Comprehensive bioinformatic analyses of lncRNA-mediated ceRNA network for uterine corpus endometrial carcinoma
Introduction
Uterine corpus endometrial cancer (UCEC), one of globally common gynecological malignancies, presents a possibly upward trend of with the increase of obese women (1-3). The choice for treatment of UCEC has considerable exploration and development prospects for the perspective of molecular biology. In previous studies, risk factors including p53 expression (4), and estrogen receptor (ER) and progesterone receptor (PR) expression (5), as well as clinical treatment manners have been identified (6). The discovery of these factors provides access to take advantage of underlying therapeutic biomarkers for personalized treatment strategies. MiRNA is a family of small non-coding RNA molecules of about 21 to 25 nucleotides long. MiRNA inhibits translation of targeted mRNA or affects its stability by specific identifications, and down regulates its expression by combining at its 3'UTR site. The abnormal miRNA expression in development of tumors has been confirmed by many studies (7,8). LncRNA is known to play a role as key signal transduction mediators in the occurrence, progression and treatment of numerous malignancies (9-11). According to the ceRNA hypothesis (12), lncRNA is a molecular sponge of miRNA, which suppresses the activity of miRNA by binding with microRNA response element (MRE) and down regulates the expression of the target genes indirectly. Based on this argument, the ceRNA network has been extensively researched and verified in lung cancer (13), breast cancer (14) and so on, while there have been little discussion on lncRNA-mediated ceRNA networks of UCEC.
Therefore, in this study, we retrieved and analyzed lncRNA expression in UCEC from The Cancer Genome Atlas (TCGA) and Clinical Proteomic Tumor Analysis Consortium (CPTAC) database separately and performed an integrated bioinformatics analysis including functional enrichment analysis, Tumor Infiltrating Immune Cell (TIIC) analysis, and constructed the UCEC-specific ceRNA network and figured out the underlying association between those ceRNAs and the progression of UCEC. We present the following article in accordance with the STREGA reporting checklist (available at https://tcr.amegroups.com/article/view/10.21037/tcr-22-249/rc).
Methods
Data collection
The study was conducted in accordance with the Declaration of Helsinki (as revised in 2013). Transcriptional and clinical data of UCEC in both TCGA data portal (https://portal.gdc.cancer.gov/) and CPTAC (https://cptac-data-portal.georgetown.edu/data-use-agreement) were retrieved. There were 555 samples downloaded from TCGA, containing 543 patients (543 UCEC tissues and 23 adjacent tumor tissues, which for further analyses) and 12 patients (excluded, for inadequate information), as well as 116 samples downloaded from CPTAC, containing 101 UCEC tissues and 15 adjacent tumor tissues, contributing to the UCEC and normal control group as a cohort. The clinical features of UCEC patients from 2 databases were respectively shown (Table 1). The clinical features of UCEC patients including age, gender, race, pathology stage, histological type and vital status were extracted. Transcriptome data were annotated with the Genecode website (https://www.gencodegenes.org/). No samples were excluded when to screen for differentially expressed RNAs [DERNAs, including three ones: differentially expressed long noncoding RNA (DElncRNA), differentially expressed microRNA (DEmiRNA), differentially expressed messenger RNA (DEmRNA)]. Both databases were publically available and were released in compliance with ethical approvals; therefore, no further application from University Ethics Committee was obtained.
Table 1
Features | Variables | Patients (n, %) |
---|---|---|
TCGA features | ||
Age, years | >60 | 334 (61.51) |
≤60 | 206 (37.94) | |
NA | 3 (0.55) | |
Gender | Female | 543 (100.00) |
Race | White | 372 (68.51) |
NA | 32 (5.89) | |
Black or African American | 106 (19.52) | |
Native Hawaiian or other pacific islander | 9 (1.66) | |
American Indian or Alaska native | 3 (0.55) | |
Asian | 20 (3.68) | |
Pathology stage | Stage I | 339 (62.43) |
Stage II | 51 (9.39) | |
Stage III | 124 (22.84) | |
Stage IV | 29 (5.34) | |
Primary diagnosis | Endometrioid carcinoma | 399 (73.48) |
Serous cystadenocarcinoma | 133 (24.49) | |
Other types | 11 (2.03) | |
Survival | Alive | 452 (83.24) |
Dead | 91 (16.76) | |
CPTAC features | ||
Age, years | >60 | 64 (63.37) |
≤60 | 37 (36.63) | |
Gender | Female | 101 (100.00) |
Race | White | 59 (58.42) |
NA | 38 (37.62) | |
Black or African American | 3 (2.97) | |
Asian | 1 (0.99) | |
Pathology stage | Stage I | 75 (74.26) |
Stage II | 8 (7.92) | |
Stage III | 15 (14.85) | |
Stage IV | 3 (2.97) | |
Histological type | Endometrioid carcinoma | 85 (84.16) |
Other types | 16 (15.84) | |
Tumor grade | G1 + G2 | 71 (70.30) |
G3 | 27 (26.73) | |
Other | 3 (2.97) | |
Survival | Alive | 84 (83.17) |
Dead | 10 (9.90) | |
Not reported | 7 (6.93) |
Staging is performed with the use of the International Federation of Gynecology and Obstetrics (FIGO) 2009 system. Stage I: tumor area limited to corpus uteri, including endocervical glandular involvement. Stage II: tumor area has invaded the stromal connective tissue of the cervix field but hasn’t extended over the uterus as well as endocervical glandular invasion. Stage III: tumor has invaded serosa, or adnexa, or vagina, or parametrium. Stage IV: tumor has invaded the bladder mucosa area, bowel mucosa area, or both, or distant metastasis. TCGA, The Cancer Genome Atlas; CPTAC, Clinical Proteomic Tumor Analysis Consortium; NA, not applicable; G1, highly differentiated and low grade, mild; G2, moderately differentiated and intermediate grade, moderate; G3, lowly differentiated and high grade, severe.
Identification of DEGs
The “edge R” was utilized to identify differentially expressed genes (DEGs) between the normal tissues and UCEC tumor tissues by the criteria [false discovery rate (FDR), adjusted P<0.01, and |log2FC| >2 (FC is fold change)]. All of the statistical tests were conducted and the heatmap and volcano plot were displayed by ggplot2 package in R software package (version:4.0.3; https://www.r-project.org/) and statistical significance was defined as a P value <0.05 unless otherwise stated.
Construction of protein-protein interaction (PPI) network
In this study, a total of 1,064 and 917 DEGs filtered by |log2FC| >3 from the two databases were subjected to perform PPI network analysis using Search Tool for the Retrieval of Interacting Genes (STRING; https://string-db.org/) (15). An interaction with a combined score by default >0.4 was considered statistically significant. Cytoscape (version 3.8.2) is a bioinformatics software platform publically used for visualizing molecular interaction networks (16). To find hub genes actively participated in UCEC progression, we employed the maximal clique centrality (MCC) algorithm to represent 20 key mRNAs with important biological functions via CytoHubba in Cytoscape (17).
TIICs profiling
To characterize proportions of TIICs in UCEC, CIBERSORT (http://cibersort.stanford.edu/) algorithm in combination with a LM22 gene signature matrix was used to assess the relative fractions of 22 invasive immune cell subtypes in each UCEC tissues.
Additionally, to investigate the immune infiltration signatures of UCEC, gene set enrichment analysis (ssGSEA) was performed by GSVA package in R (https://bioconductor.org/packages/release/bioc/html/GSVA.html) to calculate the score of immune infiltration in each sample on the basis of immune cell-specific gene expression levels. Standardized profiles of gene expression data in both TCGA and CPTAC databases were extracted and immune scores were evaluated, scoring types including different cell clusters and respective expression values corresponding to different colors.
Regarding the results of the CIBERSORT algorithm, we also accumulated the percentage of each immune cell theoretically calculated from each sample (tumor tissues & adjacent tissues, totally 566 samples) and presented those top ranked immune cell types in bar plots. Worthy to point out, analyzing results of TIIC, with P values of >0.05 derived from CIBERSORT algorithm, were omitted before visualization in bar plots.
Construction of ceRNA network and extraction of survival-related lncRNA-miRNA-mRNA subnetwork
In this study, the lncRNA-mediated ceRNA (competing endogenous RNA) network of UCEC was constructed as lncRNA-miRNA and miRNA-mRNA pairs. To build a ceRNA network, following steps were conducted. Firstly, based on miRNAs provided by the miRcode website (http://www.mircode.org), lncRNA-targeted miRNAs simultaneously in our DEmiRNAs were filtered. Secondly, to identify the miRNA-targeted mRNAs, we searched miRTarBase (18) (https://mirtarbase.cuhk.edu.cn/~miRTarBase/miRTarBase_2019/php/index.php), miRDB (19) (http://www.mirdb.org/), TargetScan (20) (http://www.targetscan.org/) in combination to obtain targeted mRNAs. Finally, Cytoscape software v3.8.2 (https://cytoscape.org/) was adopted to visualize the UCEC-related ceRNA network. The paired interactions in above network were analyzed in two UCEC datasets from TCGA and CPTAC, respectively. Subsequently, we took the intersection of the two ceRNA networks.
Furthermore, on the foundation of overall ceRNA network, we firstly conducted survival analyses and Cox regression analyses of these genes, both results of which were considered as hub genes in our ceRNA network. Thereafter, we input these hub genes to visualize their lncRNA-miRNA-mRNA regulatory relationships via Cytohubba (http://hub.iis.sinica.edu.tw/cytohubba) in Cytoscape. Eventually, we constructed a novel ceRNA subnetwork composed of lncRNA-miRNA-mRNA pairs, which may provide prognostic molecular values.
Survival analysis and lncRNA-mediated prognostic model construction
On one hand, survival R was operated for survival analysis of all DERNAs in the ceRNA network. Kaplan-Meier (K-M) curves were plotted with DERNAs by log-rank test. On the other hand, we identified the lncRNAs linked with total survival (P<0.05) to act as prognostic lncRNA signature candidates and then imported them for Cox regression analyses. According to the median risk score, the UCEC samples were divided into the high-risk and low-risk groups. To evaluate the accuracy of models survival-related DElncRNAs, we carried out the receiver operating characteristic (ROC) curve analysis (UCEC data in CPTAC using 3 years as the predicted time, UCEC data in TCGA using 5 years as the predicted time), along with the area under the receiver operating characteristic curve (AUC) analysis at a criterion of AUC >0.7. Besides, we further retrieved analyses of survival-related lncRNAs in the GEPIA database (GEPIA; http://gepia.cancer-pku.cn/index.html) by P values ≤0.1 (although 0.11 was also considered as significant in this study). Subsequently, we compared these results of survival-related lncRNAs with our analyses thus to validate and prove its reliability.
Validation of dysregulated mRNAs via HPA and GEPIA databases
The Human Protein Atlas Portal (HPA) (www.proteinatlas.org) (21) which contains different genes in specific cancer types and publicly available information of Immunohistochemistry (IHC) staining was used for survival analyses. Gene Expression Profiling Interactive Analysis (GEPIA), an interactive website, composed of 9,736 patients and 8,587 normal samples from TCGA and GTEx projects (The Genotype-Tissue Expression project) were utilized for the analysis of RNA sequencing expression. According to prognostic lncRNA-mRNA or mRNA-miRNA signatures in the lncRNA-mediated network, we took 33 mRNAs resulted from UCEC data of TCGA into two external databases mentioned above for further retrieval and analysis.
Functional and pathway enrichment analysis
To analyze functions represented in two profiles of identified DEmRNAs in the ceRNA network, Gene Ontology (GO), Kyoto Encyclopedia of genes and Genomes (KEGG) were performed by cluster Profiler R package and plotted by GO plot R package and KEGG plot R package. Three methods were utilized to enrich meaningful biological pathways by the standard of P valve less than 0.05.
Statistical analysis
A total of statistical tests in our experiments were done with R (https://www.r-project.org/) and statistically significant values were defined as P values <0.05 unless otherwise stated.
Results
Differentially expressed RNAs in UCEC
In a study of 566 tissue samples (including 543 UCEC tissues and 23 normal tissues) collected from TCGA (http://cancergenome.nih.gov/publications/publication guide lines), “edge R” (adjusted P<0.01 and |log2FC| >2) was adjusted to identify the DERNAs, including DElncRNAs, DEmiRNAs, DEmRNAs. A total of 1,513 up-regulated DEmRNAs, 914 down-regulated DEmRNAs, 686 up-regulated DElncRNAs, 330 down-regulated DElncRNAs, 124 up-regulated DEmiRNAs, and 50 down-regulated DEmiRNAs were identified in TCGA database. Similarly, a total of 116 samples were identified with the same criteria in the CPTAC database, including 2,463 DEmRNAs, 1,741 DElncRNAs and 204 DEmiRNAs. The heatmap and volcano plot of those highest dysregulated DElncRNAs, DEmiRNAs and DEmRNAs were shown in Figure 1 and the top 10 up and down regulated DElncRNAs, DEmiRNAs and DEmRNAs were shown in Table S1.
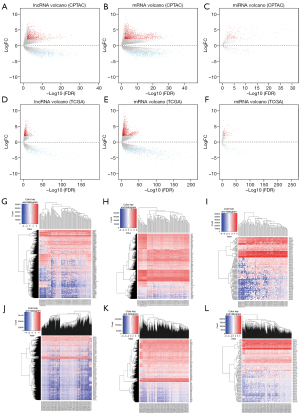
Construction of PPI network
The 1,981 DEmRNAs (|log2FC| >3) were further selected to construct PPI network to select hub genes that play crucial roles in UCEC genesis. Given the large number of DEmRNAs in this module, we used MCC algorithm in “Cytohubba” of Cytoscape software to visualize and select hub genes in the PPI network. The top 20 high score genes in in CPTAC were shown (Figure 2A). Similarly, the top 20 high score genes in TCGA belong to Histone cluster 1 H family which was shown (Figure 2B).
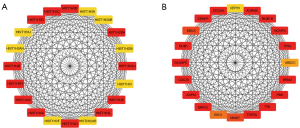
Two graphs separately describe the top 20 most dynamic hub genes and their intersection relationships evaluated by MCC algorithm in “Cytohubba”. These sub-graphs of these selected mRNA-coding protein nodes are shown from highly essential (red) to essential (yellow).
TIICs enrichment analysis
Using CIBERSORT algorithm, we evaluated 101 tumor transcriptome profiles from CPTAC database and 543 tumor transcriptome profiles from TCGA database (Figure 3A,3B). In addition, we also performed ssGSEA analysis by GSVA package to score the corresponding TIICs in each simple sample, and finally we found that TIICs in both CPTAC and TCGA database sources expressed well (Figure 3C,3D).
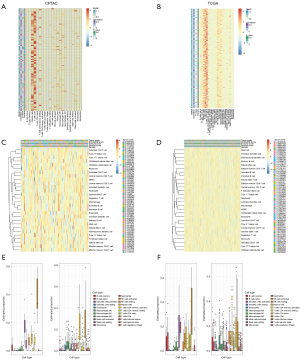
On account of 22 immune cell components in UCEC and relevant controlled samples by CIBERSORT algorithm, two pairs of bar plots were then visualized. In CPTAC malignant tissue samples, there were abundant CD8 T cells and plasma cells and other TIICs. In CPTAC normal tissue samples, those TIICs were T cells (CD8), mast cells (resting), plasma cells, T cells (CD4 memory resting), NK cells (resting) well infiltrated in order. While in TCGA malignant tissue samples, naive CD4 cells, T cells (CD8 cells), plasma cells, activated NK cells, and macrophages M0 were well infiltrated. In TCGA controls’ samples, those well infiltrated ones were listed as T cells (CD8 cells), monocytes, mast cells (resting), plasma cells and naïve B cells (Figure 3E,3F).
Construction of ceRNA Network and hub lncRNA-miRNA-mRNA subnetwork
In order to better comprehend the interactions of mRNAs, lncRNAs, and miRNAs in UCEC, we constructed an lncRNA-mediated ceRNA regulatory network. To begin with, 1,741 DElncRNAs in CPTAC database succeeded to match with 123 lncRNAs in the miRCODE database. Considering 123 of 1,741 DElncRNAs could interact with DEmiRNAs, 36 miRNAs in both miRCODE and CPTAC database were selected to construct lncRNA-miRNA pairs. Meanwhile, to interplay with 204 DEmiRNAs acquired from CPTAC database, we retrieved 1,420 mRNAs in three databases (miRTarBase, miRDB and TargetScan). The 1,420 miRNA-targeted mRNAs predicted in these databases were intersected with 2,463 DEmRNAs thus to obtain 124 miRNA-targeted mRNAs belonging to CPTAC database. Finally, an lncRNA-mediated ceRNA network consisting of 36 miRNAs, 123 lncRNAs and 124 mRNAs were achieved (Figure 4A). Meanwhile, the same workflow of UCEC-specific ceRNA network construction was repeated in data from TCGA. We obtained an lncRNA-mediated ceRNA network consisting of 38 miRNAs, 83 lncRNAs and 110 mRNAs (Figure 4B).
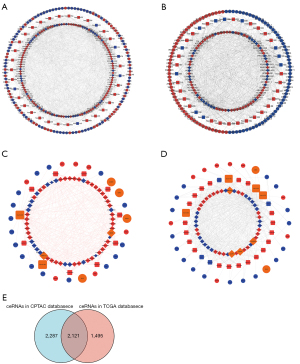
Furthermore, cytohubba was applied to visualize our extracted hub genes composing of lncRNA, miRNA and mRNAs and derived regulatory ceRNA network thus to identify potentially prognostic molecular pathways of UCEC in CPTAC and TCGA (Figure 4C,4D). A total of 6 hub lncRNA-miRNA-mRNA regulatory relationships from 2 databases were shown (Table 2). Moreover, coincident ceRNA results in the overall ceRNA network from both CPTAC and TCGA databases were shown in the Venn diagram (Figure 4E).
Table 2
LncRNA | miRNA | mRNA |
---|---|---|
TRBV11-2 | has-mir-363 | SOX11 |
MEG8 | has-mir-424 | CCNE1, CBX2 |
has-mir-363 | SOX11 | |
has-mir-183 | DLX4, NR3C1 | |
lncRNA | miRNA | mRNA |
LINC00443, C2orf48, LINC00483 | miR-183 | DLX4, NR3C1 |
DGCR5 | has-mir-195 | CCNE1 |
has-mir-383 | ||
has-mir-424 |
lncRNA, long noncoding RNA; mRNA, messenger RNA; miRNA, microRNA; CeRNA, competing endogenous RNA; DEGs, differentially expressed genes; UCEC, uterine corpus endometrial carcinoma.
Identification and validation of prognostic lncRNAs in ceRNA networks
In order to figure out the effects of interactions for survival between lncRNAs, miRNAs and mRNAs, we imported survival-related data of UCEC and genes in ceRNA to analyze its prognosis. Survival R were operated for DERNAs significantly correlated with overall survival (OS) in the ceRNA network (P<0.05), the results of which were plotted by Kaplan-Meier (K-M) curves (Figure 5A-5W).
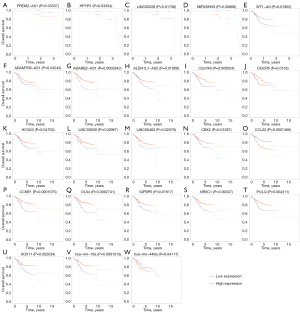
As shown in CPTAC, 4 survival-related DElncRNAs on the level of 3-year survival were identified in DElncRNA-mediated ceRNA networks, including FREM2-AS1, HPYR1, LINC00028, MIR205HG (Figure 5A-5D). Similarly, 19 survival-related DElncRNAs, 9 DEmiRNAs and 33 DEmRNAs on the level of 5-year survival were revealed in the ceRNA network for TCGA. Figure 5E-5W illustrated Kaplan-Meier curve analyses about 10 lncRNAs of 19 survival-related DElncRNAs, 5 mRNAs of 33 DEmRNAs and 2 of 9 DEmiRNAs derived from TCGA database. Besides, we successfully validated 5 survival-related lncRNAs in GEPIA database by P values ≤0.1 (Figure 6A-6E).
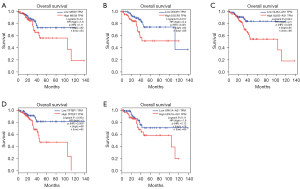
To further identify DElncRNAs with prognostic features in a more accurate way, multi-Cox regression analyses and corresponded ROC curves were carried out. After eliminating some samples lacking in survival time, 94 complete samples in CPTAC were divided into the high-risk (n=47) and low-risk (n=47) groups (cutoff value =−0.78) and 543 samples with complete survival information in TCGA into the high-risk (n=272) and low-risk (n=272) cohort by median value (cutoff value=−0.18; one sample of survival data was just in the median and counted in both groups). We performed a multi-factor COX regression analysis (Figure 7A,7B) and a global survival analysis of the model (Figure 7C,7D) thus separately identified two lncRNAs of 3-year survival data in CPTAC and eight lncRNA prognosis candidates of 5-year survival UCEC data in TCGA by P<0.05. ROC curves tested the influence on their lncRNA signatures associated with OS in UCEC. Area under ROC curve of 3-year survival rate (AUC) and 5-year survival rate (AUC) were respectively 0.967 and 0.751 (Figure 7E,7F). Besides, multivariate cox regression analysis of totally 10 prognostic lncRNAs associated with OS in UCEC tumor tissues generated from 2 databases were shown in Table 3.
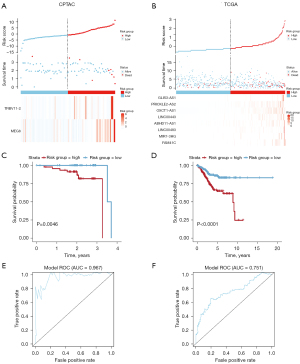
Table 3
LncRNA | Coef | HR | 95% CI_LL | 95% CI_HL | P value |
---|---|---|---|---|---|
LncRNA (in TCGA) | |||||
FAM41C | 0.017169325 | 1.017317565 | 1.007489748 | 1.027241249 | 5.27E-04 |
MIR7_3HG | 0.003483874 | 1.00348995 | 1.001209451 | 1.005775643 | 0.002688886 |
LINC00483 | 0.010635045 | 1.010691798 | 1.003527131 | 1.017907617 | 0.003389722 |
ABHD11_AS1 | 8.26E-04 | 1.000826495 | 1.00038596 | 1.001267224 | 2.35E-04 |
LINC00443 | 0.007147984 | 1.007173592 | 1.001433816 | 1.012946267 | 0.014233205 |
OXCT1_AS1 | 0.026906491 | 1.027271739 | 1.006299256 | 1.048681314 | 0.010568912 |
PRICKLE2_AS2 | 0.273785608 | 1.314932861 | 1.133483915 | 1.525428288 | 3.02E-04 |
GLIS3_AS1 | 9.53E-04 | 1.000953853 | 1.000210868 | 1.001697389 | 0.011853011 |
LncRNA (in CPTAC) | |||||
MEG8 | 0.007280976 | 1.007307546 | 1.003839185 | 1.010787892 | 3.51E-05 |
TRBV11_2 | 0.027816351 | 1.028206838 | 1.012679086 | 1.043972683 | 3.40E-04 |
TCGA, The Cancer Genome Atlas; CPTAC, Clinical Proteomic Tumor Analysis Consortium; lncRNA, long noncoding RNA; mRNA, messenger RNA; miRNA, microRNA; Coef, regression coefficient in our multivariate Cox analysis; HR, hazard ratio; CI, confidence interval; LL, lower limit; HL, higher limit.
In the multivariate Cox regression analysis derived from TCGA database, 8 lncRNAs including FAM41C, MIR7_3HG, LINC00483, ABHD11_AS1, LINC00443, OXCT1_AS1, PRICKLE2_AS2 and GLIS3_AS1 were identified to construct the OS prediction model. OS-related prediction model= (0.017169325* expression value of FAM41C) + (0.003483874* expression value of MIR7_3HG) + (0.010635045* expression value of LINC00483) + (8.26E-04* expression value of ABHD11_AS1) + (0.007147984* expression value of TRBV11_2) + (0.027816351* expression value of TRBV11_2) + (0.027816351* expression value of LINC00443) + (0.026906491* expression value of OXCT1_AS1) + (0.273785608* expression value of PRICKLE2_AS2) + (9.53E-04* expression value of GLIS3_AS1). We divided the 543 UCEC cases into the high- and low-risk groups according to the median values of the OS-related prediction model.
In the multivariate Cox regression analysis derived from CPTAC database, 2 lncRNAs including MEG8 and TRBV11_2 were identified to construct the OS prediction model. OS-related prediction model= (0.007280976* expression value of MEG8) + (0.027816351* expression value of TRBV11_2). We divided the 94 UCEC cases into the high- and low-risk groups according to the median values of the OS-related prediction model.
Validations of survival analysis and mRNA expression at the transcriptional level
To further demonstrate the prognostic significance of 33 mRNAs screened from the ceRNA network, we selected external databases for survival analysis and validation with IHC images. Firstly, we input 33 screened mRNAs into HPA database (version 20.1; https://www.Proteinatlas.org/about/assays+annotation#tcga_survival) to validate whether they were associated with the prognosis of UCEC. Consequences revealed that 8 mRNAs (CBX2, CCL22, CCNE1, DLX4, IGFBP5, NR3C1, SOX11, POLQ) highly expressed in UCEC were closely related with its prognosis (log rank P values <0.001). Subsequently, we retrieved OS analyses of 8 mRNAs generated from GEPIA by filtered criteria of P values ≤0.1 (although 0.11 was also considered as significant in this study) and verified 5 mRNAs (CCNE1, CCL22, NR3C1, IGFBP5 and POLQ).
Based on two previous steps for external verifications, two IHC images of the last screened mRNAs (CCNE1, NR3C1) in the HPA database approved the same results (Figure 8A). Survival validations of 5 mRNAs including CCNE1, CCL22, NR3C1, IGFBP5 and POLQ from GEPIA were shown in the Figure 8B-8F. In this study, we identified 5 survival-related mRNAs, there were no related IHC samples of CCL22, IGFBP5 and POLQ but CCNE1 and NR3C1 to further validate in the HPA database. The translational expression level of CCNE1 and NR3C1 was positively linked with disease status, as they were up-regulated in UCEC samples.
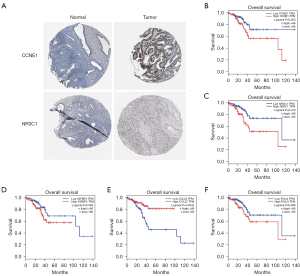
Enrichment analyses of functional pathways
To elucidate the biological functions represented in two profiles of identified DEmRNAs, we performed enrichment analyses mainly by “cluster profiler”, with the standard of P<0.05. In this study, GO analyses disclosed that top significant GO terms (P<0.05) commonly obtained from UCEC data in CPTAC, and TCGA database (Figure 9A-9D). The KEGG results derived from DEmRNAs in TCGA database were shown (Figure 9E-9H). The KEGG analyses revealed that what closely related to DEmRNAs originated from CPTAC and TCGA database were mainly enriched in pathways such as “cellular senescence”, “proteoglycans in cancer” and “microRNAs in cancer”. The top 20 GO and KEGG results for TCGA and CPTAC database were provided in Table S2.
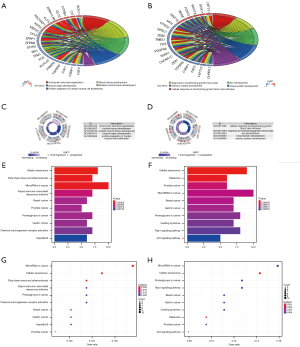
Discussion
Recently, with the increase of obese women, UCEC has become one of the leading gynecologic tumors (22). Although some diagnostic markers like CA125, CA199, and CEA are clinically used, survival results are not optimistic after routine diagnosis and therapy. Therefore, it is worthy to discover and analyze biomarkers for prognosis prediction of UCEC. LncRNAs have increasingly seized the attention of cancer research fields because of serving as regulating biomarkers (23). But due to experimental complexity, functional studies related to lncRNAs have limitations to carry out in comparison with those of protein-encoding RNAs. As is illustrated in accumulating researches, molecular mechanisms underlying ceRNA network provide an explanation for carcinogenesis and its associated development. LncRNAs act as key components of ceRNA family, through miRNA response elements (MREs), compete with molecules binding to the same miRNAs to achieve regulation of expression levels between each other.
As our lncRNA-mediated ceRNA network of UCEC respectively constructed from the CPTAC and TCGA databases indicated, there were a total of 23 lncRNAs, 9 miRNAs, and 33 mRNAs correlated with the OS results and served as promising biomarkers for predicting prognosis of UCEC. Conventional prognostic model constructions often make inadequate risk groupings and estimates of clinical outcomes (24,25). Nonetheless, this ceRNA hypothesis provides us a novel predictive insight from the angle of heterogeneity between UCEC patients to analyze OS results. For bioinformatics analysis conducted in multiple databases, it is common practice to combine sample profiles from multiple databases for further analysis after standardized quality control treatment. However, the follow-up time length of UCEC profiles from CPTAC is shorter than that from TCGA database. So, we calculated on the level of three-year survival results thus didn’t combine it with that from TCGA database.
In our present study, MIR205HG and ADARB2-AS1 were significantly correlated with survival. High expression of MIR205HG was found at the first time to predict a good prognosis, while high expression of ADARB2-AS1 had an opposite effect on the survival outcome of patients. Dong et al. showed that lncRNA MIR205HG depleted SRSF1 to increase KRT17 expression (26), while KRT17 silencing impaired cervical cancer cell proliferation and migration and activated apoptosis. LncRNA MIR205HG also acts as a ceRNA to accelerate tumor growth and progression in cervical cancer through spongiform Mir-122-5p (27). ADARB2-AS1 has been reported as a prognostic related lncRNA in UCEC (28), which again echoed the reliability of our results.
In addition, in order to make our results convincing, we put lncRNA biomarkers into GEPIA for external verification. The results showed that 5 highly expressed lncRNAs of DGCR5, GLIS3-AS1, UPK1A-AS1, MEG8, TPTEP1 indicated poor prognosis, which is in agreement with our results. To clarify our findings, we comprehensively analyzed survival results of lncRNA-regulated mRNAs in GEPIA and HPA. Through these external databases, we also concluded that high expression of CCL22, CCNE1, IGFBP5, NR3C1 and POLQ genes were associated with poor prognosis of UCEC. Furthermore, we mirrored methods in foregoing study (29) to identify our mRNA results and obtained poor survival results of NR3C1 and CCNE1 by validations of IHC images, which suggested their tumor promoter roles. Some researches have verified that CCNE1 amplification is associated with aggressive potential in UCEC tumorigenesis (30-32). CCNE1, known as Cyclin E1, is a member of Cyclins to function as regulators of CDK kinases. The protein encoded by Cyclin belongs to the highly conserved Cyclin family, characterized in its dramatic periodicity in protein abundance through cell cycle. With respect to other carcinoma progression, patients with over-expressed CCNE1 were reportedly at increased threat for poor endings of cervical cancer (33) and triple-negative breast cancer (34). Functioned as regulatory genes in the downstream, mRNA CCNE1 and NR3C1 brought potential reference values for our presently identified lncRNA-mediated ceRNA pathways.
Furthermore, on the basis of overall ceRNA network, we constructed a novel prognostic ceRNA subnetwork, which were composed of lncRNA-miRNA-mRNA axes. We firstly conducted survival analysis and multivariate analysis on lncRNAs in the ceRNA network derived from TCGA database, and identified them as hub genes in the following subnetwork. We firstly identified these key lncRNAs in the ceRNA network of TCGA, then paired their corresponding key miRNAs. Then we matched the key miRNAs with survival-related mRNAs thus to construct the survival-related subnetwork. For survival analysis in CPTAC database, although we failed to identify survival-related mRNAs and miRNAs, we surprisingly discovered that survival-related mRNAs and miRNAs in TCGA database also existed in the ceRNA network constructed by CPTAC database. Therefore, we chose to use these mRNAs, miRNAs and key lncRNAs derived from CPTAC to jointly construct survival-related subnetworks of CPTAC. In our constructed ceRNA subnetwork, there were 6 lncRNA-mediated lncRNA-miRNA-mRNA axes to role in survival outcomes of UCEC patients. A novel survival-related lncRNA DGCR5 could up-regulate CCNE1 expression by binding to miR195, miR383 and miR424, although DGCR5 had not been directly recorded in UCEC tumorigenesis procedures. DiGeorge syndrome critical region gene 5 (DGCR5), a molecular sponge to regulate cancerous signaling pathways, has been previously discovered to be extremely dysregulated in various tumors and induce the malignant phenotypes of organs such as liver, pancreas and lungs, etc. Except for DGCR5, lncRNA LINC00443, LINC00483, C2orf48, TRBV11-2 and MEG8 were identified by multivariate Cox regression analysis, which reveals more accurate ability to predict prognosis.
From multivariate Cox regression analysis in our constructed ceRNA network, we totally identified 10 lncRNA prognostic signature candidates to predict the survival events of UCEC patients. As shown in our ceRNA subnetwork diagram for TCGA, lncRNAs such as LINC00443, C2orf48, LINC00483 could regulate DLX4 and NR3C1 expression by binding to miR-183. LINC00443, LINC00483 and C2orf48 were previously proved to promote carcinoma progression (35), the same consequence of which were validated by our experiment. DLX4 has been shown to cause tumor migration, invasion, and metastasis (36). Previous one in vivo study on UCEC reported that DLX4 promoted cell proliferation, migration, and suggested poor prognosis, which is consistent with our findings (37). NR3C1 encodes glucocorticoid receptors to affect glucocorticoid response and participates in other transcription regulatory procedures. Former studies on miRNA-mRNA regulatory network in UCEC found that over expression of mRNA NR3C1 led to poor prognosis (38,39). Previously in vivo experiments proved that over-expressed LINC00483 promoted UCEC tumorigenesis, the mechanism of which was mainly to sponge with miR-508-3p to regulate RGS17 expression levels (40). In other cancer researches, LINC00483 also acted as a strong ceRNA molecule and exhibited its regulatory ability to mediate tumor progression and prognosis, such as lung adenocarcinoma (41) and gastric cancer (42). Our clinical survival and transcriptome results revealed that patients with over-expression of LINC00443, C2orf48 and LINC00483 had poor prognostic outcomes. The two lncRNAs filtered in multi-Cox regression analysis in CPTAC, TRBV11-2 and MEG8 provided potential pathways to explain ceRNA regulatory network despite of none associated reports for UCEC. Firstly, they could up-regulate SOX11 expression by binding to hsa-mir-363 to bring about poor prognosis, and survival related mRNA SOX11 hypermethylation was reported as a tumor biomarker in UCEC (43). Secondly, MEG8 competed with has-mir-424 to CBX2 and CCNE1 expression as well as competing with has-mir-183 to up-regulate DLX4 and NR3C1 expression. Therefore, we predicted that TRBV11-2 and MEG8 worked as prognostic ceRNAs to up-regulate SOX11, CCNE1 and NR3C1 expression thus resulting in poor prognosis, suggesting that these lncRNAs could promote UCEC development. Furthermore, LINC00028 existed in our survival analysis results rooted from both databases, and the over-expression of LINC00028 indicated a poor prognosis. Besides, LINC00028 has been reported to be involved in ceRNA regulatory network of osteosarcoma recurrence (44). However, its mechanisms related to UCEC pathogenesis remains unclear.
As illustrated in former studies (45,46), UCEC carcinogenesis is promoted by cell cycle acceleration. Similarly, our KEGG analyses in both databases indicated that DEmRNAs were mostly enriched in pathways such as microRNAs in cancer, cellular senescence and proteoglycans in cancer. Deoxyribonucle, and deoxyRNA were identified in tumor and adjacent normal tissue samples in a large cohort of UCEC patients.
TIIC patterns and relevant therapies has captured more recognition, acting as therapeutic targets. Therefore, patterns of immune infiltration before the construction of ceRNA network were analyzed, and significant expressions were founded in CD8 T cells and plasma cells and other TIICs, among UCEC tissues and the adjacent ones, awaiting for more thoroughly data mining.
Noteworthy to be emphasized, there are several limitations in our analyses. Firstly, even though we identified the molecular mechanism of ceRNA from 566 samples in TCGA and 116 samples in CPTAC database, there was still a limited sample size for more reliable biomarkers that hindered us from incorporating data profiles originated from CPTAC and TCGA database into a comprehensive study ideally. Secondly, when conducting TIIC analyses, it came a problem that malignant tissue counts and the control ones (<40) vary drastically, so that analyses of statistical difference between them were unreasonable. Thirdly, although it is generally believed that distinct variability exists in lncRNA expressions of UCEC patients when grouped by different pathological stages, we focused on DElncRNAs between controlled groups versus the whole UCEC tumor tissue ones. This shortcoming of our protocol was determined by not only unfairly distributed cohorts of different pathological grades, but also potentially little positivity of statistical significance reached on the premise of grouped samples.
In further study, more attentions are warranted to be paid to association between pathological stages and prognostic molecular biomarkers when having balanced distribution of UCEC samples at various pathological stages, thus to be more convincing and meaningful. Concerning the pilot study limited by failure to closely link the analysis of the two databases, multi-centric studies are supposed to carry out to support our new researches inevitably.
Conclusions
Using data obtained from CPTAC and TCGA, we screened out lncRNA prognostic signatures on the basis of ceRNA completely composed of hub genes. Besides, an lncRNA-mediated ceRNA network reveals the molecular mechanism that facilitates UCEC pathological progress. LncRNAs including DGCR5, LINC00443, C2orf48, LINC00483, TRBV11-2 and MEG8 involved in lncRNA-miRNA-mRNA regulatory network were identified as promising diagnostic, therapeutic or prognostic biomarkers. Further studies are warranted to explore meaningful biological functional pathways underlying these lncRNA roles for UCEC.
Acknowledgments
Funding: This work was supported by grants from Excellent Key Teachers in the “Qing Lan Project” of Jiangsu Colleges and Universities and “226 Project” of Nantong [No. (2018)III-436].
Footnote
Reporting Checklist: The authors have completed the STREGA checklist. Available at https://tcr.amegroups.com/article/view/10.21037/tcr-22-249/rc
Conflicts of Interest: All authors have completed the ICMJE uniform disclosure form (available at https://tcr.amegroups.com/article/view/10.21037/tcr-22-249/coif). The authors have no conflicts of interest to declare.
Ethical Statement: The authors are accountable for all aspects of the work in ensuring that questions related to the accuracy or integrity of any part of the work are appropriately investigated and resolved. The study was conducted in accordance with the Declaration of Helsinki (as revised in 2013).
Open Access Statement: This is an Open Access article distributed in accordance with the Creative Commons Attribution-NonCommercial-NoDerivs 4.0 International License (CC BY-NC-ND 4.0), which permits the non-commercial replication and distribution of the article with the strict proviso that no changes or edits are made and the original work is properly cited (including links to both the formal publication through the relevant DOI and the license). See: https://creativecommons.org/licenses/by-nc-nd/4.0/.
References
- Calle EE, Kaaks R. Overweight, obesity and cancer: epidemiological evidence and proposed mechanisms. Nat Rev Cancer 2004;4:579-91. [Crossref] [PubMed]
- Janda M, McGrath S, Obermair A. Challenges and controversies in the conservative management of uterine and ovarian cancer. Best Pract Res Clin Obstet Gynaecol 2019;55:93-108. [Crossref] [PubMed]
- Lu KH, Broaddus RR. Endometrial Cancer. N Engl J Med 2020;383:2053-64. [Crossref] [PubMed]
- Engelsen IB, Stefansson I, Akslen LA, et al. Pathologic expression of p53 or p16 in preoperative curettage specimens identifies high-risk endometrial carcinomas. Am J Obstet Gynecol 2006;195:979-86. [Crossref] [PubMed]
- Smith D, Stewart CJR, Clarke EM, et al. ER and PR expression and survival after endometrial cancer. Gynecol Oncol 2018;148:258-66. [Crossref] [PubMed]
- Wright JD, Burke WM, Wilde ET, et al. Comparative effectiveness of robotic versus laparoscopic hysterectomy for endometrial cancer. J Clin Oncol 2012;30:783-91. [Crossref] [PubMed]
- Bartel DP. MicroRNAs: genomics, biogenesis, mechanism, and function. Cell 2004;116:281-97. [Crossref] [PubMed]
- Peng Y, Croce CM. The role of MicroRNAs in human cancer. Signal Transduct Target Ther 2016;1:15004. [Crossref] [PubMed]
- Zhang Z, Gu M, Gu Z, et al. Role of Long Non-Coding RNA Polymorphisms in Cancer Chemotherapeutic Response. J Pers Med 2021;11:513. [Crossref] [PubMed]
- Schmitt AM, Chang HY. Long Noncoding RNAs in Cancer Pathways. Cancer Cell 2016;29:452-63. [Crossref] [PubMed]
- Ahadi A. Functional roles of lncRNAs in the pathogenesis and progression of cancer. Genes Dis 2020;8:424-37. [Crossref] [PubMed]
- Salmena L, Poliseno L, Tay Y, et al. A ceRNA hypothesis: the Rosetta Stone of a hidden RNA language? Cell 2011;146:353-8. [Crossref] [PubMed]
- Yang J, Qiu Q, Qian X, et al. Long noncoding RNA LCAT1 functions as a ceRNA to regulate RAC1 function by sponging miR-4715-5p in lung cancer. Mol Cancer 2019;18:171. [Crossref] [PubMed]
- Kong X, Duan Y, Sang Y, et al. LncRNA-CDC6 promotes breast cancer progression and function as ceRNA to target CDC6 by sponging microRNA-215. J Cell Physiol 2019;234:9105-17. [Crossref] [PubMed]
- Franceschini A, Szklarczyk D, Frankild S, et al. STRING v9.1: protein-protein interaction networks, with increased coverage and integration. Nucleic Acids Res 2013;41:D808-15. [Crossref] [PubMed]
- Smoot ME, Ono K, Ruscheinski J, et al. Cytoscape 2.8: new features for data integration and network visualization. Bioinformatics 2011;27:431-2. [Crossref] [PubMed]
- Chin CH, Chen SH, Wu HH, et al. cytoHubba: identifying hub objects and sub-networks from complex interactome. BMC Syst Biol 2014;8:S11. [Crossref] [PubMed]
- Huang HY, Lin YC, Li J, et al. miRTarBase 2020: updates to the experimentally validated microRNA-target interaction database. Nucleic Acids Res 2020;48:D148-54. [PubMed]
- Wong N, Wang X. miRDB: an online resource for microRNA target prediction and functional annotations. Nucleic Acids Res 2015;43:D146-52. [Crossref] [PubMed]
- Fromm B, Billipp T, Peck LE, et al. A Uniform System for the Annotation of Vertebrate microRNA Genes and the Evolution of the Human microRNAome. Annu Rev Genet 2015;49:213-42. [Crossref] [PubMed]
- Uhlén M, Fagerberg L, Hallström BM, et al. Proteomics. Tissue-based map of the human proteome. Science 2015;347:1260419. [Crossref] [PubMed]
- Siegel RL, Miller KD, Fuchs HE, et al. Cancer statistics, 2022. CA Cancer J Clin 2022;72:7-33. [Crossref] [PubMed]
- Shi X, Sun M, Liu H, et al. Long non-coding RNAs: a new frontier in the study of human diseases. Cancer Lett 2013;339:159-66. [Crossref] [PubMed]
- Huo X, Sun H, Cao D, et al. Identification of prognosis markers for endometrial cancer by integrated analysis of DNA methylation and RNA-Seq data. Sci Rep 2019;9:9924. [Crossref] [PubMed]
- Ouyang D, Li R, Li Y, et al. A 7-lncRNA signature predict prognosis of Uterine corpus endometrial carcinoma. J Cell Biochem 2019;120:18465-77. [Crossref] [PubMed]
- Dong M, Dong Z, Zhu X, et al. Long non-coding RNA MIR205HG regulates KRT17 and tumor processes in cervical cancer via interaction with SRSF1. Exp Mol Pathol 2019;111:104322. [Crossref] [PubMed]
- Li Y, Wang H, Huang H. Long non-coding RNA MIR205HG function as a ceRNA to accelerate tumor growth and progression via sponging miR-122-5p in cervical cancer. Biochem Biophys Res Commun 2019;514:78-85. [Crossref] [PubMed]
- Xia L, Wang Y, Meng Q, et al. Integrated Bioinformatic Analysis of a Competing Endogenous RNA Network Reveals a Prognostic Signature in Endometrial Cancer. Front Oncol 2019;9:448. [Crossref] [PubMed]
- Zhang X, Feng H, Li Z, et al. Application of weighted gene co-expression network analysis to identify key modules and hub genes in oral squamous cell carcinoma tumorigenesis. Onco Targets Ther 2018;11:6001-21. [Crossref] [PubMed]
- Kuhn E, Bahadirli-Talbott A, Shih IeM. Frequent CCNE1 amplification in endometrial intraepithelial carcinoma and uterine serous carcinoma. Mod Pathol 2014;27:1014-9. [Crossref] [PubMed]
- Leskela S, Pérez-Mies B, Rosa-Rosa JM, et al. Molecular Basis of Tumor Heterogeneity in Endometrial Carcinosarcoma. Cancers (Basel) 2019;11:964. [Crossref] [PubMed]
- Nakayama K, Rahman MT, Rahman M, et al. CCNE1 amplification is associated with aggressive potential in endometrioid endometrial carcinomas. Int J Oncol 2016;48:506-16. [Crossref] [PubMed]
- Zhang Y, Li X, Zhang J, et al. E6 hijacks KDM5C/lnc_000231/miR-497-5p/CCNE1 axis to promote cervical cancer progression. J Cell Mol Med 2020;24:11422-33. [Crossref] [PubMed]
- Yang R, Xing L, Zheng X, et al. The circRNA circAGFG1 acts as a sponge of miR-195-5p to promote triple-negative breast cancer progression through regulating CCNE1 expression. Mol Cancer 2019;18:4. [Crossref] [PubMed]
- Liu J, Nie S, Liang J, et al. Competing endogenous RNA network of endometrial carcinoma: A comprehensive analysis. J Cell Biochem 2019;120:15648-60. [Crossref] [PubMed]
- Zhang L, Yang M, Gan L, et al. DLX4 upregulates TWIST and enhances tumor migration, invasion and metastasis. Int J Biol Sci 2012;8:1178-87. [Crossref] [PubMed]
- Zhang L, Wan Y, Jiang Y, et al. Overexpression of BP1, an isoform of Homeobox Gene DLX4, promotes cell proliferation, migration and predicts poor prognosis in endometrial cancer. Gene 2019;707:216-23. [Crossref] [PubMed]
- Ding H, Fan GL, Yi YX, et al. Prognostic Implications of Immune-Related Genes' (IRGs) Signature Models in Cervical Cancer and Endometrial Cancer. Front Genet 2020;11:725. [Crossref] [PubMed]
- Sun R, Liu J, Nie S, et al. Construction of miRNA-mRNA Regulatory Network and Prognostic Signature in Endometrial Cancer. Onco Targets Ther 2021;14:2363-78. [Crossref] [PubMed]
- Hu P, Zhou G, Zhang X, et al. Long non-coding RNA Linc00483 accelerated tumorigenesis of cervical cancer by regulating miR-508-3p/RGS17 axis. Life Sci 2019;234:116789. [Crossref] [PubMed]
- Yang S, Liu T, Sun Y, et al. The long noncoding RNA LINC00483 promotes lung adenocarcinoma progression by sponging miR-204-3p. Cell Mol Biol Lett 2019;24:70. [Crossref] [PubMed]
- Li D, Yang M, Liao A, et al. Linc00483 as ceRNA regulates proliferation and apoptosis through activating MAPKs in gastric cancer. J Cell Mol Med 2018;22:3875-86. [Crossref] [PubMed]
- Shan T, Uyar DS, Wang LS, et al. SOX11 hypermethylation as a tumor biomarker in endometrial cancer. Biochimie 2019;162:8-14. [Crossref] [PubMed]
- Zhang S, Ding L, Li X, et al. Identification of biomarkers associated with the recurrence of osteosarcoma using ceRNA regulatory network analysis. Int J Mol Med 2019;43:1723-33. [Crossref] [PubMed]
- Wang Y, Qiu H, Hu W, et al. RPRD1B promotes tumor growth by accelerating the cell cycle in endometrial cancer. Oncol Rep 2014;31:1389-95. [Crossref] [PubMed]
- Xiong H, Li Q, Chen R, et al. A Multi-Step miRNA-mRNA Regulatory Network Construction Approach Identifies Gene Signatures Associated with Endometrioid Endometrial Carcinoma. Genes (Basel) 2016;7:26. [Crossref] [PubMed]