Study on plasma amino acids and piperonamide as potential diagnostic biomarkers of non-small cell lung cancer
Introduction
Lung cancer is the leading cause of cancer death worldwide, with an estimated 1.79 million individuals dying of lung cancer each year. Among the histological subtypes of lung cancer, approximately 85% of patients are non-small cell lung cancer (NSCLC), which mainly include lung adenocarcinoma (LUAD) and lung squamous cell carcinoma (LUSC) (1-3). The high mortality associated with lung cancer is attributed in no small part to patients frequently having reached an advanced stage by diagnosis (4). Thus, accurate and early lung cancer diagnosis is critical if we wish to improve statistics on lung cancer survival. However, the commonly used serum biomarkers such as carcinoembryonic antigen (CEA) and cytokeratin 19 fragment (Cyfra21-1) are not ideal for early diagnosis or screening of cancer (5). In recent years, molecular biomarkers such as circulating tumor DNA (ctDNA) in the blood of patients with NSCLC have been extensively studied, but due to its low sensitivity and specificity, ctDNA is ineffective for cancer screening or early diagnosis (6,7). Therefore, the search for highly sensitive and specific NSCLC blood biomarkers with early diagnostic ability has become an urgent research requirement.
Metabolomics is a powerful tool with the potential to identify cancer biomarkers. It can systematically measure small molecule metabolites in blood to provide crucial information about cancer status (8). Abnormal metabolite accumulation is closely related to tumorigenesis (9). For instance, the growth of hepatocellular carcinoma depends on the accumulation of branched chain amino acids (10,11). Moreover, the growth of xenograft tumors in mice is affected by serine and glycine (12). This suggests that understanding changes in metabolites such as amino acids in plasma may have implications for cancer therapy and even diagnosis. Numerous studies have explored and demonstrated that amino acids detected by mass spectrometry, a common platform for metabolomics studies, can be used as biomarkers for screening and diagnosis of different tumors. Certain amino acids have exhibited high sensitivity and specificity (13-18). However, the value of plasma threonine, cysteine, and piperonamide (also known as piperine) as diagnostic biomarkers for NSCLC has been rarely explored (19). Moreover, Elevated blood glucose and weight loss in cancer patients may affect amino acid metabolism. Most previous metabolomics studies of cancer did not use samples containing these confounding factors as a validation set, so it was not sufficient to verify the diagnostic value of amino acids as biomarkers.
In addition to changes in metabolism or metabolites, another major requirement for cancer cell survival is changes in transcriptional programs. Transcription and metabolism are an inseparable “community”. Metabolic changes can affect gene expression in tumor cells, and the state of gene expression can also regulate metabolic remodeling (16,20). Thus, compared with the analysis based only on the level of metabolism, the combined analysis of metabolism and transcription may better explain the causes of metabolite changes. Several studies have demonstrated that the integration of metabolites and metabolic genes expands the findings of a single omics study (21-23). Unfortunately, the joint pathway analysis of transcriptome data and plasma amino acids has not been discussed in previous NSCLC studies.
Here, we used liquid chromatography-tandem mass spectrometry (LC-MS/MS) to analyze the metabolic changes of 22 amino acids and 1 piperonamide in the plasma of patients with NSCLC. In order to validate the reliability of the results, an independent verification set 1, a verification set 2 including confounding factors, and an integrated data set were used for verification. Also, we performed weighted gene co-expression network analysis (WGCNA) on gene expression data downloaded from The Cancer Genome Atlas (TCGA) database. In addition to the above-mentioned independent analysis, a combined pathway analysis of significantly changed metabolites and differentially expressed genes (DEGs) was carried out to increase awareness of changes in these metabolites. The aims and implications of this study were to identify diagnostic biomarkers to improve diagnosis and screening of NSCLC, explore the relationship between candidate metabolites and transcripts to better understand biological processes, and provide new insights into potential molecular mechanisms to help discover unique therapeutic vulnerabilities. We present the following article in accordance with the STARD reporting checklist (available at https://tcr.amegroups.com/article/view/10.21037/tcr-22-865/rc).
Methods
Participants and study design
Plasma samples from 167 NSCLC patients and 83 gender-matched HCs (χ2 test, P=0.273, no gender difference between 167 NSCLCs and 83 HCs) were retrospectively collected at the First Affiliated Hospital of Jinzhou Medical University. Dates of sample collection ranged from 2015 to 2021. This study was conducted in accordance with the Declaration of Helsinki (as revised in 2013). This study was approved by institutional ethics committee of The First Affiliated Hospital of Jinzhou Medical University (No. 202222) and informed consent was taken from all the patients. The inclusion criteria included the following: (I) pathological diagnosis of NSCLC; (II) patients aged 20 to 85 years. The exclusion criteria included the following: (I) patients who had undergone malignant tumor resection surgery, radiotherapy, chemotherapy, targeted therapy, and immunotherapy within 1 year before the study; (II) patients with other primary malignancies; (III) patients with recent severe vomiting or diarrhea; (IV) drug addicts, alcoholics, and pregnant women. This study included a training set (66 NSCLCs and 30 HCs), a validation set 1 (36 NSCLCs and 20 HCs), a validation set 2 (65 NSCLCs and 33 HCs), and an integrated data set (training set + validation set 1 + validation set 2). Patients in the training set, validation set 1, and validation set 2 were NSCLCs from 2015 to 2018, NSCLCs from 2019 to 2021, and NSCLCs with elevated fasting glucose and weight loss from 2015 to 2021, respectively. Healthy controls (HCs) were randomly selected in a ratio of approximately 1 to 2. According to the tumor-node-metastasis (TNM) staging standard in the 8th edition of the American Joint Committee on Cancer (AJCC), the NSCLC staging was determined. The study design of this research work is shown in Figure 1. The demographic and clinicopathological characteristics of study participants are presented in Table 1.
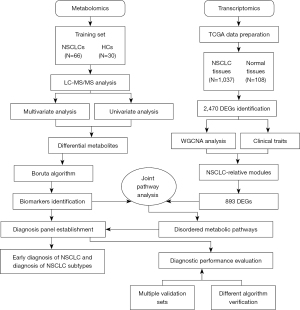
Table 1
Parameters | Training set | Validation set 1 | Validation set 2 | |||||
---|---|---|---|---|---|---|---|---|
NSCLC (n=66) | HC (n=30) | NSCLC (n=36) | HC (n=20) | NSCLC (n=65) | HC (n=33) | |||
Gender | ||||||||
Male | 42 | 20 | 23 | 15 | 40 | 23 | ||
Female | 24 | 10 | 13 | 5 | 25 | 10 | ||
Age (years) | ||||||||
Median | 60 | 39.5 | 60 | 40.5 | 61 | 34 | ||
Range | 37–82 | 25–58 | 41–77 | 23–56 | 33–77 | 25–59 | ||
TNM stage | ||||||||
I | 20 | 8 | 8 | |||||
II | 6 | 3 | 4 | |||||
III | 22 | 10 | 18 | |||||
IV | 18 | 15 | 35 | |||||
Histological type | ||||||||
LUAD | 44 | 23 | 43 | |||||
LUSC | 21 | 13 | 21 |
Since there is 1 lung adenosquamous carcinoma patient and 1 patient with unclear subtype in NSCLC patients, samples from these 2 patients were excluded from the subtype analysis. TNM, tumor-node-metastasis; LUAD, lung adenocarcinoma; LUSC, lung squamous cell carcinoma; NSCLC, non-small cell lung carcinoma; HC, healthy control.
Chemicals
High performance liquid chromatographic (HPLC) grade acetonitrile, pure water, and methanol were purchased from Thermo Fisher Scientific (Waltham, MA, USA). Acetyl chloride and 1-Butanol were acquired from Sigma-Aldrich (St. Louis, MO, USA). Internal standard kits were obtained from Cambridge Isotope Laboratories (Tewksbury, MA, USA). They contained 12 amino acid isotope-labeled internal standards. These standards were each dissolved in 1 mL of pure methanol respectively. These standards and methanol were thoroughly mixed and stored at 4 ℃. Working solutions were obtained by 100-fold dilution. The quality control (QC) standards were purchased from Chromsystems (Grafelfing, Germany).
Sample preparation
Fasting peripheral blood samples were collected from all participants in the early morning and stored in vacuum tubes containing heparin. Then, these samples were stored at 4 ℃ and sent to the laboratory for further processing within half an hour. Each piece of dried blood spots (DBS) paper was made into a disc with a diameter of 3 mm using a punch. The disc was placed into a 96-well plate for amino acids and piperonamide extraction. Then, 100 µL of fresh working solution was added to each well of this plate. The plate was centrifuged at 1,500 r/min for 2 minutes following gentle shaking at room temperature for 20 minutes. The new filtrate was collected in a new 96-well plate. Subsequently, 4 empty wells on each plate were randomly selected and 2 low and high concentration QC solutions were separately added. The filtrate and QC solution were dried at 50 ℃ via pure nitrogen. After adding 60 µL of acetyl chloride and 1-butanol mixture (10:90) to each well, the dried sample was incubated at 65 ℃ for 20 minutes to derivatize the metabolites. A second drying was performed on each sample following derivation, as described previously. Finally, the dried samples were fully dissolved in 100 µL of mobile phase solution for LC-MS/MS analysis.
LC-MS/MS analysis
For performing LC-MS/MS analysis, an AB SCIEX 4000 QTrap system (AB SCIEX LLC; Framingham, MA, USA), which was equipped with an electrospray ionization source, and operated under positive scan mode. The detailed parameters were set as described in our previous work (24-26). For each run, 20 µL of the sample was injected and 80% HPLC grade acetonitrile aqueous solution was used for the elution of gradient. The flow rate was initially set to 0.2 mL/min, and then dropped to 0.01 mL/min within 0.08 minutes and remained unchanged for 1.5 minutes. After 1.5 minutes, the flow rate returned to 0.2 mL/min within 0.01 minutes, and remained constant for 0.5 minutes. The pressure of ion source gas 1 and gas 2 was set to 35 psi. The auxiliary gas temperature was maintained at 350 ℃, the ion spray voltage was 4.5 kV, and curtain gas pressure was 20 psi (pounds per square inch).
Transcriptomics study
Data preparation and DEGs screening
The gene expression data were downloaded from TCGA database (https://cancergenome.nih.gov/). A total of 1,037 NSCLC tissues and 108 normal tissues gene expression data were included in this study. The “limma” R package (The R Foundation for Statistical Computing, Vienna, Austria) was applied to analyze the DEGs between NSCLC tissues and normal tissues. The DEGs were screened based on false discovery rate (FDR) <0.05 and |log2fold change (FC)| >2.
WGCNA
To explore the interactions between genes and between genes and clinical traits, WGCNA was performed on 2,470 DEGs. A gene co-expression network was constructed using R package “WGCNA”. Firstly, we performed sample clustering to check for outliers. Secondly, Pearson’s correlation analysis was used to calculate the correlations between genes. After that, we used network topology analysis to determine the optimal soft threshold that can enhance the strong correlations between genes and punish the weak correlations between genes. The expression matrix was converted to obtain a topological overlap matrix (TOM). After the minimum module size was set to 50, gene hierarchical clustering was performed to generate co-expression modules (27). At the same time, module eigengenes (MEs) in each module were also calculated. Finally, we evaluated the associations between ME and clinical traits to determine NSCLC-related modules for subsequent joint pathway analysis (28).
Statistical analysis
Statistical analysis was executed with the software SPSS 25.0 (IBM Corp., Armonk, NY, USA). Mann-Whitney U test was applied to analyze differences in age and metabolite levels between NSCLC and HC groups. Pearson’s chi-squared (χ2)test was used to analyze gender differences between NSCLC and HC groups. A two-tailed P value of <0.05 was considered statistically significant between the two groups. Multivariate analysis was performed on metabolic data by using MetaboAnalyst 5.0 (https://www.metaboanalyst.ca/) (29,30). According to the default on MetaboAnalyst 5.0, missing values will be replaced by 1/5 of minimum positive values of their corresponding variables. Moreover, to assess the predictive power of biomarkers, receiver operating characteristic (ROC) analyses were performed through R v.4.0.2 and GraphPad Prism 9 (GraphPad Software, San Diego, CA, USA).
Results
Participant characteristics
The participant characteristics of the training set, validation set 1, and validation set 2 are displayed in Table 1. Among them, validation set 2 included participants with elevated fasting blood glucose (6.18≤ blood glucose ≤12.89) at the time of mass spectrometry analysis and participants with weight loss (0< weight loss ≤10 kg) during the 6 months prior to pathological diagnosis. There was no difference in gender between the NSCLC and HC groups (χ2 test, P=0.774, 0.394 and 0.426 in the training set, validation set 1, and validation set 2, respectively).
The median age of the 167 NSCLC patients (61 years) was higher than that of the 83 HCs (38 years) (Mann-Whitney U test, P<0.05). To understand the effect of age on amino acid and piperonamide metabolism, NSCLC patients were grouped according to age (group 1 with median age 51, group 2 with median age 64), and the grouped data were analyzed by multivariate analysis using SIMCA 14.1 (Umetrics, Umeá, Sweden). No significant separation was detected between group 1 and group 2 (Figure S1). Therefore, in terms of our data, age had a low impact on metabolism of these metabolites.
Metabolomics data analysis
Discovery of differential metabolites in training set
The data in the training set were analyzed by MetaboAnalyst 5.0 for multivariate analysis. Sum normalization, cube root transformation, and auto scaling were applied for data normalization. To explore the disparities between the NSCLC and HC groups, principal component analysis (PCA) was first used to describe the clustering behavior of plasma amino acids and piperonamide between the two groups. As depicted in Figure 2A, the clear trend of separation manifested the existence of significant metabolic alterations. Then partial least squares-discriminant analysis (PLS-DA) further supported the different changes in plasma metabolites in the two groups (Figure 2B). Since PLS-DA is a supervised model, a 10-fold cross-validation (CV) was performed to assess whether the model overfitted. The Q2 value of 0.808 indicated that the PLS-DA model has good predictive performance on the training set (Figure 2C). The prediction reliability of the model was verified again by 1,000-times permutation test (P<0.001; Figure S2). After PLS-DA, variance in projection (VIP) analysis was applied to identify 15 important metabolites that contribute to classification (Figure 2D). Subsequently, a univariate analysis was used to determine whether these 15 metabolites changed significantly between the two groups (Table 2). Finally, 8 out of 15 metabolites were deemed differential metabolites based on the selection criteria of VIP >1, P<0.05, and FDR <0.05 (21). Compared with the HC group, the contents of threonine, piperonamide, arginine, alanine, and cysteine were significantly lowered in the NSCLC group. The other 3 metabolites including methionine, leucine, and histidine were significantly elevated in the NSCLC group.
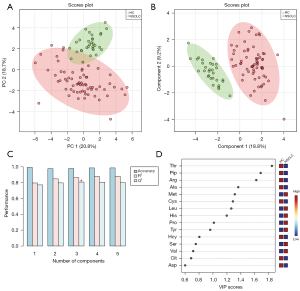
Table 2
Metabolite (μmol/L) | NSCLC, mean ± SD | HC, mean ± SD | P value | FDR |
---|---|---|---|---|
Threonine (Thr) | 26.30±7.64 | 49.10±10.42 | 7.98E-13 | 5.99E-12 |
Piperonamide (Pip) | 262.17±129.67 | 452.46±71.83 | 1.38E-10 | 5.16E-10 |
Arginine (Arg) | 12.92±8.60 | 30.05±9.53 | 4.10E-11 | 2.05E-10 |
Alanine (Ala) | 165.47±76.54 | 264.58±52.91 | 1.13E-09 | 3.38E-09 |
Methionine (Met) | 19.59±6.82 | 15.71±2.12 | 9.00E-05 | 1.93E-04 |
Cysteine (Cys) | 1.36±0.76 | 2.71±1.05 | 2.68E-08 | 6.71E-08 |
Leucine (Leu) | 127.02±41.60 | 108.86±26.58 | 0.024 | 0.03 |
Histidine (His) | 84.15±50.27 | 53.72±6.94 | 0.014 | 0.021 |
Proline (Pro) | 450.36±229.70 | 328.46±80.03 | 0.002 | 0.003 |
Tyrosine (Tyr) | 49.32±14.56 | 46.06±8.32 | 0.385 | 0.431 |
Homocysteine (Hcy) | 8.45±0.84 | 11.43±0.95 | 1.46E-14 | 2.19E-13 |
Serine (Ser) | 59.42±26.93 | 52.43±7.22 | 0.532 | 0.532 |
Valine (Val) | 138.66±36.87 | 141.07±28.10 | 0.402 | 0.431 |
Citrulline (Cit) | 15.24±13.44 | 20.56±3.77 | 0.018 | 0.025 |
Aspartic acid (Asp) | 38.16±15.07 | 51.16±19.79 | 4.23E-04 | 7.93E-04 |
NSCLC, non-small cell lung cancer; HC, healthy control; SD, standard deviation; FDR, false discovery rate.
Screening of potential diagnostic biomarkers for NSCLC
To screen for potential biomarkers, Boruta algorithm was used to select and shrink differential metabolites. The results showed that threonine, piperonamide, arginine, alanine, cysteine, methionine, and histidine were selected as potential diagnostic biomarkers for NSCLC in the training set (Figure S3). Then, ROC analyses were applied to evaluate the classification performance of these 7 metabolites for NSCLC patients and HCs. As shown in Figure 3, both boxplots and ROC curves analyzed by GraphPad prism 9 supported that each metabolite had a good diagnostic ability in the training set. Among these potential biomarkers, threonine displayed the highest accuracy in diagnosing NSCLC [area under the ROC curve (AUC) =0.958]. The results suggested that these 7 plasma differential metabolites had the potential to diagnose NSCLC.
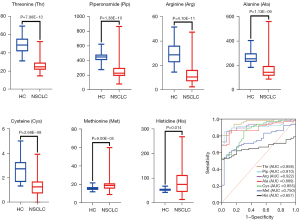
Identification of biomarkers for the diagnosis of NSCLC
To evaluate the accuracy of the above results, validation set 1, validation set 2, and integrated data set (training set + validation set 1 + validation set 2) were applied to verify the performance of these 7 potential biomarkers in the diagnosis of NSCLC. The results of Mann-Whitney U test and ROC analysis showed that the variation trend of threonine, piperonamide, arginine, alanine, and cysteine in the 3 validation sets was consistent with that in the training set, with statistical differences (Table 3). Although the AUC values of these 5 markers decreased compared with the training set, the overall diagnostic performance was still optimistic. Notably, methionine and histidine were not statistically significant in validation set 1 and validation set 2. The AUC values of these 2 potential biomarkers also declined in the 3 validation sets, but their P values and FDR values in the integrated data set were both lower than 0.05. We speculated that it might have been caused by the small sample size of validation set 1 and validation set 2. In a study by Klupczynska et al., the AUC values of methionine and histidine were 0.685 and 0.687, respectively, which were slightly higher than the calculation results in each of our validation sets (18). When these 2 biomarkers were incorporated into a diagnostic model containing 12 metabolites by Klupczynska et al., the AUC of this diagnostic model was 0.836, which was higher than the diagnostic performance of a single metabolite. Their research indicated that these 2 metabolites contributed to improving the model’s classification ability. Finally, these 2 metabolites were not excluded from the construction of our panel, and these 7 potential biomarkers were confirmed as NSCLC biomarkers.
Table 3
Data set (metabolites) | NSCLC, mean ± SD | HC, mean ± SD | P value | FDR | AUC (95% CI) | Sensitivity (95% CI) | Specificity (95% CI) | Cutoff |
---|---|---|---|---|---|---|---|---|
Validation set 1 | ||||||||
Threonine (Thr) | 33.49±16.40 | 53.17±12.32 | 1.10E-05 | 2.57E-05 | 0.857 (0.757– 0.957) | 0.750 (0.589–0.863) | 1.000 (0.839–1.000) | 37.27 |
Piperonamide (Pip) | 312.82±154.80 | 498.04±96.76 | 2.80E-05 | 4.90E-05 | 0.840 (0.738–0.943) | 0.667 (0.503–0.798) | 1.000 (0.839–1.000) | 365.40 |
Arginine (Arg) | 14.25±11.29 | 30.53±11.40 | 3.00E-06 | 2.10E-05 | 0.879 (0.788–0.971) | 0.750 (0.589–0.863) | 1.000 (0.839–1.000) | 17.40 |
Alanine (Ala) | 181.26±80.25 | 285.03±61.70 | 9.00E-06 | 2.57E-05 | 0.860 (0.759–0.961) | 0.861 (0.713–0.939) | 0.850 (0.640–0.948) | 237.70 |
Cysteine (Cys) | 1.46±0.88 | 2.34±1.45 | 0.023 | 0.032 | 0.684 (0.541–0.827) | 0.417 (0.271–0.578) | 0.950 (0.764–0.997) | 1.13 |
Methionine (Met) | 15.08±3.97 | 16.85±3.49 | 0.09 | 0.105 | 0.638 (0.491–0.784) | 0.528 (0.370–0.680) | 0.750 (0.531–0.888) | 14.56 |
Histidine (His) | 74.21±54.08 | 56.56±9.38 | 0.128 | 0.128 | 0.624 (0.475– 0.772) | 0.556 (0.396–0.705) | 0.900 (0.699–0.982) | 66.43 |
Validation set 2 | ||||||||
Threonine (Thr) | 35.15±51.64 | 47.65±9.42 | 1.40E-10 | 4.90E-10 | 0.898 (0.835–0.960) | 0.846 (0.739–0.914) | 0.909 (0.764–0.969) | 37.66 |
Piperonamide (Pip) | 294.12±166.65 | 406.32±66.42 | 1.59E-06 | 2.78E-06 | 0.798 (0.709–0.887) | 0.708 (0.588–0.804) | 1.000 (0.896–1.000) | 326.90 |
Arginine (Arg) | 12.01±8.27 | 27.70±6.23 | 2.17E-11 | 1.52E-10 | 0.915 (0.859–0.971) | 0.800 (0.687–0.879) | 0.970 (0.847–0.998) | 16.48 |
Alanine (Ala) | 187.04±77.01 | 289.27±53.24 | 9.83E-09 | 2.29E-08 | 0.857 (0.782–0.931) | 0.828 (0.718–0.901) | 0.849 (0.691–0.934) | 247.40 |
Cysteine (Cys) | 1.64±2.20 | 2.55±1.23 | 4.06E-06 | 5.68E-06 | 0.786 (0.697–0.874) | 0.492 (0.375–0.611) | 1.000 (0.896–1.000) | 1.04 |
Methionine (Met) | 19.05±8.29 | 16.37±2.66 | 0.155 | 0.155 | 0.588 (0.476–0.700) | 0.569 (0.448–0.682) | 0.667 (0.496–0.803) | 16.97 |
Histidine (His) | 87.73±55.51 | 65.94±17.74 | 0.056 | 0.065 | 0.619 (0.509–0.728) | 0.646 (0.525–0.751) | 0.667 (0.496–0.803) | 66.14 |
Integrated data set | ||||||||
Threonine (Thr) | 31.29±33.54 | 49.50±10.63 | 3.26E-26 | 2.28E-25 | 0.911 (0.876–0.947) | 0.844 (0.782–0.892) | 0.916 (0.836–0.959) | 37.48 |
Piperonamide (Pip) | 285.53±150.84 | 445.10±83.81 | 3.53E-19 | 6.18E-19 | 0.848 (0.800–0.896) | 0.713 (0.640–0.776) | 0.988 (0.935–0.999) | 327.10 |
Arginine (Arg) | 12.85±9.11 | 29.23±8.89 | 6.58E-26 | 2.30E-25 | 0.909 (0.873–0.945) | 0.844 (0.782–0.892) | 0.904 (0.821–0.950) | 20.34 |
Alanine (Ala) | 177.21±77.68 | 279.32±55.73 | 2.48E-21 | 5.79E-21 | 0.869 (0.825–0.913) | 0.765 (0.695–0.823) | 0.904 (0.821–0.950) | 212.30 |
Cysteine (Cys) | 1.49±1.51 | 2.56±1.22 | 1.86E-13 | 2.60E-13 | 0.786 (0.730–0.842) | 0.425 (0.353–0.501) | 0.988 (0.935–0.999) | 1.05 |
Methionine (Met) | 18.41±7.15 | 16.25±2.72 | 0.013 | 0.013 | 0.597 (0.527–0.666) | 0.455 (0.381–0.531) | 0.795 (0.696–0.868) | 18.05 |
Histidine (His) | 83.40±53.14 | 59.27±13.83 | 4.43E-04 | 5.17E-04 | 0.637 (0.569–0.704) | 0.539 (0.463–0.613) | 0.868 (0.778–0.924) | 70.15 |
AUC, sensitivity, and specificity were obtained by ROC analysis on GraphPad Prism 9. Specifically, the sensitivity, specificity and cutoff value are obtained according to the sensitivity, specificity and cutoff value corresponding to the maximum value of the sum of the sensitivity and specificity. ROC, receiver operating characteristic; NSCLC, non-small cell lung cancer; HC, healthy control; SD, standard deviation; FDR, false discovery rate; AUC, area under the ROC curve; CI, confidence interval.
Establishment and verification of NSCLC diagnostic panel
Due to the complexity of NSCLC metabolism, a diagnostic panel containing multiple biomarkers could more comprehensively reflect the pathological state of the disease. Therefore, a panel composed of threonine, piperonamide, arginine, alanine, cysteine, methionine, and histidine was established. Next, validations were performed using multiple validation sets including validation set 1, validation set 2, and integrated data set (training set + validation set 1 + validation set 2). Although the diagnostic ability of this panel in the training set was slightly higher than the other 3 validation sets, its overall diagnostic performance was worthy of recognition (Figure 4). The AUCs of the training set, validation set 1, validation set 2, and integrated data set reached 0.999, 0.965, 0.963, and 0.967, respectively (Table S1). Especially for validation set 2, which contained interference factors, the presence of interference factors had little effect on the detection of these 7 biomarkers, and the specificity was 0.970, similar to that in other data sets. In addition, the linear support vector machine (SVM) algorithm and the random forest algorithm were used on MetaboAnalyst 5.0 to prove the reliability of the above results again (Table S2). Thus, this diagnostic panel was regarded as reliable and would be used for subsequent exploration.
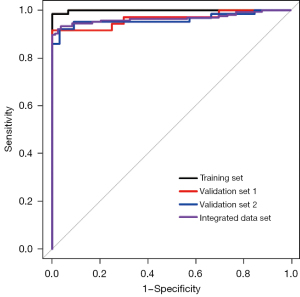
The diagnostic ability of this panel for early NSCLC and NSCLC subtypes
We use PLS-DA and ROC analysis to determine whether this panel had diagnostic value for early NSCLC. In the integrated data set, although this panel could not distinguish each stage well, it could distinguish NSCLC at each stage well from HCs (Figure 5A). Then, we conducted a separate ROC analysis on stage I NSCLC patients and HCs, and the results showed that the AUC, sensitivity, and specificity were 1.000, 1.000, and 0.988, respectively (Figure 5B). Thus, this panel showed promise for identifying patients with early NSCLC.
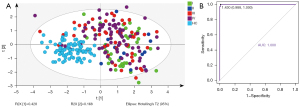
The panel’s diagnostic capabilities for NSCLC subtypes were also explored. As depicted in Figure S4, this panel had similar diagnostic performance for LUAD and LUSC with almost the same AUCs. Relative to the HC group, the AUCs of LUAD and LUSC groups were 0.972 and 0.971, respectively, within the integrated data set.
Correlation analysis of biomarkers in this panel with CEA and Cyfra21-1
The R package “correlation” was applied to explore the association of CEA and Cyfra21-1 with the 7 metabolic biomarkers we identified. As shown in Figure S5, the biomarkers in our panel were not significantly associated with clinically commonly used lung cancer markers CEA and Cyfra21-1.
Transcriptomics data analysis
DEGs screening
The gene expression profiles from TCGA data were imported into R v.4.0.2 to screen DEGs. A total of 17,938 genes were detected in the NSCLC group and the normal group. Then, a volcano map was drawn to show how each gene is distributed (Figure 6A). According to FDR <0.05 and |log2FC| >2, 2,470 of these genes were screened as DEGs, of which 1,810 genes were up-regulated and 660 were downregulated in NSCLC samples.
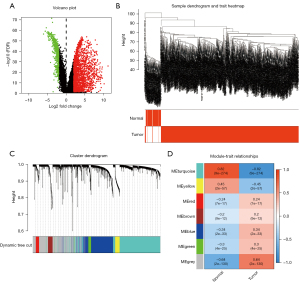
Module formation and its correlations with clinical traits
We performed WGCNA analysis on the selected 2,470 DEGs. As shown in Figure 6B, no outliers were found after clustering the samples. The soft threshold was chosen to be 6, which complied with the scale-free network rules (Figure S6A). After converting to TOM, we got 7 modules through gene clustering (Figure 6C). Next, to determine the genes related to the occurrence of NSCLC, we calculated the ME. It was found that the module most relevant to the occurrence of NSCLC was the turquoise module, which covered a total of 893 dysregulated genes (Table S3, Figure 6D). Figure S6B shows the correlation between each gene in the module and clinical features (the correlation coefficient =0.82; P<1e-200). Therefore, the turquoise module was thought to be the NSCLC-related module and would be used in the next analysis.
Joint pathway enrichment analysis and critical pathway extraction
In order to explore how metabolic biomarkers and genes interact, a joint pathway enrichment analysis was performed through MetaboAnalyst 5.0. The 893 DEGs in the turquoise module and the 7 biomarkers in the diagnostic panel were input to this network analysis platform. As depicted in Figure S7, a total of 5 metabolism pathways related to metabolic genes were significantly altered in the NSCLC samples (P<0.05). These strikingly disturbed metabolic pathways included glycine, serine and threonine metabolism, aminoacyl-tRNA biosynthesis, nitrogen metabolism, cysteine and methionine metabolism, and thiamine metabolism. When we explored these disordered metabolic processes through Kyoto Encyclopedia of Genes and Genomes (KEGG) analysis, several important metabolic pathways were found to be regulated by 4 DEGs (PSAT1, SHMT2, AOC3, and MAOB). Then, the pathways were extracted and displayed in Figure 7. Figure S8 shows the significant differences in expression of these 4 genes between NSCLC and HCs (all P<0.0001).
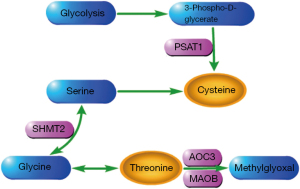
Discussion
In this study, LC-MS/MS technology was used to determine the potential of amino acids and piperonamide as diagnostic biomarkers for NSCLC. The transcriptional data were mined to increase our knowledge of these metabolite changes. Our findings indicated that NSCLC patients exhibited different plasma levels of threonine, piperonamide, arginine, alanine, cysteine, methionine, and histidine as compared with HCs (Figure 3 and Table 3). As can be seen from Figures 4,5, the panel composed of these 7 metabolites showed a promising diagnostic ability for NSCLC patients at various stages, including the early stage. The results of Tables S1,S2 showed that elevated fasting blood glucose (6.18≤ blood glucose ≤12.89) and weight loss (0< weight loss ≤10 kg) in NSCLC patients will not cause much interference in the detection of 7 biomarkers. We also revealed that these biomarkers do not differ much between subtypes, since the panel composed of these biomarkers had similar ability to diagnose NSCLC subtypes (Figure S4). The combined pathway enrichment analysis of 7 biomarkers and 893 DEGs revealed disruptions in glycine, serine, and threonine metabolism, aminoacyl-tRNA biosynthesis, nitrogen metabolism, cysteine and methionine metabolism, and thiamine metabolism (Figure S7). Finally, we found that 4 DEGs (PSAT1, SHMT2, AOC3, and MAOB) were involved in the metabolism of threonine and cysteine (Figure 7).
Threonine, as an essential amino acid, is an important biologically active molecule that has a significant impact on regulation of immune function (31-34). In vitro, lymphocytes can use threonine for their own proliferation and antibody secretion (32). In addition to the proliferation of lymphocytes and the secretion of antibodies, threonine is also related to the regulation of the expression of inflammatory cytokines. When fish are infected with bacteria, threonine deficiency will promote the expression of pro-inflammatory cytokine genes and inhibit the expression of anti-inflammatory cytokine genes to aggravate the inflammatory response (33,34). In NSCLC patients, we observed the decrease in plasma threonine concentration (Figure 3 and Table 3). A study of plasma amino acids in lung cancer patients also revealed significant changes in threonine (35). In this plasma metabolome study involving 142 Korean participants, threonine was decreased in cancer patients when compared to the control group, similar to our findings. Therefore, NSCLC cells may heavily consume threonine to establish an “immune comfort zone”. Since metabolism and transcription were inextricably linked (16,20), genes changed when threonine was lowered. As shown in Figure 7 and Figure S8, the accumulation of threonine may be caused by the up-regulation of SHMT2 and down-regulation of AOC3 and MAOB. However, the accumulation of threonine may be insignificant and does not significantly increase plasma threonine levels in NSCLC patients. Immune function will not be significantly improved.
Cysteine, a sulfur-containing amino acid, is produced by another sulfur-containing amino acid, methionine. Cysteine plays an important role in the process of cancer metabolism remodeling. It serves as a substrate for the production of hydrogen sulfide (H2S), which provides energy for the mitochondrial electron transport chain to stimulate the bioenergetics of cancer cells (36). In our study, reduced level of cysteine was indicated as a plasma metabolic signature of NSCLC patients (Figure 3 and Table 3). This abnormality of cysteine was also found in the serum of non-smoking female NSCLC patients (37). High concentrations of cysteine in the blood have been associated with a reduced risk of gastrointestinal cancers, as reported by Miller et al. and Murphy et al. (38,39). Thus, it can be assumed that the drop in blood cysteine levels is a common feature of many types of cancer, not just NSCLC. The downregulation of cysteine in plasma may be related to the production of H2S. In order to continuously utilize H2S, PSAT1 may be upregulated by cancer cells to partially replenish cysteine depletion (Figure 7 and Figure S8).
Arginine is an amino acid with anti-tumor potential that deserves special attention; arginine was down-regulated in the plasma samples of our NSCLC patients. Inconsistent with our results, serum arginine was elevated in patients with early NSCLC in the study by Klupczynska et al. (40). Although we performed a univariate analysis of early-stage NSCLC patients, arginine levels remained inconsistent. This difference may be due to different research methods. However, Kim et al. observed consistent results with reduced plasma arginine levels in lung cancer patients (35). This phenomenon of blood arginine drop in patients with lung cancer may be mainly attributed to increased uptake and utilization of circulating arginine by cancer cells and enhanced decomposition of intracellular arginine by cancer cells. This seems contradictory, but it may be because cancer cells want to meet both energy requirements and escape immune attack. Autophagy helps feed the nutrients needed for the survival of cancer cells (41). Blood is the source of nutrients for tumor cells. Poillet-Perez et al. reported that autophagy maintains the growth of tumor through arginine in the blood (42). Increasing intracellular arginine levels not only improved T cells survival capacity but also enhanced their anti-tumor activity in vivo (43). However, tumor cells suppress anti-tumor immunity by catabolizing intracellular arginine (44). The metabolism of arginine in NSCLC is complicated, and it is still necessary to study its value as a biomarker and anti-tumor medicine.
Another amino acid that was significantly reduced in the plasma of NSCLC patients was alanine, as previously reported by different authors (35,40,45). The decrease of serum alanine concentration in breast cancer patients was reported by Eniu et al. (46). A study of pancreatic duct adenocarcinoma (PDAC) found that PDAC cells use the SLC38A2 transporter to supplement the nutrient alanine (47). The low availability of alanine in different tumor types can be explained by the consumption of alanine for cancer cell proliferation. The other 2 controversial amino acids are histidine and methionine, both of which were increased in our NSCLC samples. However, these 2 amino acids were decreased in the cancer group in other studies (18,35,46,48). The reason for this difference may be the small sample size of the cancer group in our study or the different research methods.
Piperonamide, also known as piperine, has been shown to inhibit the proliferation and migration of cancer cells (19,49). In our study, piperonamide level was significantly lower in patients with NSCLC compared to HCs. This may be due to excessive degradation or reduced synthesis of piperonamide. Therefore, abnormal piperonamide metabolism can be involved in the pathogenesis of NSCLC.
After validation on multiple validation sets, we finally confirmed that these 7 metabolites have value as biomarkers for diagnosing NSCLC. The diagnostic panel composed of these 7 metabolic biomarkers had a good ability to classify NSCLC and HC, and also had the ability of screening or early diagnosis. Also, elevated fasting blood glucose and weight loss interfered little with its predictions. Finally, the integrated pathway analysis of metabolomics and transcriptomics provided more information for our understanding of changes in certain metabolic biomarkers. However, several limitations existed in the current research. First, the sample size in this study was small, especially for patients with stage I NSCLC. Second, there was no information on blood glucose and weight changes in HCs, but the design of comparisons between multiple groups of NSCLCs and HCs could compensate for the lack of information. There was also no information on collection time for HC samples. Third, the metabolism and transcription data were from different populations. Fourth, the sample types of metabolomics and transcriptomics analysis were different: the former was plasma and the latter was tissue. These may have biased the analysis, so solving the above limitations is the goal of our future research.
Conclusions
In summary, plasma threonine, piperonamide, arginine, alanine, cysteine, methionine, and histidine have the potential to serve as diagnostic biomarkers for NSCLC. The panel constructed from them performed satisfactorily in differentiating NSCLC patients from HCs. These findings may help improve NSCLC diagnosis and screening. Furthermore, the supplement of transcriptomic data improved our understanding of the changes in several of these metabolites. This has implications for studying carcinogenesis mechanisms and discovering possible therapeutic opportunities.
Acknowledgments
Funding: This work was supported by
Footnote
Reporting Checklist: The authors have completed the STARD reporting checklist. Available at https://tcr.amegroups.com/article/view/10.21037/tcr-22-865/rc
Data Sharing Statement: Available at https://tcr.amegroups.com/article/view/10.21037/tcr-22-865/dss
Conflicts of Interest: All authors have completed the ICMJE uniform disclosure form (available at https://tcr.amegroups.com/article/view/10.21037/tcr-22-865/coif). The authors have no conflicts of interest to declare.
Ethical Statement: The authors are accountable for all aspects of the work in ensuring that questions related to the accuracy or integrity of any part of the work are appropriately investigated and resolved. This study was conducted in accordance with the Declaration of Helsinki (as revised in 2013). This study was approved by institutional ethics committee of The First Affiliated Hospital of Jinzhou Medical University (No. 202222) and informed consent was taken from all the patients.
Open Access Statement: This is an Open Access article distributed in accordance with the Creative Commons Attribution-NonCommercial-NoDerivs 4.0 International License (CC BY-NC-ND 4.0), which permits the non-commercial replication and distribution of the article with the strict proviso that no changes or edits are made and the original work is properly cited (including links to both the formal publication through the relevant DOI and the license). See: https://creativecommons.org/licenses/by-nc-nd/4.0/.
References
- Bray F, Ferlay J, Soerjomataram I, et al. Global cancer statistics 2018: GLOBOCAN estimates of incidence and mortality worldwide for 36 cancers in 185 countries. CA Cancer J Clin 2018;68:394-424. [Crossref] [PubMed]
- Thai AA, Solomon BJ, Sequist LV, et al. Lung cancer. Lancet 2021;398:535-54. [Crossref] [PubMed]
- Herbst RS, Morgensztern D, Boshoff C. The biology and management of non-small cell lung cancer. Nature 2018;553:446-54. [Crossref] [PubMed]
- Nooreldeen R, Bach H. Current and Future Development in Lung Cancer Diagnosis. Int J Mol Sci 2021;22:8661. [Crossref] [PubMed]
- Sun N, Sun S, Gao Y, et al. Utility of isocitrate dehydrogenase 1 as a serum protein biomarker for the early detection of non-small-cell lung cancer: A multicenter in vitro diagnostic clinical trial. Cancer Sci 2020;111:1739-49. [Crossref] [PubMed]
- Fiala C, Diamandis EP. Utility of circulating tumor DNA in cancer diagnostics with emphasis on early detection. BMC Med 2018;16:166. [Crossref] [PubMed]
- Kalinke L, Thakrar R, Janes SM. The promises and challenges of early non-small cell lung cancer detection: patient perceptions, low-dose CT screening, bronchoscopy and biomarkers. Mol Oncol 2021;15:2544-64. [Crossref] [PubMed]
- Schmidt DR, Patel R, Kirsch DG, et al. Metabolomics in cancer research and emerging applications in clinical oncology. CA Cancer J Clin 2021;71:333-58. [Crossref] [PubMed]
- Martínez-Reyes I, Chandel NS. Cancer metabolism: looking forward. Nat Rev Cancer 2021;21:669-80. [Crossref] [PubMed]
- Ericksen RE, Lim SL, McDonnell E, et al. Loss of BCAA Catabolism during Carcinogenesis Enhances mTORC1 Activity and Promotes Tumor Development and Progression. Cell Metab 2019;29:1151-65.e6. [Crossref] [PubMed]
- Sivanand S, Vander Heiden MG. Emerging Roles for Branched-Chain Amino Acid Metabolism in Cancer. Cancer Cell 2020;37:147-56. [Crossref] [PubMed]
- Muthusamy T, Cordes T, Handzlik MK, et al. Serine restriction alters sphingolipid diversity to constrain tumour growth. Nature 2020;586:790-5. [Crossref] [PubMed]
- Yang XH, Jing Y, Wang S, et al. Integrated Non-targeted and Targeted Metabolomics Uncovers Amino Acid Markers of Oral Squamous Cell Carcinoma. Front Oncol 2020;10:426. [Crossref] [PubMed]
- Plewa S, Horała A, Dereziński P, et al. Usefulness of Amino Acid Profiling in Ovarian Cancer Screening with Special Emphasis on Their Role in Cancerogenesis. Int J Mol Sci 2017;18:2727. [Crossref] [PubMed]
- Qi SA, Wu Q, Chen Z, et al. High-resolution metabolomic biomarkers for lung cancer diagnosis and prognosis. Sci Rep 2021;11:11805. [Crossref] [PubMed]
- Loras A, Suárez-Cabrera C, Martínez-Bisbal MC, et al. Integrative Metabolomic and Transcriptomic Analysis for the Study of Bladder Cancer. Cancers (Basel) 2019;11:686. [Crossref] [PubMed]
- Zhang J, Wen X, Li Y, et al. Diagnostic approach to thyroid cancer based on amino acid metabolomics in saliva by ultra-performance liquid chromatography with high resolution mass spectrometry. Talanta 2021;235:122729. [Crossref] [PubMed]
- Klupczynska A, Dereziński P, Garrett TJ, et al. Study of early stage non-small-cell lung cancer using Orbitrap-based global serum metabolomics. J Cancer Res Clin Oncol 2017;143:649-59. [Crossref] [PubMed]
- Manayi A, Nabavi SM, Setzer WN, et al. Piperine as a Potential Anti-cancer Agent: A Review on Preclinical Studies. Curr Med Chem 2018;25:4918-28. [Crossref] [PubMed]
- Martín-Martín N, Carracedo A, Torrano V. Metabolism and Transcription in Cancer: Merging Two Classic Tales. Front Cell Dev Biol 2018;5:119. [Crossref] [PubMed]
- Wang W, He Z, Kong Y, et al. GC-MS-based metabolomics reveals new biomarkers to assist the differentiation of prostate cancer and benign prostatic hyperplasia. Clin Chim Acta 2021;519:10-7. [Crossref] [PubMed]
- Kelly RS, Chawes BL, Blighe K, et al. An Integrative Transcriptomic and Metabolomic Study of Lung Function in Children With Asthma. Chest 2018;154:335-48. [Crossref] [PubMed]
- Hassan MA, Al-Sakkaf K, Shait Mohammed MR, et al. Integration of Transcriptome and Metabolome Provides Unique Insights to Pathways Associated With Obese Breast Cancer Patients. Front Oncol 2020;10:804. [Crossref] [PubMed]
- Wang Q, Sun T, Cao Y, et al. A dried blood spot mass spectrometry metabolomic approach for rapid breast cancer detection. Onco Targets Ther 2016;9:1389-98. [PubMed]
- Jing F, Hu X, Cao Y, et al. Discriminating gastric cancer and gastric ulcer using human plasma amino acid metabolic profile. IUBMB Life 2018;70:553-62. [Crossref] [PubMed]
- Jing Y, Wu X, Gao P, et al. Rapid differentiating colorectal cancer and colorectal polyp using dried blood spot mass spectrometry metabolomic approach. IUBMB Life 2017;69:347-54. [Crossref] [PubMed]
- Wang Y, Chen L, Ju L, et al. Novel Biomarkers Associated With Progression and Prognosis of Bladder Cancer Identified by Co-expression Analysis. Front Oncol 2019;9:1030. [Crossref] [PubMed]
- Liu L, Zhao J, Chen Y, et al. Metabolomics strategy assisted by transcriptomics analysis to identify biomarkers associated with schizophrenia. Anal Chim Acta 2020;1140:18-29. [Crossref] [PubMed]
- Chong J, Wishart DS, Xia J. Using MetaboAnalyst 4.0 for Comprehensive and Integrative Metabolomics Data Analysis. Curr Protoc Bioinformatics 2019;68:e86. [Crossref] [PubMed]
- Zhang L, Zheng J, Ahmed R, et al. A High-Performing Plasma Metabolite Panel for Early-Stage Lung Cancer Detection. Cancers (Basel) 2020;12:622. [Crossref] [PubMed]
- Bröer S. Amino Acid Transporters as Targets for Cancer Therapy: Why, Where, When, and How. Int J Mol Sci 2020;21:6156. [Crossref] [PubMed]
- Zhang Q, Chen X, Eicher SD, et al. Effect of threonine deficiency on intestinal integrity and immune response to feed withdrawal combined with coccidial vaccine challenge in broiler chicks. Br J Nutr 2016;116:2030-43. [Crossref] [PubMed]
- Dong YW, Jiang WD, Liu Y, et al. Threonine deficiency decreased intestinal immunity and aggravated inflammation associated with NF-κB and target of rapamycin signalling pathways in juvenile grass carp (Ctenopharyngodon idella) after infection with Aeromonas hydrophila. Br J Nutr 2017;118:92-108. [Crossref] [PubMed]
- Habte-Tsion HM, Ge X, Liu B, et al. A deficiency or an excess of dietary threonine level affects weight gain, enzyme activity, immune response and immune-related gene expression in juvenile blunt snout bream (Megalobrama amblycephala). Fish Shellfish Immunol 2015;42:439-46. [Crossref] [PubMed]
- Kim HJ, Jang SH, Ryu JS, et al. The performance of a novel amino acid multivariate index for detecting lung cancer: A case control study in Korea. Lung Cancer 2015;90:522-7. [Crossref] [PubMed]
- Bonifácio VDB, Pereira SA, Serpa J, et al. Cysteine metabolic circuitries: druggable targets in cancer. Br J Cancer 2021;124:862-79. [Crossref] [PubMed]
- Mu Y, Zhou Y, Wang Y, et al. Serum Metabolomics Study of Nonsmoking Female Patients with Non-Small Cell Lung Cancer Using Gas Chromatography-Mass Spectrometry. J Proteome Res 2019;18:2175-84. [Crossref] [PubMed]
- Miller JW, Beresford SA, Neuhouser ML, et al. Homocysteine, cysteine, and risk of incident colorectal cancer in the Women's Health Initiative observational cohort. Am J Clin Nutr 2013;97:827-34. [Crossref] [PubMed]
- Murphy G, Fan JH, Mark SD, et al. Prospective study of serum cysteine levels and oesophageal and gastric cancers in China. Gut 2011;60:618-23. [Crossref] [PubMed]
- Klupczynska A, Dereziński P, Dyszkiewicz W, et al. Evaluation of serum amino acid profiles' utility in non-small cell lung cancer detection in Polish population. Lung Cancer 2016;100:71-6. [Crossref] [PubMed]
- Strohecker AM, Guo JY, Karsli-Uzunbas G, et al. Autophagy sustains mitochondrial glutamine metabolism and growth of BrafV600E-driven lung tumors. Cancer Discov 2013;3:1272-85. [Crossref] [PubMed]
- Poillet-Perez L, Xie X, Zhan L, et al. Autophagy maintains tumour growth through circulating arginine. Nature 2018;563:569-73. [Crossref] [PubMed]
- Geiger R, Rieckmann JC, Wolf T, et al. L-Arginine Modulates T Cell Metabolism and Enhances Survival and Anti-tumor Activity. Cell 2016;167:829-42.e13. [Crossref] [PubMed]
- Lemos H, Huang L, Prendergast GC, et al. Immune control by amino acid catabolism during tumorigenesis and therapy. Nat Rev Cancer 2019;19:162-75. [Crossref] [PubMed]
- Sarlinova M, Baranovicova E, Skalicanova M, et al. Metabolomic profiling of blood plasma of patients with lung cancer and malignant tumors with metastasis in the lungs showed similar features and promising statistical discrimination against controls. Neoplasma 2021;68:852-60. [Crossref] [PubMed]
- Eniu DT, Romanciuc F, Moraru C, et al. The decrease of some serum free amino acids can predict breast cancer diagnosis and progression. Scand J Clin Lab Invest 2019;79:17-24. [Crossref] [PubMed]
- Parker SJ, Amendola CR, Hollinshead KER, et al. Selective Alanine Transporter Utilization Creates a Targetable Metabolic Niche in Pancreatic Cancer. Cancer Discov 2020;10:1018-37. [Crossref] [PubMed]
- Shingyoji M, Iizasa T, Higashiyama M, et al. The significance and robustness of a plasma free amino acid (PFAA) profile-based multiplex function for detecting lung cancer. BMC Cancer 2013;13:77. [Crossref] [PubMed]
- de Almeida GC, Oliveira LFS, Predes D, et al. Piperine suppresses the Wnt/β-catenin pathway and has anti-cancer effects on colorectal cancer cells. Sci Rep 2020;10:11681. [Crossref] [PubMed]
(English Language Editor: J. Jones)