In silico development and validation of a novel glucose and lipid metabolism-related gene signature in gastric cancer
Introduction
Gastric cancer (GC) is among the most common and lethal digestive malignancy worldwide, according for 768,793 deaths in 2020 (1). Most patients with GC are frequently detected at later stages due to atypical and unobvious symptoms. Despite great progress in GC treatment, the 5-year survival rate for patients with advanced GC still need to be improved (2,3). The high recurrence and metastasis rate lead to shorter survival (4). Therefore, there is an urgent need to explore the mechanism of tumorigenesis and identify prognostic biomarkers of GC.
Emerging evidence has confirmed that there is a systemic link between tumor growth and metabolic pathways. Aberrant metabolic reprogramming, especially glycolysis and lipid metabolism, is currently considered a hallmark feature of cancers, including GC (5-7). Glucose metabolic reprogramming maintains the acquisition of energy allowing cancer cells to survive during different disease states (8,9). Glucose metabolism is also highly correlated to lipid metabolism (10). The lipid metabolic reprogramming refers to the sufficient supply of energy and the synthesis of structural and functional lipids, thereby satisfying the demands of increased membrane biogenesis in cancer cells (11,12). The dysfunctional metabolic programming based on glucose and lipid metabolism has also been proven to contribute to tumor metastasis, chemotherapy drug resistance, and immune escape (13). However, glucose and lipid metabolism have a high level of complexity in its regulation. No such risk model has been established based on glucose and lipid metabolism related genes. Moreover, the potential mechanism in relation to disease is still unknown. Several coding or/and noncoding RNAs have been shown to mediate a wide range of biological processes in cancer cells, including glucose and lipid metabolism (14,15). These genes exert direct or indirect effects on glucose and lipid metabolism both in vivo and in vitro.
To enhance our understanding of glucose and lipid metabolism in GC, it is necessary to perform an integrative analysis of the potential roles of glucose and lipid metabolism related genes. In this study, we identified the metabolic subtypes based on the expression of genes related to glycolysis and lipid metabolism. A GC prognostic signature was established and verified based on glucose and lipid metabolism-related genes. In addition, we constructed a nomogram based on the prognostic signature and clinical features to improve the markers’ clinical utility. Finally, we also clarified the relationship between prognostic signature and other in patients with GC, including the signaling pathway and tumor microenvironment. This study might provide research clues for the accurate prevention and treatment of GC. We present the following article in accordance with the TRIPOD reporting checklist (available at https://tcr.amegroups.com/article/view/10.21037/tcr-22-168/rc).
Methods
Data source
The RNA sequencing data and the corresponding clinical information of GC patients were downloaded from The Cancer Genome Atlas (TCGA) and Gene Expression Omnibus (GEO) dataset (Table S1), respectively. Subsequently, the Fragments per Kilobase Million (FPKM) data were normalized to transcripts per kilobase million (TPM) values. The expression values in GSE84437 and GSE62254 dataset [the Asian Cancer Research Group (ACRG) cohort] were performed to log2-transformed. Four samples without clinical follow-up information were removed. TCGA-Stomach Adenocarcinoma (STAD) (371 samples) and GSE84437 (433 samples) data sets were merged to produce the final training set (804 samples), and GSE62254 (300 samples) served as the final validation set. The R package SVA was utilized to perform batch effect removal on the different data sets. This study was conducted in accordance with the Declaration of Helsinki (as revised in 2013). The flowchart is shown in Figure 1.
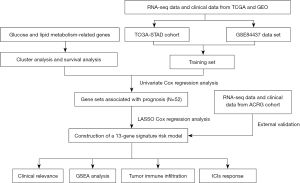
Glucose and lipid metabolism gene set
Four glucose metabolism datasets [Kyoto Encyclopedia of Genes and Genomes (KEGG) glycolysis gluconeogenesis, Hallmark glycolysis, Reactome regulation of glycolysis, and Reactome glycolysis] and four lipid metabolism datasets (KEGG glycerophospholipid metabolism, hallmark fatty acid metabolism, Reactome metabolism of lipids, and Reactome phospholipid metabolism) were obtained from MSigDB (https://www.gsea-msigdb.org/gsea/msigdb) and chosen as reference gene sets. A total of 1,118 glucose and lipid metabolism related genes were selected for the study (Tables S2,S3).
Using the consensus clustering algorithm to identify molecular typing based on glucose and lipid metabolism-related genes
Cluster analysis algorithms were gradually utilized to explore hidden groupings, and exhibited a good performance. In this study, a consensus clustering analysis was utilized to cluster samples of training set using the R package ConsensusClusterPlus. The parameters were shown below: number of repetitions =1,000, number of clusters from 2 to10, pFeature =1, and pItem =0.8. According to the inflection point, as illustrated in Figure 2, the optimal number of clusters was further determined. To evaluate the prognostic implication of glucose and lipid metabolism-related genes, the Kaplan-Meier curve was selected to compare the overall survival (OS) of the different subgroups.
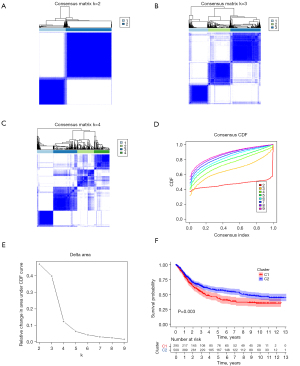
Lasso cox regression analysis
Lasso regression analysis was conducted to perform variable selection, which could compress variables to overfit the risk mode (16,17). Certain coefficients were compressed via setting others to zero to determine the optimal factor (16,17). Ten-fold cross-validation were used running lasso regression to avoid overfitting. Subsequently, these factors were utilized to establish an optimized model based on multivariate Cox regression analysis. Patients with GC were segregated into high- and low-risk groups using the median risk score value. Kaplan-Meier method was used to assess the difference in survival outcomes between two groups. The receiver operating characteristic (ROC) curve was plotted to evaluate the performance of the risk model. The risk model was evaluated using a validation set, which was consistent with its coefficient from training set.
Construction of a nomogram
Univariate and multivariate Cox regression analyses were performed to choose potential risk factors. The clinical variable was added to the nomogram model for further analysis. The nomogram was utilized to predict the 1-, 3-, and 5-year OS rate with the R package rms. The calibration and ROC curve were applied to estimate the performance of the nomogram model.
Gene set enrichment analysis (GSEA)
To elucidate relevant biological significance of risk score, GSEA analysis was utilized to expound on the noteworthy differences between the high- and low-risk groups. We performed 1,000 repetitions of gene set permutations, and utilized the normalized enrichment score (NES) as evaluation metrics. The thresholds were set as false discovery rate (FDR) <0.05.
Evaluation of immune scores and immune infiltration
To evaluate the immune status of different samples, we performed ESTIMATE and single-sample GSEA (ssGSEA) algorithms to explore differences between the different groups. The ESTIMATE algorithms were used to analyze the immunological characteristics of the high- and low-risk groups. In addition, we performed ssGSEA to analyze the immune infiltration statuses and relevant immune-related pathways based on the R package gsva.
Exploration of the potential susceptibility to anti-cancer therapy
To explore the potential effect of anti-cancer therapy, we also evaluated the correlation between the expression of key immune checkpoint genes. Besides, we performed correlation analysis based on Pearson method using R software.
Statistical analysis
All the statistical analyses were conducted with R software (Version 3.6.3). P<0.05 or FDR <0.05 was served as statistically significant.
Results
Cluster analysis based on glucose and lipid metabolism-related genes
The consensus clustering algorithm was used to cluster the training set samples (804 samples) through the R package ConsensusClusterPlus. Due to higher inter-group correlation when k=4 not k=2, the optimal number of clusters selected was 2 (Figure 2A-2E). Namely, the GC samples in the training set could be clustered into subtypes, named cluster 1 and cluster 2, based on glucose and lipid metabolism-related genes. Besides, the survival analysis was further utilized to evaluate the prognostic value of subcluster. As shown in Figure 2F, patients in cluster 2 showed more favorable OS rates than patients of cluster 1 (P=0.003) (Figure 2F). The result showed that glucose and lipid metabolism-related genes are associated with prognosis in GC.
Identification of glucose and lipid metabolism-related prognostic genes
Next, univariate Cox analysis was employed to identify the potential prognostic value of these glucose and lipid metabolism-related genes in the training set. P value less than 0.01 was selected as the threshold for filtering, and 52 genes were significantly associated with OS. These genes were selected for further analysis.
Construction and validation of the prognostic risk model in different datasets
The R package glmnet was utilized to perform Lasso-penalized Cox regression analysis for 52 genes with potential prognostic value based on training set. The optimal lambda value was associated with the determinants of penalized term. As shown in Figure S1, we obtained the theoretically optimal model when lambda =13. Therefore, 13 genes were eventually selected for detailed model building. The coefficient of risk score formula was shown in Table 1. To assess the model’s robustness, the Asian Cancer Research Group (ACRG) cohort was introduced as the independent validation datasets. Kaplan-Meier curve was further drawn to show the survival status of the GC samples (Figure 3A). This study suggested that high RiskScore sample has a worse prognosis. The same coefficients as that from the training set were utilized to obtain the risk score of each patient according to the expression level. The survival status of the GC samples in the high-risk group was still worse than that of in the low-risk group (Figure 3B). Subsequently, we evaluated 1-, 3-, and 5-year predictive capability based on ROC. As shown in Figure 3C, the model has a good area under curve (AUC) value, and the AUC values calculated from TCGA for 1, 3, and 5 years were 0.611, 0.619, and 0.641, respectively. Besides, the validation results indicated that the AUC value of the glucose and lipid metabolism-related prognostic signatures was 0.635 in 1 year, 0.644 in 3 years, and 0.634 in 5 years (Figure 3D). Moreover, we calculated the risk score based on the expression level of these prognostic genes, and the samples were divided into the high- and low-risk groups based on the median risk score (Figure 3E). A high proportion of deaths with GC were still shown in the high-risk group, which was consistent with the training set (Figure 3F). The above results showed that our model has good robustness, and could support a stable potential for a predictive prognostic signature.
Table 1
Gene | Coefficient |
---|---|
CACNA1H | 0.0073492701064191 |
CHST1 | 0.0481537527082623 |
IGFBP3 | 0.0350611211681865 |
NASP | −0.0014241982646828 |
STC1 | 0.0830987998258511 |
VCAN | 0.0209038567717716 |
NUP205 | −0.076027779878957 |
NUP43 | −0.108261343313484 |
PGM2L1 | 0.10183699405434 |
CAV1 | 0.0559587258963077 |
ELOVL4 | 0.0506717462447253 |
PRKAA2 | 0.0994553688728891 |
TNFAIP8L3 | 0.00733755211794015 |
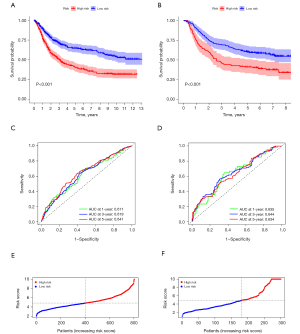
The relationship between risk score and clinical variables
Univariate and multivariate Cox regression analyses were selected for evaluation of the independent prognostic predictor successively. We demonstrated that the age [P<0.001, hazard ratio (HR) =1.026, 95% confidence interval (CI): 1.016–1.036], T (P=0.001, HR =1.255, 95% CI: 1.093−1.442), N (P<0.001, HR =1.549, 95% CI: 1.383–1.735), and risk score (P<0.001, HR =1.241, 95% CI: 1.162−1.326) were significantly correlated with OS in the training set (Figure 4A). After adjusting for confounding factors, the age (P<0.001, HR =1.029, 95% CI: 1.019−1.039), N (P<0.001, HR =1.467, 95% CI: 1.305−1.649), and risk score (P<0.001, HR =1.223, 95% CI: 1.143−1.309) were confirmed to be independent prognostic factors by multivariate Cox regression analysis (Figure 4B). Similarly, univariate and multivariate Cox regression analyses showed the age, N, and risk score were independent prognostic factors as those in external dataset (Figure 4C,4D).
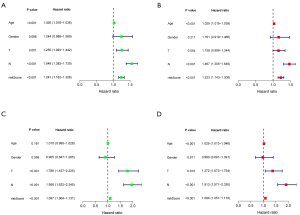
Establishment of a nomogram model to further improve the predictive power
To better improve the predictive potential of GC patients, a nomogram was then constructed to facilitate prediction of OS. The clinical variable, including age, gender, T, and N, was added to the nomogram model. As shown in Figure 5, the calibration curve for the nomogram is close to the 45° line, which indicated a good performance of the nomogram. Figure S2 showed that the nomogram model presents good accuracy in predicting OS, with AUC values of 0.715, 0.736, and 0.743 at 1, 3, and 5 years, respectively, in the training set. Besides, the nomogram model was shown with the AUC values at 1, 3 and, 5 years were 0.791, 0.761, and 0.723, respectively.
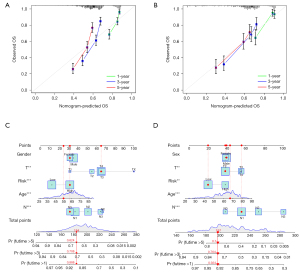
Evaluation the degree of correlation between risk score and immune score
ESTIMATE algorithm was used to calculate each GC sample’s immune and matrix scores based on R package estimate. As shown in Figure 6, there were significant differences in the StromalScore, ImmuneScore, and ESTIMATEScore between the two groups in the training set (Figure 6A-6C). Besides, these significant differences were still existed in the independent validation ACRG cohort (Figure 6D-6F). In both cohort, patients in the high-risk group had a higher StromalScore, ImmuneScore, and ESTIMATEScore. All these data demonstrated that the risk score constructed by glucose and lipid metabolism-related genes might correlate with immune response.
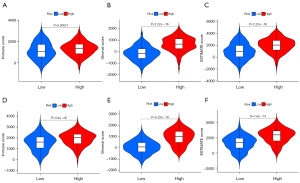
Exploration of relation between the risk score and pathways
To elucidate the potential links between the different samples’ risk scores and underlying biological significance, we performed GSEA analysis on the two risk-score groups. The results showed that several cancer-related pathways and immune-related pathways were significantly enriched in the high-risk group. Among them, the top five pathways in the high-risk group, include KEGG_CALCIUM_SIGNALING_PATHWAY, KEGG_CYTOKINE_CYTOKINE_RECEPTOR_INTERACTION, KEGG_FOCAL_ADHESION, KEGG_NEUROACTIVE_LIGAND_RECEPTOR_INTERACTION, KEGG_PATHWAYS_IN_CANCER and KEGG_AMINOACYL_TRNA_BIOSYNTHESIS, KEGG_GLYOXYLATE_AND_DICARBOXYLATE_METABOLISM, KEGG_HOMOLOGOUS_RECOMBINATION, KEGG_MISMATCH_REPAIR, KEGG_ONE_CARBON_POOL_BY_FOLATE in the low-risk group (Figure 7A,7B).

Three pathways (KEGG_CYTOKINE_CYTOKINE_RECEPTOR_INTERACTION, KEGG_FOCAL_ADHESION, KEGG_NEUROACTIVE_LIGAND_RECEPTOR_INTERACTION) in the high-risk group and two pathways (KEGG_AMINOACYL_TRNA_BIOSYNTHESIS, KEGG_GLYOXYLATE_AND_DICARBOXYLATE_METABOLISM) in the low-risk group were validated by the ACRG cohort, respectively (Figure 7C,7D). These results suggested that these pathways’ imbalance was related closely to GC development.
Correlation between the risk score and tumor immune infiltration
To further explore the correlation between the risk score and tumor immune infiltration, ssGSEA algorithm was applied to find the differences in infiltration of primary immune cells in GC samples. We revealed that several types of tumor-infiltrating immune cells were enriched in the high-risk group, including dendritic cells (DCs), macrophages, mast cells, neutrophils, and tumor-infiltrating lymphocyte (TIL). These results demonstrated good consistency between the training and validation set (Figure 8A,8B). Besides, we could differentiate between the high- and low-risk groups in both cohorts based on contents of the antigen presentation process, including chemokine-chemokine receptor (CCR), major histocompatibility complex (MHC) class I, parainflammation, and type II interferon (IFN) response (Figure 8C,8D). Interestingly, the risk score was negatively correlated to MHC class I, suggesting there was a potential trend in the high-risk group toward enhanced immunosuppression compared with the low-risk group. The result was further confirmed by the findings of immune checkpoint inhibitors (ICIs).
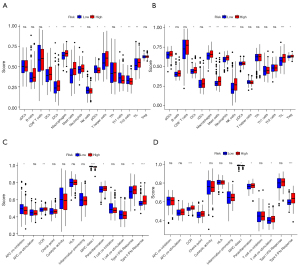
Evaluation of the association between the risk model and anti-cancer therapy
To evaluate the potential clinical application for GC treatment, we attempted to investigate the potential susceptibility to ICIs. We firstly assessed the correlation between risk scores and several genes affecting ICIs. Our study revealed that the risk score was related to the expressions of FAP, TAGLN, LOXL2, whereas it was negatively associated with POLE2, FEN1, MCM6, MSH6, MSH2 (Figure 9A,9B). Furthermore, we investigated the potential susceptibility to ICIs based on the expression level of the immune regulator. The results indicated that the expressions of CD200, CD44, TNFRSF4, NRP1, CD276, CD48, and CD28 were elevated in the high-risk groups, whereas the expression of HHLA2 was downregulated (Figure 9C,9D). Our results indicated that there might exist an immunosuppressive status in the high-risk groups.
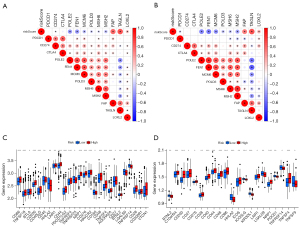
Discussion
Several macromolecules, such as proteins, nucleic acids, and lipids, have been proven to contribute to cell growth and proliferation via the production of metabolic intermediates (18,19). These agents act as the precursors necessary for life, which divert biological media from biomass production to improve organismal or cell viability (18,19). Accumulating evidence indicates that abnormal metabolic reprogramming is critically involved in the oncogenic signaling pathways, and has emerged as a hallmark of cancer (20-22). The results suggest metabolic markers might be possible therapeutic targets. As increasingly high-throughput techniques emerge, the novel technical means provide valuable molecular information to understand the potential roles of glucose and lipid metabolism. Previous studies demonstrated that a variety of biological characteristics could alter metabolic phenotype during a period of the transformation of normal cells into malignant cells, including enhanced glucose uptake and reactivation of de novo lipid biosynthesis (20-22). In addition, glucose provides energy and precursors for biosynthetic processes, and lipids function as an important component of some pathways and cell-cell communication (23-25). There is tight connection between oncogenic transformation and abnormal metabolic reprogramming. However, no specific glucose and lipid metabolism related model has been developed for GC to predict prognosis and treatment response until now. Therefore, we constructed a risk model based on glucose and lipid metabolism gene signatures that could act as prognostic and therapeutic biomarker in GC.
Previous study confirmed that GC has been identified as a glycolytic-enhanced malignancy, and metabolomic studies have shown that there exists a predominance of lipid storage over consumption in gastric epithelial neoplasms (26-29). In this study, we have applied the consensus clustering algorithm to the data set, and identified two subtypes of GC first based on genes related to glucose and lipid metabolism. Further, univariable regression analysis was performed to screen out OS-related gene sets, and 52 genes were obtained to display the cancer-related functions. Finally, the 13-gene risk signature was constructed by lasso penalized regression and multivariate Cox analysis, and demonstrated that the signature had high reliability and stable predictability. The application of Nomogram improved the predictive accuracy of the model notably, which could provide support to clinical decision-making.
The gene signature was constructed with CACNA1H, CHST1, IGFBP3, NASP, STC1, VCAN, NUP205, NUP43, PGM2L1, CAV1, ELOVL4, PRKAA2, and TNFAIP8L3, which was correlated significantly with the OS and tumor immune microenvironment (TIME). Wang et al. reported that stanniocalcin-1 (STC1) positively regulated Bcl-2 to mediate gastric carcinogenesis, metastasis and chemoresistance under hypoxia, indicating that the carcinogenic and therapeutic roles of STC1 in GC (30). Wang et al. found that Caveolin‑1 could enhance RANKLinduced multi-cancer cells migration, including stomach, lung, renal and breast cancer cells (31). Rao et al. indicated that the signaling pathway STK11-PRKAA2-ULK1 was linked to Gastrin-mediated autophagy activation in gastric adenocarcinoma cells (32). Jimenez et al. demonstrated that CACNA1H mediates the antiproliferative effects and promotes cancer stem cell suppression in hepatocellular carcinoma following exposure to tumor-specific electromagnetic fields (33). Tu et al. found that cancer-related gene vasohibin-2 was critically involved in the proliferation of breast cancer cells by activating insulinlike growth factors, including insulin like growth factor binding protein 3 (IGFBP3) and insulin like growth factor binding protein 6 (IGFBP6) (34). Kang et al. indicated that nuclear autoantigenic sperm protein (NASP) knockdown could facilitate transcription release and failure of replication initiation in hepatocellular carcinoma, which was achieved by abolished the supply of histone H3 and enhanced chromatin accessibility (35). Several bioinformatic analyses were performed to VCAN in GC without further exploration of the mechanisms using in vivo/in vitro experiments (36-38). Tian et al. found that NUP43 might predict prognosis in luminal A and in HER2+ breast tumors based on bioinformatics analysis (39). However, the potential roles of CHST1, NUP205, PGM2L1, ELOVL4, and TNFAIP8L3 remain unclear. Their roles need to be classified based on further validation.
Increasing evidence has confirmed that the TIME was strongly associated with the disease development and progression. In this study, the relationship between the risk scores and TIME in GC was under comprehensive evaluation. We demonstrated that GC patients with high-risk scores had higher StromalScore, ImmuneScore, and ESTIMATEScore than those with low-risk scores. Previous study has found that GC patients with high stromal scores had a better prognosis, which was consistent with the present study (40). In-depth analysis of immune infiltrates indicated that the risk signature was correlated positively with the expression of DCs, Macrophages, mast cells, neutrophils, and TIL. Additionally, the risk score was negatively correlated to MHC class I. MHC class I plays the role of a gatekeeper, which promotes pathogens and transformed cells recognition via displaying peptides to CD8+ T cells (41). For the tumor to arise and progress, the evolutionary mechanism will be dominant to avoid elimination by CD8+ T cells and hence the immune response (41). Down-regulation of the MHC class I expression at the cell surface was considered one of the hallmarks of immune escape, which significantly impaired the ability of CD8+ T cells to recognize the cancer cells (42). The present study indicated that the down-regulation of the MHC class I expression was more evident in our high-risk group, suggesting there might exist the reduction of tumor-associated antigenic epitopes to facilitate immune evasion. In addition, among the immune checkpoint markers, the expressions of CD200, CD44, TNFRSF4, NRP1, CD276, CD48, and CD28 were highly expressed in the high-risk groups, whereas the expression of HHLA2 was downregulated. The result indicated that GC patients grouped by this risk signature showed differences in responsiveness to immunotherapy. CD200 and CD44 are stem cell-specific markers that play an important role in immunosuppression, which are highly expressed in several cancers and are correlated with unfavorable prognosis (43,44). Blocking CD200 or CD44 inhibit immune activation, and contribute to improve the efficacy of immunotherapy (45,46). TNFRSF4, also known as OX40L, is expressed on regulatory T cells, which promotes immune escape of leukemia stem cells (47). The high expression of NRP1 is associated with the poor prognosis of several cancers, which may assist cancer immune escape and enhance tumor progression via mediating Treg cell infiltration (48). CD276, also known as B7-H3, has been detected in several malignancies and associated with tumor progression and poor outcome (49,50). Agonistic targeting of CD276 can enhance immune surveillance and promote the anti-tumor response (51,52). CD28 is a T cells costimulatory factors, which transduces a positive signal to enhance the proliferation of T cells (53). Besides, CD28 ligation can also promote the production of various cytokines, such as IL-2, IL-4 and IL-10 (53). However, the role of CD28 in Treg cell differentiation seems to be determined by some other conditions, such as the level of T cell receptor (TCR) engagement and cytokine environment. Although HHLA2 functions as the inhibitor of CD4+ and CD8+ T cells and is helpful for cancer immunotherapy (54), the potential roles in GC deserve further study.
Although we performed this study based on a large sample of data sets, there were still several limitations about this study. First, this is a retrospective analysis, and all the data were obtained from public databases. Our risk signature must be further validated by prospective cohort. Secondly, high-risk factors for GC, such as unhealthy diets (particularly diets high in salt) and infections with Helicobacter pylori, were not included in this study. These factors might also affect GC patients’ prognosis. Thirdly, the AUC value ranging from 0.6 to 0.7 is not very satisfactory. We constructed the nomogram model to improve it, and showed good performance. However, there still needs more accurate model later through developing novel algorithms. Finally, although GSEA is an accepted method for functional analysis, the potential mechanisms mediated by the glucose and lipid metabolism-related risk genes must be further explored comprehensively and in-depth.
Conclusions
In this study, we constructed a robust 13-gene signature prognostic risk model in different datasets that was better for prediction of prognosis and treatment response. It will provide novel insights into exploring the immunoregulation roles of these genes.
Acknowledgments
We acknowledge TCGA and GEO databases for providing the data.
Funding: This study was supported by the National Natural Science Foundation of China (Nos. 71964021, 81960430), and Natural Science Foundation of Gansu province (No. 21JR1RA117).
Footnote
Reporting Checklist: The authors have completed the TRIPOD reporting checklist. Available at https://tcr.amegroups.com/article/view/10.21037/tcr-22-168/rc
Data Sharing Statement: Available at https://tcr.amegroups.com/article/view/10.21037/tcr-22-168/dss
Conflicts of Interest: All authors have completed the ICMJE uniform disclosure form (available at https://tcr.amegroups.com/article/view/10.21037/tcr-22-168/coif). The authors have no conflicts of interest to declare.
Ethical Statement: The authors are accountable for all aspects of the work in ensuring that questions related to the accuracy or integrity of any part of the work are appropriately investigated and resolved. The study was conducted in accordance with the Declaration of Helsinki (as revised in 2013).
Open Access Statement: This is an Open Access article distributed in accordance with the Creative Commons Attribution-NonCommercial-NoDerivs 4.0 International License (CC BY-NC-ND 4.0), which permits the non-commercial replication and distribution of the article with the strict proviso that no changes or edits are made and the original work is properly cited (including links to both the formal publication through the relevant DOI and the license). See: https://creativecommons.org/licenses/by-nc-nd/4.0/.
References
- Sung H, Ferlay J, Siegel RL, et al. Global Cancer Statistics 2020: GLOBOCAN Estimates of Incidence and Mortality Worldwide for 36 Cancers in 185 Countries. CA Cancer J Clin 2021;71:209-49. [Crossref] [PubMed]
- Sexton RE, Al Hallak MN, Diab M, et al. Gastric cancer: a comprehensive review of current and future treatment strategies. Cancer Metastasis Rev 2020;39:1179-203. [Crossref] [PubMed]
- Rugge M, Genta RM, Di Mario F, et al. Gastric Cancer as Preventable Disease. Clin Gastroenterol Hepatol 2017;15:1833-43. [Crossref] [PubMed]
- Banks M, Graham D, Jansen M, et al. British Society of Gastroenterology guidelines on the diagnosis and management of patients at risk of gastric adenocarcinoma. Gut 2019;68:1545-75. [Crossref] [PubMed]
- Snaebjornsson MT, Janaki-Raman S, Schulze A. Greasing the Wheels of the Cancer Machine: The Role of Lipid Metabolism in Cancer. Cell Metab 2020;31:62-76. [Crossref] [PubMed]
- Abbassi-Ghadi N, Kumar S, Huang J, et al. Metabolomic profiling of oesophago-gastric cancer: a systematic review. Eur J Cancer 2013;49:3625-37. [Crossref] [PubMed]
- Liu Y, Zhang Z, Wang J, et al. Metabolic reprogramming results in abnormal glycolysis in gastric cancer: a review. Onco Targets Ther 2019;12:1195-204. [Crossref] [PubMed]
- Vaupel P, Schmidberger H, Mayer A. The Warburg effect: essential part of metabolic reprogramming and central contributor to cancer progression. Int J Radiat Biol 2019;95:912-9. [Crossref] [PubMed]
- Li Z, Zhang H. Reprogramming of glucose, fatty acid and amino acid metabolism for cancer progression. Cell Mol Life Sci 2016;73:377-92. [Crossref] [PubMed]
- Brault C, Schulze A. The Role of Glucose and Lipid Metabolism in Growth and Survival of Cancer Cells. Recent Results Cancer Res 2016;207:1-22. [Crossref] [PubMed]
- Cheng C, Geng F, Cheng X, et al. Lipid metabolism reprogramming and its potential targets in cancer. Cancer Commun (Lond) 2018;38:27. [Crossref] [PubMed]
- Boroughs LK, DeBerardinis RJ. Metabolic pathways promoting cancer cell survival and growth. Nat Cell Biol 2015;17:351-9. [Crossref] [PubMed]
- Chen L, Chen XW, Huang X, et al. Regulation of glucose and lipid metabolism in health and disease. Sci China Life Sci 2019;62:1420-58. [Crossref] [PubMed]
- Lu W, Cao F, Wang S, et al. LncRNAs: The Regulator of Glucose and Lipid Metabolism in Tumor Cells. Front Oncol 2019;9:1099. [Crossref] [PubMed]
- Hussain A, Qazi AK, Mupparapu N, et al. Modulation of glycolysis and lipogenesis by novel PI3K selective molecule represses tumor angiogenesis and decreases colorectal cancer growth. Cancer Lett 2016;374:250-60. [Crossref] [PubMed]
- Simon N, Friedman J, Hastie T, et al. Regularization Paths for Cox's Proportional Hazards Model via Coordinate Descent. J Stat Softw 2011;39:1-13. [Crossref] [PubMed]
- Tibshirani R. The lasso method for variable selection in the Cox model. Stat Med 1997;16:385-95. [Crossref] [PubMed]
- Melone MAB, Valentino A, Margarucci S, et al. The carnitine system and cancer metabolic plasticity. Cell Death Dis 2018;9:228. [Crossref] [PubMed]
- Li C, Zhang G, Zhao L, et al. Metabolic reprogramming in cancer cells: glycolysis, glutaminolysis, and Bcl-2 proteins as novel therapeutic targets for cancer. World J Surg Oncol 2016;14:15. [Crossref] [PubMed]
- Faubert B, Solmonson A, DeBerardinis RJ. Metabolic reprogramming and cancer progression. Science 2020;368:eaaw5473. [Crossref] [PubMed]
- Pavlova NN, Thompson CB. The Emerging Hallmarks of Cancer Metabolism. Cell Metab 2016;23:27-47. [Crossref] [PubMed]
- DeBerardinis RJ, Chandel NS. Fundamentals of cancer metabolism. Sci Adv 2016;2:e1600200. [Crossref] [PubMed]
- Mobasheri A. Glucose: an energy currency and structural precursor in articular cartilage and bone with emerging roles as an extracellular signaling molecule and metabolic regulator. Front Endocrinol (Lausanne) 2012;3:153. [Crossref] [PubMed]
- Record M, Carayon K, Poirot M, et al. Exosomes as new vesicular lipid transporters involved in cell-cell communication and various pathophysiologies. Biochim Biophys Acta 2014;1841:108-20. [Crossref] [PubMed]
- Fahy E, Cotter D, Sud M, et al. Lipid classification, structures and tools. Biochim Biophys Acta 2011;1811:637-47. [Crossref] [PubMed]
- Yuan LW, Yamashita H, Seto Y. Glucose metabolism in gastric cancer: The cutting-edge. World J Gastroenterol 2016;22:2046-59. [Crossref] [PubMed]
- Ruzzo A, Graziano F, Bagaloni I, et al. Glycolytic competence in gastric adenocarcinomas negatively impacts survival outcomes of patients treated with salvage paclitaxel-ramucirumab. Gastric Cancer 2020;23:1064-74. [Crossref] [PubMed]
- Abdel-Wahab AF, Mahmoud W, Al-Harizy RM. Targeting glucose metabolism to suppress cancer progression: prospective of anti-glycolytic cancer therapy. Pharmacol Res 2019;150:104511. [Crossref] [PubMed]
- Enjoji M, Kohjima M, Ohtsu K, et al. Intracellular mechanisms underlying lipid accumulation (white opaque substance) in gastric epithelial neoplasms: A pilot study of expression profiles of lipid-metabolism-associated genes. J Gastroenterol Hepatol 2016;31:776-81. [Crossref] [PubMed]
- Wang Y, Qi Z, Zhou M, et al. Stanniocalcin-1 promotes cell proliferation, chemoresistance and metastasis in hypoxic gastric cancer cells via Bcl-2. Oncol Rep 2019;41:1998-2008. [Crossref] [PubMed]
- Wang Y, Song Y, Che X, et al. Caveolin-1 enhances RANKL-induced gastric cancer cell migration. Oncol Rep 2018;40:1287-96. [Crossref] [PubMed]
- Rao SV, Solum G, Niederdorfer B, et al. Gastrin activates autophagy and increases migration and survival of gastric adenocarcinoma cells. BMC Cancer 2017;17:68. [Crossref] [PubMed]
- Jimenez H, Wang M, Zimmerman JW, et al. Tumour-specific amplitude-modulated radiofrequency electromagnetic fields induce differentiation of hepatocellular carcinoma via targeting Cav3.2 T-type voltage-gated calcium channels and Ca2+ influx. EBioMedicine 2019;44:209-24. [Crossref] [PubMed]
- Tu M, Liu X, Han B, et al. Vasohibin-2 promotes proliferation in human breast cancer cells via upregulation of fibroblast growth factor-2 and growth/differentiation factor-15 expression. Mol Med Rep 2014;10:663-9. [Crossref] [PubMed]
- Kang X, Feng Y, Gan Z, et al. NASP antagonize chromatin accessibility through maintaining histone H3K9me1 in hepatocellular carcinoma. Biochim Biophys Acta Mol Basis Dis 2018;1864:3438-48. [Crossref] [PubMed]
- Huang XY, Liu JJ, Liu X, et al. Bioinformatics analysis of the prognosis and biological significance of VCAN in gastric cancer. Immun Inflamm Dis 2021;9:547-59. [Crossref] [PubMed]
- Li T, Gao X, Han L, et al. Identification of hub genes with prognostic values in gastric cancer by bioinformatics analysis. World J Surg Oncol 2018;16:114. [Crossref] [PubMed]
- Ji Y, Gao L, Zhang C, et al. Identification of the hub genes and prognostic indicators of gastric cancer and correlation of indicators with tumor-infiltrating immune cell levels. J Cancer 2021;12:4025-38. [Crossref] [PubMed]
- Tian C, Zhou S, Yi C. High NUP43 expression might independently predict poor overall survival in luminal A and in HER2+ breast cancer. Future Oncol 2018;14:1431-42. [Crossref] [PubMed]
- Mao M, Yu Q, Huang R, et al. Stromal score as a prognostic factor in primary gastric cancer and close association with tumor immune microenvironment. Cancer Med 2020;9:4980-90. [Crossref] [PubMed]
- Reeves E, James E. Antigen processing and immune regulation in the response to tumours. Immunology 2017;150:16-24. [Crossref] [PubMed]
- Dhatchinamoorthy K, Colbert JD, Rock KL. Cancer Immune Evasion Through Loss of MHC Class I Antigen Presentation. Front Immunol 2021;12:636568. [Crossref] [PubMed]
- Xin C, Zhu J, Gu S, et al. CD200 is overexpressed in neuroblastoma and regulates tumor immune microenvironment. Cancer Immunol Immunother 2020;69:2333-43. [Crossref] [PubMed]
- Herbrich S, Baran N, Cai T, et al. Overexpression of CD200 is a Stem Cell-Specific Mechanism of Immune Evasion in AML. J Immunother Cancer 2021;9:e002968. [Crossref] [PubMed]
- Xiong Z, Ampudia-Mesias E, Shaver R, et al. Tumor-derived vaccines containing CD200 inhibit immune activation: implications for immunotherapy. Immunotherapy 2016;8:1059-71. [Crossref] [PubMed]
- Klement JD, Paschall AV, Redd PS, et al. An osteopontin/CD44 immune checkpoint controls CD8+ T cell activation and tumor immune evasion. J Clin Invest 2018;128:5549-60. [Crossref] [PubMed]
- Hinterbrandner M, Rubino V, Stoll C, et al. Tnfrsf4-expressing regulatory T cells promote immune escape of chronic myeloid leukemia stem cells. JCI Insight 2021;6:151797. [Crossref] [PubMed]
- Hansen W, Hutzler M, Abel S, et al. Neuropilin 1 deficiency on CD4+Foxp3+ regulatory T cells impairs mouse melanoma growth. J Exp Med 2012;209:2001-16. [Crossref] [PubMed]
- Wang F, Wang G, Liu T, et al. B7-H3 was highly expressed in human primary hepatocellular carcinoma and promoted tumor progression. Cancer Invest 2014;32:262-71. [Crossref] [PubMed]
- Maeda N, Yoshimura K, Yamamoto S, et al. Expression of B7-H3, a potential factor of tumor immune evasion in combination with the number of regulatory T cells, affects against recurrence-free survival in breast cancer patients. Ann Surg Oncol 2014;21:S546-54. [Crossref] [PubMed]
- Lu H, Ma Y, Wang M, et al. B7-H3 confers resistance to Vγ9Vδ2 T cell-mediated cytotoxicity in human colon cancer cells via the STAT3/ULBP2 axis. Cancer Immunol Immunother 2021;70:1213-26. [Crossref] [PubMed]
- Wang C, Li Y, Jia L, et al. CD276 expression enables squamous cell carcinoma stem cells to evade immune surveillance. Cell Stem Cell 2021;28:1597-1613.e7. [Crossref] [PubMed]
- Soskic B, Qureshi OS, Hou T, et al. A transendocytosis perspective on the CD28/CTLA-4 pathway. Adv Immunol 2014;124:95-136. [Crossref] [PubMed]
- Janakiram M, Shah UA, Liu W, et al. The third group of the B7-CD28 immune checkpoint family: HHLA2, TMIGD2, B7x, and B7-H3. Immunol Rev 2017;276:26-39. [Crossref] [PubMed]