Development and validation of endoplasmic reticulum stress-related eight-gene signature for predicting the overall survival of lung adenocarcinoma
Introduction
As one of the most serious malignant tumors, lung cancer had a high mortality rate of 13.5% (1). Among them, the most common type of lung cancer is lung adenocarcinoma (LUAD), accounting for approximately 40% of all lung cancers (2). Etiologically lung cancer is strongly associated with tobacco smoking, air pollution and inherited genetic susceptibility (3). In recent years, despite the dramatic improvements in lung cancer therapies, the 5‐year survival rate is far from being satisfactory (4). Accurate prognostic assessment to allow optimal clinical decision-making is vital to improving patient outcomes. At present, tumor-nodal-metastasis stage (TNM-stage) is widely used to predict the prognosis of LUAD patients (5). However, the outcomes for patients with a similar TNM-stage are highly variable because of tumor heterogeneity, suggesting that additional molecular markers in combination with TNM-staging system are urgently needed to improve prognosis prediction of patients with lung cancer. In recent years, tremendous efforts have been undertaken to integrate biomarkers and clinicopathologic information into a single multivariate model to predict outcomes. Gao et al. found that the ferroptosis-related gene signature has been used to predict survival in LUAD patients (6). A previous study has demonstrated that the 15 DNA damage repair-related gene signature have predictive and prognostic value in LUAD (7). Nevertheless, the lack of a larger sample size limits our ability to determine the prognostic value of biomarker with more precision.
Accumulation of unfolded proteins in the ER lumen results in ER stress. Hostile microenvironmental within tumor disturb the protein folding capacity of the ER, thereby provoking a cellular state of “ER stress”. Sustained activation of ER stress sensors endows cells with greater tumorigenic conditions (8). Findings from a recent study suggest that endoplasmic reticulum (ER) stress confers 5-fluorouracil resistance in breast cancer cell via the GRP78/OCT4/lncRNA MIAT/AKT pathway (9). There is also a study suggests that GRP78 mediates the sensitivity of human colon cancer cells to 5-FU via ER stress induction (10). There are also studies suggesting that ER stress is associated with apoptosis of colon cancer cells (11,12). ER stress is associated with many pathologic processes and has been shown to participate in the development of cancer.
In the present study, we identified 8-gene prognostic signature associated with ER stress and risk scores from The Cancer Genome Atlas (TCGA) dataset. Then we created a prognostic nomogram based on characteristics of 8-gene signature and TNM-stage to assess reliability and clinical applicability of our model. Finally, in order to reveal the complex mechanisms of carcinogenesis, Gene Ontology (GO), Kyoto Encyclopedia of Genes and Genomes (KEGG) and gene set enrichment analysis (GSEA) were carried out. We further validate our models in four independent datasets. Importantly, the sample size for both training and test sets is relatively large. Our results show that 8-gene signature can be used as a novel prognostic tool for LUAD and provide more insights into the molecular mechanisms. We present the following article in accordance with the TRIPOD reporting checklist (available at https://tcr.amegroups.com/article/view/10.21037/tcr-22-106/rc).
Methods
Data source
Level 3 RNA-seq expression data and clinical data were obtained from TCGA project. The tumor expression datasets used in validation were downloaded from GEO database (http://www.ncbi.nlm.nih.gov/geo/) with the accession number GSE30219 (13), GSE31210 (14), GSE50081 (15) and GSE37745 (16), corresponding clinical information was also obtained from these databases. The known ER stress -associated genes were searched from the GeneCards database. We excluded patients without any available follow-up information and removed the data with incomplete survival time and survival status information. Finally, RNA sequencing (RNA-Seq) data consisting of 535 LUAD and 59 normal lung samples were acquired from TCGA-LUAD. Among them, the TCGA-LUAD dataset included 294 stage I, 123 stage II, 84 stage III, and 26 stage IV tumours. GSE30219 consisting of 293 lung tumor samples and 14 non-tumoral lung samples. GSE31210 consisting of 226 LUADs samples with stage I–II. GSE50081 consisting of 127 LUADs samples. GSE37745 consisting of 106 LUADs samples. Expression profiling was performed using an Affymetrix Human Genome U133 Plus 2.0 Array.
Construction overall survival (OS)-related gene signature in TCGA-LUAD
The “glmnet” and “survival” package from R software (version 3.6.3) was used to perform the LASSO Cox regression model analysis. The “lambda.1se” value, which was determined by tenfold cross-validation, was set as the lambda for model fitting. Subsequently, we calculated a risk score for each sample, which was calculated as a linear combination of expression levels of genes in one signature set weighted by their respective LASSO regression coefficients according to a previous report by the following formula: “risk score” = Σ (regression coefficient) × (expression value of each prognostic mRNA). A median risk score was used to divide patients into high- and low-risk groups and then subjected to Kaplan-Meier survival analysis. Kaplan-Meier analysis was performed using R survminer and survival packages, with P<0.05 taken as significant. The package “timeROC” and “ggplot2” were used to evaluate the sensitivity and specificity of the prediction model through the area under the ROC curve (AUC). Differential expression analysis of gene signature between the high-risk and low-risk groups were analysed with the Mann-Whitney U-test, differences were considered statistically significant when P<0.05.
The nomogram establishing
A nomogram based on the TNM staging system and risk score was created by R software, using the “rms” and “survival” package. Calibration curves were assessed graphically by plotting the actual observed survival rates and the nomogram-predicted survival rates. The discrimination performance of nomograms was measured quantitatively by concordance indices (C-index).
External dataset verifies the robustness of the eight -gene signature model
GSE50081 and GSE37745 were obtained from the same microarray platform (Affymetrix Human Genome U133 Plus 2.0 Array). We performed an integrative analysis by combining the two datasets to increase the sample size and detection power, because the sample size was relatively small for GSE50081 and GSE37745. Next, GSE30219, GSE31210 and (GSE50081+GSE37745) were used for validation of the models. The same formula and regression coefficients were applied for the validation set for risk score calculation. Similarly, KM survival curves and histogram between high‐risk score group and low‐risk score group and the distribution of risk scores, the survival status and gene expression heat maps were plotted for each of the validation sets.
GO, KEGG and GSEA enrichment analyses
Spearman’s correlation test was used to search for genes related to eight genes in TCGA cohort (TCGA-LUAD). P<0.01 is considered statistically significant. We calculated correlation coefficient absolute values, and the top 400 hub genes were selected for functional enrichment analysis. Spearman correlation was calculated with the cor.test function in the R stats package (v.3.6.3). The KEGG pathway analysis and GO analysis were performed using the cluster Profiler R package, the R package org.Hs.eg.db and the R package Goplot. GO and KEGG analyses were performed with P.adj<0.05 and q value <0.2 as a threshold. The threshold for significance in GSEA was set at 0.05 where we considered a significant FDR as below 0.25.
Statistical analysis
All statistical analyses were performed using the R software package. Categorical variables were expressed as numbers and percentages. We use the non-parametric Wilcoxon signed-rank test to compare differences between groups. Survival analyses were performed using the Kaplan–Meier method. Cox-proportional hazards models were used to estimate hazard ratios (HRs), β regression coefficients, HRs, P values and 95% confidence intervals (CIs). A nomogram for predicting the OS was built using the R library “rms” package in R version 3.6.3 The performance of the nomogram models was evaluated by discrimination (C-index) and calibration (calibration plots) P≤0.05 was considered to be statistically significant.
Ethical statement
The study was conducted in accordance with the Declaration of Helsinki (as revised in 2013).
Results
Flowchart on construction and validation of the 8-mRNA signature is shown in Figure 1.
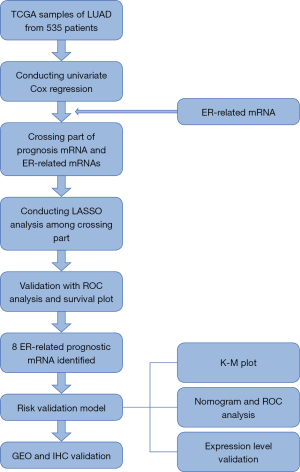
Clinical characteristics
The clinical information of 535 patients was obtained from TCGA, including TNM-stage, clinical stage, gender, race, age and histologic grade. The details are shown in Table 1.
Table 1
Characteristic | Levels | Overall |
---|---|---|
Patients, n | 535 | |
T stage, n (%) | T1 | 175 (32.9) |
T2 | 289 (54.3) | |
T3 | 49 (9.2) | |
T4 | 19 (3.6) | |
N stage, n (%) | N0 | 348 (67.1) |
N1 | 95 (18.3) | |
N2 | 74 (14.3) | |
N3 | 2 (0.4) | |
M stage, n (%) | M0 | 361 (93.5) |
M1 | 25 (6.5) | |
Pathologic stage, n (%) | Stage I | 294 (55.8) |
Stage II | 123 (23.3) | |
Stage III | 84 (15.9) | |
Stage IV | 26 (4.9) | |
Gender, n (%) | Female | 286 (53.5) |
Male | 249 (46.5) | |
Race, n (%) | Asian | 7 (1.5) |
Black or African American | 55 (11.8) | |
White | 406 (86.8) | |
Age, years, n (%) | ≤65 | 255 (49.4) |
>65 | 261 (50.6) | |
Smoker, n (%) | No | 75 (14.4) |
Yes | 446 (85.6) | |
Age, years, median (IQR) | 66 (59, 72) |
Construction of a prognostic prediction model for LUAD based on the TCGA training dataset
To identify mRNAs that correlated with the OS patients with LUAD, univariate Cox regression analysis was performed. The statistical analysis of survival was performed by R package survival and survminer, 742 mRNAs were associated with LUAD patients’ OS (P<0.01). In addition, a total of 7,157 ER-associated genes were retrieved in LUAD using GeneCards database. 347 mRNAs were common between the two groups, shown in a Venn diagram (Figure 2A). Then, the least absolute shrinkage and selection operator (LASSO) regression was used to avoid overfitting and screened eight mRNAs (LDHA, DKK1, MELTF, VDAC1, FUT4, PLK1, KRT6A and GALNT2) as variates to construct prognostic for LUAD patients (Figure 2B,2C).
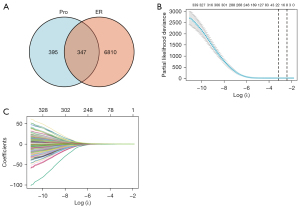
The internal validation data sets in TCGA verifies the robustness of the prognostic model
The predictive model was defined as the linear combination of the expression levels of the eight mRNAs weighted by their relative coefficient in lasso regression coefficients, as follows: risk score = (LDHA × 0.09667454) + (DKK1 × 0.06514894) + (MELTF × 0.02890339) + (VDAC1 × 0.02789367) + (FUT4 × 0.02732278) + (PLK1 × 0.01364355) + (KRT6A × 0.01078567) + (GALNT2 × 0.00537792). Risk scores for each patient in the training set were calculated based on the risk-score values, patients were stratified into low-risk (n=261) and high-risk groups (n=261), using the median risk score as the cutoff point. As shown in Figure 3A, Kaplan-Meier analysis revealed that the low-risk patients have a better OS than those with high-risk (HR 2.72, 95% CI: 2.00 to 3.69, P<0.001). Compared with the low risk score group, the patients with high risk score had worse prognosis. In time-dependent ROC curve analyses, the AUC for the eight -mRNA signature prognostic model was 0.749 at one year, 0.737 at two years and 0.707 at four years of OS, this suggests that the model had good discrimination (AUCmax =0.749) (Figure 3B). Next, we conducted the gene expression analysis of 8-gene signature between high and low risk group. Results showed that the expression of LDHA, DKK1, MELTF, VDAC1, FUT4, PLK1, KRT6A and GALNT2 were significantly higher in the high-risk group than in the low-risk group, implying that high expressions of these genes were to be positively correlated with the high-risk score (P<0.001) (Figure 3C). The distribution of risk scores, the survival status and mRNA expression of patients were ranked by risk score and were shown in Figure 3D, we found that patients with higher risk scores were more likely to die.
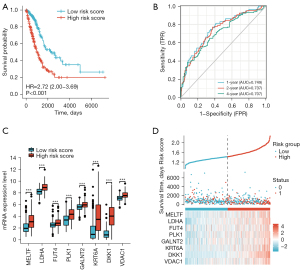
Kaplan-Meier survival analysis of LDHA, DKK1, MELTF, VDAC1, FUT4, PLK1, KRT6A and GALNT2 in TCGA-LUAD patients
We further performed the survival analysis of the eight genes expression for TCGA-LUAD patients. We separated the patients into high and low mRNA-expressing groups based on the median mRNA expression of each gene signatures. The results showed that high expression of LDHA (P<0.001, HR =1.72, Figure 4A), DKK1 (P<0.001, HR =2.01, Figure 4B), MELTF (P=0.001, HR =1.6, Figure 4C), VDAC1 (P<0.001, HR =1.88, Figure 4D), FUT4 (P<0.001, HR =1.74, Figure 4E), PLK1 (P<0.001, HR =1.87, Figure 4F), KRT6A (P=0.002, HR =1.59, Figure 4G), GALNT2 (P<0.001, HR =1.71, Figure 4H) has been associated with worse prognosis. This result was consistent with the heat map in Figure 3D. This result suggests that high expression of LDHA, DKK1, MELTF, VDAC1, FUT4, PLK1, KRT6A and GALNT2 correlates with poor prognosis in LUAD. Tables of descriptive statistics and statistically different for survival analysis are shown in website: https://cdn.amegroups.cn/static/public/tcr-22-106-01.docx.
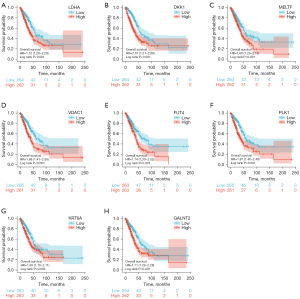
Univariate, and multivariate analysis were used to evaluate the prognostic value of TNM-stage, pathologic stage, gender, age and risk score
To identify the independence of risk score in clinical applications, risk score and clinical information for the training cohort was used to analyze the relevant HR and P value using univariate and multivariate Cox regression. Univariate analysis revealed that the pathologic stage and risk score were significant risk factors affecting patient survival (HR: 2.247; 95% CI: 1.598–3.160; P<0.001) (Figure 5A). In multivariate Cox regression analysis pathologic stage and risk score remained an independent prognostic factor (HR: 2.062; 95% CI: 1.455–2.922; P<0.001) (Figure 5B). Univariate and multivariate Cox regression analysis of TNM-stage, gender, age and risk score are shown in Table S1. Univariate and multivariate analysis were used to evaluate the prognostic value of pathologic stage, gender and risk score. The detail is shown in Table 2. The above results show that the prediction model showed good predictive power. The above results show that the prediction model showed good predictive power.
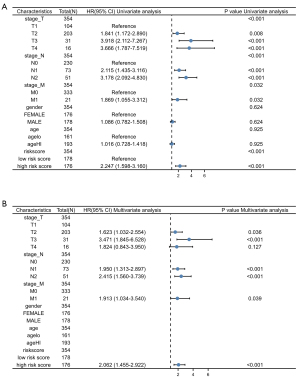
Table 2
Characteristics | Total (N) | Univariate analysis | Multivariate analysis | |||
---|---|---|---|---|---|---|
Hazard ratio (95% CI) | P value | Hazard ratio (95% CI) | P value | |||
Risk score | 514 | |||||
Low risk score | 257 | Reference | ||||
High risk score | 257 | 2.643 (1.946–3.588) | <0.001 | 2.462 (1.808–3.354) | <0.001 | |
Gender | 514 | |||||
Male | 237 | Reference | ||||
Female | 277 | 0.963 (0.721–1.286) | 0.799 | |||
Pathologic stage | 514 | |||||
Stage I | 288 | Reference | ||||
Stage II | 121 | 2.421 (1.693–3.462) | <0.001 | 2.320 (1.621–3.319) | <0.001 | |
Stage III | 80 | 3.474 (2.383–5.065) | <0.001 | 3.096 (2.119–4.525) | <0.001 | |
Stage IV | 25 | 3.797 (2.198–6.561) | <0.001 | 3.852 (2.223–6.676) | <0.001 |
Establishment of the prognostic nomogram
The optimism-corrected C-index values (for OS, C‐index = 0.739, 95% CI: 0.717–0.762) indicated that the proposed nomograms could accurately predict the 1‐, 3‐, and 5‐year OS of LUAD patients. In addition, the 1‐, 3‐, and 5‐year calibration curves of OS visually confirm good fit between predicted survival and observed survival, which could also prove the prediction accuracy of prognostic nomograms (Figure 6).
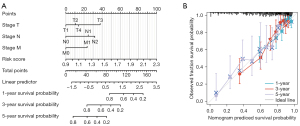
External dataset verifies the robustness of the eight-gene signature model
As a validation set we used the GSE30219, GSE31210 and (GSE50081+GSE37745) separately. Kaplan-Meier curves in the GSE31210 dataset confirmed that patients with high‐risk scores had a worse OS than patients with low‐risk scores (HR 1.60, 95% CI: 1.21–2.12, P =0.001) (Figure 7A). The risk score distribution of independent validation dataset GSE30219 was then plotted, as shown in Figure 7B. There were more deaths in high‐risk score group. The expression level of LDHA, DKK1, VDAC1, PLK1, KRT6A and GALNT2 were significantly higher in the high-risk group than in the low-risk group, implying that high expressions of these genes were to be positively correlated with the high-risk score (Figure 7C). Again, Kaplan–Meier curves in the GSE31210 dataset confirmed that patients with high‐risk scores had a worse OS than patients with low‐risk scores (HR 5.66, 95% CI: 2.91–11.00, P<0.001), (Figure 7D). The distribution of risk scores, the survival status and mRNA expression of patients were ranked by risk score and were shown in Figure 7E. The expression level of LDHA, DKK1, VDAC1, PLK1, KRT6A and GALNT2 were significantly higher in the high-risk group than in the low-risk group, implying that high expressions of these genes were to be positively correlated with the high-risk score (Figure 7F). Finally, Kaplan–Meier curves in (GSE50081+GSE37745) dataset confirmed that patients with high‐risk scores had a worse OS than patients with low‐risk scores (HR 1.66, 95% CI: 1.27–2.16, P<0.001) (Figure 8A). The distribution of risk scores, the survival status and mRNA expression of patients were ranked by risk score and were shown in Figure 8B. The expression level of LDHA, DKK1, MELTF, VDAC1, FUT4, PLK1, KRT6A and GALNT2 were significantly higher in the high-risk group than in the low-risk group, implying that high expressions of these genes were to be positively correlated with the high-risk score (Figure 8C). Tables of descriptive statistics and statistically different for survival analysis are shown in website: https://cdn.amegroups.cn/static/public/tcr-22-106-02.docx. Notably, the variation trend of subgroups eight mRNAs is consistent within the training set, even when the training and test sets consist of individuals from distinct populations. As such, the model is both robust and reliable.
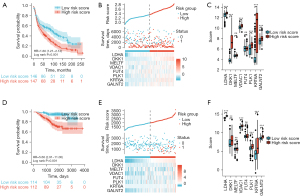
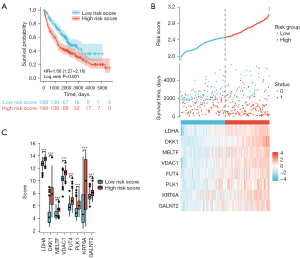
GO, KEGG and GSEA enrichment analyses
GO enrichment analysis showed correlated genes to be enriched for mitotic sister chromatid segregation, chromosome, centromeric region and cadherin binding etc. In addition, KEGG pathway analysis showed enrichment of genes associated with cell cycle glycolysis etc. (Figure 9A), for more detailed information, please see the list in Table 3. GSEA showed enrichment in cell cycle etc. (Figure 9B). The top 5 most enriched GSEA are provided in Table S2.
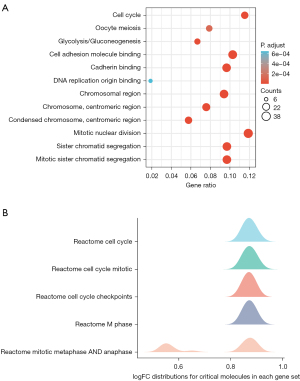
Table 3
Ontology | ID | Description | Gene ratio | Bg ratio | P value | P.adjust | Q value |
---|---|---|---|---|---|---|---|
BP | GO:0000070 | Mitotic sister chromatid segregation | 31/320 | 151/18670 | 1.04e-24 | 3.51e-21 | 2.95e-21 |
BP | GO:0140014 | Mitotic nuclear division | 38/320 | 264/18670 | 3.44e-24 | 5.79e-21 | 4.86e-21 |
BP | GO:0000819 | Sister chromatid segregation | 31/320 | 189/18670 | 1.25e-21 | 1.40e-18 | 1.17e-18 |
BP | GO:0007059 | Chromosome segregation | 38/320 | 321/18670 | 4.13e-21 | 3.48e-18 | 2.92e-18 |
BP | GO:0000280 | Nuclear division | 42/320 | 407/18670 | 6.06e-21 | 4.08e-18 | 3.43e-18 |
CC | GO:0000775 | Chromosome, centromeric region | 25/330 | 193/19,717 | 2.18e-15 | 9.56e-13 | 6.92e-13 |
CC | GO:0098687 | Chromosomal region | 31/330 | 349/19,717 | 3.72e-14 | 8.15e-12 | 5.89e-12 |
CC | GO:0000779 | Condensed chromosome, centromeric region | 19/330 | 118/19,717 | 9.77e-14 | 1.43e-11 | 1.03e-11 |
CC | GO:0005819 | Spindle | 30/330 | 347/19,717 | 1.99e-13 | 2.18e-11 | 1.58e-11 |
CC | GO:0000776 | Kinetochore | 19/330 | 135/19,717 | 1.19e-12 | 1.05e-10 | 7.56e-11 |
MF | GO:0045296 | Cadherin binding | 31/321 | 331/17,697 | 7.04e-14 | 3.94e-11 | 3.44e-11 |
MF | GO:0050839 | Cell adhesion molecule binding | 33/321 | 499/17,697 | 1.51e-10 | 4.22e-08 | 3.68e-08 |
MF | GO:0003688 | DNA replication origin binding | 6/321 | 24/17,697 | 3.47e-06 | 6.48e-04 | 5.65e-04 |
MF | GO:0008017 | Microtubule binding | 16/321 | 246/17,697 | 1.15e-05 | 0.002 | 0.001 |
MF | GO:0015631 | Tubulin binding | 18/321 | 336/17,697 | 4.51e-05 | 0.005 | 0.004 |
KEGG | hsa04110 | Cell cycle | 19/165 | 124/8,076 | 5.02e-12 | 9.99e-10 | 8.82e-10 |
KEGG | hsa00010 | Glycolysis/gluconeogenesis | 11/165 | 67/8,076 | 8.87e-08 | 8.82e-06 | 7.79e-06 |
KEGG | hsa04114 | Oocyte meiosis | 13/165 | 129/8,076 | 2.04e-06 | 1.35e-04 | 1.19e-04 |
KEGG | hsa01230 | Biosynthesis of amino acids | 8/165 | 75/8,076 | 1.35e-04 | 0.006 | 0.005 |
KEGG | hsa05166 | Human T-cell leukemia virus 1 infection | 14/165 | 219/8,076 | 1.48e-04 | 0.006 | 0.005 |
GO, Gene Ontology; KEGG, Kyoto Encyclopedia of Genes and Genomes; BP, biological process; CC, cellular component; MF, molecular function.
Differential expression in normal and tumor tissues from the TCGA-LUAD database and immunohistochemistry (IHC) results of the seven genes from the Human Protein Atlas (HPA) database
We found the immunohistochemical results of 7 key molecules (LDHA, MELTF, VDAC1, FUT4, PLK1, KRT6A and GALNT2) in the HPA database, we could qualitatively observe the obvious expression difference of LDHA, MELTF, VDAC1, PLK1, KRT6A and GALNT2 between normal and LUAD samples at the protein levels. There was no difference in FUT4 expression at the protein level (Figure 10A-10G). All of the results were consistent with data in TCGA-LUAD datasets. Unfortunately, DKK1 was absent from the HPA database.
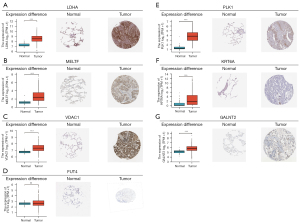
Discussion
In this study, we identified 8-gene prognostic signature associated with ER stress and risk scores from TCGA dataset. Furthermore, univariate and multivariate Cox regression analysis showed that risk score is an independent prognostic factor and is associated with poor outcomes. The nomograms showed excellent predictive ability. Finally, enrichment analysis showed enrichment in cell cycle, glycolysis, and others.
Dysregulation of the cell cycle is a hallmark of cancer (17). A study by Duan et al. found that ROS can inhibit cell growth by cell cycle arrest via ER stress (18). Hepatitis C virus (HCV) induces ER stress and alters a cascade of signal transduction pathways that control cell cycle and cellular growth (19). Our study results confirm this conclusion. In addition, while normal cells primarily rely on oxidative phosphorylation or anaerobic glycolysis to generate ATP, cancer cells often favor aerobic glycolysis in a phenomenon known as the Warburg effect. There is evidence that low oxygen tension activates complex III of the mitochondrial electron transport chain to increase cytosolic ROS production, required for stabilizing the key hypoxia response transcription factor HIF1α (20). ROS can also generate highly reactive peroxidized lipid byproducts, which form destructive covalent adducts with various ER chaperones (21,22). This conclusion was consistent with our findings. In our study, ER is involved in several tumor-related signaling pathways. Although many studies have explored the mechanisms underlying ER, it cannot be ignored, however, that the stress of the ER can be induced by various factors. This is also the potential reason for the model diversity.
In addition, a previous study has shown that LDHA upregulation independently predicts poor survival in LUAD. DKK1 promotes migration and invasion of non-small cell lung cancer via β-catenin signaling pathway (23). There is also a study shown that FAM83A-AS1 promotes LUAD cell proliferation, migration, invasion and the epithelial-mesenchymal transition (EMT) in vitro and tumor growth in vivo. Mechanistically, FAM83A-AS1 functions as an endogenous sponge of miR-150-5p by directly targeting it, removing inhibition of MMP14, a target of miR-150-5p (24). Findings from a recent study suggest that decreased expression of microRNA-320a promotes proliferation and invasion of non-small cell lung cancer cells by increasing VDAC1 expression (25). FUT4 is involved in PD-1-related immunosuppression and leads to worse survival in patients with operable LUAD (26). There is also a study suggests that Plk1 promotes the migration of human LUAD epithelial cells via STAT3 signaling (27). KRT6A promotes lung cancer cell growth and invasion through MYC-Regulated pentose phosphate pathway (28). Furthermore, GALNT2 promotes cell proliferation, migration and invasion by activating the Notch/Hes1-PTEN-PI3K/Akt signaling pathway in LUAD (29). The above results show that LDHA, DKK1, MELTF, VDAC1, FUT4, PLK1, KRT6A and GALNT2 promotes tumor progression in LUAD suggesting its role as an oncogene. It is consistent with our study.
However, this study also has some limitations. First of all, these findings also needed a wet lab validation to determine their true oncogenic potential, this is something to study in future work. Furthermore, in our study, due to the inclusion of multiple data sets, and fail to fully remove the batch effect in such cases. Despite these shortcomings, our results also provided valuable information about prognostic predictions for patients with LUAD.
Conclusions
A prognostic model based on 8 genes was constructed. Extensive validation shows that this prediction model was proved to be robust and reliable. Our study further suggests that this difference between risk-score groups is primarily associated with cell cycle and glycolysis. The 8-mRNA signature may enable improved the prediction of clinical events and decisions about management of LUAD.
Acknowledgments
Funding: This work was supported by a grant from the Natural Science Foundation of Heilongjiang Province of China (Grant No. LH2020H060).
Footnote
Reporting Checklist: The authors have completed the TRIPOD reporting checklist. Available at https://tcr.amegroups.com/article/view/10.21037/tcr-22-106/rc
Data Sharing Statement: Available at https://tcr.amegroups.com/article/view/10.21037/tcr-22-106/dss
Conflicts of Interest: All authors have completed the ICMJE uniform disclosure form (available at https://tcr.amegroups.com/article/view/10.21037/tcr-22-106/coif). The authors have no conflicts of interest to declare.
Ethical Statement: The authors are accountable for all aspects of the work in ensuring that questions related to the accuracy or integrity of any part of the work are appropriately investigated and resolved. The study was conducted in accordance with the Declaration of Helsinki (as revised in 2013).
Open Access Statement: This is an Open Access article distributed in accordance with the Creative Commons Attribution-NonCommercial-NoDerivs 4.0 International License (CC BY-NC-ND 4.0), which permits the non-commercial replication and distribution of the article with the strict proviso that no changes or edits are made and the original work is properly cited (including links to both the formal publication through the relevant DOI and the license). See: https://creativecommons.org/licenses/by-nc-nd/4.0/.
References
- Lubuzo B, Ginindza T, Hlongwana K. Exploring barriers to lung cancer patient access, diagnosis, referral and treatment in Kwazulu-Natal, South Africa: the health providers' perspectives. Transl Lung Cancer Res 2019;8:380-91. [Crossref] [PubMed]
- Kim N, Kim HK, Lee K, et al. Single-cell RNA sequencing demonstrates the molecular and cellular reprogramming of metastatic lung adenocarcinoma. Nat Commun 2020;11:2285. [Crossref] [PubMed]
- Shvedova AA, Kisin ER, Yanamala N, et al. MDSC and TGFβ Are Required for Facilitation of Tumor Growth in the Lungs of Mice Exposed to Carbon Nanotubes. Cancer Res 2015;75:1615-23. [Crossref] [PubMed]
- Schoenfeld AJ, Arbour KC, Rizvi H, et al. Severe immune-related adverse events are common with sequential PD-(L)1 blockade and osimertinib. Ann Oncol 2019;30:839-44. [Crossref] [PubMed]
- McCarthy CM, Klassen AF, Cano SJ, et al. Patient satisfaction with postmastectomy breast reconstruction: a comparison of saline and silicone implants. Cancer 2010;116:5584-91. [Crossref] [PubMed]
- Gao X, Tang M, Tian S, et al. A ferroptosis-related gene signature predicts overall survival in patients with lung adenocarcinoma. Future Oncol 2021;17:1533-44. [Crossref] [PubMed]
- Gu L, Xu Y, Jian H. Identification of a 15 DNA Damage Repair-Related Gene Signature as a Prognostic Predictor for Lung Adenocarcinoma. Comb Chem High Throughput Screen 2021; [Epub ahead of print]. [PubMed]
- Cubillos-Ruiz JR, Bettigole SE, Glimcher LH. Tumorigenic and Immunosuppressive Effects of Endoplasmic Reticulum Stress in Cancer. Cell 2017;168:692-706. [Crossref] [PubMed]
- Yao X, Tu Y, Xu Y, et al. Endoplasmic reticulum stress confers 5-fluorouracil resistance in breast cancer cell via the GRP78/OCT4/lncRNA MIAT/AKT pathway. Am J Cancer Res 2020;10:838-55. [PubMed]
- Yun S, Han YS, Lee JH, et al. Enhanced Susceptibility to 5-Fluorouracil in Human Colon Cancer Cells by Silencing of GRP78. Anticancer Res 2017;37:2975-84. [PubMed]
- Hsu HH, Chen MC, Baskaran R, et al. Oxaliplatin resistance in colorectal cancer cells is mediated via activation of ABCG2 to alleviate ER stress induced apoptosis. J Cell Physiol 2018;233:5458-67. [Crossref] [PubMed]
- Lin Y, Jiang M, Chen W, et al. Cancer and ER stress: Mutual crosstalk between autophagy, oxidative stress and inflammatory response. Biomed Pharmacother 2019;118:109249. [Crossref] [PubMed]
- Rousseaux S, Debernardi A, Jacquiau B, et al. Ectopic activation of germline and placental genes identifies aggressive metastasis-prone lung cancers. Sci Transl Med 2013;5:186ra66. [Crossref] [PubMed]
- Yamauchi M, Yamaguchi R, Nakata A, et al. Epidermal growth factor receptor tyrosine kinase defines critical prognostic genes of stage I lung adenocarcinoma. PLoS One 2012;7:e43923. [Crossref] [PubMed]
- Der SD, Sykes J, Pintilie M, et al. Validation of a histology-independent prognostic gene signature for early-stage, non-small-cell lung cancer including stage IA patients. J Thorac Oncol 2014;9:59-64. [Crossref] [PubMed]
- Botling J, Edlund K, Lohr M, et al. Biomarker discovery in non-small cell lung cancer: integrating gene expression profiling, meta-analysis, and tissue microarray validation. Clin Cancer Res 2013;19:194-204. [Crossref] [PubMed]
- de Leeuw R, McNair C, Schiewer MJ, et al. MAPK Reliance via Acquired CDK4/6 Inhibitor Resistance in Cancer. Clin Cancer Res 2018;24:4201-14. [Crossref] [PubMed]
- Duan X, Chan C, Han W, et al. Immunostimulatory nanomedicines synergize with checkpoint blockade immunotherapy to eradicate colorectal tumors. Nat Commun 2019;10:1899. [Crossref] [PubMed]
- Ali N, Allam H, May R, et al. Hepatitis C virus-induced cancer stem cell-like signatures in cell culture and murine tumor xenografts. J Virol 2011;85:12292-303. [Crossref] [PubMed]
- Guzy RD, Hoyos B, Robin E, et al. Mitochondrial complex III is required for hypoxia-induced ROS production and cellular oxygen sensing. Cell Metab 2005;1:401-8. [Crossref] [PubMed]
- Cubillos-Ruiz JR, Silberman PC, Rutkowski MR, et al. ER Stress Sensor XBP1 Controls Anti-tumor Immunity by Disrupting Dendritic Cell Homeostasis. Cell 2015;161:1527-38. [Crossref] [PubMed]
- Vladykovskaya E, Sithu SD, Haberzettl P, et al. Lipid peroxidation product 4-hydroxy-trans-2-nonenal causes endothelial activation by inducing endoplasmic reticulum stress. J Biol Chem 2012;287:11398-409. [Crossref] [PubMed]
- Zhang J, Zhang X, Zhao X, et al. DKK1 promotes migration and invasion of non-small cell lung cancer via β-catenin signaling pathway. Tumour Biol 2017;39:1010428317703820. [Crossref] [PubMed]
- Xiao G, Wang P, Zheng X, et al. FAM83A-AS1 promotes lung adenocarcinoma cell migration and invasion by targeting miR-150-5p and modifying MMP14. Cell Cycle 2019;18:2972-85. [Crossref] [PubMed]
- Zhang G, Jiang G, Wang C, et al. Decreased expression of microRNA-320a promotes proliferation and invasion of non-small cell lung cancer cells by increasing VDAC1 expression. Oncotarget 2016;7:49470-80. [Crossref] [PubMed]
- Liu C, Li Z, Wang S, et al. FUT4 is involved in PD-1-related immunosuppression and leads to worse survival in patients with operable lung adenocarcinoma. J Cancer Res Clin Oncol 2019;145:65-76. [Crossref] [PubMed]
- Yan W, Yu H, Li W, et al. Plk1 promotes the migration of human lung adenocarcinoma epithelial cells via STAT3 signaling. Oncol Lett 2018;16:6801-7. [Crossref] [PubMed]
- Che D, Wang M, Sun J, et al. KRT6A Promotes Lung Cancer Cell Growth and Invasion Through MYC-Regulated Pentose Phosphate Pathway. Front Cell Dev Biol 2021;9:694071. [Crossref] [PubMed]
- Wang W, Sun R, Zeng L, et al. GALNT2 promotes cell proliferation, migration, and invasion by activating the Notch/Hes1-PTEN-PI3K/Akt signaling pathway in lung adenocarcinoma. Life Sci 2021;276:119439. [Crossref] [PubMed]