Autophagy related DNA methylation signature predict clinical prognosis and immune microenvironment in low-grade glioma
Introduction
Glioma is the most usual primary malignant tumor of the central nervous system with a worse clinical prognosis. According to the 2016 World Health Organization (WHO) Classification of Tumors of the Central Nervous System, it is divided into grades I–IV, of which grades II–III are named diffuse low-grade gliomas (LGG) (1,2). The prognosis of LGGs is significantly different, some patients relapse into recurrent high-grade gliomas, while other patients have longer progression-free survival after treatment (3). LGG appears diffuse and infiltrative, often closely associated with important functional areas of the brain. Complete surgical resection is difficult, and despite the continuous advances in postoperative radiotherapy and chemotherapy in the past few decades, the clinical prognosis has not improved significantly (4). Novel treatment and potential targets need to be further detected.
Epigenetic modification refers to changing the expression of key genes in the development of tumors without transforming the DNA sequence, including the loss of tumor suppressor genes and the abnormal activation of proto-oncogenes (5). Various epigenetic mechanisms, including DNA methylation, histone modifications, and noncoding RNAs, were proved to regulate tumorigenesis, progression, and treatment in multiple tumors (6). DNA methylation is the most widely studied epigenetic modification, and abnormal DNA methylation can be found in a variety of tumors, including gliomas (7,8). DNA methylation can affect the changes in various biological behaviors and signaling pathways in immune cells, normal cells and tumor cells, which has a faster regulation speed compared with changes in genome sequences. It is an important target for effective tumor therapy.
Autophagy is a highly conserved cellular dynamic self-digestion process, which mainly includes the formation of autophagosomes, the fusion and degradation of autophagosomes and lysosomes (9). Autophagy is beneficial to regulate the energy metabolism of cells, eliminate damaged organelles and misfolded proteins, can maintain the stability of the intracellular environment (10). The occurrence of excessive autophagy can cause type II programmed cell death (11). Autophagy plays a two-sided role in the tumor field, not only regulating the death of tumor cells, but also maintaining the homeostasis of tumor cells to face various stress. Multiple autophagy-related genes (ARGs) in glioma have been shown to be closely related to the proliferation, invasion, metastasis and apoptosis of glioma cells (12,13). However, the detailed regulatory mechanism of autophagy in glioma and the effect of ARGs on the prognosis of LGG patients in actual clinical practice are still unclear. ARGs are often regulated by epigenetic modifications at both the transcriptional and translational levels, and DNA methylation of autophagy regulatory elements plays an important role in LGG. For example, methylation silencing of the ARG ULK2 is critical for astrocyte transformation and tumor growth (14). Epigenetic modification of autophagy is an important direction in cancer and cancer therapeutics (15).
The heterogeneity of the tumor microenvironment (TME) in LGGs is currently a major obstacle to clinical treatment (16). Changes in the autophagy pathway of immune cells and tumor cells in the microenvironment affect tumor progression and therapy (17). Tumor cells can escape from immunity by suppressing immune responses and reducing immunogenicity (18,19). Zhan et al. reported that the autophagy pathway mediates tumor progression by regulating immune responses including immune cell function and cytokine production (20). Therefore, targeting autophagy to modulate changes in the glioma immune microenvironment has great potential to combat LGGs and predict prognosis. At present, there are few studies on the effect of changes in the methylation level of ARGs on the tumor immune microenvironment, and the definite mechanism remains unexplored. This study preliminarily explored the effect of DNA methylation on ARGs, screened ARGs methylation levels related to prognosis, constructed a risk prognosis model, and analyzed the impact of the model on the tumor immune microenvironment. This study helps to decipher the possible mechanism of the epigenetic modification of autophagy on prognosis and treatment sensitivity, and provides a new research target for the prognosis prediction and treatment of LGG. We present the following article in accordance with the TRIPOD reporting checklist (available at https://tcr.amegroups.com/article/view/10.21037/tcr-22-310/rc).
Methods
Data of LGG collecting from TCGA and pretreatment
The DNA methylation data (Illumina HumanMethylation450 BeadChip), gene expression profile and corresponding clinical information of LGG patients were originated from The Cancer Genome Atlas (TCGA; https://portal.gdc.cancer.gov/) and MEXPRESS (21,22). The workflow of this article was depicted in Figure 1. The collection criteria for LGG were still in accordance with the 2016 WHO classification of central nervous system tumors. By matching DNA methylation data and expression profile, and removing samples with incomplete clinical information, we finally collected 451 LGG samples. The average β value of multiple cg sites of one gene were used as the methylation level of the gene. The study was conducted in accordance with the Declaration of Helsinki (as revised in 2013).
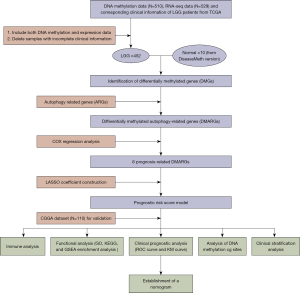
Screening of ARGs whose methylation levels associating with prognosis [prognosis-related differentially methylated ARGs (DMARGs)]
Differentially methylated genes (DMGs) of normal and LGG patients were obtained from DiseaseMeth version, which were screened by ‘limma’ package with the standard of |log2fold change (FC)| >0.2 and P<0.05. The datasets of ARGs were downloaded from Human Autophagy Database (HADb; http://autophagy.lu/clustering/index.html). Selected DMARGs associated with prognosis by COX regression analysis (23). The intersections of these results were showed by Venn diagram.
Construction and validation of the prognostic risk model
The prognostic risk score model was constructed by least absolute shrinkage and selection operator (LASSO) coefficient and the corresponding relative methylation level by formula: risk score = sum (the relative methylation level × corresponding coefficient) (24). The risk score of each patient was calculated and divided into high- and low-risk groups by median risk score. The Kaplan-Meier (KM) survival curve was used to contrast the difference in prognosis between the two groups. The ROC curve and the Chinese Glioma Genome Atlas (CGGA) database data was introduced as a validation set to assess the performance of this prognostic signature.
Functional enrichment in high- and low-risk groups
R packages ‘org.Hs.eg.db, clusterProfiler’ were applied to perform Gene Ontology (GO) and Kyoto Encyclopedia of Genes and Genomes (KEGG) functional enrichment analysis on the differentially expressed genes of high- and low-risk groups, the standard is P<0.05. Gene set enrichment analysis (GSEA) software (version 4.1.0) was used to analyze enrichment results on two groups based on hallmark gene sets (H). Normalized enrichment score |NES| >1.5 and nominal (NOM) P<0.05 was considered statistical significance.
Distinctions in the immune microenvironment analysis between two groups
To explore the potential differences of immune microenvironment in two groups, the ESTIMATE algorithm was used to calculate the immune scores, stromal scores of the high- and low-risk groups (25). The Cibersort algorithm which calculated enrichment of different immune cells by deconvolution algorithm, was applied to examine distribution of 22 types of immune infiltrating cells (26). We also examined gene expression of inhibitory immune checkpoints to assess the effect of immunotherapy.
Analysis of DNA methylation cg sites
To further explore the impact of methylation sites on gene expression and prognosis, the heatmap showed the relationship between different cg sites of 6 DMARGs and clinical features. The correlation between DNA methylation cg sites and the relative expression of the corresponding genes were examined by Pearson correlation analysis.
Statistical analysis
Statistical analysis and graphing were performed using R language (version 4.1), GraphPad Prism (version 8.0.1) and Adobe Illustrator 2019. Differences between the two groups were tested by t-test, analysis of variance (ANOVA) was used to test the significance of the difference of more than two samples, and log-rank test was used for survival curves. P<0.05 was regarded as statistically significant.
Results
Identification of DMARGs
The ARG set was downloaded from HADb, and 35 differential methylation of ARGs (28 down-regulated, 7 up-regulated) were extracted between the normal tissue and LGG patients (|log2FC|>0.1, P<0.05, from DiseaseMeth). The results were displayed in the heatmap and volcano plot (Figure 2A,2B). STRING network showed the association of 35 DMARGs (Figure 2C) and 21 prognosis related DMARGs (Figure 2D). Through univariate and multivariate COX regression analysis, we finally screened out 6 DMARGs (ARSB, CFLAR, WIPI2, RB1, ERN1, RAB24) for constructing prognostic signature (Figure 2E). Venn diagrams revealed that the intersections were used for further analysis (Figure 2F).
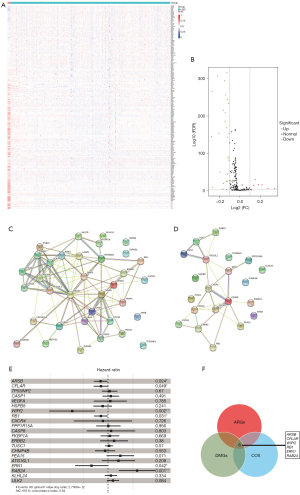
Establishment and validation of survival prognostic model with DMARGs in LGG cohort
The regression coefficient of 6 DMARGs was determined by LASSO COX regression analyses. According to coefficient values and corresponding methylation values of 6 DMARGs obtained by multivariate COX regression analysis, we calculated the risk score of LGG patients. The risk score = (5.7092 × methylation level of RAB24) + (−1.1684 × methylation level of ARSB) + (−4.3193 × methylation level of WIPI2) + (−1.4733 × methylation level of RB1) + (−3.2461 × methylation level of ERN1) + (−1.4838 × methylation level of CFLAR). Patients were divided into high- and low-risk groups according to the median of risk score (Figure 3A). The survival status of each patient was shown in Figure 3B, and the number of patients who died increased with the risk score increasing. The heatmap (Figure 3C) showed methylation levels of 6 DMARGs between high- and low-risk groups. KM survival analysis (Figure 3D) showed that the survival prognosis of patients in the high-risk group was significantly worse (log-rank P=3E-10). The prediction accuracy of the model was evaluated using the receiver operating characteristic (ROC) curve, and the results showed that the model had good prediction accuracy (Figure 3E), with the best effect at 30 months [area under the curve (AUC)max =0.96]. The analysis of CGGA methylation data as a validation dataset showed similar results, proving that the prognostic signature has a good effect on the prognosis assessment of LGG (Figure 3F,3G). The nomogram based on risk score and other clinical information predicted 1-, 2-, and 3-year prognosis of LGG patients (Figure 3H).
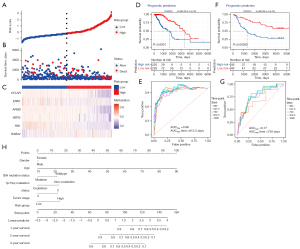
The relationship between risk scores of prognostic model and clinical features
The heatmap combined the clinical information of the patients [age, tumor stage, isocitrate dehydrogenase (IDH) mutation status, 1p/19q co-deletion status] to display the methylation levels and mRNA expression of the 6 DMARGs (Figure 4A,4B). ARSB, CFLAR, WIPI2, RB1, and ERN1 were hypermethylated in the low-risk group, which were protective factors, and RAB24 was hypomethylated in the low-risk group, which was a risk factor for the prognosis of LGG. The 6 DMARGs were divided into hypermethylated and hypomethylated groups according to their methylation levels, and the KM survival curves showed prognostic differences (Figure 4C). Pearson correlation analysis showed the relationship between the overall methylation levels of the 6 DMARGs and the corresponding mRNA expression (Figure 4D).
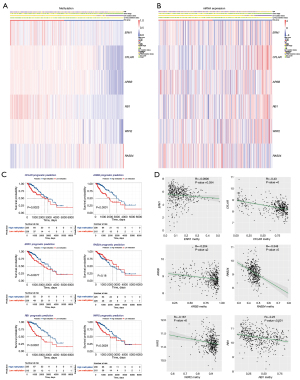
Function enrichment analysis between low- and high-risk groups
To analyze the biological functions of DEGs in the low- and high-risk groups, we performed functional enrichment analysis. GO enrichment analysis revealed that the down-regulated DEGs of high-risk group were significantly enriched in terms of skeletal system development, antigen processing and presentation via major histocompatibility complex (MHC) class II, extracellular matrix organization in biological process (BP), MHC class II protein complex, collagen-containing extracellular matrix, MHC protein complex in cellular component (CC) and MHC class II receptor activity, extracellular matrix structural constituent, immune receptor activity in molecular function (MF) (Figure 5A). The KEGG enrichment analysis showed that DEGs mainly involved in infection and immune diseases, immune cell differentiation and antigen processing and presentation (Figure 5B). GSEA enrichment analysis showed that DNA repair, G2M checkpoint, E2F targets, P53 pathway, and glycolysis were enriched in high-risk group in hallmark gene sets (Figure 5C).
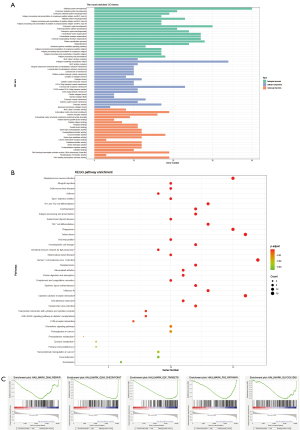
Differences in the immune microenvironment of two groups
The enrichment analysis results demonstrated that the risk assessment model constructed by DMARGs was closely related to the tumor immune microenvironment. Immunotherapy targeting immune checkpoints is an important direction for current tumor treatment. Therefore, we detected the expression of 7 common immune checkpoints (PD-1, PD-L1, PD-L2, LAG3, TIM3, B7H3, CTLA4) between the high- and low-risk groups. The results showed that the expression of immune checkpoints in the high-risk group was generally increased, which was more conducive to the immune escape of tumors (Figure 6A). The ESTIMATE algorithm revealed that the immune, stromal, and ESTIMATE scores of the high-risk group were significantly higher than those of the low-risk group (Figure 6B). In addition, we used the CIBERSORT algorithm to calculate the abundance of 22 types of immune cells in LGG patients, as well as the differences in the degree of immune cell infiltration between high- and low-risk groups. The results showed that compared with the low-risk group, resting memory CD4 T cells, regulatory T cells (Tregs), resting NK cells, M2 macrophages, resting mast cells significantly increased, Plasma cells, naive CD4 T cells, and activated mast cells reduced in the high-risk group (Figure 6C,6D).
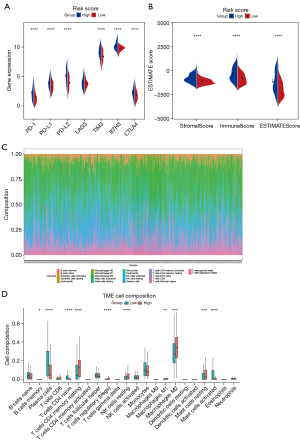
Associations between cg sites of methylations and expressions of the 6 DMARGs
To further explore the role of each cg site in DMARGs, as shown in Figure 7A, the heatmap showed the methylation levels of each cg site in 6 DMARGs between high- and low-risk groups. Pearson correlation analysis showed the relationship between each cg sites and the relative expression of the corresponding gene, clinical information (gender, histological type, tumor location) (Figure 7B).
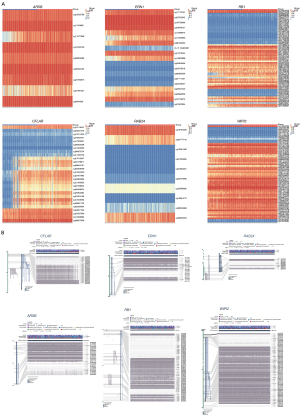
Discussion
LGGs were prone to relapse to higher pathological grades of gliomas. Although a variety of therapeutic measures had been used in clinical practice in the past years, the survival expectations of patients with LGGs were still not optimistic (3,27). The clinical prognosis and therapeutic effects of gliomas are quite different, and the current histopathological classification could not meet the needs. Classification based on genomic changes and epigenetic differences will help us to identify carcinogenic factors, prognostic markers and knowledge of treatments in LGGs (28,29). IDH mutation, 1p/19q co-deletion, TERT promoter mutation and MGMT methylation have been certified to be prognostic biomarkers (30,31). Therefore, screening new biomarkers that can predict prognosis and serve as therapeutic targets is an important direction for LGG research (32).
The factors mediating tumor progression were complex, and epigenetic modification was considered to be an important part of tumor development and treatment (33). Epigenetics referred to heritable changes in gene expression that are not accompanied by changes in DNA sequence, including DNA methylation, chromatin remodeling, and noncoding RNAs. Many studies had shown that DNA methylation was significantly different in normal cells and different subtypes of gliomas, and extensive hypermethylation and hypomethylation of different CpG islands are characteristic of glioma (34). For example, Wang et al. demonstrated that hypermethylation of the miR-338-5p promoter regulated the aberrant expression of ETS-1, which was a key regulator of tumor cell proliferation and invasion, and was associated with prognosis of astrocytoma patients (35). There was also a public database-based bioinformatics analysis showing that PODNL1 methylation acted as a prognostic biomarker and correlates with immune infiltration and immune checkpoints in LGG (36). da Costa Rosa et al. evaluated the effect of a DNA demethylating agent combined with all-trans retinoic acid in IDH1 mutant gliomas, and the combination of 5-Aza and atRA reduced the growth of IDH1 R132H glioma cells and increased differentiation and apoptosis (37). Therefore, the methylation level of various genes in glioma cells is a standard biomarker for predicting tumor prognosis (38).
On the other hand, the role of ARG methylation in LGG was still not fully understood. Autophagy played a dual role in LGG with different subtypes, stages and gene expression, could either promote tumor cell survival or cause tumor cell death (39). The epigenetic regulation of autophagy was very complex and regarded as a critical role in tumor progression. For example, the generation of cancer stem cells depends on autophagy, which helps to improve the viability of tumor cells, leading to drug resistance and recurrence of tumors (40). Epigenetic modifications of autophagy were also involved in multiple tumor-related signaling pathways. Some modifications can reduce tumorigenicity by inhibiting proliferation and metastasis, promoting apoptosis and lethal autophagy, improving therapeutic response and achieving a better prognosis. One study had confirmed that the down-regulation of HIF1A gene in medulloblastoma cells leads to a significant increase in the methylation of the ATG16L1 promoter and a significant decrease in the methylation of the EPAS1 promoter, which decrease cell proliferation (41). In addition, the development and drug resistance of tumors are also related to the epigenetic modification of autophagy. Silencing of MAP1LC3Av1 methylation might attenuate autophagy and promote the occurrence of gastric cancer (42). MicroRNA-20a-5p inhibited autophagy and reduced cisplatin resistance by regulating DNMT3B-mediated RBP1 DNA methylation in ovarian cancer (43). Long non-coding RNA H19 induced autophagy activation by reducing Beclin1 promoter methylation through the SAHH/DNMT3B axis, leading to tamoxifen resistance in breast cancer (44). Therefore, abnormal epigenetic modification of autophagy regulators was a key factor in regulating tumor progression, epigenetic modification is reversible, and abnormal epigenetic modification state could be restored to normal state by treatment (45). The epigenetic regulation of autophagy was also related to the glioma immune microenvironment (46).
Tumor cells had a variety of immune evasion mechanisms. Clinical immunotherapy was limited by the difference in the tumor immune microenvironment. Various signaling pathways affected the changes in the autophagy of immune cells and tumor cells in the TME (47). Autophagy could affect the proliferation, antigen presentation and function of immune cells, in addition, tumor cells could reduce the immune response by producing abnormal immune checkpoint signals, resulting in immune escape (48,49). PD-1 was an important T cell suppressor, and its binding to PD-L1 of tumor cells could significantly inhibit the proliferation and activity of cytotoxic T cells, resulting in immune escape of tumor cells. Study had shown that autophagy enables pancreatic cancer cells to perform immune evasion by degrading MHC I, and autophagy inhibition reverses immune evasion in animal models of pancreatic cancer (50). Yang et al. demonstrated that knockdown of m6A mRNA demethylase FTO increased m6A methylation in PD-1 (PDCD1), CXCR4 and SOX10 in melanoma cells, regulating melanoma tumorigenicity and sensitive to anti-PD-1 therapy (51). A study proved that highly selective HDAC6 inhibitors promote HDAC6 degradation in glioblastoma and induce antitumor immune responses by inhibiting autophagy (52). Therefore, utilizing the immune microenvironment was an important way to treat tumors including gliomas. Autophagy could regulate the immune microenvironment of tumors, and regulating autophagy might improve the effectiveness of immunotherapy.
In this study, LGG patients were collected from the public database, and DMARGs between normal tissues and LGGs were screened. COX regression analysis was performed on the DMARG set, and 6 DMARGs (ARSB, CFLAR, WIPI2, RB1, ERN1, RAB24) associated with prognosis were further screened to construct a risk assessment model. The median of the risk score was used as the cut-off point to divide into high- and low-risk group, and the KM survival curve proved that the survival prognosis of the high-risk group was poorer. At the same time, the ROC curve (AUC =0.96) proved the excellent prediction effect of the model. In addition, we demonstrated that risk score is an important factor affecting the prognosis of LGGs through a nomogram prediction model. The results of GO and KEGG enrichment analysis suggested differences in the immune microenvironment between high- and low-risk groups. We found that the expression of inhibitory immune checkpoints was significantly elevated in the high-risk group, which was beneficial for tumor immune escape. The immune infiltration scores and immune cell infiltration were also significantly different between high- and low-risk groups, providing a theoretical basis for the clinical application of immunotherapy. However, LGG sometimes were considered to be immunologically cold tumors, calculation of immune cell infiltration using CIBERSORT might be biased. The true differences between the two groups still needs further verification. Finally, we analyzed the relationship between gene methylation cg sites and the relative expression of corresponding genes, and further explored the methylation sites of ARGs that affect the prognosis of LGG patients.
Autophagy was an important factor in regulating the tumor immune microenvironment, the 6 ARGs in this model had also been reported to be related to the immune response of tumors. The innate immune response was regulated by toll-like receptors (TLRs), and dysregulation of TLRs’ function could occur in multiple malignant tumors. West et al. found that the expression of TLR2 was significantly increased in gastric cancer, and the DNA expression matrix showed that the growth response of human gastric cancer cells induced by TLR2 was associated with the up-regulation of the anti-apoptotic gene CFLAR (53). Meanwhile, Plaza-Sirvent et al. showed that the low expression of CFLAR would contribute to high apoptosis rate of Treg, which was important for regulating immune responses and might be a target for tumor immunotherapy (54). A study had shown that dysregulation of RAB24 is closely related to oncogenic genetic alterations of MXD3, which mediate immune cell dysfunction in a variety of tumors (55). Guo et al. demonstrated cocaine-induced autophagy could reduce microglia activation and the release of inflammatory factors, while the activation of ERN1-dependent endoplasmic reticulum stress pathway was involved in this induction of autophagy (56). Liao et al. reported rVP1 increased the formation of LC3-associated autophagosomes via WIPI1 and WIPI2 and promotes the migration of macrophages, which was important for regulating immune responses and antitumor activity. Knockdown of WIPI1 and WIPI2 could inhibited rVP1-mediated autophagy and reduce MAPK1/3 phosphorylation and activation of MMP9 (57). A recent study showed that SNX5 initiated autophagy during viral infection via recruitment of WIPI2 to regulate intracellular immune and inflammatory responses (58). Regrettably, the impact of these 6 ARGs on the immune microenvironment remained to be studied in LGG because there were few related studies in LGG.
However, risk assessment models based on bioinformatics still had certain limitations. The genes used to build prognostic models from public databases had only statistically significant associations, and their interactions cannot be confirmed for the time being. In this study, the biological functions and signaling pathways of genes still need to be verified in vivo and in vitro experiments, and their clinical significance also needs to be verified in a large-scale multi-center clinical cohort.
Conclusions
In this study, a total of 6 DMARG associated with prognosis were screened. The methylation level of these genes could be used to predict prognosis of LGG patients and have a close relationship with immune microenvironment of tumor. This study further demonstrated the link between clinical prognosis, TME and methylation of ARG, providing a theoretical basis for the study of epigenetic regulation of autophagy. The results should be verified by more experiments.
Acknowledgments
Funding: This work was supported by Natural Science Foundation of China (Grant No. 81771270 to QP).
Footnote
Reporting Checklist: The authors have completed the TRIPOD reporting checklist. Available at https://tcr.amegroups.com/article/view/10.21037/tcr-22-310/rc
Peer Review File: Available at https://tcr.amegroups.com/article/view/10.21037/tcr-22-310/prf
Conflicts of Interest: All authors have completed the ICMJE uniform disclosure form (available at https://tcr.amegroups.com/article/view/10.21037/tcr-22-310/coif). QP reports that this work was supported by the National Natural Science Foundation of China (81771270). The other authors have no conflicts of interest to declare.
Ethical Statement: The authors are accountable for all aspects of the work in ensuring that questions related to the accuracy or integrity of any part of the work are appropriately investigated and resolved. The study was conducted in accordance with the Declaration of Helsinki (as revised in 2013).
Open Access Statement: This is an Open Access article distributed in accordance with the Creative Commons Attribution-NonCommercial-NoDerivs 4.0 International License (CC BY-NC-ND 4.0), which permits the non-commercial replication and distribution of the article with the strict proviso that no changes or edits are made and the original work is properly cited (including links to both the formal publication through the relevant DOI and the license). See: https://creativecommons.org/licenses/by-nc-nd/4.0/.
References
- Louis DN, Perry A, Reifenberger G, et al. The 2016 World Health Organization Classification of Tumors of the Central Nervous System: a summary. Acta Neuropathol 2016;131:803-20. [Crossref] [PubMed]
- Wesseling P, Capper D. WHO 2016 Classification of gliomas. Neuropathol Appl Neurobiol 2018;44:139-50. [Crossref] [PubMed]
- Feng E, Liang T, Wang X, et al. Correlation of alteration of HLA-F expression and clinical characterization in 593 brain glioma samples. J Neuroinflammation 2019;16:33. [Crossref] [PubMed]
- Ostrom QT, Cioffi G, Waite K, et al. CBTRUS Statistical Report: Primary Brain and Other Central Nervous System Tumors Diagnosed in the United States in 2014-2018. Neuro Oncol 2021;23:iii1-iii105. [Crossref] [PubMed]
- Huo M, Zhang J, Huang W, et al. Interplay Among Metabolism, Epigenetic Modifications, and Gene Expression in Cancer. Front Cell Dev Biol 2021;9:793428. [Crossref] [PubMed]
- Yu M, Hazelton WD, Luebeck GE, et al. Epigenetic Aging: More Than Just a Clock When It Comes to Cancer. Cancer Res 2020;80:367-74. [Crossref] [PubMed]
- Jiang MC, Ni JJ, Cui WY, et al. Emerging roles of lncRNA in cancer and therapeutic opportunities. Am J Cancer Res 2019;9:1354-66. [PubMed]
- Nishiyama A, Nakanishi M. Navigating the DNA methylation landscape of cancer. Trends Genet 2021;37:1012-27. [Crossref] [PubMed]
- Dikic I, Elazar Z. Mechanism and medical implications of mammalian autophagy. Nat Rev Mol Cell Biol 2018;19:349-64. [Crossref] [PubMed]
- Qiang L, Sample A, Shea CR, et al. Autophagy gene ATG7 regulates ultraviolet radiation-induced inflammation and skin tumorigenesis. Autophagy 2017;13:2086-103. [Crossref] [PubMed]
- Schwartz LM. Autophagic Cell Death During Development - Ancient and Mysterious. Front Cell Dev Biol 2021;9:656370. [Crossref] [PubMed]
- Chen Y, Zhang Z, Henson ES, et al. Autophagy inhibition by TSSC4 (tumor suppressing subtransferable candidate 4) contributes to sustainable cancer cell growth. Autophagy 2022;18:1274-96. [Crossref] [PubMed]
- Xu Q, Zhang H, Liu H, et al. Inhibiting autophagy flux and DNA repair of tumor cells to boost radiotherapy of orthotopic glioblastoma. Biomaterials 2022;280:121287. [Crossref] [PubMed]
- Shukla S, Patric IR, Patil V, et al. Methylation silencing of ULK2, an autophagy gene, is essential for astrocyte transformation and tumor growth. J Biol Chem 2014;289:22306-18. [Crossref] [PubMed]
- Peeters JGC, Picavet LW, Coenen SGJM, et al. Transcriptional and epigenetic profiling of nutrient-deprived cells to identify novel regulators of autophagy. Autophagy 2019;15:98-112. [Crossref] [PubMed]
- Ribeiro Franco PI, Rodrigues AP, de Menezes LB, et al. Tumor microenvironment components: Allies of cancer progression. Pathol Res Pract 2020;216:152729. [Crossref] [PubMed]
- Poillet-Perez L, Sarry JE, Joffre C. Autophagy is a major metabolic regulator involved in cancer therapy resistance. Cell Rep 2021;36:109528. [Crossref] [PubMed]
- Tsiouplis NJ, Bailey DW, Chiou LF, et al. TET-Mediated Epigenetic Regulation in Immune Cell Development and Disease. Front Cell Dev Biol 2021;8:623948. [Crossref] [PubMed]
- Pan X, Zheng L. Epigenetics in modulating immune functions of stromal and immune cells in the tumor microenvironment. Cell Mol Immunol 2020;17:940-53. [Crossref] [PubMed]
- Zhan Z, Xie X, Cao H, et al. Autophagy facilitates TLR4- and TLR3-triggered migration and invasion of lung cancer cells through the promotion of TRAF6 ubiquitination. Autophagy 2014;10:257-68. [Crossref] [PubMed]
- Koch A, De Meyer T, Jeschke J, et al. MEXPRESS: visualizing expression, DNA methylation and clinical TCGA data. BMC Genomics 2015;16:636. [Crossref] [PubMed]
- Koch A, Jeschke J, Van Criekinge W, et al. MEXPRESS update 2019. Nucleic Acids Res 2019;47:W561-5. [Crossref] [PubMed]
- Linden A, Yarnold PR. Modeling time-to-event (survival) data using classification tree analysis. J Eval Clin Pract 2017;23:1299-308. [Crossref] [PubMed]
- Friedman J, Hastie T, Tibshirani R. Regularization Paths for Generalized Linear Models via Coordinate Descent. J Stat Softw 2010;33:1-22. [Crossref] [PubMed]
- Yoshihara K, Shahmoradgoli M, Martínez E, et al. Inferring tumour purity and stromal and immune cell admixture from expression data. Nat Commun 2013;4:2612. [Crossref] [PubMed]
- Newman AM, Liu CL, Green MR, et al. Robust enumeration of cell subsets from tissue expression profiles. Nat Methods 2015;12:453-7. [Crossref] [PubMed]
- Chang SM, Cahill DP, Aldape KD, et al. Treatment of Adult Lower-Grade Glioma in the Era of Genomic Medicine. Am Soc Clin Oncol Educ Book 2016;35:75-81. [Crossref] [PubMed]
- Cancer Genome Atlas Research Network. Comprehensive, Integrative Genomic Analysis of Diffuse Lower-Grade Gliomas. N Engl J Med 2015;372:2481-98. [Crossref] [PubMed]
- Capper D, Jones DTW, Sill M, et al. DNA methylation-based classification of central nervous system tumours. Nature 2018;555:469-74. [Crossref] [PubMed]
- Jelski W, Mroczko B. Molecular and Circulating Biomarkers of Brain Tumors. Int J Mol Sci 2021;22:7039. [Crossref] [PubMed]
- Oldrini B, Vaquero-Siguero N, Mu Q, et al. MGMT genomic rearrangements contribute to chemotherapy resistance in gliomas. Nat Commun 2020;11:3883. [Crossref] [PubMed]
- Delgado-López PD, Corrales-García EM, Martino J, et al. Diffuse low-grade glioma: a review on the new molecular classification, natural history and current management strategies. Clin Transl Oncol 2017;19:931-44. [Crossref] [PubMed]
- Lu Y, Chan YT, Tan HY, et al. Epigenetic regulation in human cancer: the potential role of epi-drug in cancer therapy. Mol Cancer 2020;19:79. [Crossref] [PubMed]
- de Souza CF, Sabedot TS, Malta TM, et al. A Distinct DNA Methylation Shift in a Subset of Glioma CpG Island Methylator Phenotypes during Tumor Recurrence. Cell Rep 2018;23:637-51. [Crossref] [PubMed]
- Wang J, Huo C, Yin J, et al. Hypermethylation of the Promoter of miR-338-5p Mediates Aberrant Expression of ETS-1 and Is Correlated With Disease Severity Of Astrocytoma Patients. Front Oncol 2021;11:773644. [Crossref] [PubMed]
- Noor H, Zaman A, Teo C, et al. PODNL1 Methylation Serves as a Prognostic Biomarker and Associates with Immune Cell Infiltration and Immune Checkpoint Blockade Response in Lower-Grade Glioma. Int J Mol Sci 2021;22:12572. [Crossref] [PubMed]
- da Costa Rosa M, Yamashita AS, Riggins GJ. Evaluation of a DNA demethylating agent in combination with all-trans retinoic acid for IDH1-mutant gliomas. Neuro Oncol 2022;24:711-23. [Crossref] [PubMed]
- Wu Q, Berglund AE, Etame AB. The Impact of Epigenetic Modifications on Adaptive Resistance Evolution in Glioblastoma. Int J Mol Sci 2021;22:8324. [Crossref] [PubMed]
- Bhol CS, Panigrahi DP, Praharaj PP, et al. Epigenetic modifications of autophagy in cancer and cancer therapeutics. Semin Cancer Biol 2020;66:22-33. [Crossref] [PubMed]
- Das CK, Mandal M, Kögel D. Pro-survival autophagy and cancer cell resistance to therapy. Cancer Metastasis Rev 2018;37:749-66. [Crossref] [PubMed]
- Cruzeiro GAV, Dos Reis MB, Silveira VS, et al. HIF1A is Overexpressed in Medulloblastoma and its Inhibition Reduces Proliferation and Increases EPAS1 and ATG16L1 Methylation. Curr Cancer Drug Targets 2018;18:287-94. [Crossref] [PubMed]
- Muhammad JS, Nanjo S, Ando T, et al. Autophagy impairment by Helicobacter pylori-induced methylation silencing of MAP1LC3Av1 promotes gastric carcinogenesis. Int J Cancer 2017;140:2272-83. [Crossref] [PubMed]
- Li H, Lei Y, Li S, et al. MicroRNA-20a-5p inhibits the autophagy and cisplatin resistance in ovarian cancer via regulating DNMT3B-mediated DNA methylation of RBP1. Reprod Toxicol 2022;109:93-100. [Crossref] [PubMed]
- Wang J, Xie S, Yang J, et al. The long noncoding RNA H19 promotes tamoxifen resistance in breast cancer via autophagy. J Hematol Oncol 2019;12:81. [Crossref] [PubMed]
- Martinez-Useros J, Martin-Galan M, Florez-Cespedes M, et al. Epigenetics of Most Aggressive Solid Tumors: Pathways, Targets and Treatments. Cancers (Basel) 2021;13:3209. [Crossref] [PubMed]
- Xia H, Green DR, Zou W. Autophagy in tumour immunity and therapy. Nat Rev Cancer 2021;21:281-97. [Crossref] [PubMed]
- Clarke AJ, Simon AK. Autophagy in the renewal, differentiation and homeostasis of immune cells. Nat Rev Immunol 2019;19:170-83. [Crossref] [PubMed]
- Jiang T, Chen X, Ren X, et al. Emerging role of autophagy in anti-tumor immunity: Implications for the modulation of immunotherapy resistance. Drug Resist Updat 2021;56:100752. [Crossref] [PubMed]
- Jiang X, Wang J, Deng X, et al. Role of the tumor microenvironment in PD-L1/PD-1-mediated tumor immune escape. Mol Cancer 2019;18:10. [Crossref] [PubMed]
- Yamamoto K, Venida A, Yano J, et al. Autophagy promotes immune evasion of pancreatic cancer by degrading MHC-I. Nature 2020;581:100-5. [Crossref] [PubMed]
- Yang S, Wei J, Cui YH, et al. m6A mRNA demethylase FTO regulates melanoma tumorigenicity and response to anti-PD-1 blockade. Nat Commun 2019;10:2782. [Crossref] [PubMed]
- Liu JR, Yu CW, Hung PY, et al. High-selective HDAC6 inhibitor promotes HDAC6 degradation following autophagy modulation and enhanced antitumor immunity in glioblastoma. Biochem Pharmacol 2019;163:458-71. [Crossref] [PubMed]
- West AC, Tang K, Tye H, et al. Identification of a TLR2-regulated gene signature associated with tumor cell growth in gastric cancer. Oncogene 2017;36:5134-44. [Crossref] [PubMed]
- Plaza-Sirvent C, Schuster M, Neumann Y, et al. c-FLIP Expression in Foxp3-Expressing Cells Is Essential for Survival of Regulatory T Cells and Prevention of Autoimmunity. Cell Rep 2017;18:12-22. [Crossref] [PubMed]
- Wu SY, Lin KC, Lawal B, et al. MXD3 as an onco-immunological biomarker encompassing the tumor microenvironment, disease staging, prognoses, and therapeutic responses in multiple cancer types. Comput Struct Biotechnol J 2021;19:4970-83. [Crossref] [PubMed]
- Guo ML, Liao K, Periyasamy P, et al. Cocaine-mediated microglial activation involves the ER stress-autophagy axis. Autophagy 2015;11:995-1009. [Crossref] [PubMed]
- Liao CC, Ho MY, Liang SM, et al. Recombinant protein rVP1 upregulates BECN1-independent autophagy, MAPK1/3 phosphorylation and MMP9 activity via WIPI1/WIPI2 to promote macrophage migration. Autophagy 2013;9:5-19. [Crossref] [PubMed]
- Dong X, Yang Y, Zou Z, et al. Sorting nexin 5 mediates virus-induced autophagy and immunity. Nature 2021;589:456-61. [Crossref] [PubMed]