Analysis of the key prognostic genes and potential traditional Chinese medicine therapeutic targets in glioblastoma based on bioinformatics and network pharmacology methods
Introduction
Glioblastoma (GBM) is the most frequent and aggressive malignant tumor of the adult central nervous system (1), with high rates of incidence and recurrence. The median survival is less than 15 months (2), and the 5-year overall survival rate is less than 5% (3,4). At present, the clinical treatment for GBM is still surgical resection, supplemented by radiation and chemotherapy. Temozolomide has the advantages of high blood-brain barrier permeability and fewer adverse reactions, and has become the most commonly employed drug clinically, either alone or in combination with radiotherapy; however, it still faces the problems of drug resistance and genotype insensitivity (non-methylation of O6-methylguanine-DNA methyltransferase, MGMT) (5). Therefore, identifying the key genes affecting the prognosis of GBM and analyzing the potential targeted therapeutic compounds for the treatment of GBM is crucial.
Based on tumor microarray or sequencing data, tumor bioinformatics analysis was conducted to screen differentially-expressed genes (DEGs), providing a new target reference for molecular mechanism research and prognostic evaluation of cancer. Washah et al. (6) analyzed the principle and prospects of bioinformatics tools and databases in detail and concluded that they offer significant advantages and have broad prospects in the treatment of cancer. Relevant predictions indicate that CCNB1, CCNA2, COL1A1, and COL1A2 (extracted by bioinformatics screening) play a key role in malignant progression and the prognosis of hepatocellular carcinoma and colon cancer (7,8).
Different from other studies on potential target genes of glioblastoma, through network pharmacology, we tried to find a traditional Chinese medicine component aiming at the above target genes, which is of great value for targeted therapy of glioblastoma. Network pharmacology, as a novel research and analytical method, has been gradually explored and improved. Researchers can obtain data on diseases, target genes, and target proteins via the Network Information Database, and identify the intervention mechanism of drugs against diseases using tool software. Furthermore, the active ingredients of traditional Chinese medicines as well as their interaction with target genes can also be deduced based on the “disease-gene-target-drug” (9) interaction network, which is helpful in clarifying the mechanisms of chemical drugs acting on the human body. This can also provide new directions for the development of novel drugs that act on highly effective targets, which has become a particular focus of research (10,11). For the treatment of tumors, traditional Chinese medicine schemes have the advantages of low risk, high safety, and low price, and have been widely verified both theoretically and experimentally. It has been found that saffron, ginseng, and other traditional Chinese medicines have anti-tumor effects (12,13). The present study regarding the relationship between traditional Chinese medicine and GBM will assist in guiding the development of new drugs and improving the prognosis of patients.
The present study aimed to explore new methods that improve the therapeutic effect of GBM and establish a theoretical foundation for subsequent research. We employed bioinformatics and network pharmacology to screen reliable biomarkers of GBM and explore potential targeted therapeutic compounds with anti-cancer effects. Multiple analytical tools were applied to strengthen the reliability of our data. We present the following article in accordance with the STREGA reporting checklist (available at https://tcr.amegroups.com/article/view/10.21037/tcr-22-1122/rc).
Methods
Data resources
Three groups of GBM-related clinical data and gene expression profiles (GSE104291, GSE29796, and GSE35493, respectively) were obtained from the Gene Expression Omnibus (GEO) database (https://www.ncbi.nlm.nih.gov/geo/). Based on the chip-standardized data, the online analysis software GEO2R was used to divide into cancer tissue and normal brain tissue according to the tissue source to set the control. The DEGs between GBM and normal tissues were screened and volcano maps were obtained using GEO2R. The study was conducted in accordance with the Declaration of Helsinki (as revised in 2013).
Data processing
The criteria for genes with significant expression differences were set and the screening conditions were as follows: |logFC| ≥2 and the corrected P value (adj.P.Val) was less than 0.01. The DEGs obtained using the above screening method were intersected with the expression spectrum data of the three genes by utilizing custom Venn diagrams to obtain the common DEGs.
Building the protein-protein interaction (PPI) network
The STRING database search tool (https://string-db.org) can be used to build a PPI network to map possible interactions of DEGs, and download STRING tabular text. We imported different genes into the STRING database, set multiple proteins, selected the Homo sapiens organism, and hid the disconnected nodes in the network. To draw the PPI network diagram, the minimum required interaction score was set to >0.4 and the rest were set according to the default settings.
Screening hub genes
Cytoscape is an important visual network-building tool that was used to build the interaction network we required. We imported the STRING interactions into Cytoscape 3.8.2 software and obtained the network topology parameters (such as connectivity) using the cytoHubba plug-in to analyze and screen important nodes and interactions that were significantly associated with other DEGS and confirmed as hub genes.
Gene Ontology (GO) and Kyoto Encyclopedia of Genes and Genomes (KEGG) enrichment analysis of DEGs
GO is an important bioinformatics tool. GO enrichment analysis includes biological pathways (BP), cellular components (CC), and molecular functions (MF). KEGG is a database resource. KEGG analysis provides the annotation information of gene signal transduction and disease pathways, which provides the basis for the study of gene functions and pathways. GO and KEGG pathway enrichment analyses of the DEGs were performed using the DAVID bioinformatics resource (https://david.ncifcrf.gov/tools.jsp). False discovery rate (FDR) <0.01 and count ≥10 were used as the screening thresholds to make the functions and pathways statistically significant, and the enrichment dot bubble function in the bioinformatics analysis (http://www.bioinformatics.com.cn) was used to visualize GO and KEGG.
Hub genes survival curve
For the Gene Expression Profiling Interactive Analysis 2 (GEPIA 2, http://gepia2.cancer-pku.cn/#index) database developed by Peking University (using data from The Cancer Genome Atlas (TCGA) and The Genotype-Tissue Expression (GTEx) projects), the Survival Analysis function module was selected. We set the dataset as glioblastoma (GBM) + low-grade glioma (LGG), and the rest were set as the default setting to draw the Kaplan-Meier survival curves of GBM hub genes, including overall survival and disease-free survival.
Differential expression of hub genes in normal tissues and tumors
The Box Plot in GEPIA 2 was used to determine the differential expression of hub genes in normal tissues and glioma tissues of different grades. Tumor Immune Estimation Resource (TIMER) 2.0 is an interactive web service database that comprehensively evaluates and visualizes tumor-infiltrating immune cells and uses this to draw the immune-infiltration map of the hub genes.
Acquisition of active ingredients of traditional Chinese medicine
The Traditional Chinese Medicine Systems Pharmacology (TCMSP) Database and Analysis Platform is an important tool for retrieving the active components of traditional Chinese medicines and their target genes. To study the targets of prescription drug ingredients, the chemical components of four traditional Chinese medicines, Zanthoxylum nitidum, rhizoma belamcandae, black bean, and suberect spatholobus stem were obtained and analyzed using the TCMSP database according to the related ingredients of GBM. We screened the attribute value of absorption, distribution, metabolism and excretion (ADME) based on the criteria that oral bioavailability (OB) ≥30% and drug-likeness (DL) ≥0.18 to obtain the active compounds and their gene targets. The Uniprot protein database (https://www.uniprot.org) is composed of The European Institute of Bioinformatics (EMBL-EBI), the Swiss Institute of Bioinformatics (SIB), and the Protein Information Resource (PIR). It is the most abundant and ideal protein database of traditional Chinese medicine information resources at present and facilitates the matching of gene names corresponding to protein targets to standardize target genes.
Construction of an interaction network between traditional Chinese medicine components and DEGs of GBM
The intersection of drug target genes and DEGs was obtained using the custom Venn diagram tool (http://bioinformatics.psb.ugent.be/webtools/Venn/). This study analyzed the intersection genes using the STRING database search tool, setting the biological species as Homo sapiens, and constructed the PPI network by setting the maximum number of interaction units in the first layer to no more than 20, the second layer to no more than 50, and the rest as the default setting (14). Cytoscape 3.8.2 software was utilized to construct the network diagram between active components and target genes, and the cytoHubba plug-in was employed to obtain the network topology parameters (such as the degree of connectivity) to screen the active components of traditional Chinese medicine that were significantly associated with most target genes.
Molecular docking verification
The three-dimensional structures of target proteins and active ingredients of traditional Chinese medicine were downloaded from the Protein Data Bank (PDB https://www.rcsb.org/)and ZINC (https://zinc.docking.org/) databases, respectively. The former was used as the receptor, while the latter was used as the ligand to identify the molecular interaction and predict the stable binding conformation of the molecule and protein, so as to evaluate the binding strength and activity of the active compounds.
Statistical analysis
The web tool GEO2R is based on R software version 3.2.3 and the “limma”, “GEOquery” and “Biobase” packages. The FC in log2FC is fold change, which represents the ratio of the expression levels between normal samples and glioblastoma samples, and the logarithm to the base 2 is log2FC. FDR stands for False Discovery Rate, which is obtained by correcting the P value of the significance of the difference. Based on R (version 4.1.3), the GEPIA 2 uses the log-rank test, sometimes called the Mantel-Cox test, for the hypothesis evaluation. Unpaired Student’s t-tests were used to evaluate the differences. Generally, by default, the absolute value of log2FC is greater than 1 as the screening criterion for differential genes. P or FDR <0.05 was considered statistically significant.
Results
Acquisition of target gene sets and screening of DEGs
In this study, 1,459 DEGs (839 up-regulated genes and 620 down-regulated genes), 2,854 DEGs (2,639 up-regulated genes and 215 down-regulated genes), and 3,818 DEGs (1,606 up-regulated genes and 2,212 down-regulated genes) were identified from the GSE104291, GSE29796, and GSE35493 datasets respectively by using the GEO database. A total of 271 common DEGs (212 up-regulated genes and 59 down-regulated genes) were obtained through the Venn diagram software. The volcano maps and the Venn maps of the DEGs from the GSE104291, GSE29796, and GSE35493 datasets are shown in Figure 1. The up-regulated and down-regulated genes were marked with respective colors; red represented the up-regulated genes and green denoted the down-regulated genes, as shown in Figure 2.
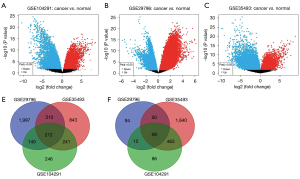
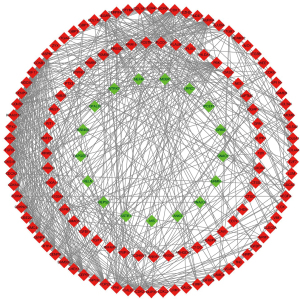
Building the PPI network
The PPI network of 271 DEGs was constructed using the STRING database, and the result is displayed in Figure 3. We imported the STRING tabular text into Cytoscape 3.8.2 software to extract the network diagram consisting of 138 nodes representing the common DEGs as well as 594 proteins interactions.
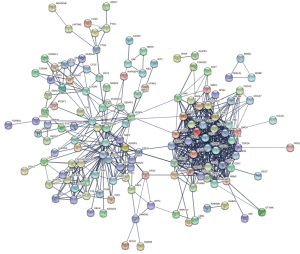
Screening hub genes
We calculated the degree of correlation, and 10 key hub genes were screened using the cytoHubba plug-in. According to the degree scores, the 10 key hub genes were ranked as follows: AURKA, BIRC5, CCNB1, CCNB2, CDK1, CENPF, FN1, KIF11, NDC80, and UBE2C. The heatmap of the hub genes is shown in Figure 4. The Cor heatmap plot of the hub genes is displayed in Figure 5.
GO and KEGG enrichment analysis and visualization
The GO and KEGG enrichment analyses of DEGs using the DAVID functional annotation tool were visualized through the enrichment dot bubble function. As shown in Figure 6, the X-axis indicated the proportion of genes, the Y-axis represented the name of the pathway, the size of the bubbles denoted the number of genes, and the color of the bubbles signified the P value. We respectively visualized the BP of the GO enrichment analysis, the CC, the MF enrichment projects, and the KEGG pathway projects. As illustrated in Figure 6, these DEGs were mostly enriched in the extracellular matrix tissues BP. In CC, they were mainly concentrated in the extracellular matrix, and in MF, they were primarily involved in the intercellular adhesion concentration in the binding of cadherin. The KEGG enrichment results showed that the p53 signaling pathway and adhesion plaques were mostly involved.
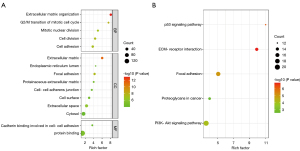
Survival analysis
Using the GEPIA2 website’s survival analysis function, the survival curves (both overall survival and disease-free survival) of hub genes in GBM were drawn to intuitively illustrate the prognosis of GBM. We found that the log-rank P values of the overall survival and disease-free survival curves of the 10 hub genes were all less than 0.001, indicating statistical significance. This suggested that the hub genes were correlated with the prognosis of GBM, as shown in Figures 7 and 8.
Differential expression of the hub genes in normal and GBM tissues
The box plots of the differentially-expressed hub genes in normal and GBM tissues of different levels are shown in Figure 9 (from A to J: AURKA, BIRC5, CCNB1, CCNB2, CDK1, CENPF, FN1, KIF11, NDC80, and UBE2C. The results showed that the 10 hub genes were lowly expressed in normal tissues, and the expression in GBM was positively correlated with grade. The immune infiltration maps of the hub genes are shown in Figure 10. The red boxes indicated a significant positive correlation, the blue boxes indicated a significant negative correlation, and the gray boxes indicated no correlation. The results showed that the expression values of the 10 hub genes were positively correlated with the infiltration level of B cells, and the P values were all less than 0.01.
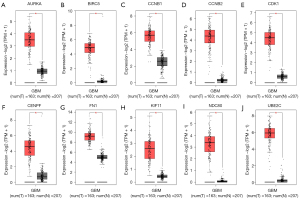
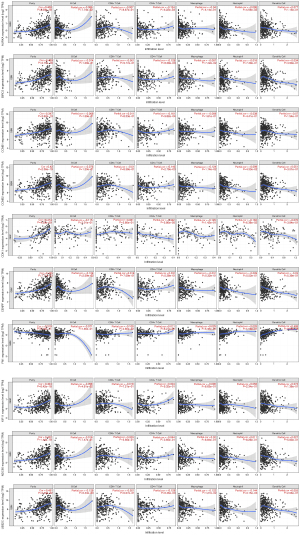
Screening drugs and targets
Based on the chemical composition data of Zanthoxylum nitidum, rhizoma belamcandae, black bean, and suberect spatholobus stem, ADME (absorption, distribution, metabolism, and excretion) can be used as the threshold to screen active components (Table 1).
Table 1
Drug | MOLID | Main active ingredients | OB (%) | DL |
---|---|---|---|---|
Zanthoxylum nitidum | MOL001458 | Coptisine | 30.67 | 0.86 |
MOL005084 | 7-demethyl-6-methoxy-5,6-dihydrochelerythrine | 30.74 | 0.83 | |
MOL002881 | Diosmetin | 31.14 | 0.27 | |
MOL001461 | Dihydrochelerythrine | 32.73 | 0.81 | |
MOL005103 | 6-[(2R)-2,3-dihydroxy-3-methyl-butyl]-5,7-dimethoxy-coumarin | 33.55 | 0.22 | |
MOL005106 | Oxyterihanine | 33.93 | 0.81 | |
MOL005097 | 5-[(1S,3aS,4R,6aS)-1-(1,3-benzodioxol-5-yl)-1,3,3a,4,6,6a-hexahydrofuro[4,3-c]furan-4-yl]-2-methoxyphenol | 36.73 | 0.67 | |
MOL000359 | Sitosterol | 36.91 | 0.75 | |
MOL000358 | Beta-sitosterol | 36.91 | 0.75 | |
MOL001474 | Sanguinarine | 37.81 | 0.86 | |
MOL005108 | Isofagaridine | 38.34 | 0.77 | |
MOL002663 | Skimmianin | 40.14 | 0.2 | |
MOL002644 | Phellopterin | 40.19 | 0.28 | |
MOL005105 | Oxychelerythrine | 44.22 | 0.84 | |
Rhizoma belamcandae | MOL002322 | Isovitexin | 31.29 | 0.72 |
MOL001735 | Dinatin | 30.97 | 0.27 | |
MOL000449 | Stigmasterol | 43.83 | 0.76 | |
MOL000354 | Isorhamnetin | 49.6 | 0.31 | |
MOL003742 | Ardisianone A | 44.22 | 0.25 | |
MOL003753 | Dihydrokaempferide | 50.56 | 0.27 | |
MOL003758 | Iristectorigenin (9CI) | 71.55 | 0.34 | |
MOL003759 | Iristectorigenin A | 63.36 | 0.34 | |
MOL003769 | Irolone | 46.87 | 0.36 | |
MOL000006 | Luteolin | 36.16 | 0.25 | |
Suberect Spatholobus stem | MOL000296 | Hederagenin | 36.91 | 0.75 |
MOL000033 | (3S,8S,9S,10R,13R,14S,17R)-10,13-dimethyl-17-[(2R,5S)-5-propan-2-yloctan-2-yl]-2,3,4,7,8,9,11,12,14,15,16,17-dodecahydro-1H-cyclopenta[a]phenanthren-3-ol | 36.23 | 0.78 | |
MOL000358 | Beta-sitosterol | 36.91 | 0.75 | |
MOL000392 | Formononetin | 69.67 | 0.21 | |
MOL000417 | Calycosin | 47.75 | 0.24 | |
MOL000449 | Stigmasterol | 43.83 | 0.76 | |
MOL000461 | 3,7-dihydroxy-6-methoxy-dihydroflavonol | 43.8 | 0.26 | |
MOL000468 | 8-o-Methylreyusi | 70.32 | 0.27 | |
MOL000469 | 3-Hydroxystigmast-5-en-7-one | 40.93 | 0.78 | |
MOL000470 | 8-C-α-L-arabinosylluteolin | 35.54 | 0.66 | |
MOL000471 | Aloe-emodin | 83.38 | 0.24 | |
MOL000483 | (Z)-3-(4-hydroxy-3-methoxy-phenyl)-N-[2-(4-hydroxyphenyl)ethyl]acrylamide | 118.35 | 0.26 | |
MOL000490 | Petunidin | 30.05 | 0.31 | |
MOL000492 | (+)-catechin | 54.83 | 0.24 | |
MOL000493 | Campesterol | 37.58 | 0.71 | |
MOL000493 | Campesterol | 37.58 | 0.71 | |
MOL000497 | Licochalcone A | 40.79 | 0.29 | |
MOL000500 | Vestitol | 74.66 | 0.21 | |
MOL000501 | Consume close grain | 68.12 | 0.27 | |
MOL000502 | Cajinin | 68.8 | 0.27 | |
MOL000503 | Medicagol | 57.49 | 0.6 | |
MOL000506 | Lupinidine | 61.89 | 0.21 | |
MOL000507 | Psi-Baptigenin | 70.12 | 0.31 | |
MOL000006 | Luteolin | 36.16 | 0.25 | |
Black bean | MOL000449 | Stigmasterol | 43.83 | 0.76 |
MOL000358 | Beta-sitosterol | 36.91 | 0.75 | |
MOL003629 | Daidzein-4,7-diglucoside | 47.27 | 0.67 | |
MOL000433 | FA | 68.96 | 0.71 | |
MOL004798 | Delphinidin | 40.63 | 0.28 | |
MOL005030 | Gondoic acid | 30.7 | 0.2 | |
MOL009706 | Pramoxine | 49.08 | 0.19 | |
MOL009711 | Cycloartenone | 40.57 | 0.79 | |
MOL009722 | L-Folinic acid | 31.79 | 0.74 | |
MOL009729 | Methyl (E)-nonadec-10-enoate | 30.66 | 0.19 | |
MOL009733 | Methyl 12-oxooctadec-9-enoate | 37.45 | 0.19 | |
MOL009735 | N-(p-Hydroxyphenethyl) actinidine | 62.16 | 0.19 |
OB, oral bioavailability; DL, drug-likeness.
Construction of the PPI network of traditional Chinese medicine components-target genes
Using the custom Venn diagram tool to draw a Venn diagram, eight core genes with widely recognized differential expression and pharmacological target activity were screened out: CDK2, CHEK1, PLAU, CCNB1, BIRC5, EGFR, VEGFA, and TOP2A. The core genes were imported into the STRING database to construct the PPI network and expand it, as shown in Figures 11 and 12, respectively.
Screening active components of traditional Chinese medicine
CytoScape 3.8.2 software was employed to construct the interaction network between traditional Chinese medicine active components-GBM DEGs, as shown in Figure 13. The degree score of each traditional Chinese medicine component node was calculated by using the cytoHubba plug-in in order to select the core active components, as shown in Table 2. In particular, the interaction network between core genes and active ingredients of traditional Chinese medicine is shown in Figure 14. The purple, gray, green, and blue indicated that the nodes come from rhizoma belamcandae, black bean, suberect spatholobus stem, and zanthoxylum nitidum, respectively, and components with high degree scores were specially marked according to Table 2. See annex for the abbreviation comparison table of Figure 2.
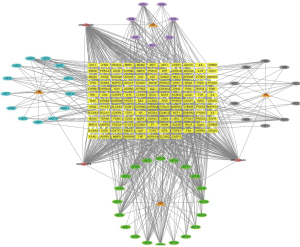
Table 2
Name | Molecular name | Degree |
---|---|---|
MOL000006 | Luteolin | 112 |
MOL000449 | Stigmasterol | 87 |
MOL000358 | Beta-sitosterol | 71 |
MOL003629 | Daidzein-4,7-diglucoside | 38 |
MOL000354 | Isorhamnetin | 32 |
MOL000497 | Licochalcone A | 32 |
MOL000500 | Vestitol | 29 |
MOL000468 | 8-o-Methylreyusi | 27 |
MOL000296 | Hederagenin | 25 |
MOL000471 | Aloe-emodin | 23 |
PPI, protein-protein interaction.
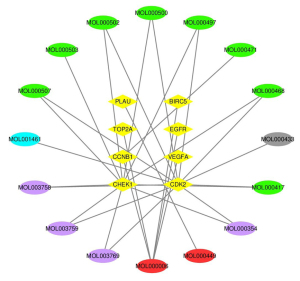
Molecular docking
The intersecting genes from between the 10 hub genes and eight core genes were BIRC5 and CCNB1, with markedly up-regulated expression and significant potential as drug targets. Autodocktools-1.5.6 and PyMol software were applied for the molecular docking of BIRC5 and CCNB1 with luteolin, respectively. The detailed data are shown in Table 3. According to the data, luteolin established several hydrogen bonds with BIRC5 and CCNB1 to form a stable complex (Figure 15). Molecular docking verified that luteolin could directly or indirectly affect key targets proteins such as BIRC5 and CCNB1, which could be regarded as the main evidence of luteolin inhibiting the growth of GBM, and predicted their potential in targeted therapies for GBM.
Table 3
Receptor | Ligand | Bine energy | Hydrogen bond |
---|---|---|---|
BIRC5 | Luteolin | −4.62 kcal/mol | ASP29 (length: 1.9Å) |
ASP29 (length: 2.2Å) | |||
GLU125 (length: 2.3Å) | |||
GLU125 (length: 3.2Å) | |||
GLU125 (length: 3.2Å) | |||
GLU126 (length: 2.0Å) | |||
CCNB1 | Luteolin | −4.3 kcal/mol | VAL227 (length: 1.8Å) |
VAL227 (length: 2.5Å) | |||
SER248 (length: 2.0Å) | |||
SER248 (length: 3.1Å) | |||
TYR270 (length: 2.5Å) |
Discussion
GBM is an extremely malignant tumor that has puzzled researchers. Most LGGs will relapse within 5–10 years and then develop into GBM. Malignant progression of GBM is a complex process; the formation and increase in the degree of malignancy are caused by the accumulation of multiple genetic changes involving the distortion of a variety of signaling pathways and interaction networks (14). O6-methylguanine-DNA methyltransferase (MGMT) methylation, epidermal growth factor receptor (EGFR), and isocitrate dehydrogenase 1, 2 (IDH1, IDH2), which are involved in tumorigenesis and promote the progression of GBM, have been identified as molecular markers for GBM (3). Clinical practice has shown that GBM patients with MGMT-promoter methylation and IDH1 mutation have a better prognosis than those without mutation (15). This study is aimed to identify DEGs (such as MGMT) and understand their differential molecular mechanisms and biological effects in normal and cancerous tissues, which are involved in tumorigenesis and conducive to the diagnosis and treatment of GBM (16,17).
At present, bioinformatics and network pharmacology are playing more and more important roles in clinical research. With the update and improvement of the database, researchers can propose innovative and potential treatment schemes for more diseases. Recently, the therapeutic effects of patchouli alcohol on gastric cancer (18), luteolin on prostate cancer (19) and Xihuang Pill on triple-negative breast cancer (20) were preliminarily verified by network pharmacology and further confirmed by experiments, which proved the feasibility of our research.
In this study, we conducted a detailed and in-depth investigation and screened out core intersecting genes and active components of traditional Chinese medicine based on the existing analysis data and literature. By analyzing the intersecting genes and active components of traditional Chinese medicine, we obtained two potential DEGs that may be closely related to the malignant progression of GBM: BIRC5 and CCNB1. Our innovation lies in finding the possibility of applying traditional Chinese medicine ingredients to glioblastoma through network pharmacology. We construct the “disease-gene-target-drug” interaction network by using Cytoscape software to analyze topological parameters to find luteolin, the active ingredient of traditional Chinese medicine, and verify its feasibility by molecular docking analysis. We also identified the potential active component of traditional Chinese medicine, which has been extensively correlated with various target genes and widely verified for its anti-tumor effects: luteolin. Furthermore, Anson et al. found that in addition to promoting termination of the cell cycle, luteolin can also induce the cleavage of Caspase (cysteinyl aspartate specific proteinase) and PARP (poly ADP-ribose polymerase) in GBM cells, thereby inhibiting the proliferation of cancer cells (18), as shown in Table 2. These findings further demonstrate the feasibility of this research direction. Therefore, the present study focused on BIRC5, CCNB1, and luteolin, which have considerable significance for GBM and provide a theoretical and practical basis for the development of new drugs in the future.
Baculoviral IAP Repeat Containing 5 (BIRC5) is an apoptotic inhibitor protein (IAP), which has the characteristics of cell cycle-dependent expression and significantly up-regulated expression in the G2/M phase (19). BIRC5 is believed to inhibit the activity of Caspase-3 and Caspase-7 to impede cell apoptosis and promote cell mitosis and tumor progression (20,21). Also, it is widely considered a potential therapeutic target (22,23). Previous studies have shown that BIRC5 is up-regulated in oral squamous cell carcinoma (24), breast cancer, and renal cell carcinoma (25). For our purpose, BIRC5 has been shown to be associated with the occurrence of GBM. G2/mitotic-specific cyclin-B1 (CCNB1) is a key factor in initiating mitosis (26), which plays a certain regulatory role in G2/M transition (27,28). It performs this by acting as the regulatory subunit of the maturity promoter factor (MPF) and interacting with cyclin-dependent kinase (CDK1). This leads to the transition of cells from the G2 phase to the M phase (29), which is related to the occurrence and prognosis (30) in tumors such as colon cancer (31), ovarian cancer (32), pancreatic cancer (33), and hepatocellular carcinoma (34).
Luteolin (3,4,5,7-tetra hydroxy flavone) is a common flavonoid compound, which has significant pharmacological properties, such as anti-inflammation, anti-oxidation, and anti-tumor (35), as well as extremely high research and application value. In terms of its anti-tumor properties, luteolin has been shown to inhibit the cell cycle and the mitogen-activated protein kinase (MAPK) signaling transduction pathway, and induce tumor suppressor p53, which exerts good prevention and treatment effects on the progression of colon, breast, and gastric cancers (36-40). Other studies have shown that luteolin can block the cell cycle and induce apoptosis of cancer cells by activating the phosphatidylinositol 3 kinase (PI3K)/protein kinase B (Akt) pathway, thereby inducing the expression of Endoplasmic Reticulumb (ER) stress-related proteins, increasing the level of intracellular reactive oxygen species (ROS), and inducing mitochondrial autophagy (41-45) and other mechanisms. This leads to inhibition of the occurrence and malignant progression of GBM. Therefore, it is speculated that the possibility of occurrence and malignant progression of GBM can be reduced through the regulation of the key target genes, BIRC5 and CCNB1, by luteolin.
In this study, we found and preliminarily verified the abnormally up-regulated genes, BIRC5 and CCNB1, in GBM, which were associated with tumorigenesis and malignant progression to a certain extent. We also further screened out the active traditional Chinese medicine component, luteolin, for GBM via the network pharmacology method. Therefore, luteolin could potentially affect the occurrence and progression of GBM by interfering with cell division through potential prognostic biomarkers (BIRC5 and CCNB1), which provides a foundation for new drug development of GBM-targeted therapies.
Acknowledgments
Funding: This work was supported by
Footnote
Reporting Checklist: The authors have completed the STREGA reporting checklist. Available at https://tcr.amegroups.com/article/view/10.21037/tcr-22-1122/rc
Conflicts of Interest: All authors have completed the ICMJE uniform disclosure form (available at https://tcr.amegroups.com/article/view/10.21037/tcr-22-1122/coif). The authors have no conflicts of interest to declare.
Ethical Statement: The authors are accountable for all aspects of the work in ensuring that questions related to the accuracy or integrity of any part of the work are appropriately investigated and resolved. This study was conducted in accordance with the Declaration of Helsinki (as revised in 2013).
Open Access Statement: This is an Open Access article distributed in accordance with the Creative Commons Attribution-NonCommercial-NoDerivs 4.0 International License (CC BY-NC-ND 4.0), which permits the non-commercial replication and distribution of the article with the strict proviso that no changes or edits are made and the original work is properly cited (including links to both the formal publication through the relevant DOI and the license). See: https://creativecommons.org/licenses/by-nc-nd/4.0/.
References
- Wirsching HG, Galanis E, Weller M. Glioblastoma. Handb Clin Neurol 2016;134:381-97. [Crossref] [PubMed]
- Stupp R, Mason WP, van den Bent MJ, et al. Radiotherapy plus concomitant and adjuvant temozolomide for glioblastoma. N Engl J Med 2005;352:987-96. [Crossref] [PubMed]
- Xu B. Prediction and analysis of hub genes between glioblastoma and low-grade glioma using bioinformatics analysis. Medicine (Baltimore) 2021;100:e23513. [Crossref] [PubMed]
- Davis ME. Glioblastoma: Overview of Disease and Treatment. Clin J Oncol Nurs 2016;20:S2-8. [Crossref] [PubMed]
- Hegi ME, Liu L, Herman JG, et al. Correlation of O6-methylguanine methyltransferase (MGMT) promoter methylation with clinical outcomes in glioblastoma and clinical strategies to modulate MGMT activity. J Clin Oncol 2008;26:4189-99. [Crossref] [PubMed]
- Washah HN, Salifu EY, Soremekun O, et al. Integrating Bioinformatics Strategies in Cancer Immunotherapy: Current and Future Perspectives. Comb Chem High Throughput Screen 2020;23:687-98. [Crossref] [PubMed]
- Song X, Du R, Gui H, et al. Identification of potential hub genes related to the progression and prognosis of hepatocellular carcinoma through integrated bioinformatics analysis. Oncol Rep 2020;43:133-46. [PubMed]
- Wang Y, Yang C, Li W, et al. Identification of colon tumor marker NKD1 via integrated bioinformatics analysis and experimental validation. Cancer Med 2021;10:7383-94. [Crossref] [PubMed]
- Tao Q, Du J, Li X, et al. Network pharmacology and molecular docking analysis on molecular targets and mechanisms of Huashi Baidu formula in the treatment of COVID-19. Drug Dev Ind Pharm 2020;46:1345-53. [Crossref] [PubMed]
- Zhang R, Zhu X, Bai H, et al. Network Pharmacology Databases for Traditional Chinese Medicine: Review and Assessment. Front Pharmacol 2019;10:123. [Crossref] [PubMed]
- Li S, Zhang B. Traditional Chinese medicine network pharmacology: theory, methodology and application. Chin J Nat Med 2013;11:110-20. [Crossref] [PubMed]
- Shakeri M, Hashemi Tayer A, Shakeri H, et al. Toxicity of Saffron Extracts on Cancer and Normal Cells: A Review Article. Asian Pac J Cancer Prev 2020;21:1867-75. [Crossref] [PubMed]
- Li ZH, Yu D, Huang NN, et al. Immunoregulatory mechanism studies of ginseng leaves on lung cancer based on network pharmacology and molecular docking. Sci Rep 2021;11:18201. [Crossref] [PubMed]
- Dubois LG, Campanati L, Righy C, et al. Gliomas and the vascular fragility of the blood brain barrier. Front Cell Neurosci 2014;8:418. [Crossref] [PubMed]
- Dahlrot RH, Dowsett J, Fosmark S, et al. Prognostic value of O-6-methylguanine-DNA methyltransferase (MGMT) protein expression in glioblastoma excluding nontumour cells from the analysis. Neuropathol Appl Neurobiol 2018;44:172-84. [Crossref] [PubMed]
- Martin DH, Bianchi E, Ben Mustapha S, et al. Glioblastoma. Rev Med Liege 2021;76:419-24. [PubMed]
- Waitkus MS, Diplas BH, Yan H. Biological Role and Therapeutic Potential of IDH Mutations in Cancer. Cancer Cell 2018;34:186-95. [Crossref] [PubMed]
- Anson DM, Wilcox RM, Huseman ED, et al. Luteolin Decreases Epidermal Growth Factor Receptor-Mediated Cell Proliferation and Induces Apoptosis in Glioblastoma Cell Lines. Basic Clin Pharmacol Toxicol 2018;123:678-86. [Crossref] [PubMed]
- Li Y, Zhao ZG, Luo Y, et al. Dual targeting of Polo-like kinase 1 and baculoviral inhibitor of apoptosis repeat-containing 5 in TP53-mutated hepatocellular carcinoma. World J Gastroenterol 2020;26:4786-801. [Crossref] [PubMed]
- Varughese RK, Torp SH. Survivin and gliomas: A literature review. Oncol Lett 2016;12:1679-86. [Crossref] [PubMed]
- Oparina N, Erlandsson MC, Fäldt Beding A, et al. Prognostic Significance of BIRC5/Survivin in Breast Cancer: Results from Three Independent Cohorts. Cancers (Basel) 2021;13:2209. [Crossref] [PubMed]
- Wheatley SP, Altieri DC. Survivin at a glance. J Cell Sci 2019;132:jcs223826. [Crossref] [PubMed]
- Frazzi R. BIRC3 and BIRC5: multi-faceted inhibitors in cancer. Cell Biosci 2021;11:8. [Crossref] [PubMed]
- Troiano G, Guida A, Aquino G, et al. Integrative Histologic and Bioinformatics Analysis of BIRC5/Survivin Expression in Oral Squamous Cell Carcinoma. Int J Mol Sci 2018;19:2664. [Crossref] [PubMed]
- Li F, Aljahdali IAM, Zhang R, et al. Kidney cancer biomarkers and targets for therapeutics: survivin (BIRC5), XIAP, MCL-1, HIF1, HIF2, NRF2, MDM2, MDM4, p53, KRAS and AKT in renal cell carcinoma. J Exp Clin Cancer Res 2021;40:254. [Crossref] [PubMed]
- Alfonso-Pérez T, Hayward D, Holder J, et al. MAD1-dependent recruitment of CDK1-CCNB1 to kinetochores promotes spindle checkpoint signaling. J Cell Biol 2019;218:1108-17. [Crossref] [PubMed]
- Wang F, Chen X, Yu X, et al. Degradation of CCNB1 mediated by APC11 through UBA52 ubiquitination promotes cell cycle progression and proliferation of non-small cell lung cancer cells. Am J Transl Res 2019;11:7166-85. [PubMed]
- Kim BK, Kim I, Lee AR, et al. Mouse-specific up-regulation of Ccnb1 expression by miR-199a-5p in keratinocyte. FEBS Open Bio 2016;6:1131-40. [Crossref] [PubMed]
- Zou Y, Ruan S, Jin L, et al. CDK1, CCNB1, and CCNB2 are Prognostic Biomarkers and Correlated with Immune Infiltration in Hepatocellular Carcinoma. Med Sci Monit 2020;26:e925289. [Crossref] [PubMed]
- Si T, Huang Z, Jiang Y, et al. Expression Levels of Three Key Genes CCNB1, CDC20, and CENPF in HCC Are Associated With Antitumor Immunity. Front Oncol 2021;11:738841. [Crossref] [PubMed]
- Li J, Zhou L, Liu Y, et al. Comprehensive Analysis of Cyclin Family Gene Expression in Colon Cancer. Front Oncol 2021;11:674394. [Crossref] [PubMed]
- Fei F, Qu J, Liu K, et al. The subcellular location of cyclin B1 and CDC25 associated with the formation of polyploid giant cancer cells and their clinicopathological significance. Lab Invest 2019;99:483-98. [Crossref] [PubMed]
- Zhang H, Zhang X, Li X, et al. Effect of CCNB1 silencing on cell cycle, senescence, and apoptosis through the p53 signaling pathway in pancreatic cancer. J Cell Physiol 2018;234:619-31. [Crossref] [PubMed]
- Xia P, Zhang H, Xu K, et al. MYC-targeted WDR4 promotes proliferation, metastasis, and sorafenib resistance by inducing CCNB1 translation in hepatocellular carcinoma. Cell Death Dis 2021;12:691. [Crossref] [PubMed]
- Caporali S, De Stefano A, Calabrese C, et al. Anti-Inflammatory and Active Biological Properties of the Plant-Derived Bioactive Compounds Luteolin and Luteolin 7-Glucoside. Nutrients 2022;14:1155. [Crossref] [PubMed]
- Ambasta RK, Gupta R, Kumar D, et al. Can luteolin be a therapeutic molecule for both colon cancer and diabetes? Brief Funct Genomics 2018;18:230-9. [Crossref] [PubMed]
- Fasoulakis Z, Koutras A, Syllaios A, et al. Breast Cancer Apoptosis and the Therapeutic Role of Luteolin. Chirurgia (Bucur) 2021;116:170-7. [Crossref] [PubMed]
- Tuorkey MJ. Molecular targets of luteolin in cancer. Eur J Cancer Prev 2016;25:65-76. [Crossref] [PubMed]
- Ahmed S, Khan H, Fratantonio D, et al. Apoptosis induced by luteolin in breast cancer: Mechanistic and therapeutic perspectives. Phytomedicine 2019;59:152883. [Crossref] [PubMed]
- Ganai SA, Sheikh FA, Baba ZA, et al. Anticancer activity of the plant flavonoid luteolin against preclinical models of various cancers and insights on different signalling mechanisms modulated. Phytother Res 2021;35:3509-32. [Crossref] [PubMed]
- Imran M, Rauf A, Abu-Izneid T, et al. Luteolin, a flavonoid, as an anticancer agent: A review. Biomed Pharmacother 2019;112:108612. [Crossref] [PubMed]
- Lin Y, Shi R, Wang X, et al. Luteolin, a flavonoid with potential for cancer prevention and therapy. Curr Cancer Drug Targets 2008;8:634-46. [Crossref] [PubMed]
- Farooqi AA, Butt G, El-Zahaby SA, et al. Luteolin mediated targeting of protein network and microRNAs in different cancers: Focus on JAK-STAT, NOTCH, mTOR and TRAIL-mediated signaling pathways. Pharmacol Res 2020;160:105188. [Crossref] [PubMed]
- Wang Q, Wang H, Jia Y, et al. Luteolin reduces migration of human glioblastoma cell lines via inhibition of the p-IGF-1R/PI3K/AKT/mTOR signaling pathway. Oncol Lett 2017;14:3545-51. [Crossref] [PubMed]
- Wang Q, Wang H, Jia Y, et al. Luteolin induces apoptosis by ROS/ER stress and mitochondrial dysfunction in gliomablastoma. Cancer Chemother Pharmacol 2017;79:1031-41. [Crossref] [PubMed]
(English Language Editor: A. Kassem)