A potential EBV-related classifier is associated with the efficacy of immunotherapy in gastric cancer
Introduction
Gastric cancer (GC) is a global health problem and the third leading cause of cancer death worldwide (1-3). The Cancer Genome Atlas (TCGA) Research Network recognized four distinct molecular subtypes of GC in 2014, including Epstein-Barr virus (EBV)-positive, microsatellite instable (MSI), genomically stable (GS), and chromosomal instable (CIN) GC (4). EBV-positive GC (EBVaGC) is defined by EBV infection in cancer cells, which accounts for approximately 9% of all GC cases (5,6). Compared to EBV-negative GC (EBVnGC) patients, those infected patients have a favorable survival (7,8), which suggests a unique characteristic of EBVaGC.
Therapeutic strategies for GC remain limited, and immunotherapies, such as programmed cell death 1 (PD-1) inhibitors, only benefit a few GC patients. Previous research observed dramatic responses of EBVaGC patients after immunotherapy. Several studies reported that patients with EBV-positive tumors derived longer clinical benefits with anti-programmed cell death-ligand 1 (PD-L1) antibody treatments (9-13). This beneficial effect may be associated with the active immune microenvironment induced by EBV infection. Compared to EBV-negative tumors, EBV-positive status induces a tumor microenvironment with ample immune cell infiltration and elevated expression of immune response genes (5,14-16). However, the effect of immunotherapy in EBVaGC is inconsistent, and no predictive biomarkers for effectiveness were reported (7,11,14). Therefore, it is necessary to explore EBV infection-induced changes of immune status and the tumor microenvironment and identify potential biomarkers for the selection of EBVaGC patients who might obtain greater benefits from immunotherapy.
To explain the distinct molecular features of EBV-positive status, we first employed differential gene expression analysis by comparing RNA-seq of EBVaGC patients and EBVnGC patients in the TCGA-Stomach Adenocarcinoma (TCGA-STAD) cohort and generated a co-expression network using the weighted gene co-expression network analysis (WGCNA) method. Subsequently, we further performed Gene Ontology (GO) enrichment analysis and Kyoto Encyclopedia of Genes and Genomes (KEGG) pathway analysis to investigate the functions of these differentially expressed genes (DEGs). To explore the EBV infection-induced changes in immune status, the “Estimation of Stromal and Immune cells in Malignant Tumours using Expression data” (ESTIMATE) and CIBERSORT algorithms were used to evaluate the tumor microenvironment compositions in each tumor tissue sample. Notably, we identified a potential classifier related to the efficacy of immunotherapy. Our results suggested that this classifier may be used as a potential biomarker to identify patients with GC who are most likely to benefit from immunotherapy. We present the following article in accordance with the TRIPOD reporting checklist (available at https://tcr.amegroups.com/article/view/10.21037/tcr-22-461/rc).
Methods
Data resources and pre-processing
In this study, we utilized 3 different datasets for analysis. TCGA-STAD was defined as the discovery dataset, including 375 tumor samples and 32 normal samples. The RNA-seq data at the count and Fragments Per Kilobase per Million (FPKM) levels were downloaded through the “TCGAbiolinks” (17) R package. Count data were used for differential gene expression analysis through the “DESeq2” (18) R package, and the FPKM values were then transformed into Transcripts Per Kilobase Million (TPM) values for subsequent analysis. Genome annotation files were downloaded from Gencode (http://ftp.ebi.ac.uk/pub/databases/gencode/Gencode_human/release_22/gencode.v22.annotation.gtf.gz) to transform the Ensembl ID to gene symbols and separate protein-coding genes. EBV information was obtained as described in a previous publication (19), which included 263 GC patients. We then took the intersection between 375 tumor samples and 263 samples that had EBV information and obtained a total of 228 tumor samples that contained exact EBV information (including 207 EBV-negative tumor samples and 21 EBV-positive tumor samples) for subsequent analyses.
The second dataset was from our hospital, named the Sun Yat-sen University Cancer Center (SYSUCC) cohort. A total of 137 fresh-frozen tumor tissues, including 70 archived EBV-positive GC specimens and 67 EBV-negative tissue samples, were obtained from GC patients who underwent surgical resection at SYSUCC. The clinicopathological characteristics of the patients were summarized in Table S1. The Institutional Research Ethics Committee of SYSUCC approved the study.
The last dataset was a GC cohort that received anti-PD-1 therapy (11). We downloaded the transcriptome fastq files of this dataset from the European Nucleotide Archive through accession PRJEB25780. FastQC v0.11.9 software and MultiQC v1.0.dev0 (20) software were used to examine the quality of reads, and fastp v0.23.1 (21) software was used to trim reads with low quality. STAR v2.7.9a (22) software was applied to align the reads to the human reference genome (hg38), and RSEM v1.3.3 (23) software was employed to quantify the expression of genes and transcripts.
Differential gene expression analysis
TCGA-STAD RNA-seq data at the count level was applied for differential gene expression analysis by the “DESeq2” (18) R package. To identify DEGs between the EBV-positive group and EBV-negative group, we set the cutoff as |log2FC| >1.5 and adjusted P value <0.05. Only genes that met this filtering criterion were considered as the DEGs, and we generated a volcano plot to visualize these DEGs using the “ggplot2” R package.
Weighted gene co-expression network analysis
To investigate the relationship between the expression of DEGs and clinical EBV phenotype, we performed weighted gene co-expression network analysis through the “WGCNA” (24) R package. Firstly, the expression matrix of DEGs was set as the input file, and Pearson correlations between each gene and the other genes were calculated to generate a gene-gene correlation matrix. After that, the optimal solution of the parameter β of the adjacency function was determined, and the adjacency matrix was constructed to make the gene-gene correlation coefficient matrix meet the requirements of a scale-free network. Next, we constructed a topological overlap measure (TOM) matrix. In addition to simply considering the direct relationship of genes in pairs, the TOM matrix can also be more in line with the mutual effects of genes in the organism. Finally, we converted the TOM matrix to a dissimilarity matrix and used the dynamic cut tree method to perform hierarchical clustering and construct the gene modules. The minimal gene number in each module was set as 50. We correlated these gene modules with the clinical EBV feature to determine the best correlation module with the EBV phenotype.
Functional enrichment analysis
GO functional enrichment analysis and KEGG pathway analysis for genes in the selected module were performed using the “clusterProfiler” (25) R package. The function “dotplot” was used to visualize the enrichment results. An adjusted P value <0.05 was considered statistically significant.
Estimation of the tumor microenvironment and proportions of immune cells
To study differences in the composition of the tumor immune microenvironment between the EBV-positive group and the EBV-negative group, we used the “Estimation of Stromal and Immune cells in Malignant Tumours using Expression data” (ESTIMATE) R package (26) and CIBERSORT (27) algorithms to evaluate the immune microenvironment of the tumor tissues. ESTIMATE calculated the stromal score, immune score, ESTIMATE score, and tumor purity. CIBERSORT was performed to evaluate the proportion of 22 different types of tumor immune infiltrating cells (TIICs) in each tumor sample. Differences in the scores and infiltrating cell proportions between the EBV-positive group and the EBV-negative group were compared by using the Wilcoxon test.
Protein-protein interaction (PPI) network construction and hub gene identification
We uploaded all genes in the selected module to the STRING website (https://string-db.org/) to construct the PPI network. All parameters were set as default, and the generated network was exported for visualization and subsequent network-based analysis in Cytoscape v3.8.2 software (28). The CytoHubba plug-in (29) provides 12 different methods to identify the vital nodes in the PPI network. To identify the key genes with the most recognition times among these 12 methods, we first analyzed the top 10 key genes in the PPI network using the 12 methods in the CytoHabba plug-in and then counted the occurrence of these genes. Genes that occurred 5 or more times were finally identified as the hub genes.
Quantitative real-time polymerase chain reaction (RT-qPCR)
Total RNA from tissues was extracted using TRIzol reagent (15596018, Invitrogen, Carlsbad, California, USA) and converted to cDNA using the Prime Script RT Master Mix Kit (RR036A, Takara, Tokyo, Japan) according to the manufacturer’s instructions. RT-qPCR analysis was performed using a LightCycler 480 instrument (Roche Diagnostics, Switzerland) by using GoTaq qPCR Master Mix (A6002, Promega, Madison, Wisconsin, USA). Relative mRNA expression was normalized to β-actin, and the fold change was calculated with the 2−ΔΔCt method. The primers used in this study were listed in Table S2.
Survival analysis of hub genes
We used gene expression data and survival information from the SYSUCC cohort to analyze the effect of hub genes on prognosis. Each gene was divided into two groups, high expression and low expression, according to the median value. The R packages “survival” and “survminer” were employed to plot the Kaplan-Meier survival curves and perform log-rank tests to compare the survival differences of the two groups. P<0.05 was considered statistically significant.
Immunotherapy efficacy analysis
As mentioned earlier, the cohort of Kim et al. (11) was an anti-PD-1-treated GC cohort, and we downloaded their transcriptome data for reprocessing and quantified the expression profiles. After that, we extracted the TPM expression values of 11 key genes, used the R package “pheatmap” to plot the expression heatmap of these 11 genes, and clustered the genes according to the default parameters of the pheatmap function. According to the clustering result, we counted the number of treatment response cases and calculated the objective response rate (ORR) and clinical benefit rate (CBR) in each subclass.
Statistical analysis
In addition to the results of RT-qPCR, the statistical analyses and visualization of all other data were completed using R 4.1.0 software. Statistical analyses and visualization of RT-qPCR results were performed by GraphPad Prism 7 software. All statistical methods are described in detail above.
Ethical statement
The study was conducted in accordance with the Declaration of Helsinki (as revised in 2013).
Results
The DEGs and the most relevant module to EBVaGC
The flowchart of this study is shown in Figure 1. To explore the relationship between gene expression and EBV infection phenotype, we performed a differential gene expression analysis by comparing the EBV-positive group and the EBV-negative group in the TCGA-STAD cohort, which included 207 EBV-negative and 21 EBV-positive tumor samples for subsequent analysis. As shown in Figure 2A and website: https://cdn.amegroups.cn/static/public/tcr-22-461-01.xlsx, a total of 1,686 DEGs were obtained, including 127 upregulated DEGs and 1,559 downregulated DEGs. To investigate the relationship between the expression of DEGs and the clinical EBV phenotype, we used these DEGs to perform weighted gene co-expression network analysis using the “WGCNA” (24) R package. As shown in Figure 2B, the optimal value of parameter β was set as 3. Subsequently, we performed the dynamic tree cut method and obtained 16 gene modules (Figure 2C). The yellow module was the most correlated module to the EBV-positive phenotype (Figure 2D), which had 144 genes, including 90 upregulated DEGs and 54 downregulated DEGs. In summary, we obtained DEGs and the most relevant module to EBVaGC by performing differential gene expression analysis and WGCNA.
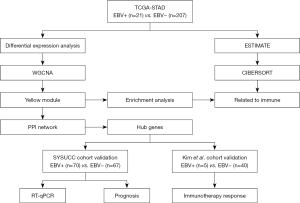
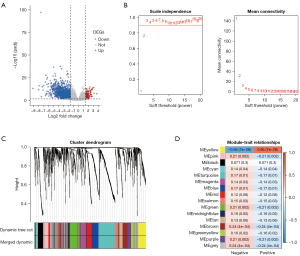
Genes in the yellow module primarily perform immune-related functions
To explore the function of genes in the yellow module, we performed GO enrichment analysis and KEGG pathway analysis. At the biological process level, these genes primarily regulate the activation and adhesion of immune cells, including T cell activation, regulation of cell-cell adhesion, regulation of T cell activation, calcium ion homeostasis, and regulation of leukocyte cell-cell adhesion (Figure 3A). At the molecular function level, these genes primarily regulate the activities of cytokines and chemokines, including receptor-ligand activity, signaling receptor activator activity, cytokine activity, cytokine receptor binding, and chemokine activity (Figure 3B). Furthermore, these genes were primarily located on the external side of the plasma membrane (Figure 3C) at the cell component level and primarily enriched in several pathways regulating immune factors and cells, such as cytokine-cytokine receptor interactions, natural killer cell-mediated cytotoxicity, chemokine signaling pathways and viral protein interactions with cytokines and cytokine receptors (Figure 3D). Besides, these genes were also enriched in cancer signaling pathways, such as the JAK-STAT signaling pathway (Figure 3D), which may lead to the initiation and progression of cancer. In conclusion, genes in the yellow module, which presented the characteristics of EBV status, primarily performed immune-related roles, and it may elucidate the molecular mechanisms of this special subset of EBVaGC.
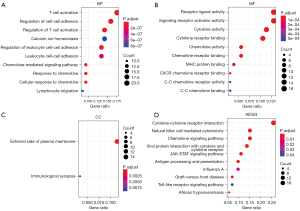
Tumor microenvironment changes in the EBV-positive group
Gene functional enrichment analysis showed that the DEGs in the EBV-positive group primarily functioned in an immune-regulating role, which prompted further exploration of the tumor microenvironment composition in tumor tissues. We first used the ESTIMATE algorithm to calculate the immune score, stromal score, ESTIMATE score, and tumor purity in each tumor tissue sample then compared these scores between the EBV-positive group and the EBV-negative group (Figure 4A-4D). We found that the immune score and ESTIMATE score were both higher in the EBV-positive group than the EBV-negative group (Figure 4A,4B), but the stromal score between the two groups was not significantly different (Figure 4C). The tumor purity of the EBV-positive group was lower than the EBV-negative group (Figure 4D). These results suggested that the changed tumor microenvironment included more immune components in the EBV-positive group. The CIBERSORT algorithm calculates the proportion of different types of immune cells in tumor samples in more detail. Therefore, to further validate this inference, we next performed the CIBERSORT analysis to reveal the changes. As shown in Figure 4E, CIBERSORT calculated the proportions of 22 different types of immune cells and we found that CD8+ T cells, M1-like macrophages, and CD4+ memory-activated T cells were all elevated in the EBV-positive group. Furthermore, M0 macrophages and CD4+ memory resting T cells were decreased in the EBV-positive group (Figure 4E). In summary, the components of the tumor microenvironment may be changed in EBV-positive GC, and this change might lead to an immune-activating phenotype.
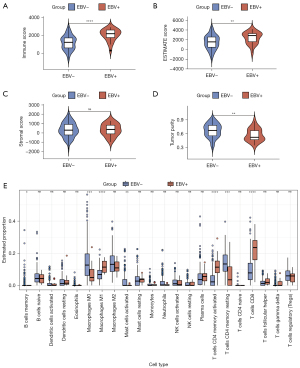
Hub genes were increased in EBV-positive tumors
There are complex interactions and connections between genes. Hub genes, which are often pivotal nodes in a PPI network, may play the most important role. The above analysis revealed a total of 144 genes in the yellow module using differential gene analysis and WGCNA. Therefore, to identify the hub genes of these 144 genes in the yellow module, we first used these genes to construct the PPI network using the STRING website (Figure S1). Next, we counted the occurrence of the top 10 genes obtained by 12 algorithms and identified the genes with a count greater than or equal to 5 as the final hub genes (as described in the Methods and Materials section). As shown in Figure 5A and Table S3, CD8A, CXCL10, CCR5, IFNG, CXCL9, GZMA, GZMB, KLRK1, TBX21, CCL5, and CD38 were identified as the hub genes in the PPI network, which suggests that these 11 genes might have a vital function in the EBV-positive group. Next, we used the SYSUCC cohort (including 70 EBV-positive samples and 67 EBV-negative samples) to perform RT-qPCR and validate the expression of these hub genes. Figure 5B-5L showed that all 11 hub genes were elevated in the EBV-positive group, which was consistent with the differential gene expression analysis (website: https://cdn.amegroups.cn/static/public/tcr-22-461-01.xlsx). We analyzed the prognostic role of these hub genes. As shown in Figure S2A-S2K, although there were no statistically significant differences in the overall survival of patients with EBV-positive GC between high and low hub gene expression, a trend of a better prognosis in patients with high expression of these genes was observed. We hypothesize that the lack of a statistically significant P value was due to the relatively small sample size. Overall, these data suggested that several hub genes were increased in the EBV-positive group, but the prognostic roles of these genes need further validation.
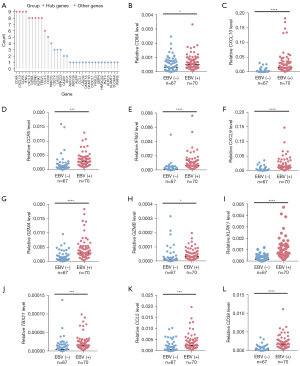
A potential classifier was associated with immunotherapy efficacy
Because these hub genes were primarily immune-related, we then explored whether these genes were related to the efficacy of immunotherapy. GC has a complicated heterogeneity, and the response of EBV to immunotherapy is controversial. We investigated the role of these 11 hub genes in predicting immune efficacy in EBVaGC. To this purpose, we utilized Kim et al.’s cohort (11) which was a gastric cohort that received anti-PD-1 immunotherapy. The cohort contained 45 RNA-seq samples, including 5 EBV-positive samples and 40 EBV-negative samples. We classified GC into 4 clusters according to the expression of the 11 hub genes: cluster 1 (C1), cluster 2 (C2), cluster 3 (C3), and cluster 4 (C4). The expression of these 11 genes increased gradually from C1 to C4, and the different subtypes clustered according to these 11 hub genes had different responses to immunotherapy (Figure 6). Among the EBV-positive samples, we found that 4 out of 5 cases were in the C4 subtype, while 1 sample was in the C2 subtype (Figure 6). EBV-negative tumor tissues were also present across the 4 various subclasses, which suggests heterogeneity of the expression levels in different tumor tissues. We analyzed the immune efficacy of each cluster in this cohort sequentially. As shown in Table 1, the ORR of C1 was 8.33%, and the CBR was 50%. The ORR of C2 was 16.67%, and the CBR was 55.56%. The ORR of C3 was 33.33%, and the CBR was 55.56%. The ORR of C4 was 83.33%, and the CBR was 100%. These results suggest that groups with higher expression of these 11 hub genes were more sensitive to immunotherapy, regardless of EBV status. In summary, these data demonstrated that the classifier composed of these 11 hub genes may be used to predict immunotherapeutic response in EBVaGC and the preclinical value of this classifier may be extended to all GC patients.
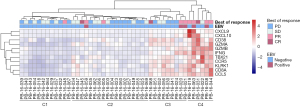
Table 1
Cluster | All (n) | PD (n) | SD (n) | PR (n) | CR (n) | ORR (%) | CBR (%) |
---|---|---|---|---|---|---|---|
C1 | 12 | 6 | 5 | 1 | 0 | 1/12 (8.33) | 6/12 (50.00) |
C2 | 18 | 8 | 7 | 2 | 1 | 3/18 (16.67) | 10/18 (55.56) |
C3 | 9 | 4 | 2 | 2 | 1 | 3/9 (33.33) | 5/9 (55.56) |
C4 | 6 | 0 | 1 | 4 | 1 | 5/6 (83.33) | 6/6 (100.00) |
C1, cluster 1; C2, cluster 2; C3, cluster 3; C4, cluster 4. PD, progressive disease; SD, stable disease; PR, partial response; CR, complete response; ORR, objective response rate; CBR, clinical benefit rate.
Discussion
EBVaGC was recently identified as a subtype of GC, and infected individuals are predominantly male, younger, and have better prognoses than EBVnGC patients (4,13,30). Therefore, exploring the relationship between gene expression and the EBV-infected phenotype in GC is essential to elucidate the diversity caused by EBV infection. Here, we observed 1,686 DEGs between EBV-positive and EBV-negative patients by performing RNA-seq analysis, and the most relevant module to the EBV-positive phenotype was discovered using WGCNA. These findings may partially explain the distinct molecular characteristics of EBVaGC.
Several reports showed that EBVaGC was often accompanied by greater immune cell infiltration, such as CD8+ cytotoxic T cells and mature dendritic cells, than EBVnGC. Therefore, EBVaGC is mostly associated with an immune-active tumor microenvironment (15,31). The present study observed that genes in the yellow module mostly regulated immune cells, cytokines, and chemokines. The immune score and ESTIMATE score were both elevated in the EBV-positive group compared to the EBVnGC group, but the stromal score was not significantly different. These results further demonstrated that EBV infection changed the tumor microenvironment, which was related to an immune-activating phenotype.
A previous study showed that most EBVaGC patients were resistant to current chemotherapy options (5). Considering the higher efficacy of immunotherapy in some EBV-infected individuals, there is a need to identify predictive biomarkers of patients who are sensitive to immunotherapy. Here, we used genes in the EBV-phenotype module to construct a PPI network using STRING database and identified 11 genes as the final hub genes. Then we employed RT-qPCR using RNA from tumor tissues in SYSUCC clinical cohorts to demonstrate the higher expression of these hub genes in EBVaGC.
In terms of the purpose of this study, we identified these 11 hub genes that may be used to evaluate the efficacy of immunotherapy. We can classify GC into 4 clusters according to the expression of the 11 hub genes and the expression of these 11 genes increased gradually from C1 to C4. Notably, different subtypes clustered according to these 11 hub genes had different responses to immunotherapy and individuals with elevated expression of these 11 genes were more prone to be beneficial in immunotherapy. Therefore, this classifier may be an applicable predictor for evaluating the efficacy of immune checkpoint therapy.
There are some limitations to this study. First, our research primarily analyzed tumor tissue using a series of bioinformatics-related algorithms. Although we used our hospital cohort to validate the expression of hub genes using RT-qPCR, further experimental validation studies on the changes in the immune microenvironment are needed. Second, when using 11 hub genes for clustering, we tried to establish a signature to predict immune efficacy through various statistical methods, but we could not use these genes to establish a predictive model due to the small sample size and relatively large number of independent variables, which is also a direction for future in-depth research.
In conclusion, our study identified several hub genes associated with EBV status that are closely related to the immune microenvironmental features of EBVaGC and may be used as molecular markers for the prediction of immune response in GC. Higher expression of these genes indicated a greater benefit of immunotherapy in GC patients.
Acknowledgments
Funding: This work was supported by the National Natural Science Foundation of China (No. 82072612).
Footnote
Reporting Checklist: The authors have completed the TRIPOD reporting checklist. Available at https://tcr.amegroups.com/article/view/10.21037/tcr-22-461/rc
Conflicts of Interest: All authors have completed the ICMJE uniform disclosure form (available at https://tcr.amegroups.com/article/view/10.21037/tcr-22-461/coif). The authors have no conflicts of interest to declare.
Ethical Statement: The authors are accountable for all aspects of the work in ensuring that questions related to the accuracy or integrity of any part of the work are appropriately investigated and resolved. The study was conducted in accordance with the Declaration of Helsinki (as revised in 2013). The Institutional Research Ethics Committee of SYSUCC approved the study.
Open Access Statement: This is an Open Access article distributed in accordance with the Creative Commons Attribution-NonCommercial-NoDerivs 4.0 International License (CC BY-NC-ND 4.0), which permits the non-commercial replication and distribution of the article with the strict proviso that no changes or edits are made and the original work is properly cited (including links to both the formal publication through the relevant DOI and the license). See: https://creativecommons.org/licenses/by-nc-nd/4.0/.
References
- Thrift AP, El-Serag HB. Burden of Gastric Cancer. Clin Gastroenterol Hepatol 2020;18:534-42. [Crossref] [PubMed]
- Yusefi AR, Bagheri Lankarani K, Bastani P, et al. Risk Factors for Gastric Cancer: A Systematic Review Asian Pac J Cancer Prev 2018;19:591-603. [PubMed]
- Johnston FM, Beckman M. Updates on Management of Gastric Cancer. Curr Oncol Rep 2019;21:67. [Crossref] [PubMed]
- Cancer Genome Atlas Research Network. Comprehensive molecular characterization of gastric adenocarcinoma. Nature 2014;513:202-9. [Crossref] [PubMed]
- Naseem M, Barzi A, Brezden-Masley C, et al. Outlooks on Epstein-Barr virus associated gastric cancer. Cancer Treat Rev 2018;66:15-22. [Crossref] [PubMed]
- Saito M, Kono K. Landscape of EBV-positive gastric cancer. Gastric Cancer 2021;24:983-9. [Crossref] [PubMed]
- Wei XL, Liu QW, Liu FR, et al. The clinicopathological significance and predictive value for immunotherapy of programmed death ligand-1 expression in Epstein-Barr virus-associated gastric cancer. Oncoimmunology 2021;10:1938381. [Crossref] [PubMed]
- He CY, Qiu MZ, Yang XH, et al. Classification of gastric cancer by EBV status combined with molecular profiling predicts patient prognosis. Clin Transl Med 2020;10:353-62. [Crossref] [PubMed]
- Jia X, Guo T, Li Z, et al. Clinicopathological and Immunomicroenvironment Characteristics of Epstein-Barr Virus-Associated Gastric Cancer in a Chinese Population. Front Oncol 2021;10:586752. [Crossref] [PubMed]
- Panda A, Mehnert JM, Hirshfield KM, et al. Immune Activation and Benefit From Avelumab in EBV-Positive Gastric Cancer. J Natl Cancer Inst 2018;110:316-20. [Crossref] [PubMed]
- Kim ST, Cristescu R, Bass AJ, et al. Comprehensive molecular characterization of clinical responses to PD-1 inhibition in metastatic gastric cancer. Nat Med 2018;24:1449-58. [Crossref] [PubMed]
- Xie T, Liu Y, Zhang Z, et al. Positive Status of Epstein-Barr Virus as a Biomarker for Gastric Cancer Immunotherapy: A Prospective Observational Study. J Immunother 2020;43:139-44. [Crossref] [PubMed]
- Qiu MZ, He CY, Yang DJ, et al. Observational cohort study of clinical outcome in Epstein-Barr virus associated gastric cancer patients. Ther Adv Med Oncol 2020;12:1758835920937434. [Crossref] [PubMed]
- Huang B, Li Q, Geng Q, et al. ASTE1 frameshift mutation triggers the immune response in Epstein-Barr virus-associated gastric cancer. Signal Transduct Target Ther 2022;7:4. [Crossref] [PubMed]
- Cho J, Kang MS, Kim KM. Epstein-Barr Virus-Associated Gastric Carcinoma and Specific Features of the Accompanying Immune Response. J Gastric Cancer 2016;16:1-7. [Crossref] [PubMed]
- Sasaki S, Nishikawa J, Sakai K, et al. EBV-associated gastric cancer evades T-cell immunity by PD-1/PD-L1 interactions. Gastric Cancer 2019;22:486-96. [Crossref] [PubMed]
- Colaprico A, Silva TC, Olsen C, et al. TCGAbiolinks: an R/Bioconductor package for integrative analysis of TCGA data. Nucleic Acids Res 2016;44:e71. [Crossref] [PubMed]
- Love MI, Huber W, Anders S. Moderated estimation of fold change and dispersion for RNA-seq data with DESeq2. Genome Biol 2014;15:550. [Crossref] [PubMed]
- Rooney MS, Shukla SA, Wu CJ, et al. Molecular and genetic properties of tumors associated with local immune cytolytic activity. Cell 2015;160:48-61. [Crossref] [PubMed]
- Ewels P, Magnusson M, Lundin S, et al. MultiQC: summarize analysis results for multiple tools and samples in a single report. Bioinformatics 2016;32:3047-8. [Crossref] [PubMed]
- Chen S, Zhou Y, Chen Y, et al. fastp: an ultra-fast all-in-one FASTQ preprocessor. Bioinformatics 2018;34:i884-90. [Crossref] [PubMed]
- Dobin A, Davis CA, Schlesinger F, et al. STAR: ultrafast universal RNA-seq aligner. Bioinformatics 2013;29:15-21. [Crossref] [PubMed]
- Li B, Dewey CN. RSEM: accurate transcript quantification from RNA-Seq data with or without a reference genome. BMC Bioinformatics 2011;12:323. [Crossref] [PubMed]
- Langfelder P, Horvath S. WGCNA: an R package for weighted correlation network analysis. BMC Bioinformatics 2008;9:559. [Crossref] [PubMed]
- Wu T, Hu E, Xu S, et al. clusterProfiler 4.0: A universal enrichment tool for interpreting omics data. Innovation (Camb) 2021;2:100141. [Crossref] [PubMed]
- Yoshihara K, Shahmoradgoli M, Martínez E, et al. Inferring tumour purity and stromal and immune cell admixture from expression data. Nat Commun 2013;4:2612. [Crossref] [PubMed]
- Newman AM, Liu CL, Green MR, et al. Robust enumeration of cell subsets from tissue expression profiles. Nat Methods 2015;12:453-7. [Crossref] [PubMed]
- Shannon P, Markiel A, Ozier O, et al. Cytoscape: a software environment for integrated models of biomolecular interaction networks. Genome Res 2003;13:2498-504. [Crossref] [PubMed]
- Chin CH, Chen SH, Wu HH, et al. cytoHubba: identifying hub objects and sub-networks from complex interactome. BMC Syst Biol 2014;8:S11. [Crossref] [PubMed]
- Yang J, Liu Z, Zeng B, et al. Epstein-Barr virus-associated gastric cancer: A distinct subtype. Cancer Lett 2020;495:191-9. [Crossref] [PubMed]
- Ma J, Li J, Hao Y, et al. Differentiated tumor immune microenvironment of Epstein-Barr virus-associated and negative gastric cancer: implication in prognosis and immunotherapy. Oncotarget 2017;8:67094-103. [Crossref] [PubMed]