N6-methyladenosine methylation related immune biomarkers correlates with clinicopathological characteristics and prognosis in clear cell renal cell carcinoma
Introduction
Renal cell carcinoma (RCC) is one of the most common malignancies of the human urinary system that originates from renal tubular epithelial cells, accounting for more than 80% of primary renal malignancies (1). Clear cell renal cell carcinoma (ccRCC) is the most common and most malignant pathological type of RCC, accounting for approximately 70–80% of all RCC (2). Due to its insidious onset, about 30% of ccRCC is diagnosed in advanced stage with a 5-year survival rate of about 11.7%. The surgery is the conventional treatment for early stage ccRCC, however 10% to 20% of patients will relapse and metastasize after treatment (3). Therefore, prolonging the survival time of patients with advanced renal cancer has become the mainstream of current study.
N6-methyladenosine (m6A) methylation is regarded as the most common internal modification of RNA in eukaryotic cells that has been found to implicate various fundamental cellular functions, including pre-mRNA splicing, translation regulation, 3'-end and microRNA processing, and nuclear transport, indicating associations with diverse pathophysiologies (4,5). For example, METTL3, a m6A methyltransferase installing m6A modification on target RNAs, has been demonstrated to accelerate tumor formation and progression of bladder cancer in a m6A-dependent manner by promoting the maturation of pri-microRNA221/222 (6). Chen et al. identified the risk signature involving m6A methylation genes FTO, YTHDC1 and WTAP that taken part in the malignant progression of bladder cancer, and this risk signature showed independent prognostic value for patients with bladder cancer (7).
Additionally, it has been reported that m6A modification involves the regulation of the immune response and T cell homeostasis. For example, the m6A modification was found to initiate naïve T cells re-programming for proliferation and differentiation by inducing the mRNA degradation of suppressor of cytokine signaling genes (8). Su et al. suggested that inhibiting of m6A demethylase FTO could promote the sensitizing of leukemia cells T cell cytotoxicity, and prevent the immune evasion triggered by hypomethylation agent (9). However, the role of m6A modification related immune genes in ccRCC has not been investigated.
In this study, we identified several independent prognostic immunes genes that showed strong expression correlations with m6A modification genes (methyltransferases, demethylases, and effectors) based on the expression data and clinical phenotype of ccRCC in the Cancer Genome Atlas (TCGA) database. Then, a prognosis risk model was established on the basis of these genes, and the clinical value and predictive performance were further investigated. This study will provide potential therapeutic targets and prognostic markers for the treatment of ccRCC. We present the following article in accordance with the STARD reporting checklist (available at https://tcr.amegroups.com/article/view/10.21037/tcr-21-1953/rc).
Methods
Data acquisition and data preprocessing
The RNA-seq FPKM expression data and clinical phenotype data of 602 kidney renal clear cell carcinoma samples in TCGA database (TCGA-KIRC) were downloaded, of which 531 samples were tumor samples. For genes annotation, the Ensembl_ID were converted into Symbol_ID based on the reference genome hg38 (version 22) in GENCODE database (https://www.gencodegenes.org/). The mean value was selected when different Ensembl_ID mapping to the same Symbol_ID. Genes with expression value of 0 in more than 80% samples were removed. The analyses in this study were performed as the procedure in Figure S1. The study was conducted in accordance with the Declaration of Helsinki (as revised in 2013).
Identification of m6A-related immune genes
The expression data of 21 m6A-related genes (methyltransferases: METTL3, METTL14, METTL15, WTAP, VIRMA, RBM15, RBM15B, KIAA1429, ZC3H13; demethylases: FTO, ALKBH5; effectors: RBMX, YTHDC1, YTHDC2, IGF2BP1, IGF2BP2, IGF2BP3, YTHDF1, YTHDF2, YTHDF3, HNRNPA2B1, HNRNPC) were retrieved from the KIRC tumor samples. In addition, the immune-related genes list that recorded in the ImmPort were downloaded from InnateDB database (https://www.innatedb.ca/), then the expression data of these immune-related genes were also retrieved from the KIRC tumor samples. Then, correlation analysis was performed to calculate the Pearson correlation coefficient utilizing cor test in R. The m6A-related immune genes were identified by cut-off value as follows: absolute value of correlation coefficient >0.6 and P<0.001.
Prognostic independent m6A-related immune genes
After filtrating samples with survival time less than 30 days, there remained 518 samples. Samples were divided into high and low expression group on the basis of median expression value of each m6A-related immune gene, followed by survival analysis utilizing Kaplan-Meier (KM) curve and log-rank test, and genes with P<0.001 in survival analysis were selected. We randomly grouped patients into training-set (n=259) and valid-set (n=259) in a ratio of 5:5. In training-set, univariate Cox regression analysis was applied to explore genes which was associated with patient survival, and genes with log-rank P<0.001 were included in multivariate Cox regression analysis. Prognostic independent m6A-related immune genes were screened by log-rank P<0.001.
Prognostic risk model
Prognostic independent m6A-related immune genes (genes with log-rank P<0.001 in multivariate Cox regression analysis) were utilized to establish prognostic risk model with the following formula: Risk score = ∑ Coef genes ×Exp genes. The Coef in formula refers to the coefficient β in multivariate Cox regression, while Exp refers to the expression value of each gene. Risk score was calculated for all samples, and patients were categorized into different risk groups according to the median of risk score, followed by survival analysis. This analysis was carried out in training-set (n=259), valid-set (n=259) and the whole set (n=518).
Clinical factors and immune checkpoint genes in high and low risk group
Clinical factors, including pathologic T/N/M, tumor stage, age and gender were compared between high and low risk groups utilizing ggstatsplot (version: 0.5.0) in R package, and chi-square test was used to calculate the P value. Similarly, the difference on expression level of immune checkpoint genes were also explored, including PD1 (PDCD1), PD-L1 (CD274), CTLA-4, CD278 (ICOS), Tim3 (HAVCR2), LAG3, CD73, CD47, BTLA, TIGIT, myd1 (SIRPA), OX40 (TNFRSF4), 4-1BB (TNFRSF9) and B7-H4 (VTCN1).
Gene set variation analysis (GSVA)
In order to explore the differences on KEGG pathways between the high and low risk groups, GSVA was performed (10). In brief, on the basis of the enrichment background (c2.cp.kegg.v7.2.symbols.gmt) in MSigDB v7.2 database, the enrichment scores of KEGG pathways in all samples were calculated to obtain a scoring matrix using GSVA (version: 1.36.2) in R package. Then, differential analysis was conducted for these KEGG pathways in high vs. low-risk groups utilizing Limma in R package. The significant results were selected with P<0.05.
Evaluation of prognostic risk model
Univariate Cox regression analysis was carried out to screen factors (clinical factors and risk score) that impacted on survival of patients, in which factors with log-rank P<0.05 were included in multivariate Cox regression analysis. Factors with log-rank P<0.05 were considered to have independent prognostic value. Additionally, Normgram was utilized to evaluate the independent prognostic factors.
Statistical analysis
The correlations of immune genes with OS were evaluated based on univariate and multivariate Cox regression analysis in Survival package (version3.2-7). The Pearson correlation coefficient of m6A-related immune and m6A genes were calculated by the cor test in R (http://77.66.12.57/R-help/cor.test.html). The differences on clinical phenotype (including age, gender, TNM stage, and tumor grades) between high- and low-risk group were compared using chi-square test. Cox regression were performed to analyze the independent predictive value of the prognostic model with the P<0.05 as the statistical significance.
Results
Identification of prognostic independent m6A-related immune genes
After data preprocessing, we obtained expression data of 3,788 immune genes from TCGA dataset. Then, the correlation analysis was carried out on the expression level between 3,788 immune genes and the 21 m6A-related genes, and 856 co-expression pairs involving 458 immune genes and 19 m6A-related genes were obtained with the cut-off value of absolute value of correlation coefficient >0.6 and P<0.001.
Survival analysis was then performed for these 458 immune genes, and K-M curves showed that 195 immune genes were associated with survival of ccRCC patients. Univariate Cox regression analysis for these 195 immune genes revealed that 85 immune genes had significant impact on prognosis of ccRCC patients (Table S1). These 85 immune genes were then included in multivariate Cox regression analysis, and five genes were found to be independent prognosis immune genes, including PKHD1, IGF2BP3, RORA, FRK and MZF1 (Figure 1A, Table S2). Figure 1B showed the correlation heatmap of the five immune genes with m6A-related genes. It could be seen that MZF1 showed strong positive correlation with METTL3, while showed strong negative correlation with KIAA1429 and YTHDF3. PKHD1 was negatively correlated with IGF2BP3 and IGF2BP2. Therefore, these five genes were considered as prognostic independent m6A-related immune genes. Survival analysis indicated that the low expression of PKHD1, RORA and FRK were associated with poor survival for ccRCC patients. While patients with low expression of IGF2BP3 and MZF1 showed long survival time than patients with high expression level (Figure 2).
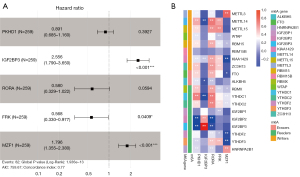
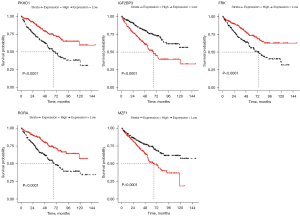
Prognosis risk model
Prognosis risk model based on these five genes was established, and risk score was calculated for all samples. Patients were categorized into different risk groups according to the median of risk score, followed by survival analysis. The K-M curve displayed that patients in high-risk group were associated with worse survival than patients in low-risk group in both training-set (n=259), valid-set (n=259) and the whole set (n=518) (Figure 3).
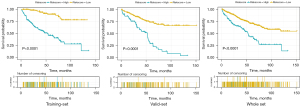
Clinical factors and immune checkpoint genes in high and low risk group
We further compared clinical factors between high-risk and low-risk groups. Except for age and gender, pathologic T, pathologic N, pathologic M and tumor stage were significant associated with risk score. High-risk group had a high proportion of patients in tumor stage III-IV and a high proportion of patients with invasiveness (pathologic T3 and T4), lymph node metastasis (N1) and distant metastasis (M1) (Figure 4). In addition, the expression of multiple immune checkpoint genes showed significant difference between high-risk and low-risk groups (Figure 5). For example, the expression of well-known immune checkpoint genes PD1 and CTLA-4 were significant higher in high-risk group.
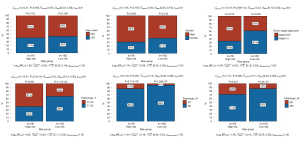
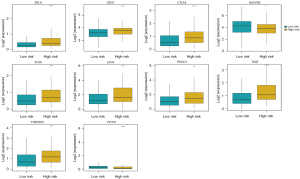
KEGG pathways in high and low risk group
GSVA analysis found that 11 KEGG pathways were different between high-risk and low-risk groups, such as cell cycle, P53 signaling pathway and various metabolism pathways, including histidine/butanoate/propanoate/ ascorbate and aldarate metabolism (Figure S2, Table 1).
Table 1
KEGG pathways | logFC | AveExpr | t | P value | adj.P value | B |
---|---|---|---|---|---|---|
KEGG_Cell cycle | 0.035095001 | 0.340633 | 4.613654 | 4.98E-06 | 0.00092606 | 3.244114 |
KEGG_P53 signaling pathway | 0.030019521 | 0.3599513 | 4.359866 | 1.57E-05 | 0.0014572 | 2.152439 |
KEGG_Histidine metabolism | 0.034572315 | 0.412188 | 3.812736 | 0.000154 | 0.00953346 | −0.00446 |
KEGG_Oocyte meiosis | 0.022081193 | 0.335807 | 3.530919 | 0.000451 | 0.01778489 | −1.00885 |
KEGG_Pathogenic escherichia coli infection | 0.028072745 | 0.3945073 | 3.51484 | 0.000478 | 0.01778489 | −1.06394 |
KEGG_Butanoate metabolism | 0.037942885 | 0.434312 | 3.378098 | 0.000784 | 0.02430781 | −1.52277 |
KEGG_Steroid hormone biosynthesis | 0.024499612 | 0.3601932 | 3.237615 | 0.001282 | 0.02993686 | −1.97599 |
KEGG_Ascorbate and aldarate metabolism | 0.038805757 | 0.4794297 | 3.236242 | 0.001288 | 0.02993686 | −1.98033 |
KEGG_Valine leucine and isoleucine degradation | 0.040939103 | 0.4557947 | 3.11005 | 0.001972 | 0.04075974 | −2.3715 |
KEGG_Drug metabolism cytochrome P450 | 0.024330769 | 0.369035 | 3.020577 | 0.002646 | 0.04921436 | −2.63977 |
KEGG_Propanoate metabolism | 0.036001688 | 0.4484454 | 2.991018 | 0.002911 | 0.04922557 | −2.72673 |
KEGG, Kyoto Encyclopedia of Genes and Genomes.
Evaluation of prognostic risk model
In univariate Cox regression analysis, pathologic T, pathologic N, pathologic M and risk group were associated with prognosis of ccRCC patients. These factors were included in multivariate Cox regression analysis, the results indicated that pathologic M (hazard ratio, HR =2.50, 95% CI: 1.53–4.08, P=0.0002), pathologic T (HR =2.18, 95% CI: 1.37–3.46, P=0.0010) and risk group (HR =1.69, 95% CI: 1.07–2.68, P=0.0246) were independent prognostic factors (Figure 6A, Table 2). These three independent prognostic factors were used to establish a nomogram, and it suggested that the nomogram could accurately predict the 1-, 2-, and 3-year survival probability for ccRCC patients (Figure 6B).
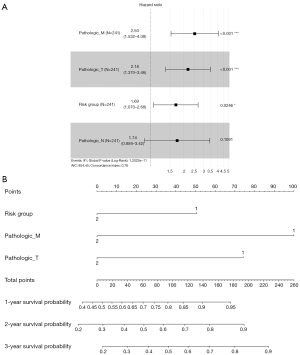
Table 2
Clinical characteristics | Univariable cox | Multi-variables cox | |||||||
---|---|---|---|---|---|---|---|---|---|
HR | Lower.95 | Upper.95 | P value | HR | Lower.95 | Upper.95 | P value | ||
Pathologic_M | 4.228869 | 2.731769 | 6.54643 | 9.97E-11 | 2.4997257 | 1.531582 | 4.079851 | 0.000247 | |
Pathologic_T | 3.165791 | 2.075339 | 4.829203 | 8.86E-08 | 2.176092 | 1.369936 | 3.456642 | 0.000991 | |
Risk Group | 2.523951 | 1.646848 | 3.868193 | 2.14E-05 | 1.6936021 | 1.069647 | 2.681527 | 0.024632 | |
Pathologic_N | 3.517107 | 1.865563 | 6.630729 | 0.000101 | 1.738996 | 0.88384 | 3.421553 | 0.109074 | |
Age | 0.756154 | 0.494296 | 1.156732 | 0.197509 | – | – | – | – | |
Gender | 1.040269 | 0.681729 | 1.587373 | 0.854721 | – | – | – | – |
HR, hazard ratio.
Discussion
m6A modification has also been reported to act crucial roles in mediating the diversity and complexity of tumor immune microenvironment (11,12). In this study, we identified five prognostic independent m6A-related immune genes (PKHD1, IGF2BP3, RORA, MZF1 and FRK) in ccRCC based on the expression data in TCGA. Low expression of PKHD1, RORA and FRK were associated with poor survival, while high expression of IGF2BP3 and MZF1 were associated with poor survival in ccRCC patients. Their expression showed correlations of survival time with various m6A methylation genes.
IGF2BP3 (insulin-like growth factor 2 mRNA-binding protein 3), a m6A reader, IGF2BP3 had been reported to drive malignancy progression of ccRCC by stabilizing CDKN2B-AS1 which in turn to activate the expression of Ndc80 kinetochore complex component 2 (13). Gu et al. suggested that IGF2BP3 could promote the G1/S transition and cell proliferation in ccRCC, and its high expression showed correlations with worse prognosis (14). RORA encodes RAR related orphan receptor A, and the inhibition of RORA has been found to contribute to tumor proliferation and metastasis in gastric cancer (15). MZF1 (myeloid zinc finger 1) is an oncogenic transcription factor, which plays important roles in driving cancer malignancy and epithelial-mesenchymal transition, has been considered as a regulator of tumor invasion and metastasis (16). FRK is a fyn related Src family tyrosine kinase. The inhibition of FRL was reported to implicated in promoting tumor proliferation and metastasis in RCC (17). Jing et al. indicated that FRK could repress cell proliferation in ccRCC by the phosphorylation of PTEN, and its expression was decreased in ccRCC and associated with worse outcomes (18). These findings were consistent with our results. Therefore, we suggested that these five genes were therapeutic targets and potential prognostic biomarkers in ccRCC.
Based on these five genes, a prognosis risk model was established, and the risk score could stratify ccRCC patients into high-risk and low risk group. Patients with high-risk were associated with short survival time than patients with low-risk. This further confirmed the prognosis value of the five genes-based risk model. Zhao et al. suggested that three-m6A related gene based prognostic risk model could precisely indicate the survival of patients in ccRCC (19). Additionally, in order to investigate the possible reasons of worse outcomes for patients with high-risk, the differences on clinical factors and immune checkpoint genes were compared between high-risk and low-risk group. The results suggested that high-risk group had a high proportion of patients in tumor stage III–IV and a high proportion of patients with invasiveness, lymph node metastasis and distant metastasis. In addition, ten immune checkpoint genes were found to show differential expression in high-risk and low-risk group, for example, PD1 and CTLA4 were highly expressed in high-risk in comparison with that of low-risk. The blockade of PD-1 had been proved to be a therapeutic target in the immunotherapy of ccRCC patients, suggesting the associations between its expression and prognosis (20,21). It had been demonstrated that CTLA4 inhibitors could lengthen the overall survival in various tumors. CTLA4 was found to be elevated in ccRCC and its high expression showed strong correlations with tumor progression and worse outcomes (22). Xiao et al. showed that high level of CTLA4 was associated with an obvious decreased survival time, pathologic stage and local recurrence, as well as negatively related to tumor purity (23). These results might explain why patients with high risk had poor survival.
Besides, our GSVA analysis displayed that cell cycle and P53 signaling pathway were differentially enriched by immune related genes in high-risk group comparable to low-risk group. Cell cycle was up regulated in high-risk group. Previous evidence showed that inhibition of cell cycle could trigger immune response in cancers (24,25). Hurvitz et al. found that perturbation of cell cycle in breast cancer cells by treatment of abemaciclib in combination with anastrozole, up-regulated immune response reflected by enhanced antigen presentation and T-cell activation (26). In addition, the role of p53 in immune response has been emergingly highlighted. P53 is a well-known tumor suppressor, which is always mutated or deleted in various cancers (27). Recent evidence has demonstrated that p53 elicits function in immune cells and impacts immune response by modulating recruitment and activity of myeloid and T cells (28). P53 elicited different effects on immune cells, resulting in tumor progression facilitation or inhibition (28). Our data also showed that the high-risk group was associated with poor prognosis compared with low-risk group, which might be explained by the differential activation of cell cycle and p53 signaling pathways by immune genes.
Conclusions
We identified five prognostic independent m6A-related immune genes (PKHD1, IGF2BP3, RORA, MZF1 and FRK) in ccRCC. The prognosis risk model-based on these five genes could predict survival for ccRCC patients. Patients with high-risk scores were associated with short survival time. Cell cycle and p53 signaling pathway were differentially enriched by immune related genes in high-risk group and low risk group, which impacted immune responses resulting in different outcomes in high risk and low risk group. The risk score was closely associated with tumor stage and pathologic T/N/M and immune checkpoint expression, and the risk score showed an independent prognostic value for patients with ccRCC.
Acknowledgments
Funding: The study was funded by
Footnote
Reporting Checklist: The authors have completed the STARD reporting checklist. Available at https://tcr.amegroups.com/article/view/10.21037/tcr-21-1953/rc
Data Sharing Statement: Available at https://tcr.amegroups.com/article/view/10.21037/tcr-21-1953/dss
Conflicts of Interest: All authors have completed the ICMJE uniform disclosure form (available at https://tcr.amegroups.com/article/view/10.21037/tcr-21-1953/coif). The authors have no conflicts of interest to declare.
Ethical Statement: The authors are accountable for all aspects of the work in ensuring that questions related to the accuracy or integrity of any part of the work are appropriately investigated and resolved. The study was conducted in accordance with the Declaration of Helsinki (as revised in 2013).
Open Access Statement: This is an Open Access article distributed in accordance with the Creative Commons Attribution-NonCommercial-NoDerivs 4.0 International License (CC BY-NC-ND 4.0), which permits the non-commercial replication and distribution of the article with the strict proviso that no changes or edits are made and the original work is properly cited (including links to both the formal publication through the relevant DOI and the license). See: https://creativecommons.org/licenses/by-nc-nd/4.0/.
References
- Escudier B, Porta C, Schmidinger M, et al. Renal cell carcinoma: ESMO Clinical Practice Guidelines for diagnosis, treatment and follow-up. Ann Oncol 2016;27:v58-68. [Crossref] [PubMed]
- Wolf MM, Kimryn Rathmell W, Beckermann KE. Modeling clear cell renal cell carcinoma and therapeutic implications. Oncogene 2020;39:3413-26. [Crossref] [PubMed]
- Zimpfer A, Glass Ä, Zettl H, et al. Renal cell carcinoma diagnosis and prognosis within the context of the WHO classification 2016. Urologe A 2019;58:1057-65. [Crossref] [PubMed]
- Sun T, Wu R, Ming L. The role of m6A RNA methylation in cancer. Biomed Pharmacother 2019;112:108613. [Crossref] [PubMed]
- Yang G, Sun Z, Zhang N. Reshaping the role of m6A modification in cancer transcriptome: a review. Cancer Cell Int 2020;20:353. [Crossref] [PubMed]
- Han J, Wang JZ, Yang X, et al. METTL3 promote tumor proliferation of bladder cancer by accelerating pri-miR221/222 maturation in m6A-dependent manner. Mol Cancer 2019;18:110. [Crossref] [PubMed]
- Chen M, Nie ZY, Wen XH, et al. m6A RNA methylation regulators can contribute to malignant progression and impact the prognosis of bladder cancer. Biosci Rep 2019;39:BSR20192892. [Crossref] [PubMed]
- Li HB, Tong J, Zhu S, et al. m6A mRNA methylation controls T cell homeostasis by targeting the IL-7/STAT5/SOCS pathways. Nature 2017;548:338-42. [Crossref] [PubMed]
- Su R, Dong L, Li Y, et al. Targeting FTO Suppresses Cancer Stem Cell Maintenance and Immune Evasion. Cancer Cell 2020;38:79-96.e11. [Crossref] [PubMed]
- Hänzelmann S, Castelo R, Guinney J. GSVA: gene set variation analysis for microarray and RNA-seq data. BMC Bioinformatics 2013;14:7. [Crossref] [PubMed]
- Zhang B, Wu Q, Li B, et al. m6A regulator-mediated methylation modification patterns and tumor microenvironment infiltration characterization in gastric cancer. Mol Cancer 2020;19:53. [Crossref] [PubMed]
- Xu S, Tang L, Dai G, et al. Expression of m6A Regulators Correlated With Immune Microenvironment Predicts Therapeutic Efficacy and Prognosis in Gliomas. Front Cell Dev Biol 2020;8:594112. [Crossref] [PubMed]
- Xie X, Lin J, Fan X, et al. LncRNA CDKN2B-AS1 stabilized by IGF2BP3 drives the malignancy of renal clear cell carcinoma through epigenetically activating NUF2 transcription. Cell Death Dis 2021;12:201. [Crossref] [PubMed]
- Gu Y, Niu S, Wang Y, et al. DMDRMR-Mediated Regulation of m6A-Modified CDK4 by m6A Reader IGF2BP3 Drives ccRCC Progression. Cancer Res 2021;81:923-34. [Crossref] [PubMed]
- Li J, Zou X. MiR-652 serves as a prognostic biomarker in gastric cancer and promotes tumor proliferation, migration, and invasion via targeting RORA. Cancer Biomark 2019;26:323-31. [Crossref] [PubMed]
- Brix DM, Bundgaard Clemmensen KK, Kallunki T. Zinc Finger Transcription Factor MZF1-A Specific Regulator of Cancer Invasion. Cells 2020;9:223. [Crossref] [PubMed]
- Yamada Y, Kimura N, Takayama KI, et al. TRIM44 promotes cell proliferation and migration by inhibiting FRK in renal cell carcinoma. Cancer Sci 2020;111:881-90. [Crossref] [PubMed]
- Jing ZF, Bi JB, Li ZL, et al. miR-19 promotes the proliferation of clear cell renal cell carcinoma by targeting the FRK-PTEN axis. Onco Targets Ther 2019;12:2713-27. [Crossref] [PubMed]
- Zhao Y, Tao Z, Chen X. Identification of a three-m6A related gene risk score model as a potential prognostic biomarker in clear cell renal cell carcinoma. PeerJ 2020;8:e8827. [Crossref] [PubMed]
- Hu J, Chen Z, Bao L, et al. Single-Cell Transcriptome Analysis Reveals Intratumoral Heterogeneity in ccRCC, which Results in Different Clinical Outcomes. Mol Ther 2020;28:1658-72. [Crossref] [PubMed]
- McKay RR, Bossé D, Xie W, et al. The Clinical Activity of PD-1/PD-L1 Inhibitors in Metastatic Non-Clear Cell Renal Cell Carcinoma. Cancer Immunol Res 2018;6:758-65. [Crossref] [PubMed]
- Liu S, Wang F, Tan W, et al. CTLA4 has a profound impact on the landscape of tumor-infiltrating lymphocytes with a high prognosis value in clear cell renal cell carcinoma (ccRCC). Cancer Cell Int 2020;20:519. [Crossref] [PubMed]
- Xiao GF, Yan X, Chen Z, et al. Identification of a Novel Immune-Related Prognostic Biomarker and Small-Molecule Drugs in Clear Cell Renal Cell Carcinoma (ccRCC) by a Merged Microarray-Acquired Dataset and TCGA Database. Front Genet 2020;11:810. [Crossref] [PubMed]
- Bao Z, Yang H, Hua J. Perturbation of cell cycle regulation triggers plant immune response via activation of disease resistance genes. Proc Natl Acad Sci U S A 2013;110:2407-12. [Crossref] [PubMed]
- Alvaro T, Lejeune M, García JF, et al. Tumor-infiltrated immune response correlates with alterations in the apoptotic and cell cycle pathways in Hodgkin and Reed-Sternberg cells. Clin Cancer Res 2008;14:685-91. [Crossref] [PubMed]
- Hurvitz SA, Martin M, Press MF, et al. Potent Cell-Cycle Inhibition and Upregulation of Immune Response with Abemaciclib and Anastrozole in neoMONARCH, Phase II Neoadjuvant Study in HR+/HER2- Breast Cancer. Clin Cancer Res 2020;26:566-80. [Crossref] [PubMed]
- Huang J. Current developments of targeting the p53 signaling pathway for cancer treatment. Pharmacol Ther 2021;220:107720. [Crossref] [PubMed]
- Blagih J, Buck MD, Vousden KH. p53, cancer and the immune response. J Cell Sci 2020;133:jcs237453. [Crossref] [PubMed]