Construction of miRNA-mRNA network and a nomogram model of prognostic analysis for prostate cancer
Introduction
Prostate Cancer is a high incidence malignant tumor that afflicts men worldwide. In China, prostate cancer accounted for 8.16% of the total number of new cancer cases in males, with a mortality rate of 13.61% (1). In the United States, prostate cancer is the most common (comprising 26% of all cases) malignant tumor in males (2). The incidence of prostate cancer has continuously increased globally (3). Prostate cancer patients undergo biochemical relapse (BCR), which results in the poor prognosis (4,5). BCR is defined as two consecutive rising prostate-specific antigen (PSA) values >2 ng/mL above the nadir PSA value after radiation therapy (RT) or >0.2 ng/mL after radical prostatectomy (RP) (6).
Urologists have made great progress in the diagnosis and treatment of prostate cancer, especially with the discovery of new biomarkers for liquid biopsies and imaging techniques that can assist clinicians in making decisions. Prostate cancer is a group of heterogeneous tumors with individual differences, molecular markers play an important role in assisting diagnosis and predicting prognosis (7,8). Therefore, it is essential to find novel and effective molecular markers associated with the diagnosis and prognosis for PCa.
Non-coding microRNAs (miRNAs) are single-stranded small RNA molecules involved in post-transcriptional gene regulation (9). Accumulating evidence has revealed that miRNAs participate in regulating cellular biological and pathophysiological processes of cancer cells. In addition, miRNAs can be detected stably in biofluids to achieve non-invasive tumor diagnosis and efficacy evaluation, which have been applied to assist clinical diagnosis and prognosis. However, few studies on multi-dataset analysis of combined RRA of miRNA-mRNA in prostate cancer have been reported. This study aims to construct miRNA-mRNA regulatory networks of prostate cancer progression by using bioinformatics methods, to identify core miRNAs and hub target genes. Hub genes may be used to build-up a nomogram model for clinical prognosis. We present the following article in accordance with the TRIPOD reporting checklist (available at https://tcr.amegroups.com/article/view/10.21037/tcr-22-653/rc).
Methods
Data collection and preprocessing
The datasets were downloaded from the GEO database (https://www.ncbi.nlm.nih.gov/geo/). We selected 3 miRNA datasets (GSE60117, GSE21036 and GSE76260), 4 mRNA datasets (GSE79021, GSE62872, GSE70768 and GSE70769), and The Cancer Genome Atlas (TCGA) database (https://gdc-portal.nci.nih.gov/). The characteristics of these datasets were shown in Table 1. PCa patients were confirmed by pathological examination and included both metastatic and in situ cancers. In GSE70769 and TCGA datasets, the survival information of the patient who had undergone RP or RT was collected for the subsequent biochemical relapse-free survival (BCRFS) analysis. The study was conducted based on the Declaration of Helsinki (as revised in 2013).
Table 1
Dataset | Omics | Data type | GPL | Number of tissues | Number of genes | |
---|---|---|---|---|---|---|
Healthy | Cancer | |||||
GSE60117 | miRNA | Microarray | GPL13264 | 21 | 56 | 2,735 |
GSE21036 | miRNA | Microarray | GPL8227 | 28 | 99 | 881 |
GSE76260 | miRNA | Microarray | GPL8179 | 32 | 32 | 1,146 |
GSE79021 | mRNA | Microarray | GPL19370 | 49 | 153 | 20,280 |
GSE62872 | mRNA | Microarray | GPL19370 | 160 | 264 | 20,280 |
GSE70768 | mRNA | Microarray | GPL10558 | 74 | 112 | 48,107 |
GSE70769 | mRNA | Microarray | GPL10558 | NA | 94 | 48,107 |
TCGA | mRNA | RNA-Seq | NA | 52 | 492 | 55,268 |
GSE, Gene Expression Omnibus Series; GPL, Gene Expression Omnibus Platform; TCGA, The Cancer Genome Atlas; miRNA, microRNA.
Differential miRNA and mRNA screening
Differentially expressed miRNAs (DEMs) and differentially expressed genes (DEGs) were determined by comparing two groups between normal and cancerous tissues in each GEO dataset. Datasets were corrected using the ‘normalizeBetweenArrays’ package of R software (version 4.1.2). The ‘limma’ package of R software was used to screen DEGs in the dataset, and the screening criteria for DEMs and DEGs were adjusted P values <0.05. The correction methods were Benjamini and Hochberg (BH), to address false-positivity (10). The DEMs and DEGs in these datasets were analyzed using the R package ‘RobustRankAggreg’ (11). The RRA analysis was applied to calculate the significance probability of all genes in the final sequence, which might integrate the differential expression matrix of each dataset (11).
Construction of miRNA-mRNA regulatory networks
FunRich was a bioinformatics tool, which could predict miRNA target genes for functional enrichment (12). Common DEGs (co-DEGs) could be obtained by using FunRich (version 3.1.3) to predict miRNA target genes. Co-DEGs were used to construct miRNA-mRNA regulatory networks. Cytoscape (version 3.8.2) was used to visually display each network (13). Top 3 miRNAs with Edge Percolated Component (EPC) scores (connectivity greater than 30) were selected to build-up the core network of miRNAs using the EPC topology algorithm. Co-DEGs regulated by miRNAs in the core network were selected as core network genes.
Gene function enrichment analysis
The GO (Gene Ontology) function and KEGG (Kyoto Encyclopedia of Genes and Genomes) signal pathway analysis of co-DEGs were performed using the R software package “clusterProfiler” (14). GO enrichment included biological process (BP), cellular component (CC) and molecular function (MF). For KEGG functional enrichment-related signalling pathways, the screening criteria were adjusted P<0.05 to control false discovery rate (FDR).
Differential expression and prognostic value of hub regulatory genes
TCGA and Genotype-Tissue Expression (GTEx) databases contain clinical samples and demographic/clinical information. Therefore, they can be used to verify statistical differences in the expression levels of core network genes between cancer and normal tissues in a large cohort. Hub target genes were analyzed using GEPIA2 web tools (http://gepia2.cancer-pku.cn/).
Establishment of nomogram
The risk score of patients was calculated by ridge regression analysis. The TCGA and GSE70769 cohort was treated as training and validation set, respectively. Multivariate and univariate COX regression analyses were performed for each candidate. A nomogram prediction model was established for independent prognostic factors using R package ‘rms’ (https://CRAN.R-project.org/package=rms). 100 points were allocated to the most dangerous predictors with the highest B coefficient, and corresponding scores were assigned to other predictors according to relative weight. The accuracy of prediction was evaluated using calibration diagram and AUC.
Statistical analysis
Statistical analysis was performed using R software (version 4.1.2). Differences between groups were compared with Wilcoxon tests. The BCRFS curves were generated by using Kaplan-Meier (K-M) plots, while the differences were evaluated by log-rank tests. Univariate and multivariate Cox proportional hazard models were applied to estimate potentially predictive values of parameters. Time-ROC curves were plotted and utilized to assess potential prognostic power of risk scores. Calibration plots and AUCs were applied to evaluate the prediction accuracy of a nomogram. A P<0.05 was considered statistically significant.
Results
Screening of differential miRNAs and mRNAs associated with prostate cancer
Three miRNA datasets were analyzed with the RRA method. A total of 22 DEMs were up-regulated whereas 14 down-regulated (Figure 1A). A total of 896 DEGs were up-regulated whereas 915 down-regulated in prostate cancer. As shown in Figure 1B, the top 20 up-regulated and down-regulated genes were sorted by Log2 Fold Change.
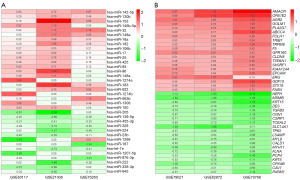
Construction of the miRNA-mRNA network
Among the 36 DEMs screened from miRNA datasets, 17 miRNAs could be predicted by FunRich software, and a total of 2,575 target genes could be predicted. Among them, 184 genes were selected based on intersection of co-DEGs and target genes. Then, miRNA-mRNA reaction network was constructed according to regulatory relationship using Cytoscape3.8.2 (Figure 2A). In addition, three core network miRNAs were obtained by EPC topology algorithm screening, which were highly expressed in prostate cancer tissues, including hsa-miR-106b-5p, hsa-miR-17-5p and hsa-miR-183-5p. The key gene network comprised of 32 core genes as shown in Cytoscape (Figure 2B), while the key network module information was summarized in Table 2.
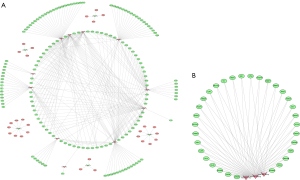
Table 2
Gene | Category | Change trend | Degree value | EPC score |
---|---|---|---|---|
hsa-miR-17-5p | miRNA | Up | 35 | 42.675 |
hsa-miR-106b-5p | miRNA | Up | 34 | 42.662 |
hsa-miR-183-5p | miRNA | Up | 44 | 41.37 |
NTN4 | mRNA | Down | 6 | 34.588 |
SIK1 | mRNA | Down | 5 | 31.116 |
HECTD2 | mRNA | Down | 4 | 30.801 |
EPHA7 | mRNA | Down | 4 | 29.436 |
FRMD6 | mRNA | Down | 4 | 28.987 |
RND3 | mRNA | Down | 4 | 28.796 |
CFL2 | mRNA | Down | 4 | 27.316 |
RNF38 | mRNA | Down | 4 | 26.19 |
RBBP7 | mRNA | Down | 3 | 25.813 |
TGFBR2 | mRNA | Down | 3 | 25.73 |
TMEM100 | mRNA | Down | 3 | 25.676 |
ANO6 | mRNA | Down | 3 | 25.587 |
DPYSL2 | mRNA | Down | 3 | 25.573 |
STARD4 | mRNA | Down | 3 | 25.292 |
RBMS1 | mRNA | Down | 3 | 25.215 |
HLF | mRNA | Down | 3 | 25.042 |
MMP2 | mRNA | Down | 3 | 25.019 |
GJA1 | mRNA | Down | 3 | 25.018 |
CALD1 | mRNA | Down | 3 | 24.922 |
TGFB1I1 | mRNA | Down | 3 | 24.912 |
SYTL4 | mRNA | Down | 3 | 24.902 |
TSHZ3 | mRNA | Down | 3 | 24.844 |
FOXF1 | mRNA | Down | 3 | 24.817 |
CLIP4 | mRNA | Down | 3 | 24.696 |
CYBRD1 | mRNA | Down | 3 | 24.69 |
CNN1 | mRNA | Down | 3 | 24.508 |
AHNAK | mRNA | Down | 3 | 24.488 |
TIMP2 | mRNA | Down | 3 | 24.318 |
NBL1 | mRNA | Down | 3 | 24.293 |
APCDD1 | mRNA | Down | 3 | 23.427 |
SMOC1 | mRNA | Down | 3 | 23.331 |
PDGFRA | mRNA | Down | 2 | 18.842 |
EPC, Edge Percolated Component; miRNA, microRNA.
GO and KEGG enrichment analysis
GO analysis of genes included biological process (BP), molecular function (MF) and cellular component (CC) (Figure 3A). The biological processes of co-DEGs mainly enriched in actin filaments regulation, mesenchymal development and muscular system. In terms of cellular components, “focal adhesions”, “cell-substrate adherens junction”, and “cell-substrate junctions” were the most dominant elements. For molecular functions, the GO terms enriched with co-DEGs included actin binding, platelet-derived growth factor receptor binding, and activation of 3',5' cyclic-AMP phosphodiesterase. In addition, KEGG analysis indicated involvement of focal adhesion, cGMP-PKG signaling pathway and actin skeleton regulatory pathway in the pathogenesis of prostate cancer (Figure 3B). Afterwards, co-DEGs were mapped to 18 KEGG pathways, as shown in Figure 3C.
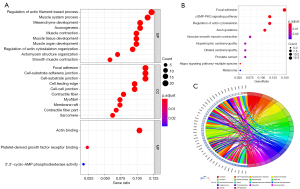
Validation of hub target genes
We performed gene expression values analyses of the four hub target genes (TMEM100, FRMD6, NBL1 and STARD4) by GEPIA2. These gene expression levels in prostate cancer were lower than those in normal control (P<0.05), as shown in Figure 4.
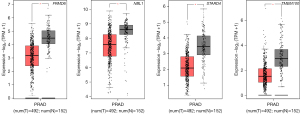
Establishment and verification of the nomogram
A risk score was calculated by ridge regression. The formula was as follows: risk score = (−0.018372 × STARD4) + (−0.017621 × FRMD6) + (−0.000546 × NBL1) + (−0.000064 × TMEM100). According to univariate and multivariate COX regression analyses, Gleason and risk scores might be used as independent prognostic factors (Figure 5A). Nomogram can predict BCRFS for PCa patients (Figure 5B). The calibration plot demonstrated good consistency between the prediction by nomogram and actual observation of 1-, 3- and 5-year BCRFS in prostate cancer (Figure 5C). The novel nomogram predicted the AUC of BCRFS at 1-, 3- and 5-year of 0.713, 0.732 and 0.753 (Figure 5D). The BCRFS time of high-risk group was significantly lower than that of the low-risk group (Figure 5E).
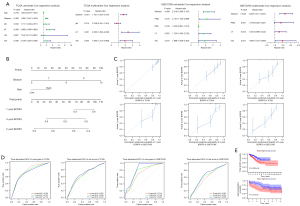
Discussion
Previous studies have indicated that aberrant expression of miRNAs may dysregulate expression of tumour-related genes, and thus affect tumour development and progression (9,15-17). Although many genes have been proposed as miRNA regulatory targets in PCa, their functions remain unknown. The pathogenesis of prostate cancer involves various signalling pathways regulated by miRNAs-mRNAs. Several miRNAs have been well studied in prostate cancer. For example, circRNA-HIPK3 may upregulate CDC25B and CDC2, promote G2/M transformation and induce cell proliferation of prostate cancer by inhibiting miR-338-3p (18). In addition, overexpression of miR-338-3p may downregulate BCL2 in LNCaP cells, and suppress apoptosis of prostate cancer cells (17). By contrast, MiR-338-3p directly targets the RAB23 gene and inhibits tumour growth in prostate cancer (19). Thus, it is critical to explore inter-regulatory relationship of miRNAs-mRNAs in tumour development and progression of prostate cancer.
In this study, hsa-miR-106b-5p, hsa-miR-17-5p and hsa-miR-183-5p were identified to construct a miRNA-mRNA regulatory network of prostate cancer. Hsa-miR-106b-5p, as an oncogene, may down-regulate RBMS1 expression in prostate cancer tissues and affect cell proliferation, gap closure and colony formation (20). Meanwhile, hsa-miR-106b in the blood of prostate cancer patients is significantly higher than that of healthy controls (21). Moreover, lncRNA-FER1L4 competitively binds a specific miRNA through its miRNA response element and represses the inhibitory effects of hsa-miR-106b-5p on its target mRNAs. This process participates in the post-transcriptional regulation of cancer-related genes (22). In addition, high expression of hsa-miR-17-5p in the epithelium was a predictive marker for poor prognosis in patients with PCa (23). Furthermore, circRNA-ITCH inhibited PCa progression by sponging hsa-miR-17-5p and increasing HOXB13 expression (24). In addition, high expression of hsa-miR-183-5p in local tissues of advanced prostate cancer may be related to lymph node metastasis and diffusion (25). Collectively, the three core miRNAs in this study were abnormally highly expressed in prostate cancer tissues, which was consistent with previous studies (16,21,23).
Four hub mRNAs were also identified associated with prostate cancer in this study, including TMEM100, FRMD6, NBL1 and STARD4. Previous studies have investigated the relationship between these hub genes and prostate cancer. For example, FRMD6 was abnormally hypermethylated and significantly down-regulated in prostate cancer tissues. Relatively low expression of FRMD6 was associated with postoperative biochemical recurrence. FRMD6 is proposed as a tumour-suppressive gene in prostate cancer (26). In addition, NBL1, a secreted protein, was highly expressed in the normal prostate. Low expression of NBL1 was associated with prostate cancer progression as a candidate biomarker (27). Notably, NBL1 expression in prostate cancer gland was down-regulated compared with normal gland, which might act as a tumour suppressor gene and a new therapeutic target (28). In this study, FRMD6 and NBL1 were significantly downregulated in prostate cancer tissues, and associated with disease-free survival (DFS) in prostate cancer patients. Its regulatory effects on TMEM100 and STARD4 in PCa necessitate further investigations.
Through GO functional enrichment analysis, co-DEGs were significantly enriched in actin filament regulation process, adhesion spots and actin-binding function. KEGG pathway analysis identified co-DEGs enriched signalling pathways, including focal adhesion, cGMP-PKG, actin skeleton regulation, prostate cancer, and PI3K-Akt and cAMP. These cellular functions and signalling pathways were related to tumour development and progression of prostate cancer, which were consistent with previous studies (15,29,30). Meanwhile, inflammatory signalling pathways (PI3K-Akt and cAMP) indicate the importance of inflammation in tumour progression of localized prostate cancer. Inflammation markers of prostate cancer will help to discover accurate biomarkers and effective therapeutic targets (31). More importantly, in the validation set with expanded sample size by using the exogenous database “GEPIA2”, the hub target genes were significantly differentially expressed in prostate cancer versus normal prostate tissue. These genes were strongly associated with either overall survival (OS) or DFS, with good value for clinical diagnosis and prediction.
In this study, Gleason is an independent prognostic factor for biochemical recurrence in prostate cancer patients, which is consistent with previous studies (5,32). This novel nomogram based on two independent risk factors may be accurate for predicting 1-, 3- and 5-year BCRFS in patients with PCa, and its AUC is superior to that under the risk score alone or combined Gleason model.
This study has several limitations. Firstly, this study was a retrospective study using public databases. Secondly, the conclusion from this study needs to be validated with a large number of clinical samples.
In conclusion, our study suggests that this novel nomogram may be used as a biomarker for predicting biochemical recurrence and prognosis in patients with prostate cancer, with better sensitivity and specificity than existing clinical indicator models. Multi-center, prospective studies with large-sample sizes can be carried out in the future to verify our conclusion and clinical predictive value of this model.
Acknowledgments
Funding: This study was supported by the National Key Research and Development Program of China (No. 2020YFC2005404); the Scientific research and Cultivation program of Haidian District health development (No. HP2022-19-506005); the Youth Fund of Beijing Shijitan Hospital (No. 2020-q06).
Footnote
Reporting Checklist: The authors have completed the TRIPOD reporting checklist. Available at https://tcr.amegroups.com/article/view/10.21037/tcr-22-653/rc
Conflicts of Interest: All authors have completed the ICMJE uniform disclosure form (available at https://tcr.amegroups.com/article/view/10.21037/tcr-22-653/coif). The authors have no conflicts of interest to declare.
Ethical Statement: The authors are accountable for all aspects of the work in ensuring that questions related to the accuracy or integrity of any part of the work are appropriately investigated and resolved. The study was conducted based on the Declaration of Helsinki (as revised in 2013).
Open Access Statement: This is an Open Access article distributed in accordance with the Creative Commons Attribution-NonCommercial-NoDerivs 4.0 International License (CC BY-NC-ND 4.0), which permits the non-commercial replication and distribution of the article with the strict proviso that no changes or edits are made and the original work is properly cited (including links to both the formal publication through the relevant DOI and the license). See: https://creativecommons.org/licenses/by-nc-nd/4.0/.
References
- Cao W, Chen HD, Yu YW, et al. Changing profiles of cancer burden worldwide and in China: a secondary analysis of the global cancer statistics 2020. Chin Med J (Engl) 2021;134:783-91. [Crossref] [PubMed]
- Sung H, Ferlay J, Siegel RL, et al. Global Cancer Statistics 2020: GLOBOCAN Estimates of Incidence and Mortality Worldwide for 36 Cancers in 185 Countries. CA Cancer J Clin 2021;71:209-49. [Crossref] [PubMed]
- Kimura T, Egawa S. Epidemiology of prostate cancer in Asian countries. Int J Urol 2018;25:524-31. [Crossref] [PubMed]
- Jiang LN, Liu YB, Li BH. Lycopene exerts anti-inflammatory effect to inhibit prostate cancer progression. Asian J Androl 2018;21:80-5. [PubMed]
- Su Q, Liu Z, Chen C, et al. Gene signatures predict biochemical recurrence-free survival in primary prostate cancer patients after radical therapy. Cancer Med 2021;10:6492-502. [Crossref] [PubMed]
- Cornford P, Bellmunt J, Bolla M, et al. EAU-ESTRO-SIOG Guidelines on Prostate Cancer. Part II: Treatment of Relapsing, Metastatic, and Castration-Resistant Prostate Cancer. Eur Urol 2017;71:630-42. [Crossref] [PubMed]
- Nicolosi P, Ledet E, Yang S, et al. Prevalence of Germline Variants in Prostate Cancer and Implications for Current Genetic Testing Guidelines. JAMA Oncol 2019;5:523-8. [Crossref] [PubMed]
- Su Q, Lei T, Zhang M. Association of ferritin with prostate cancer. J BUON 2017;22:766-70. [PubMed]
- Jackson BL, Grabowska A, Ratan HL. MicroRNA in prostate cancer: functional importance and potential as circulating biomarkers. BMC Cancer 2014;14:930. [Crossref] [PubMed]
- Ritchie ME, Phipson B, Wu D, et al. limma powers differential expression analyses for RNA-sequencing and microarray studies. Nucleic Acids Res 2015;43:e47. [Crossref] [PubMed]
- Kolde R, Laur S, Adler P, et al. Robust rank aggregation for gene list integration and meta-analysis. Bioinformatics 2012;28:573-80. [Crossref] [PubMed]
- Fonseka P, Pathan M, Chitti SV, et al. FunRich enables enrichment analysis of OMICs datasets. J Mol Biol 2021;433:166747. [Crossref] [PubMed]
- Shannon P, Markiel A, Ozier O, et al. Cytoscape: a software environment for integrated models of biomolecular interaction networks. Genome Res 2003;13:2498-504. [Crossref] [PubMed]
- Yu G, Wang LG, Han Y, et al. clusterProfiler: an R package for comparing biological themes among gene clusters. OMICS 2012;16:284-7. [Crossref] [PubMed]
- Hudson RS, Yi M, Esposito D, et al. MicroRNA-106b-25 cluster expression is associated with early disease recurrence and targets caspase-7 and focal adhesion in human prostate cancer. Oncogene 2013;32:4139-47. [Crossref] [PubMed]
- Schitcu VH, Raduly L, Nutu A, et al. MicroRNA Dysregulation in Prostate Cancer. Pharmgenomics Pers Med 2022;15:177-93. [Crossref] [PubMed]
- Zhang X, Pan Y, Fu H, et al. microRNA-205 and microRNA-338-3p Reduces Cell Apoptosis in Prostate Carcinoma Tissue and LNCaP Prostate Carcinoma Cells by Directly Targeting the B-Cell Lymphoma 2 (Bcl-2) Gene. Med Sci Monit 2019;25:1122-32. [Crossref] [PubMed]
- Liu F, Fan Y, Ou L, et al. CircHIPK3 Facilitates the G2/M Transition in Prostate Cancer Cells by Sponging miR-338-3p. Onco Targets Ther 2020;13:4545-58. [Crossref] [PubMed]
- Wang Y, Qin H. miR-338-3p targets RAB23 and suppresses tumorigenicity of prostate cancer cells. Am J Cancer Res 2018;8:2564-74. [PubMed]
- Dankert JT, Wiesehöfer M, Wach S, et al. Loss of RBMS1 as a regulatory target of miR-106b influences cell growth, gap closing and colony forming in prostate carcinoma. Sci Rep 2020;10:18022. [Crossref] [PubMed]
- Porzycki P, Ciszkowicz E, Semik M, et al. Combination of three miRNA (miR-141, miR-21, and miR-375) as potential diagnostic tool for prostate cancer recognition. Int Urol Nephrol 2018;50:1619-26. [Crossref] [PubMed]
- Xia T, Liao Q, Jiang X, et al. Long noncoding RNA associated-competing endogenous RNAs in gastric cancer. Sci Rep 2014;4:6088. [Crossref] [PubMed]
- Stoen MJ, Andersen S, Rakaee M, et al. High expression of miR-17-5p in tumor epithelium is a predictor for poor prognosis for prostate cancer patients. Sci Rep 2021;11:13864. [Crossref] [PubMed]
- Wang X, Wang R, Wu Z, et al. Circular RNA ITCH suppressed prostate cancer progression by increasing HOXB13 expression via spongy miR-17-5p. Cancer Cell Int 2019;19:328. [Crossref] [PubMed]
- Pudova EA, Krasnov GS, Nyushko KM, et al. miRNAs expression signature potentially associated with lymphatic dissemination in locally advanced prostate cancer. BMC Med Genomics 2020;13:129. [Crossref] [PubMed]
- Haldrup J, Strand SH, Cieza-Borrella C, et al. FRMD6 has tumor suppressor functions in prostate cancer. Oncogene 2021;40:763-76. [Crossref] [PubMed]
- Hayashi T, Sentani K, Oue N, et al. The search for secreted proteins in prostate cancer by the Escherichia coli ampicillin secretion trap: expression of NBL1 is highly restricted to the prostate and is related to cancer progression. Pathobiology 2013;80:60-9. [Crossref] [PubMed]
- Adler D, Lindstrot A, Buettner R, et al. Analysis of laser-microdissected prostate cancer tissues reveals potential tumor markers. Int J Mol Med 2011;28:605-11. [PubMed]
- Wang Z, Zhang C, Chang J, et al. LncRNA EMX2OS, Regulated by TCF12, Interacts with FUS to Regulate the Proliferation, Migration and Invasion of Prostate Cancer Cells Through the cGMP-PKG Signaling Pathway. Onco Targets Ther 2020;13:7045-56. [Crossref] [PubMed]
- Zacharopoulou N, Tsapara A, Kallergi G, et al. The epigenetic factor KDM2B regulates cell adhesion, small rho GTPases, actin cytoskeleton and migration in prostate cancer cells. Biochim Biophys Acta Mol Cell Res 2018;1865:587-97. [Crossref] [PubMed]
- Archer M, Dogra N, Kyprianou N. Inflammation as a Driver of Prostate Cancer Metastasis and Therapeutic Resistance. Cancers (Basel) 2020;12:2984. [Crossref] [PubMed]
- Nørgaard M, Haldrup C, Storebjerg TM, et al. Comprehensive Evaluation of TFF3 Promoter Hypomethylation and Molecular Biomarker Potential for Prostate Cancer Diagnosis and Prognosis. Int J Mol Sci 2017;18:2017. [Crossref] [PubMed]