) and progression-free survival (Figure 2E).
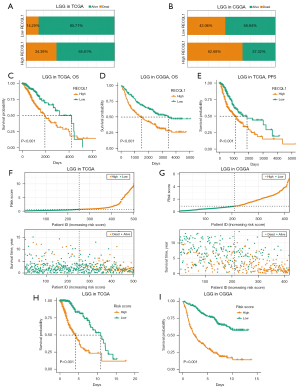
Subsequently, we included RECQL1 expression level and the six clinical characteristics mentioned in the methods into the Cox regression analysis to explore the independent prognostic factors of LGG patients. The univariate analysis of TCGA and CGGA cohorts revealed that high RECQL1 expression was significantly relevant to a poorer OS (Table S2).
In multivariate analysis, high RECQL1 expression was independently associate with a poorer OS in LGG (Table 1). Both univariate and multivariate analysis of TCGA cohort indicated RECQL1 was also independently associated with PFS (Table 2). Other variables associated with patient’ survival including age, WHO grade, IDH mutation status and 1p/19q codeletion status. We further calculated the risk scores of each patient by combining the expression of RECQL1 and the four independent index (Figure 2F,2G). The clinical outcome of the high-risk group was significantly worse than that of the low-risk group (Figure 2H,2I). Overall, these results indicated that RECQL1 expression level can strongly predict the prognosis of LGG patients independently.
Table 1
Covariates | TCGA cohort (n=505) | CGGA cohort (n=418) | |||||
---|---|---|---|---|---|---|---|
HR | 95% CI | P | HR | 95% CI | P | ||
Age | 1.054 | 1.037–1.071 | <0.001 | 1.013 | 1.001–1.025 | 0.041 | |
Grade | 1.91 | 1.252–2.914 | <0.01 | 2.496 | 1.847–3.373 | <0.001 | |
IDH | 0.372 | 0.206–0.671 | <0.01 | 0.647 | 0.481–0.871 | <0.01 | |
1p/19q | 0.429 | 0.254–0.725 | <0.01 | 0.322 | 0.22–0.47 | <0.001 | |
MGMT | 1.163 | 0.682–1.984 | 0.579 | – | – | – | |
RECQL1 | 1.464 | 1.165–1.84 | <0.01 | 1.412 | 1.228–1.623 | <0.001 |
OS, overall survival; TCGA, The Cancer Genome Atlas; CGGA, the Chinese Glioma Genome Atlas; HR, hazard ratio; CI, confidence interval; P, P value; IDH, IDH mutation status;1p/19q, 1p/19q codeletion status; MGMT, MGMT promoter status.
Table 2
Covariates | Univariate Cox | Multivariate Cox | |||||
---|---|---|---|---|---|---|---|
HR | 95% CI | P | HR | 95% CI | P | ||
Age (continuous, years) | 1.03 | 1.018–1.041 | <0.001 | 1.02 | 1.008–1.033 | <0.01 | |
Gender (ref. female) | 0.867 | 0.651–1.154 | 0.328 | – | – | – | |
Grade (ref. WHO II) | 1.649 | 1.232–2.208 | <0.001 | 1.082 | 0.79–1.481 | 0.624 | |
IDH (ref. mutant) | 0.176 | 0.128–0.243 | <0.001 | 0.258 | 0.161–0.412 | <0.001 | |
1p/19q | 0.426 | 0.299–0.607 | <0.001 | 0.542 | 0.367–0.802 | <0.01 | |
MGMT (ref. unmethylated). | 0.426 | 0.309–0.586 | <0.001 | 1.111 | 0.734–1.68 | 0.619 | |
RECQL1 (continuous) | 1.38 | 1.177–1.617 | <0.001 | 1.237 | 1.054–1.452 | <0.01 |
PFS, progression-free survival; TCGA, The Cancer Genome Atlas; HR, hazard ratio; CI, confidence interval; P, P value; IDH, IDH mutation status;1p/19q, 1p/19q codeletion status; MGMT, MGMT promoter status.
Construction of clinical prediction model of prognostic factors
According to the results of Cox regression analysis, RECQL1 and four clinical characteristics can independently predict the clinical outcomes of LGG patients. Time-dependent receiver operating characteristic (ROC) curves shows that the areas under the ROC curve for all different follow-up years exceeds 0.7, which testified the prediction efficiency of those variables (Figure 3A,3B). Combining the above findings, a prognostic model was established and visualized by plotting nomogram, through which the total number of points can be acquired to calculate the survival probability of each LGG patient. C-index of the model based on the TCGA data was 0.85 (Figure 3C), while the value obtained by external validation was 0.722 (data not shown). To further evaluate the accuracy of the model, we generated calibration plots. The observed probability (Y-axis) and predicted probability (X-axis) of 1-, 3- and 5-year OS show excellent consistency (Figure 3D,3E). Both demonstrate moderate accuracy and applicability of the prediction model.
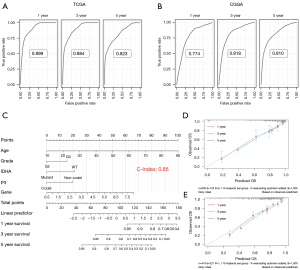
Functional enrichment analysis of RECQL1
GSEA software was used to explore the potential biological pathways that are significantly different between the RECQL1high and RECQL1low groups. We only screened out pathways in the RECQL1high group that meet the criteria mentioned in the methods. Ten pathways of KEGG and five pathways of each GO subtype were selected. GO analysis suggested that high RECQL1 expression is involved in cell proliferation, division, death, metabolism and other processes (Table S3).
In addition to the foregoing results, we also observed that high RECQL1 expression is associated with a variety of tumors, including leukemia, colorectal cancer and prostate cancer (Figure 4A). High RECQL1 expression may affect tumor cell adhesion and JAK-STAT signaling pathway, which suggests that RECQL1 may be able to affect development and immune regulation of LGG.
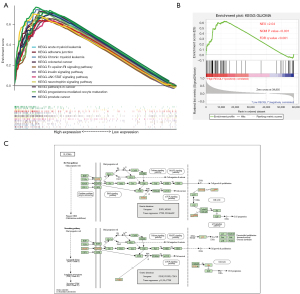
Pathways related to glioma were also obtained according to the results of GSEA analysis (Figure 4B,4C), which demonstrated RECQL1 may participate the biological processes and signal pathways related to the occurrence and development of glioma. All of the above are direct evidence that RECQL1 is involved in LGG-related pathological processes.
RECQL1 expression correlated with tumor immune infiltration
The degree of infiltration of TIICs plays a crucial role in the tumor environment (38), and the degree of immune infiltration is related to the prognosis of patients (39). As the ssGSEA results shown in Figure 5A, the quantification of the degree of infiltration of most immune cells is entirely distinct under different expression states of RECQL1 (RECQL1high and RECQL1low) except five types (effector memory CD4 T cell, type 17 T helper cell, CD56 bright natural killer cell, plasmacytoid dendritic cell and eosinophil).
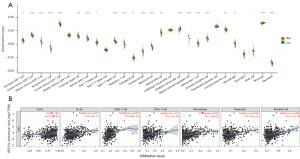
The expression of RECQL1 is positively correlated with the enrichment scores of activated CD8 T cell, central memory CD8 T cell, effector memory CD8 T cell, activated CD4 T cell, central memory CD4 T cell, T follicular helper cell, gamma delta T cell, type 1 T helper cell, type 2 T helper cell, regulatory T cell, activated B cell, immature B cell, memory B cell, natural killer cell, myeloid derived suppressor cell, natural killer T cell, activated dendritic cell, immature dendritic cell, macrophage, mast cell and neutrophil, while negatively correlated with the enrichment scores CD56dim natural killer cell and monocyte. Meanwhile, a considerably positive correlation was found between RECQL1 expression level and infiltrating levels of B cells, CD8+ T cells, CD4+ T cells, macrophages, neutrophils, and dendritic cells (Figure 5B). The RECQL1 expression was also markedly associated with almost all genetic markers of tumor-infiltrating lymphocytes (Table S4).
To find out the connection between clinical outcomes and immune infiltration abundance, a Cox proportional hazard model was established for the expression of RECQL1 and six TIICs through the TIMER database (Table 3). Results derived from the model illustrated that the expression levels of macrophages and RECQL1 are the poor prognosis factors that affects the survival of LGG patients. All the analysis above suggested that RECQL1 may participates in tumor immune infiltration and may affect prognosis of LGG.
Table 3
Covariates | Univariate Cox | Multivariate Cox | |||||
---|---|---|---|---|---|---|---|
HR | 95% CI | P | HR | 95% CI | P | ||
B cell | 830.428 | 54.364–12,685 | 0 | 4.273 | 0.015–1,248.020 | 0.616 | |
CD8+ T cell | 19,943.51 | 1,320.611–301,181.6 | 0 | 76.760 | 0.077–76,085.829 | 0.218 | |
CD4+ T cell | 47.835 | 6.336–361.158 | 0 | 0.022 | 0.000–44.862 | 0.327 | |
Macrophage | 296.664 | 52.011–1,692.124 | 0 | 747.470 | 15.304–36,506.741 | 0.001 | |
Neutrophil | 881.918 | 66.197–11,749.39 | 0 | 0.029 | 0.000–61.884 | 0.365 | |
Dendritic | 10.994 | 4.24–28.506 | 0 | 3.988 | 0.082–193.711 | 0.485 | |
RECQL1 | 1.869 | 1.515–2.305 | 0 | 1.376 | 1.054–1.795 | 0.019 |
OS, overall survival; TCGA, The Cancer Genome Atlas; HR, hazard ratio; CI, confidence interval; P, P value.
Discussion
Attributing to their aggressive nature, LGG cannot be completely excised, and progress frequently to glioblastoma (40). More than 50% of LGG patients die from tumor recurrence or disease progression (41). Biomarkers related to LGG can be identified by large-scale bioinformatics analysis, which can be used as therapeutic targets and may help to predict patient prognosis. RECQL1 is not only involved in DNA damage repair and maintenance of genome stability, but a large number of studies have revealed its potential accomplice role in tumorigenesis and development.
In this study, we identified RECQL1 expression is up-regulated in LGG contrast to non-tumor counterparts. High RECQL1 expression deteriorate the prognosis of LGG patients and corelate with tumor immune infiltration. Previous studies have demonstrated that RECQL1, whose expression is dysregulated in a variety of cancers including glioblastoma, participate in carcinogenic processes such as promoting cell proliferation and inhabiting apoptosis (16-22). For instance, RECQL1 is over-expression in hypopharyngeal carcinomas, which belongs to the worst-prognosis type of the head and neck squamous cell carcinoma (HNSCC), and can be used as a potential therapeutic target (20). In the study focusing on hepatocellular carcinoma, RECQL1 has the potential as a biomarker for predicting the malignancy and progression of HCC (21). RECQL1 was detected in the nucleus of ovarian cancer (OC) cells and was found to be a marker of highly proliferating cells in OC (18,19). What is more noteworthy is that, in the research published in 2011, it was proposed that RECQL1 is up-regulated in GBM and participated in cell proliferation (22). All these studies suggest that it is essential to explore the connection between RECQL1 and LGG. With the exception of supplementing the expression information and prognostic significance of RECQL1 in LGG, we proposed that RECQL1 could be used as a standalone index predicting the clinical outcomes of LGG patients. And a clinical prediction model was also conducted, which accuracy was verified by calculating C-index and drawing ROC curves. We have convincing reasons to deem that the differential expression and prognostic significance of RECQL1 in LGG, but the signaling pathway and molecular mechanism behind it are still elusive and worthy of further study.
We performed GSEA analysis to explore the potential mechanisms related to RECQL1 in LGG. KEGG analysis by GSEA shown that high RECQL1 expression not only significantly associated with pathways of different cancers including leukemia, colorectal cancer and prostate cancer, but also with cell proliferation, division and etc. In particular, we found that high RECQL1 expression was also related to pathway of glioma, more specific and strongly suggesting the villain role of RECQL1 in glioma. It should be pointed out that we did not find a pathway that meets the criteria in the low RECQL1 expression group. Besides, among the 10 KEGG pathways shown above, two are worth noting, including adherens junction and JAK-STAT signaling pathways. Adherens junction is vital for maintaining tissue structure and cell polarity, and can limit cell movement and proliferation. The maintenance of its integrity can inhibit tumor invasiveness (42). JAK-STAT signaling pathway not only regulates tumorigenesis and development, but also participates in almost all immune regulation processes (43). The results of pathway enrichment analysis highly demonstrated that RECQL1 may plays a tumorigenic role in LGG.
One of the important factors of tumor occurrence and development is immune cell infiltration. A study published in 2014 showed that knocking out RECQL1 was followed by the down-regulation of two immunosuppressive factors (IL-10 and VEGF) in tongue squamous cell carcinoma (17). High RECQL1 expression is also related to the JAK-STAT signal according to our analysis before, which can regulate the immune process. Taking the above results into consideration, it is necessary to explore the association between RECQL1 and tumor immune infiltration in LGG. After analyzing multiple databases, we identified that RECQL1 is significantly associated with tumor infiltration of TIICs. The combined analysis of RECQL1 and six TIICs once again confirmed that RECQL1 can strongly and independently predicting the prognosis of LGG population.
Our current research demonstrates the expression, prognostic significance, immune infiltration and possible pathways of RECQL1 in LGG, and reports evidence supporting the candidacy of RECQL1 as a cancer treatment target of LGG for the first time. Nonetheless, there are still some inevitable limitations in the current research that need to be considered and resolved. This study included LGG samples for analysis based on the criteria published by the WHO in 2016, but the classification standards for gliomas are constantly being revised and improved. Hence, it is necessary to further group LGG patients and analyze them to improve the accuracy of the research results. In addition, the concrete molecular mechanism and process of RECQL1 in LGG need to be further determined by in vivo and in vitro experiments.
Acknowledgments
Funding: This work was supported by the Natural Science Foundation of Southwest Medical University (grant No. 2016XNYD217 and No. 2018-ZRQN-032) and Science and Technology Projects of Sichuan Province (grant No. 2018JY0403).
Footnote
Reporting Checklist: The authors have completed the TRIPOD checklist. Available at https://tcr.amegroups.com/article/view/10.21037/tcr-21-2762/rc
Conflicts of Interest: All authors have completed the ICMJE uniform disclosure form (available at https://tcr.amegroups.com/article/view/10.21037/tcr-21-2762/coif). The authors have no conflicts of interest to declare.
Ethical Statement: The authors are accountable for all aspects of the work in ensuring that questions related to the accuracy or integrity of any part of the work are appropriately investigated and resolved. The study was conducted in accordance with the Declaration of Helsinki (as revised in 2013). Our research is based on open-source data from public databases. The patients involved in the databases have obtained ethical approval, so there are no ethical issues.
Open Access Statement: This is an Open Access article distributed in accordance with the Creative Commons Attribution-NonCommercial-NoDerivs 4.0 International License (CC BY-NC-ND 4.0), which permits the non-commercial replication and distribution of the article with the strict proviso that no changes or edits are made and the original work is properly cited (including links to both the formal publication through the relevant DOI and the license). See: https://creativecommons.org/licenses/by-nc-nd/4.0/.
References
- Ostrom QT, Patil N, Cioffi G, et al. CBTRUS Statistical Report: Primary Brain and Other Central Nervous System Tumors Diagnosed in the United States in 2013-2017. Neuro Oncol 2020;22:iv1-iv96. [Crossref] [PubMed]
- Jiang T, Mao Y, Ma W, et al. CGCG clinical practice guidelines for the management of adult diffuse gliomas. Cancer Lett 2016;375:263-73. [Crossref] [PubMed]
- Molinaro AM, Taylor JW, Wiencke JK, et al. Genetic and molecular epidemiology of adult diffuse glioma. Nat Rev Neurol 2019;15:405-17. [Crossref] [PubMed]
- Aldape K, Brindle KM, Chesler L, et al. Challenges to curing primary brain tumours. Nat Rev Clin Oncol 2019;16:509-20. [Crossref] [PubMed]
- Yan H, Parsons DW, Jin G, et al. IDH1 and IDH2 mutations in gliomas. N Engl J Med 2009;360:765-73. [Crossref] [PubMed]
- Jenkins RB, Blair H, Ballman KV, et al. A t(1;19)(q10;p10) mediates the combined deletions of 1p and 19q and predicts a better prognosis of patients with oligodendroglioma. Cancer Res 2006;66:9852-61. [Crossref] [PubMed]
- Geng P, Zhao X, Ou J, et al. TERT Genetic Mutations as Prognostic Marker in Glioma. Mol Neurobiol 2017;54:3665-9. [Crossref] [PubMed]
- Ma Q, Long W, Xing C, et al. Cancer Stem Cells and Immunosuppressive Microenvironment in Glioma. Front Immunol 2018;9:2924. [Crossref] [PubMed]
- Audia A, Conroy S, Glass R, et al. The Impact of the Tumor Microenvironment on the Properties of Glioma Stem-Like Cells. Front Oncol 2017;7:143. [Crossref] [PubMed]
- Lim M, Xia Y, Bettegowda C, et al. Current state of immunotherapy for glioblastoma. Nat Rev Clin Oncol 2018;15:422-42. [Crossref] [PubMed]
- Le Rhun E, Preusser M, Roth P, et al. Molecular targeted therapy of glioblastoma. Cancer Treat Rev 2019;80:101896. [Crossref] [PubMed]
- Newman JA, Gileadi O. RecQ helicases in DNA repair and cancer targets. Essays Biochem 2020;64:819-30. [Crossref] [PubMed]
- Hickson ID. RecQ helicases: caretakers of the genome. Nat Rev Cancer 2003;3:169-78. [Crossref] [PubMed]
- Yu CE, Oshima J, Fu YH, et al. Positional cloning of the Werner's syndrome gene. Science 1996;272:258-62. [Crossref] [PubMed]
- German J, Archibald R, Bloom D. Chromosomal breakage in a rare and probably genetically determined syndrome of man. Science 1965;148:506-7. [Crossref] [PubMed]
- Zhang P, Zhang Z, Zhou X, et al. Identification of genes associated with cisplatin resistance in human oral squamous cell carcinoma cell line. BMC Cancer 2006;6:224. [Crossref] [PubMed]
- Tao J, Tao S, Han J, et al. RECQL1 plays an important role in the development of tongue squamous cell carcinoma. Cell Physiol Biochem 2014;33:1579-90. [Crossref] [PubMed]
- Matsushita Y, Yokoyama Y, Yoshida H, et al. The level of RECQL1 expression is a prognostic factor for epithelial ovarian cancer. J Ovarian Res 2014;7:107. [Crossref] [PubMed]
- Sanada S, Futami K, Terada A, et al. RECQL1 DNA repair helicase: a potential therapeutic target and a proliferative marker against ovarian cancer. PLoS One 2013;8:e72820. [Crossref] [PubMed]
- Arai A, Chano T, Futami K, et al. RECQL1 and WRN proteins are potential therapeutic targets in head and neck squamous cell carcinoma. Cancer Res 2011;71:4598-607. [Crossref] [PubMed]
- Futami K, Ogasawara S, Goto H, et al. RecQL1 DNA repair helicase: A potential tumor marker and therapeutic target against hepatocellular carcinoma. Int J Mol Med 2010;25:537-45. [PubMed]
- Mendoza-Maldonado R, Faoro V, Bajpai S, et al. The human RECQ1 helicase is highly expressed in glioblastoma and plays an important role in tumor cell proliferation. Mol Cancer 2011;10:83. [Crossref] [PubMed]
- Liu J, Lichtenberg T, Hoadley KA, et al. An Integrated TCGA Pan-Cancer Clinical Data Resource to Drive High-Quality Survival Outcome Analytics. Cell 2018;173:400-16.e11. [Crossref] [PubMed]
- Ceccarelli M, Barthel FP, Malta TM, et al. Molecular Profiling Reveals Biologically Discrete Subsets and Pathways of Progression in Diffuse Glioma. Cell 2016;164:550-63. [Crossref] [PubMed]
- Zhao Z, Zhang KN, Wang Q, et al. Chinese Glioma Genome Atlas (CGGA): A Comprehensive Resource with Functional Genomic Data from Chinese Glioma Patients. Genomics Proteomics Bioinformatics 2021;19:1-12. [Crossref] [PubMed]
- Gravendeel LA, Kouwenhoven MC, Gevaert O, et al. Intrinsic gene expression profiles of gliomas are a better predictor of survival than histology. Cancer Res 2009;69:9065-72. [Crossref] [PubMed]
- Gusev Y, Bhuvaneshwar K, Song L, et al. The REMBRANDT study, a large collection of genomic data from brain cancer patients. Sci Data 2018;5:180158. [Crossref] [PubMed]
- Sun L, Hui AM, Su Q, et al. Neuronal and glioma-derived stem cell factor induces angiogenesis within the brain. Cancer Cell 2006;9:287-300. [Crossref] [PubMed]
- Tang Z, Kang B, Li C, et al. GEPIA2: an enhanced web server for large-scale expression profiling and interactive analysis. Nucleic Acids Res 2019;47:W556-60. [Crossref] [PubMed]
- Subramanian A, Tamayo P, Mootha VK, et al. Gene set enrichment analysis: a knowledge-based approach for interpreting genome-wide expression profiles. Proc Natl Acad Sci U S A 2005;102:15545-50. [Crossref] [PubMed]
- Subramanian A, Kuehn H, Gould J, et al. GSEA-P: a desktop application for Gene Set Enrichment Analysis. Bioinformatics 2007;23:3251-3. [Crossref] [PubMed]
- Li T, Fan J, Wang B, et al. TIMER: A Web Server for Comprehensive Analysis of Tumor-Infiltrating Immune Cells. Cancer Res 2017;77:e108-10. [Crossref] [PubMed]
- Danaher P, Warren S, Dennis L, et al. Gene expression markers of Tumor Infiltrating Leukocytes. J Immunother Cancer 2017;5:18. [Crossref] [PubMed]
- Sousa S, Määttä J. The role of tumour-associated macrophages in bone metastasis. J Bone Oncol 2016;5:135-8. [Crossref] [PubMed]
- Wang SS, Liu W, Ly D, et al. Tumor-infiltrating B cells: their role and application in anti-tumor immunity in lung cancer. Cell Mol Immunol 2019;16:6-18. [Crossref] [PubMed]
- Hänzelmann S, Castelo R, Guinney J. GSVA: gene set variation analysis for microarray and RNA-seq data. BMC Bioinformatics 2013;14:7. [Crossref] [PubMed]
- Ru B, Wong CN, Tong Y, et al. TISIDB: an integrated repository portal for tumor-immune system interactions. Bioinformatics 2019;35:4200-2. [Crossref] [PubMed]
- Meng J, Lu X, Zhou Y, et al. Characterization of the prognostic values and response to immunotherapy/chemotherapy of Krüppel-like factors in prostate cancer. J Cell Mol Med 2020;24:5797-810. [Crossref] [PubMed]
- Jia D, Li S, Li D, et al. Mining TCGA database for genes of prognostic value in glioblastoma microenvironment. Aging (Albany NY) 2018;10:592-605. [Crossref] [PubMed]
- van den Bent MJ. Practice changing mature results of RTOG study 9802: another positive PCV trial makes adjuvant chemotherapy part of standard of care in low-grade glioma. Neuro Oncol 2014;16:1570-4. [Crossref] [PubMed]
- Keles GE, Lamborn KR, Berger MS. Low-grade hemispheric gliomas in adults: a critical review of extent of resection as a factor influencing outcome. J Neurosurg 2001;95:735-45. [Crossref] [PubMed]
- Li X, Padhan N, Sjöström EO, et al. VEGFR2 pY949 signalling regulates adherens junction integrity and metastatic spread. Nat Commun 2016;7:11017. [Crossref] [PubMed]
- Owen KL, Brockwell NK, Parker BS. JAK-STAT Signaling: A Double-Edged Sword of Immune Regulation and Cancer Progression. Cancers (Basel) 2019;11:2002. [Crossref] [PubMed]