Identification of an immune-related gene pair signature in breast cancer
Introduction
Breast cancer is the most common malignancy affecting women worldwide (1). In 2018, a new case was reported every 18 seconds, and 2.1 million women were diagnosed with breast cancer (2). The global incidence of breast cancer increases by 3.1% every year, from 641,000 cases in 1980 to 1.6 million in 2010 (3).
The precise mechanisms of how breast cancer emerges remain unclear (4); however, the oncogenesis and the development of breast cancer are closely related to immunity (5). The breast cancer microenvironment contains a large number of lymphocytes, macrophages, and bone marrow-derived stromal cells, and most of these cell types are involved in the immune response (6). In addition, the number of tumor-infiltrating lymphocytes reflects the strength of the immune response, which has a positive effect on the immune response and the prognosis of breast cancer patients after specific treatment (7). Previous studies reported that the immune microenvironment during the early stages of tumorigenesis mainly plays an anti-tumor role through the cytokines produced by activated CD8+ and CD4+ T cells (1). This suggests that the status of immunity can reflect the patient’s prognosis.
Regarding breast cancer, the presence and number of metastases axillary nodes is the most important prognostic marker (8). However, the extent of axillary nodes does not actually reflect prognosis, as Jennifer (9) reported that about 30% of untreated breast cancer patients without node metastasis developed metastasis/recurrent 10 years later. However, about 50% of patients with node involvement could be cured by local treatment. Tumor size and grade are the other two widely used clinical markers (10-12). Because of tumor heterogeneity, the same tumor size or grade does not share a common pathological outcome. Because personalized treatment is promoting, clinical prognostic markers (e.g., tumor size, tumor grade, and lymph node metastases) are not sufficient for the suitable management of early patient diagnosis. Biomarkers have become new tools in the early diagnosis of tumors. Several attempts have been made to construct prognostic models using gene expression data, and good prognostic efficacy has been observed in individual datasets (13-15). However, challenges still remain, such as the overfitting of the data and lack of sufficient validation. Currently, several different sequencing platforms are available, and the data obtained from these platforms by different strategies may yield batch effects and have a significant impact on the results (16,17). The key will be to find a way to make use of gene expression data while avoiding the influence of different gene testing methods. Therefore, in this study, a novel method based on relative gene expression is proposed to reduce the adverse effects introduced by the batch effect and data processing. This approach has been successfully used in the past for predicting the prognosis of several tumors, such as colorectal cancer and serous ovarian carcinoma (18-21). Specifically, in this study, immune-related gene pairs (IRGPs) were constructed to develop a prognostic signature for breast cancer. We present the following article in accordance with the TRIPOD checklist (available at https://tcr.amegroups.com/article/view/10.21037/tcr-21-2309/rc).
Methods
Public datasets
The whole analysis process is shown in Figure 1. The Cancer Genome Atlas (TCGA) is a cancer genomics program led by the National Cancer Institute and National Human Genome Research Institute, which contains the genomic data of 33 different cancer types (22). For this study, the RNA sequencing (RNAseq) Level 3 data and clinical information of the breast cancer (BRCA) project were directly downloaded from the TCGA. Due to lack of recording, not all clinical information of patients can be provided. Gene Expression Omnibus (GEO) is a public functional genomics data repository for array- and sequence-based data. Thus, normalized RNAseq or array data of breast cancer samples were retrieved from the GEO database. The search criteria on the GEO database were as follows: (I) data of breast cancer samples having a sample size of more than 50; (II) data on clinical information, especially the survival status and the last follow-up time; and (III) RNAseq data or array data from the HG-U133_Plus_2 or HG-U133A platform. The study was conducted in accordance with the Declaration of Helsinki (as revised in 2013). Since the data were de-identified and publicly available, no institutional review board approval was necessary and no informed consent was signed for this study. Regarding gene screening, the immunity dataset, which contains 2,498 immune genes of 17 immune processes, was downloaded from the ImmPort database (https://immport.niaid.nih.gov/home). Finally, two RNAseq datasets, such as the TCGA and GSE96058, were used as training set for the identification of the signature, and six microarray datasets (GSE7390, GSE124647, GSE42568, GSE20711, GSE48391, and GSE20685) were used as validation set for validating the signature. Patients who received chemotherapy or for which no clear survival information was available were excluded from the datasets. Overall, a total of 3,496 cases were analyzed.
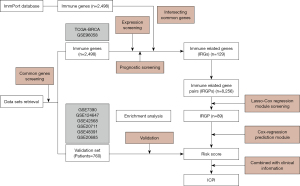
Data processing
In this study, to illustrate that the developed model is valid for different types of gene data, training and validation sets were generated according to the platforms. Regarding the sequencing datasets (TCGA and GSE96058), the normalized data of the fragments per kilobase of transcript per million mapped reads (FPKM) was downloaded from the corresponding database and used as training set. Regarding the microarray data derived from the Affymetrix company (including HG-U133_plus_2 or HG-U133A platforms) in the validation set, raw microarray data were downloaded. Then, the background was corrected and subjected to quantile normalization using the Robust Multichip Average (RMA) function of the package affy (v 1.50.0), using default parameters (23).
Screening of the immune-related prognostic genes
Genes were screened prior to the construction of gene pairs. First, the genes in the TCGA and GSE96058 datasets were screened separately. Genes with an average expression in the top 50% of each dataset were selected and considered sufficiently expressed genes. Genes with a mean absolute deviation (MAD) in the top 30% of the sufficiently expressed genes were selected and considered informatic genes of each dataset. Only informatic genes that were present in both TCGA and GSE96058 datasets, and the immune gene set were used, and were subjected to Cox survival regression analysis in the training set (including TCGA and GSE96058). In this study, overall survival (OS) was used as prognosis outcome. Genes that were significantly correlated (P<0.05) with OS were selected for the construction of gene pairs.
Construction and screening of IRGPs
Pairwise pairing was used to construct the IRGPs in the training set. An IRGP consisted of two genes. If the expression value of the first gene was lower than the expression value of the second gene, the value of this IRGP was considered to be 1. Otherwise, it was considered to be 0. IRGPs that were 1 or 0 in more than 90% of the samples in the training set were removed. The score of IRGPs was used to build the prognostic signature. The least absolute shrinkage and selection operator (Lasso)-Cox regression analysis were used to streamline IRGPs. In this study, 200 times of 10-fold cross-validation Lasso-Cox regression analysis was performed to select the parameter lambda in the Lasso-Cox regression model, thus determining the complexity of the model. For each running time, the lambda.min, which was the optimal lambda for the regression model, was extracted. The median lambda.min of the 200 times of 10-fold cross-validation was used in the final Lasso-Cox regression model. IRGPs with non-zero coefficients in the final Lasso-Cox regression model were taken as candidate IRGPs for constructing the prediction model.
Construction and validation of the prediction model based on the immune related gene pair index (IRGPI)
In this section, the risk scoring system is constructed. After the selection of gene pairs, the general Cox regression model was constructed based on the 89 candidate IRGPs in the training set. The risk scores of each sample in the training set and validation set were calculated based on the constructed Cox regression model. The optimal thresholds were selected using the survivalROC package (v 1.0.3) to stratify samples into high- and low-risk groups with risk score (24). The receiver operating characteristic (ROC) curve of the three-year survival in the training set was plotted, and the risk score at the point closest to the coordinate (0,1) on the curve was selected as optimal threshold. At this point, the highest predicted specificity and sensitivity could be achieved. Subsequently, the log-rank survival analysis was performed on the high- and low-risk group in both the training set and validation set.
Immune cell infiltration and gene ontology (GO) analysis
According to the RNAseq data, the infiltration of the immune cells in the samples from the TCGA and GSE96058 datasets was evaluated using the online CIBERSORT platform (https://cibersort.stanford.edu/) (11,25). The function of the IRGPs that were used to construct the Cox regression model was explored by GO enrichment analysis using the package clusterProfile (v 3.11) under default options (26), with q-value <0.05.
Construction and validation of a composite immune-clinical prognostic index (ICPI)
Univariate Cox regression analysis was performed for the risk score and other clinical characteristics [e.g., age, estrogen receptor (ER) status, human epidermal growth factor receptor 2 (HER2) status, node, and molecular subtype] to identify prognostic factors. Variables that were statistically significant in the univariate Cox regression analysis were then included in the multivariate Cox regression analysis. In the multivariate Cox regression analysis, adjustment analysis was performed to identify independent prognostic factors considering confounding effects. Then, the variables that were statistically significant in the multivariable Cox regression analysis were included in the final Cox regression model in the training set to further improve predictive ability. In the model, age was used as continuous variable and the HER2 status was used as binary variable, where a positive HER2 status was defined as 1 and a negative HER2 status as 0. The prognostic performance of the ICPI and risk score were evaluated in terms of the C-index.
Statistical analysis
Statistical analysis was performed using the R language (v 3.6.3) and associated packages. Lasso-Cox regression analysis was performed using the glmnet package (version 4.0). The optimal threshold for the risk score was calculated using the survivalROC package (version 1.0.3), and Cox regression analysis was performed using the survival package (version 3.1). GO enrichment analysis was performed using the clusterprofile package (version 3.11), and the C-index was calculated using the survcomp package (version 3.11) (27). P<0.05 was considered to indicate statistically significant differences.
Results
Patients stratification into high- and low-risk group using IRGPs based prediction model
In this study, a total of 2,736 patients were included in the training set and 760 patients were included in the validation set (Table 1, Table S1). A total of 129 immune-related genes (IRGs) were screened out according to gene expression and prognosis in the training set. Then, 8,256 gene pairs were constructed based on these IRGs. After removal of gene pairs with values of 1 or of 0 in more than 90% of samples, 5,144 gene pairs were used. The optimal lambda.min value in the Lasso-Cox regression model was 0.014, and 89 gene pairs were used under this lambda. Detailed information on the 89 gene pairs is provided in Table S2. The Cox regression model was constructed using these 89 gene pairs. The risk scores of all samples in the training set and validation set were calculated and were based on the 89 gene pairs of each sample using the constructed Cox regression model. The optimal threshold in the time-dependent ROC curve analysis for classifying the samples into high- and low-risk group was set to 0.81 (Figure 2).
Table 1
Characteristics | Training set | Meta-validation set (n=760) | P value | |
---|---|---|---|---|
TCGA (n=763) | GSE96058 (n=1,973) | |||
Age, years, median [range] | 58 [26–90] | 68 [34–96] | 52 [24–91] | <0.0001 |
ER status | <0.0001 | |||
Positive | 414 | 1,865 | 296 | |
Negative | 122 | 55 | 171 | |
HER2 status | <0.0001 | |||
Positive | 72 | 80 | 60 | |
Negative | 454 | 1,818 | 109 | |
PR status | <0.0001 | |||
Positive | 366 | 1,693 | 80 | |
Negative | 169 | 144 | 60 | |
Node status | 0.7317 | |||
Positive | 269 | 499 | 118 | |
Negative | 274 | 1,414 | 272 | |
Molecular subtype | <0.0001 | |||
Luminal A | 344 | 1,282 | 45 | |
Luminal B | 160 | 380 | 37 | |
HER2-enriched | 52 | 98 | 35 | |
Basal-like | 111 | 85 | 32 | |
Normal-like | 96 | 128 | 13 | |
Stage | NA | |||
I | 149 | – | – | |
II | 318 | – | – | |
III | 57 | – | – | |
IV | 16 | – | – |
ER, estrogen receptor; HER2, human epidermal growth factor receptor 2; NA, not available; PR, progesterone receptor; TCGA, The Cancer Genome Atlas.
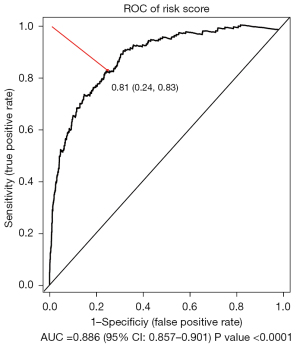
Risk score as an indicator of patients’ prognosis in breast cancer
Patients in the training and validation sets were divided into high- and low-risk groups. The high-risk patients in the training set had a significantly poorer OS prognosis compared to low-risk patients [hazard ratio (HR): 5.9, 95% confidence interval (CI): 4.61–7.54, P<0.0001]. The subgroup analysis of the ER status, HER2 status, node status, and molecular subtype demonstrated consistent results. The progesterone receptor (PR) status and tumor stage information were available in the TCGA datasets, and the subgroup analysis of the PR status and stage demonstrated significantly poorer OS in patients in the high-risk group than in patients in the low-risk group. The high-risk patients in the validation set had a significantly poorer OS compared to the low-risk patients (HR: 1.52, 95% CI: 1.16–1.98) (Figure 3). In addition, all subgroups in the subgroup analysis of the validation set, except for the basal-like group, exhibited poorer prognosis in the high- risk group compared to the low-risk group (HR >1) (Figure 3).
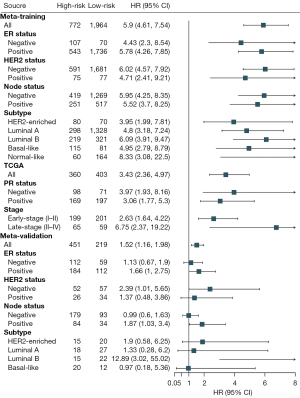
IRGs included in the prediction model as indicator of immune cell infiltration and immune processes
The infiltration of CD8+ T cells was significantly lower in the high-risk group in the training set (including TCGA and GSE96058) compared to the low-risk group (Figure 4, Figure S1). Furthermore, the enrichment analysis of 89 unique genes of the 86 gene pairs in the immune-related risk model demonstrated that these genes mainly played a role in cell proliferation, adhesion, activation, and other functions of immune cells (Figure 5).
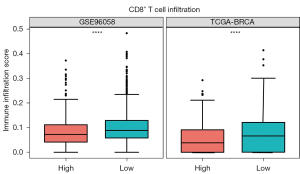
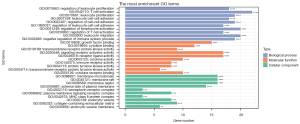
Integrated risk score with clinical characteristics to achieve higher predictive ability
Univariate Cox regression analysis showed that risk score, age, ER status, HER2 status, and node status were significantly correlated with clinical prognosis (P<0.05). The above-mentioned variables were analyzed using multivariable Cox regression analysis where only risk score, age, and HER2 status significantly correlated with OS (P<0.05; Table 2). The results indicated that the prognostic effect of the risk score was independent from other covariates, such as age and HER2 status. A novel prognostic index ICPI was constructed by combining age, HER2 status, and IRGPI in the Cox regression model. The new ICPI had a median c-index of 0.84 (range, 0.82–0.86), which was higher than that of the median risk score 0.82 (range, 0.72–0.84) alone in the training set. Next, a nomogram was generated as clinical reference, which included age, HER2 status, and risk score (Figure 6). The risk score of a patient was calculated based on the expression of the gene pairs and combined with the patient’s age and HER2 status to calculate a total score, from which both the 3- and 5-year survival rates could be predicted in the nomogram.
Table 2
Characteristics | Univariate regression analysis | Multivariate regression analysis | |||||
---|---|---|---|---|---|---|---|
Hazard ratio | 95% CI | P value | Hazard ratio | 95% CI | P value | ||
Age | 1.06 | 1.05–1.07 | <0.0001 | 1.05 | 1.03–1.06 | <0.0001 | |
ER status | 0.59 | 0.41–0.85 | 0.005 | 1.23 | 0.85–1.77 | 0.28 | |
HER2 status | 1.90 | 1.34–2.68 | <0.0001 | 1.49 | 1.05–2.14 | 0.027 | |
Node | 1.59 | 1.27–1.99 | <0.0001 | 1.18 | 0.97–1.56 | 0.095 | |
Risk score | 2.72 | 2.48–2.98 | <0.0001 | 2.56 | 2.27–2.81 | <0.0001 | |
Subtype | 1.09 | 0.97–1.23 | 0.146 | – | – | – |
CI, confidence interval; ER, estrogen receptor; HER2, human epidermal growth factor receptor 2.
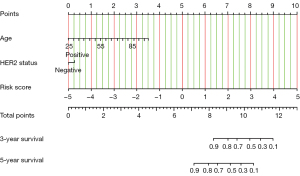
Discussion
Breast cancer is the most common malignancy affecting women worldwide (2). Breast cancer prognosis has been markedly improved because of the establishment of different molecular subtypes and the use of targeted drugs. However, the prognosis of tumors of different molecular subtype still varies significantly (28). Therefore, the establishment of a prognostic system, independent of molecular subtypes may help to better understand the disease and promote a more personalized treatment. The development of high-throughput sequencing technology presents new opportunities because the expression of tens of thousands of genes can provide high dimension information that may enable a better evaluation of the patients’ condition. Increasing attention has been focused on the role of immune processes in tumors, as they may reflect tumor prognosis and response to treatment in a certain extent.
In this study, IRGs were used to construct gene pairs, which in turn were used to construct a Cox regression model to predict patients’ prognoses. High-dimensional data of gene expression were used while reducing the impact of the batch effects from different sequencing platforms in the model. The results showed that patients in the high-risk group had significantly poorer prognosis when compared to patients in the low-risk group in all subgroups in the training set. Patients in the high-risk group in the meta-validation set had poorer prognosis compared to patients in the low-risk group in most of the subgroups (HR >1). Taken together, these results suggested that the risk score is an independent prognostic factor, which was confirmed by multivariate Cox regression analysis.
Immune cell infiltration analysis revealed a reduction in immune cell infiltration, especially in CD8+ T cells, in breast cancer patients in the high-risk group. CD8+ T cells participate in the adaptive immune response and are the main immune cells involved in immune surveillance (29). Once tumor cells are identified in the body, CD8+ T cells are activated by the T cell receptor (TCR) antigen recognition, and rapidly undergo proliferation and differentiation into cytotoxic T lymphocytes (CTLs) to destroy tumor cells through cell-cell contact (30). Previous studies showed that CD8+ T cells can be used as part of the immune score to better evaluate prognosis regardless of the patient’s tumor stage instead of the standard pathological criteria (31). This partially explains the poor prognosis of the high-risk group. Furthermore, GO enrichment analysis showed that the IRGs used in the prediction model primarily played a role in immune cell activation. These findings indicate that the risk score could in part reflect the immune activation state.
Next, the individual’s prognostic clinical factors, such as age and HER2 status, were combined with the risk score, and a higher prognosis prediction accuracy was obtained. Thus, the results suggest that clinical data, especially age and HER2 status, are still important prognostic indicators, that can be used to help correct predicted results.
This study has certain limitations. Currently, RNAseq and microarray are expensive techniques and a long time is needed to perform them. Therefore, performing these techniques in a standard clinical practice currently remains challenging. In addition, details regarding patient follow-up, which represent an important factor affecting prognosis, remain limited. Patients from different data sets showed significant differences in the baseline level, which also influenced the accuracy of the prediction model. Therefore, additional multi-center clinical studies are required to validate these results. The analysis of the immune cell infiltration is based on the training model CIBERSORT, and differences with the actual situation may be present.
In conclusion, an independent IRGP signature was constructed. Through pairwise comparison of a set of genes, the OS of patients could be predicted. This method avoids the impact of the batch effect caused by different sequencing platforms and has a promising application prospect.
Acknowledgments
We thank P Rosaria for professional editing service.
Funding: This work was supported by the Jilin Provincial Department of Finance (No. 2018SCZWSZX-035 to AS) and the Jilin Science and Technology Department (No. 20190701041GH to AS).
Footnote
Reporting Checklist: The authors have completed the TRIPOD reporting checklist. Available at https://tcr.amegroups.com/article/view/10.21037/tcr-21-2309/rc
Conflicts of Interest: All authors have completed the ICMJE uniform disclosure form (available at https://tcr.amegroups.com/article/view/10.21037/tcr-21-2309/coif). The authors have no conflicts of interest to declare.
Ethical Statement: The authors are accountable for all aspects of the work, ensuring that questions related to the accuracy or integrity of any part of the work are appropriately investigated and resolved. The study was conducted in accordance with the Declaration of Helsinki (as revised in 2013).
Open Access Statement: This is an Open Access article distributed in accordance with the Creative Commons Attribution-NonCommercial-NoDerivs 4.0 International License (CC BY-NC-ND 4.0), which permits the non-commercial replication and distribution of the article with the strict proviso that no changes or edits are made and the original work is properly cited (including links to both the formal publication through the relevant DOI and the license). See: https://creativecommons.org/licenses/by-nc-nd/4.0/.
References
- Boudreau A, van't Veer LJ, Bissell MJ. An "elite hacker": breast tumors exploit the normal microenvironment program to instruct their progression and biological diversity. Cell Adh Migr 2012;6:236-48. [Crossref] [PubMed]
- Harbeck N, Penault-Llorca F, Cortes J, et al. Breast cancer. Nat Rev Dis Primers 2019;5:66. [Crossref] [PubMed]
- Bray F, Ferlay J, Laversanne M, et al. Cancer Incidence in Five Continents: Inclusion criteria, highlights from Volume X and the global status of cancer registration. Int J Cancer 2015;137:2060-71. [Crossref] [PubMed]
- Landskron G, De la Fuente M, Thuwajit P, et al. Chronic inflammation and cytokines in the tumor microenvironment. J Immunol Res 2014;2014:149185. [Crossref] [PubMed]
- Byrne A, Savas P, Sant S, et al. Tissue-resident memory T cells in breast cancer control and immunotherapy responses. Nat Rev Clin Oncol 2020;17:341-8. [Crossref] [PubMed]
- Ruffell B, Au A, Rugo HS, et al. Leukocyte composition of human breast cancer. Proc Natl Acad Sci U S A 2012;109:2796-801. [Crossref] [PubMed]
- Dieci MV, Radosevic-Robin N, Fineberg S, et al. Update on tumor-infiltrating lymphocytes (TILs) in breast cancer, including recommendations to assess TILs in residual disease after neoadjuvant therapy and in carcinoma in situ: A report of the International Immuno-Oncology Biomarker Working Group on Breast Cancer. Semin Cancer Biol 2018;52:16-25. [Crossref] [PubMed]
- Barzaman K, Karami J, Zarei Z, et al. Breast cancer: Biology, biomarkers, and treatments. Int Immunopharmacol 2020;84:106535. [Crossref] [PubMed]
- Litton JK, Burstein HJ, Turner NC. Molecular Testing in Breast Cancer. Am Soc Clin Oncol Educ Book 2019;39:e1-7. [Crossref] [PubMed]
- Cianfrocca M, Goldstein LJ. Prognostic and predictive factors in early-stage breast cancer. Oncologist 2004;9:606-16. [Crossref] [PubMed]
- Carter CL, Allen C, Henson DE. Relation of tumor size, lymph node status, and survival in 24,740 breast cancer cases. Cancer 1989;63:181-7. [Crossref] [PubMed]
- Donegan WL. Tumor-related prognostic factors for breast cancer. CA Cancer J Clin 1997;47:28-51. [Crossref] [PubMed]
- Sparano JA, Gray RJ, Makower DF, et al. Prospective Validation of a 21-Gene Expression Assay in Breast Cancer. N Engl J Med 2015;373:2005-14. [Crossref] [PubMed]
- Cardoso F, van't Veer LJ, Bogaerts J, et al. 70-Gene Signature as an Aid to Treatment Decisions in Early-Stage Breast Cancer. N Engl J Med 2016;375:717-29. [Crossref] [PubMed]
- Foukakis T, Lövrot J, Matikas A, et al. Immune gene expression and response to chemotherapy in advanced breast cancer. Br J Cancer 2018;118:480-8. [Crossref] [PubMed]
- Yang L, Roberts D, Takhar M, et al. Development and Validation of a 28-gene Hypoxia-related Prognostic Signature for Localized Prostate Cancer. EBioMedicine 2018;31:182-9. [Crossref] [PubMed]
- Han LO, Li XY, Cao MM, et al. Development and validation of an individualized diagnostic signature in thyroid cancer. Cancer Med 2018;7:1135-40. [Crossref] [PubMed]
- Li B, Cui Y, Diehn M, et al. Development and Validation of an Individualized Immune Prognostic Signature in Early-Stage Nonsquamous Non-Small Cell Lung Cancer. JAMA Oncol 2017;3:1529-37. [Crossref] [PubMed]
- Peng PL, Zhou XY, Yi GD, et al. Identification of a novel gene pairs signature in the prognosis of gastric cancer. Cancer Med 2018;7:344-50. [Crossref] [PubMed]
- Wu J, Zhao Y, Zhang J, et al. Development and validation of an immune-related gene pairs signature in colorectal cancer. Oncoimmunology 2019;8:1596715. [Crossref] [PubMed]
- Zhang L, Zhu P, Tong Y, et al. An immune-related gene pairs signature predicts overall survival in serous ovarian carcinoma. Onco Targets Ther 2019;12:7005-14. [Crossref] [PubMed]
- Cancer Genome Atlas Research Network. The Cancer Genome Atlas Pan-Cancer analysis project. Nat Genet 2013;45:1113-20. [Crossref] [PubMed]
- Irizarry RA, Bolstad BM, Collin F, et al. Summaries of Affymetrix GeneChip probe level data. Nucleic Acids Res 2003;31:e15. [Crossref] [PubMed]
- Hanna YM, Baglan KL, Stromberg JS, et al. Acute and subacute toxicity associated with concurrent adjuvant radiation therapy and paclitaxel in primary breast cancer therapy. Breast J 2002;8:149-53. [Crossref] [PubMed]
- Newman AM, Liu CL, Green MR, et al. Robust enumeration of cell subsets from tissue expression profiles. Nat Methods 2015;12:453-7. [Crossref] [PubMed]
- Yu G, Wang LG, Han Y, et al. clusterProfiler: an R package for comparing biological themes among gene clusters. OMICS 2012;16:284-7. [Crossref] [PubMed]
- Schröder MS, Culhane AC, Quackenbush J, et al. survcomp: an R/Bioconductor package for performance assessment and comparison of survival models. Bioinformatics 2011;27:3206-8. [Crossref] [PubMed]
- Waks AG, Winer EP. Breast Cancer Treatment: A Review. JAMA 2019;321:288-300. [Crossref] [PubMed]
- Maimela NR, Liu S, Zhang Y. Fates of CD8+ T cells in Tumor Microenvironment. Comput Struct Biotechnol J 2018;17:1-13. [Crossref] [PubMed]
- Jiang X, Xu J, Liu M, et al. Adoptive CD8+ T cell therapy against cancer:Challenges and opportunities. Cancer Lett 2019;462:23-32. [Crossref] [PubMed]
- Church SE, Galon J. Regulation of CTL Infiltration Within the Tumor Microenvironment. Adv Exp Med Biol 2017;1036:33-49. [Crossref] [PubMed]