A comprehensive pan-cancer analysis on the immunological role and prognostic value of TYMP in human cancers
IntroductionOther Section
Cancer is major cause of death worldwide, the incidence and mortality rates of which are rapidly rising globally. According to Global Cancer Statistics (GLOBOCAN 2020), in 2020 there were 19.3 million new cancer diagnoses and about 10 million cancer deaths (1). Most cancer deaths are due to metastasis and no effective treatment currently exists for cancer (2). Fortunately, in recent years, cancer immunotherapy including immune checkpoint inhibitors has become an increasingly successful strategy (3,4). Therefore, new therapeutic targets and sensitive tumor biomarkers are required for the diagnosis and treatment of cancer.
The TYMP gene encoding thymidine phosphorylase (TP) is located on chromosome 22q13.33 (5). TP is an enzyme involved in nucleoside metabolism in the salvage pathway of pyrimidine nucleosides. It is also known as platelet-derived endothelial cell growth factor (PD-ECGF), which plays an important role in cell proliferation and angiogenesis (6). Mutations in TYMP have been found to cause mitochondrial neurogastrointestinal encephalopathy (MNGIE) (7). Also, much evidence has suggested that TYMP is expressed in several human tumors, such as in lung cancer (8), head and neck cancer (HNSC) (9), esophageal squamous cell carcinoma (10), gastric carcinoma (11), and colorectal cancer (12). Moreover, TYMP is also suggested to play multiple roles in tumor progression and response to therapy. On the one hand, TYMP promotes cell proliferation, enhances the epithelial to mesenchymal transition, as well as angiogenesis, activities which all accelerate tumor invasiveness and progression (13). On the other hand, because the TYMP gene encodes TP which is an important rate-limiting enzyme in thymidine catabolism involved in the 5-fluorouracil (5-FU) activation pathway, quantification of TYMP expression will be extremely useful in predicting the effects of chemotherapeutic drugs, such as 5-FU and capecitabine (14). In addition, TYMP is a target of anti-cancer drugs to inhibit its expression and promote the suppression of angiogenesis and enhance apoptosis.
Several studies have reported that increased TYMP expression in different cancers is linked to poor outcomes (11,15,16). Furthermore, tumor cells and stromal cells express TYMP in the tumor microenvironment (TME) (13) and a previous study suggested that TYMP may be a target for immunotherapy in solid tumors (17). Taken together, available evidence suggests that TYMP influences cancer prognosis, and may potentially represent a useful prognostic biomarker.
Nevertheless, the significance of TYMP for tumor immunity and prognosis in many diverse malignancies remains unclear, and a comprehensive pan-cancer study on the relationship between TYMP expression and cancer biology has not yet been conducted. Therefore, we performed such a pan-cancer analysis to better understand the role of TYMP in cancer. In our investigation, we examined the TYMP expression profiles in tumors and cancer cell lines by leveraging the The Cancer Genome Atlas (TCGA), Oncomine, Gene Expression Profiling Interactive Analysis (GEPIA), and Cancer Cell Line Encyclopedia (CCLE) databases. Additionally, the expression of TYMP and its connection with cancer prognosis was investigated using PrognoScan and Kaplan-Meier Plotter. We also performed a genetic mutation analysis, and microsatellite instability (MSI), tumor mutational burden (TMB), and immune scores were calculated to study their relationships with TYMP expression in tumors. Subsequently, co-expression analyses of ICGs and TYMP, drug sensitivity analysis, and gene set enrichment analyses (GSEAs) were carried out. Our findings suggest that TYMP may be a predictive factor in a variety of malignancies and a critical in tumor immunity, but further laboratory evidence is needed in future. We present the following article in accordance with the REMARK reporting checklist (available at https://tcr.amegroups.com/article/view/10.21037/tcr-22-502/rc).
MethodsOther Section
Data acquisition and TYMP expression analysis
Gene expression data was downloaded using the UCSC Xena platform (https://xena.ucsc.edu) including RNA sequencing, somatic mutation, and related clinical data for 33 cancer types from TCGA. TYMP gene expression data were obtained from datasets and converted into a gene expression matrix for analysis using a Perl script written in Strawberry Perl for Windows (version 5.32.1; available at https://strawberryperl.com/). The Oncomine (https://www.oncomine.org/) database was searched for differences in TYMP expression between around 20 cancer types and paired normal tissue. TYMP expression was compared between human malignant tumors and associated normal tissue using the GEPIA database (http://gepia.cancer-pku.cn/). Further, we accessed TYMP expression levels in 33 different cancer cell lines, through the CCLE database (https://sites.broadinstitute.org/ccle). TYMP expression data from TCGA were compared in normal tissue and tumor tissue among 33 cancer entities by Wilcoxon testing, and a P value of <0.05 was regarded as significant. R software v4.05 (https://www.r-project.org/) was used to perform the statistical analysis, and box plots was drawn with the “ggpubr” package of R software. The study was conducted in accordance with the Declaration of Helsinki (as revised in 2013).
Analysis of correlations between TYMP expression and prognosis of tumor patients
Survival data of patients using 10,121 tumor samples from 33 tumors were downloaded from TCGA. The relationships between TYMP expression and patient prognosis, including overall survival (OS), disease-specific survival (DSS), disease-free interval (DFI), and progression-free interval (PFI) were investigated. Cox proportional hazard regression analysis and the Kaplan-Meier method were used for survival analysis, presented as forest plots and Kaplan-Meier survival curves, using the R packages “survival” and “forestplot”, survival” and “survminer” packages, respectively.
Genetic alteration analysis
Genetic alterations of TYMP were analyzed in cancers via cBioPortal (https://www.cbioportal.org/) using data from TCGA (18). Applying cBioPortal, we investigated the frequency, mutation type, and copy number alteration (CNA) of TYMP in all TCGA cancers. These results can be obtained from the ‘Cancer Types Summary’ tab. We explored the TYMP mutation sites in pan-cancer and also compared clinical outcomes in TCGA cancer samples with or without TYMP gene mutations.
Associations analysis of TYMP expression with tumor stage, TMB and MSI
Clinical information on pathological tumor stage of all the tumor samples derived from TCGA was downloaded and associations of TYMP with tumor stage analyze using the R-packages “limma” and “ggpubr”. The total amount of mutations in tumor cells is referred to as TMB, and is used to predict responsiveness to immune checkpoint inhibitor therapy (19). MSI in tumor cells is related to defects in the DNA mismatch repair system (20). We downloaded mutation data from TCGA of 33 tumors from 10,114 samples and then calculated TMB scores for each sample. TCGA provided data on somatic mutations of tumor samples and the calculated MSI scores. Finally, the link between TYMP expression and TMB, as well as MSI, was investigated using Spearman’s rank correlation testing. TMB and MSI correlation radar maps were created using the R-package “fmsb”.
Correlations between TYMP expression and immunity
In all tumor samples, immune scores and stromal scores were estimated by the ESTIMATE (estimation of stromal and immune cells in malignant tumor tissues using expression) algorithm method. This can assist in determining the level of stromal and immune cell infiltration (21). To assess the association between TYMP expression and these two scores, we further applied the R packages “estimate” and “limma”. Moreover, we used CIBERSORT, a computational approach, to study tumor cell infiltration abundance among 33 human malignancies (22). We also used “ggplot2”, “ggpubr”, and “ggExtra” to examine associations between TYMP expression and the degree of infiltration of immune cells into each cancer. Finally, we generated a heatmap of connections between TYMP expression and 47 ICGs across 33 human cancers using the R-package “limma”, “reshape2” and “RColorBrewer”.
Drug sensitivity analysis
The CellMiner database (https://discover.nci.nih.gov/cellminer/) contains molecular and pharmacological datasets for the 60 different National Cancer Institute (NCI) human tumor cell lines from nine cancer types (NCI-60 cell lines) (23). Relationships between TYMP mRNA expression and drug sensitivity were also investigated using Pearson correlations. Data processing and correlation analysis visualization were conducted through the “impute”, “limma”, “ggplot2”, and “ggpubr” packages, with significance set to P<0.05.
GSEA based on TYMP expression in tumors
GSEA (https://www.gsea-msigdb.org/gsea/index.jsp) of TYMP was performed to gain insight into tumor biology. Enrichment analyses were carried out via the “limma”, “org.Hs.eg.db”, “clusterProfiler” and “enrichplot” packages.
Statistical analysis
TYMP expression was log2-transformed and TYMP expression was analyzed using the “limma” package and the Student’s t-test. Survival analyses adopted the Kaplan-Meier method, log-rank testing, and Cox proportional hazard regression analysis. Correlations between two variables were determined using Pearson’s or Spearman’s correlation test. R software (version 4.0.5) was used to conduct statistical analysis and data visualizations. P<0.05 is regarded as statistically significant.
ResultsOther Section
Pan-cancer analysis of expression profiles of TYMP from different databases
To determine TYMP expression in different cancer types, TYMP levels in tumor and normal samples from the Oncomine database were analyzed. This revealed that TYMP expression was significantly increased in bladder cancer (BLCA), brain and central nervous system (CNS) cancer, breast cancer (BRCA), cervical cancer (CESC), gastric cancer, HNSC, kidney cancer, leukemia, lung cancer, lymphoma, ovarian cancer (OV), and other cancer types (Figure 1A). We next analyzed TYMP expression levels in all 33 cancers in the TCGA database. TYMP expression was detectable in different cancers and matched normal tissues (Figure 1B). Compared with matched non-tumor tissue, differential TYMP expression was present in 16 of the 33 cancer types. TYMP expression was higher in cancer tissues versus normal tissues in BLCA, BRCA, CESC, bile duct cancer (CHOL), esophageal cancer (ESCA), glioblastoma (GBM), HNSC, kidney clear cell carcinoma (KIRC), kidney papillary cell carcinoma (KIRP), liver cancer (LIHC), lung adenocarcinoma (LUAD), lung squamous cell carcinoma (LUSC), rectal cancer (READ), stomach adenocarcinoma (STAD), and endometrioid cancer (UCEC). The expression of TYMP in CESC showed the greatest difference between tumor tissues and non-tumor tissues. In contrast to most tumors, lower levels of TYMP were also observed in tumor tissues versus normal tissues in pancreatic cancer (PAAD). Finally, no significant differences in TYMP levels were discernible between colon cancer (COAD), prostate adenocarcinoma (PRAD), pheochromocytoma and paraganglioma (PCPG), and thyroid cancer (THCA). Furthermore, TYMP expression profiles were analyzed using the GEPIA database, confirming that the majority of tumor types exhibited significant upregulation of TYMP expression, except adrenocortical cancer (ACC), kidney chromophobe (KICH), and THCA (Figure 1C). Figure 1D displays gene expression of TYMP across human cell lines and ranks them from low to high based on CCLE data. The results of differential expression analysis across cancer types further confirmed that TYMP expression was generally upregulated in cancer, suggesting that it may play an important role in carcinogenesis, and warranting more detailed analyses in further.
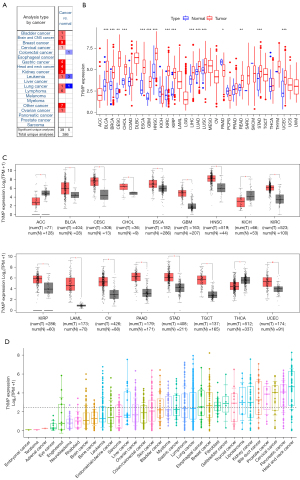
The prognostic signature of TYMP in human cancers
We investigated the relationships of TYMP expression and survival in terms of OS, DSS, DFI, and PFI in each cancer type using the datasets from TCGA. Initially, we employed Cox proportional hazards modeling to evaluate associations between TYMP expression and patient prognosis (OS, DSS, DFI, and PFI) across different tumors. Notably, analysis of OS showed that TYMP could classified as a high-risk gene in ACC, GBM, KIRC, lower grade glioma (LGG), PAAD, thymoma (THYM), and uveal melanomas (UVM). However, it could be classified as a low-risk gene in patients with BRCA and skin cutaneous melanoma (SKCM), as shown in Figure 2A. From the forest plot in Figure 2B, in the DSS survival analysis, elevated TYMP expression was correlated with poor clinical outcome in patients with COAD (P=0.033), GBM (P=0.027), KIRC (P=0.001), LGG (P<0.001), PAAD (P=0.042), and UVM (P<0.001). In contrast, gene expression of TYMP was positively correlated with patient prognosis in BRCA (P=0.010) and SKCM (P<0.001). Furthermore, an association between TYMP expression and DFI was only seen in patients with PRAD (P=0.016; hazard ratio =1.610) as shown in Figure 2C. Regarding associations between TYMP expression and PFI, Cox regression analysis indicated that high levels of TYMP were related to poor PFI in GBM (P=0.005), KIRC (P=0.031), LGG (P<0.001), PAAD (P=0.036), PRAD (P=0.002), THYM (P=0.025), and UVM (P=0.009) (Figure 2D).
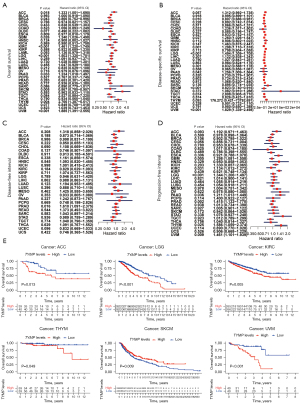
Next, we employed Kaplan-Meier survival analysis to investigate associations between TYMP expression and cancer patient prognosis. Kaplan-Meier survival estimates showed that high TYMP expression was associated with worse OS than low levels of TYMP in 5 malignancies, namely, ACC (P=0.013), LGG (P<0.001), KIRC (P=0.005), THYM (P=0.049), and UVM (P<0.001), while SKCM patients with high levels of TYMP survived longer (P=0.009) (Figure 2E). Low TYMP expression correlated with poor DSS in patients with BRCA (P=0.009) and SKCM (P<0.001). However, for patients with KIRC (P=0.020), LGG (P<0.001), ACC (P=0.014), THYM (P=0.021), and UVM (P<0.001), increased TYMP expression was significantly related to shorter DSS (Figure 3). In addition, estimates indicated a negative association between TYMP expression and DFI in LUAD (P=0.045) and PRAD (P=0.003) (Figure 4A,4B). An analysis of TYMP and PFI showed that in patients with ACC (P=0.032), GBM (P=0.022), LGG (P<0.001), KIRC (P=0.020), PRAD (P=0.017) and UVM (P=0.005), high TYMP expression had poor PFI (Figure 4C-4H). Collectively, these results imply that upregulation of the TYMP gene in multiple but not all cancers predicts a poor prognosis.
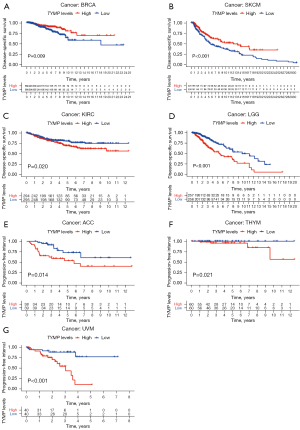
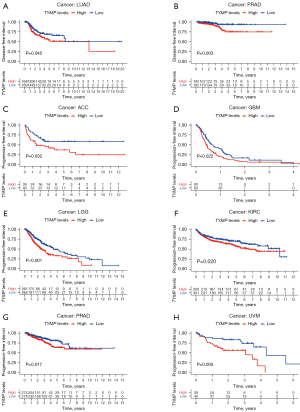
TYMP mutations in tumors
Mutations of the TYMP gene in different cancers were investigated further in the TCGA. Ovarian serous cystadenocarcinoma had the highest frequency of TYMP mutations (>10%), which was linked to “deep deletion” (Figure 5A). Not only in ovarian serous cystadenocarcinoma, but also in diffuse large B-cell lymphoma, UCEC, stomach adenocarcinoma, bladder urothelial carcinoma, LUAD, sarcoma (SARC), LUSC, and others, the most common type of CNA was “deep deletion”. Figure 5B depicts the types, sites, and numbers of cases with TYMP genetic alterations. However, TYMP mutations had no significant influence on OS and DSS, but there were some statistically significant differences of DFS and PFS between patients with tumors harboring TYMP gene mutants versus wild-type TYMP (Figure 5C).
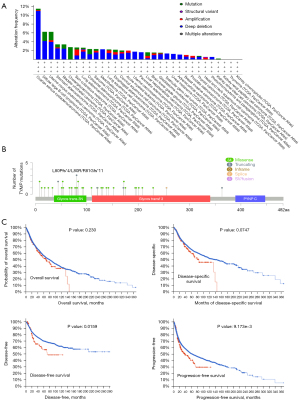
Associations between TYMP expression levels and clinicopathology, TMB, and MSI in pan-cancer
We subsequently examined TYMP expression among tumor at different stages. TYMP expression was linked to clinical tumor stage in 12 different cancers, as shown in Figure 6A. We also found that changes in TYMP expression were not consistent across tumor stages in different tumors. Notably, TYMP expression increased from early stages (stage I/II) to advanced stages (stage III/IV) in ACC, BLCA, KIRC, and STAD, as shown in Figure 6A (P<0.05). For instance, TYMP expression levels in ACC at stage IV were higher than at stage II. Conversely, TYMP levels decreased from early stages (stage I/II) to advanced stages (stage III/IV) in KIRP, LUAD, and testicular cancer (TGCT) (P<0.05). For example, TYMP expression was generally higher in stage II LUAD than in stage III LUAD. We also probed any possible links between TYMP expression and TMB and MSI in these tumor samples. TYMP expression was significantly correlated with TMB in 15 tumors such as BRCA, COAD, ESCA, and KIRC (Figure 6B) and was also closely linked with MSI in 10 tumors, such as THCA, TGCT, READ, and PAAD (Figure 6C).
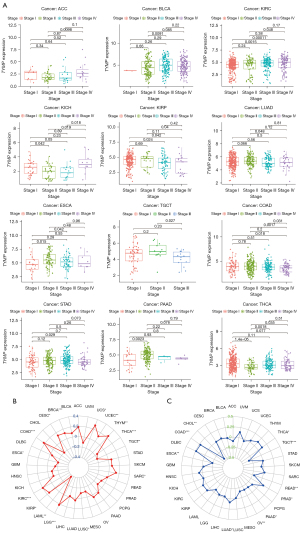
Associations between TYMP expression and the TME
The TME is composed of tumor cells, immune cells and stromal cells. Its constitution affects therapeutic responses and clinical outcomes (24). Hence, in the present study, we analyzed relationships between immune and stromal scores, and TYMP expression. Using the ESTIMATE algorithm, scores for stromal and immune cells were calculated for 33 tumor types, and correlations between them and TYMP expression were analyzed. In KICH, OV, PCPG, SARC, and TGCT, TYMP and immune scores were positively correlated according to this analysis. The most significant associations between TYMP expression and stromal scores were observed in GBM, KICH, LGG, PCPG, and UVM. Figure 7 displays the results for the top 5 tumor types with the highest correlation coefficients, and results of the remaining cancers can be found in Figures S1,S2.
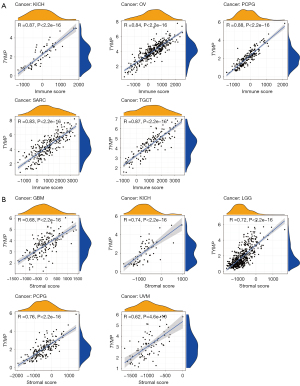
Associations between TYMP expression and immune cell infiltration and immune checkpoint gene (ICG) expression
We explored TYMP expression in relation to the degree of infiltration of 22 immune cell types. TYMP expression was closely linked with the levels of immune cell infiltration in numerous malignancies according to this analysis (Table S1). As presented in Table 1, we selected five tumors that had the most meaningful correlation between TYMP expression and infiltrating immune cells for the next analysis, namely BRCA (n=13), CESC (n=13), KIRC (n=13), SKCM (n=14), and STAD (n=13). In these five tumors, infiltrating CD8 T cells, activated CD4 memory T cells, activated NK cells, and M1 macrophages all had a substantial positive correlation with TYMP expression, while TYMP expression was found to be inversely linked with infiltrating resting CD4 memory T cells. There was also no link between naïve CD4 T cell infiltration and TYMP expression. TYMP expression was also linked to a variety of macrophage subgroups. For instance, infiltrating M1 macrophages were positively associated with TYMP expression in these five tumors, but infiltrating M0 macrophages (except in BRCA) and infiltrating M2 macrophages (except in CESC and STAD) were negatively correlated with TYMP expression. Additionally, differential associations between TYMP expression levels and distinct subgroups of tumor-infiltrating NK cells were also observed. The level of infiltrating activated NK cells was strongly associated with TYMP expression. Except in patients with BRCA, TYMP expression was found to be negatively correlated with invading resting NK cells. Finally, co-expression analysis was conducted to explore the relationships between TYMP expression and ICGs across 33 tumors. As illustrated in Figure 8, we assessed 47 ICGs and found that among all the significant associations, TYMP expression was positively correlated with CTLA4, CD200R1, HAVCR2, PDCD1LG2, VSIR, CD86, and TNFRSF9 in multiple cancer types, but negatively associated with VTCN1 and CD200 in multiple cancers.
Table 1
Cell type | BRCA (P value/Cor) | CESC (P value/Cor) | KIRC (P value/Cor) | SKCM (P value/Cor) | STAD (P value/Cor) |
---|---|---|---|---|---|
Naïve B cells | ***/−0.10 | ***/−0.26 | ***/−0.22 | 0.012 | ***/−0.18 |
Memory B cells | ***/0.17 | **/0.17 | ***/0.17 | 0.047 | */0.11 |
Plasma cells | 0.053 | −0.065 | ***/0.24 | **/0.15 | **/0.16 |
CD8 T cells | ***/0.26 | ***/0.22 | ***/0.45 | ***/0.43 | ***/0.3 |
Naïve CD4 T cells | 0 | 0 | 0 | 0 | 0 |
Resting CD4 memory T cells | ***/−0.31 | ***/−0.31 | ***/−0.32 | ***/−0.29 | ***/−0.48 |
Activated CD4 memory T cells | ***/0.24 | ***/0.24 | ***/0.23 | ***/0.32 | ***/0.31 |
Follicular T helper cells | **/0.081 | ***/0.21 | ***/0.37 | 0.076 | ***/0.26 |
Regulatory T cells | ***/0.22 | 0.027 | ***/0.36 | ***/0.26 | **/0.14 |
Gamma delta T cells | 0.019 | −0.000007 | 0.015 | **/−0.14 | 0 |
Resting NK cells | 0 | −0.058 | ***/−0.27 | */−0.12 | −0.084 |
Activated NK cells | ***/0.34 | ***/0.21 | ***/0.29 | ***/0.27 | ***/0.27 |
Monocytes | **/0.09 | 0.095 | 0.014 | */0.12 | 0.074 |
M0 macrophages | 0.034 | ***/−0.20 | −0.069 | ***/−0.21 | −0.044 |
M1 macrophages | ***/0.20 | ***/0.40 | ***/0.16 | **/0.15 | ***/0.27 |
M2 macrophages | ***/−0.13 | ***/0.22 | ***/−0.39 | ***/−0.29 | 0.054 |
Resting dendritic cells | −0.043 | **/0.18 | −0.02 | 0.059 | −0.026 |
Activated dendritic cells | ***/0.13 | −0.011 | −0.029 | 0.061 | ***/0.27 |
Resting mast cells | ***/−0.12 | */0.13 | ***/−0.34 | */−0.11 | 0.047 |
Activated mast cells | 0 | */−0.13 | 0 | −0.08 | ***/−0.19 |
Eosinophils | 0 | 0 | 0 | 0 | */−0.13 |
Neutrophils | 0.0087 | −0.04 | −0.038 | */0.12 | 0.087 |
*P<0.05; **P<0.01; ***P<0.001. BRCA, breast cancer; CESC, cervical cancer; KIRC, kidney clear cell carcinoma; SKCM, skin cutaneous melanoma; STAD, stomach adenocarcinoma.
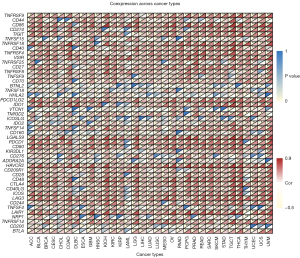
Associations of TYMP with drug sensitivity in pan-cancer
A Pearson correlation analysis was performed to explore relationships between TYMP expression in the NCI-60 tumor cell lines and susceptibility to 263 antitumor drugs, in order to evaluate potential drug responsiveness. As shown in Figure 9, displaying all significant results, we found that TYMP expression correlated positively with susceptibility to 8 antitumor drugs, namely SCH-900776, EPZ-015666, alectinib, VE-822, LDK-378, vismodegib, CCT-251545, and itraconazole. However, there was a significant negative association with sensitivity to ARQ-087. Taken together, these findings suggest that TYMP could be useful for predicting drug response.
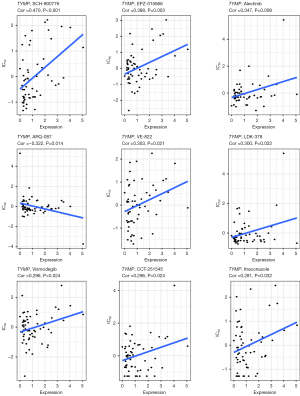
GSEA
Finally, a GSEA analysis of TYMP expression in 33 types of tumor tissues was performed to determine its biological role. Gene Ontology (GO) and Kyoto Encyclopedia of Genes and Genomes (KEGG) analyses were conducted to explore the functional equivalents of high or low TYMP expression in cancers. Figure 10 depicts the major pathways identified by GO and KEGG analyses for eight different cancer types. GO enrichment terms revealed that TYMP positively regulated biological processes including immune response regulating signaling pathways and leukocyte migration in ACC, GBM, KICH, and LGG. TYMP expression was mainly associated with immunity-related activities in PRAD, as shown in Figure 10A. Additionally, TYMP was associated with intermediate filament, intermediate filament cytoskeleton, RNA polymerase binding, and RNA polymerase core enzyme binding in LIHC (Figure 10A). At the same time, KEGG enrichment terms showed that TYMP expression was mainly connected to immune-related pathways, metabolic-related activities, and tumor biological activity. Cytokine receptor interactions and chemokine signaling pathways were positively regulated by TYMP in ACC, LIHC, KICH, and GBM, and TYMP was involved in the signaling pathways of T cell receptors in ACC, LGG, and PCPG, TYMP also participated in the B cell receptor signaling pathway in PCPG. However, TYMP expression was negatively correlated with several drug metabolic enzymes in HNSC. In parallel, TYMP expression was positively correlated with the JAK/STAT signaling pathway in LGG and PRAD, and in LIHC, PCPG, and KICH, TYMP expression was enriched in the Nod-like receptor signaling pathway (Figure 10B).
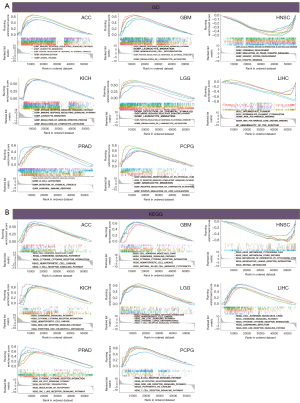
DiscussionOther Section
Based on available studies, TYMP participates in the metabolism of chemotherapeutic drugs such as 5-FU and capecitabine, and is associated with chemoresistance, as well possibly assisting in predicting susceptibility to chemotherapeutic drugs (25-27). TYMP plays a vital role in promoting tumor progression, and overexpression of TP enhanced viability and decreased apoptosis in human cholangiocarcinoma cell lines, as well as promoting angiogenesis in intrahepatic cholangiocarcinoma (28). Furthermore, TYMP itself serves as a therapeutic target in cancers and has been utilized in cancer treatment as a TP inhibitor (13). However, its connection to the tumor immune microenvironment remains unclear. Therefore, the role of TYMP across human cancers in a pan-cancer analysis was comprehensively explored here. We report that TYMP expression is significantly increased in 15 types of cancer in TCGA database, and is also highly expressed in multiple tumor cells, especially in the PRAD, cervical, pancreatic, and HNSC cells. TYMP expression levels were higher in BLCA, BRCA, ESCA, KIRC, and LIHC, consistent with the results of previous studies (16,29-32). TYMP is a major enzyme in the 5-FU pathway and is frequently mutated in gastric cancer (33). Upregulation of TYMP, one of the most commonly altered genes leading to 5-FU resistance, was found to be the origin of this increased vasculogenic potential (34). Our Cox proportional hazards analysis demonstrated that PAAD patients with higher TYMP expression had a poorer OS, while in BRCA patients it tended to be associated with a better outcome. Similarly, high expression of TYMP predicted a longer survival time in BRCA patients, and low TYMP expression a higher OS in pancreatic adenocarcinoma, although not statistically significant. These data are also similar to those reported in previous studies, and are further supported by assessments of prognostic value in our work (35,36). Moreover, prognosis is also affected by TYMP expression among multiple cancers reported in previous studies, including gastric carcinoma, CESC, renal cell carcinoma, and hepatocellular carcinoma (11,15,37,38). Overall, in many types of cancer, TYMP expression is associated with a poor prognosis. Additionally, TYMP expression was shown to be associated with tumor stage among 12 types of cancer. Of these, TYMP expression was associated with the tumor stage in most malignant tumors, with a notable difference between early and advanced cancers. In ACC, BLCA, KIRC, the three types of cancer exhibited a trend that TYMP expression was at a higher level in patients who were in advanced stages. In contrast, TYMP expression was higher in patients at the early stage of COAD, ESCA, and KIRP. This information may help to improve therapies of tumors based on the clinical characteristics of each cancer type.
The TMB represents the number of mutations identified in a tumor, with a higher TMB implying greater numbers of potential tumor neoantigens, which correlates with a better response to immune checkpoint inhibitors (39). Recent studies demonstrated that the TMB can serve as a biomarker to predict the effectiveness of immunotherapy in colorectal cancer and gastric cancer (40,41). In addition, the TMB predicts the prognosis of patients treated by immunotherapy across multiple cancer types (42). Currently, MSI also functions as a biomarker for PD1/PD-L1-blockade. A previous review contended that MSI status was helpful for deciding on cancer treatment because of its predictive value (43). Colorectal cancer patients with high MSI had a longer PFI when treated with pembrolizumab than with chemotherapy (44). TYMP expression was linked to the TMB in 15 tumor types and MSI in 10 tumor types, according to our findings. This implies that TYMP expression influences the TMB and MSI of tumors and affects how patients respond to immune checkpoint inhibitors, and may thus represent a novel prognostic biomarker for immunotherapies in many different tumors.
Features of the TME can be used to assess immune responses to cancer, and assess influence on clinical outcomes (24). Our results suggest that TYMP is crucial for cancer immunity. Regarding immune scores and stromal scores, positive correlations between the former and TYMP expression were identified in 32 tumor types, and for stromal scores in 25 tumor types. The degree of immune cell infiltration contributes to predicting cancer patient prognosis (45,46). We also examined associations between TYMP and immune cell infiltration in 33 different tumor types, and found that TYMP expression was positively associated with the presence of memory B cells and CD8 T cells. An earlier study had identified TYMP-positive macrophages as promoters of angiogenesis and metastasis in gastric cancer (47). In BRCA patients, TYMP expression in macrophages had also been linked to tumor angiogenesis and prognosis (48). Hence, we further conducted co-expression analyses of TYMP and ICGs, most of which were significantly related to TYMP. Results showed that TYMP expression and tumor immunity were closely associated, influencing cancer progression and the patient prognosis.
Moreover, our findings showed that TYMP expression was correlated to responses to a number of chemotherapeutic drugs, such as SCH-900776, EPZ-015666, and alectinib. A previous study had demonstrated that TYMP expression was substantially linked to the capecitabine response (26). Finally, our GSEA indicated that TYMP influenced many biological processes in different tumors through pathways involving cell adhesion, immune response regulating signaling pathway, leukocyte migration, T cell, and B cell receptor pathways. Nonetheless, the molecular mechanisms of TYMP involvement in tumorigenesis remain poorly understood. GSEA can help uncover some important biological processes.
In sum, we conclude that the TYMP gene expressed at a higher level in the majority of tumors relative to the respective normal tissues. We explored correlations between TYMP expression, clinical prognosis and tumor stage. We suggest that TYMP could be employed as a prognostic marker for a range of malignancies. Moreover, in various types of cancers, TYMP expression has been related to TMB, MSI, and immune cell infiltration. Furthermore, TYMP expression is associated with the effectiveness of several chemotherapeutic drugs. These findings reveal something of TYMP’s function in cancer etiology, pathology and progression, and further contribute to immunotherapeutic strategies. Validation will require future experimental work.
AcknowledgmentsOther Section
The authors would like to thank the public databases including TCGA, Oncomine, GEPIA, CCLE, cBioPortal, and CellMiner databases for providing data. We would like to express our gratitude to EditSprings (https://www.editsprings.cn/) for the expert linguistic services provided.
Funding: This work was supported by
FootnoteOther Section
Reporting Checklist: The authors have completed the REMARK reporting checklist. Available at https://tcr.amegroups.com/article/view/10.21037/tcr-22-502/rc
Data Sharing Statement: Available at https://tcr.amegroups.com/article/view/10.21037/tcr-22-502/dss
Conflicts of Interest: All authors have completed the ICMJE uniform disclosure form (available at https://tcr.amegroups.com/article/view/10.21037/tcr-22-502/coif). The authors have no conflicts of interest to declare.
Ethical Statement: The authors are accountable for all aspects of the work in ensuring that questions related to the accuracy or integrity of any part of the work are appropriately investigated and resolved. The study was conducted in accordance with the Declaration of Helsinki (as revised in 2013).
Open Access Statement: This is an Open Access article distributed in accordance with the Creative Commons Attribution-NonCommercial-NoDerivs 4.0 International License (CC BY-NC-ND 4.0), which permits the non-commercial replication and distribution of the article with the strict proviso that no changes or edits are made and the original work is properly cited (including links to both the formal publication through the relevant DOI and the license). See: https://creativecommons.org/licenses/by-nc-nd/4.0/.
ReferencesOther Section
- Sung H, Ferlay J, Siegel RL, et al. Global Cancer Statistics 2020: GLOBOCAN Estimates of Incidence and Mortality Worldwide for 36 Cancers in 185 Countries. CA Cancer J Clin 2021;71:209-49. [Crossref] [PubMed]
- Steeg PS. Targeting metastasis. Nat Rev Cancer 2016;16:201-18. [Crossref] [PubMed]
- Kishton RJ, Sukumar M, Restifo NP. Metabolic Regulation of T Cell Longevity and Function in Tumor Immunotherapy. Cell Metab 2017;26:94-109. [Crossref] [PubMed]
- Ribas A, Wolchok JD. Cancer immunotherapy using checkpoint blockade. Science 2018;359:1350-5. [Crossref] [PubMed]
- Wang J, Chen W, Wang F, et al. Nutrition Therapy for Mitochondrial Neurogastrointestinal Encephalopathy with Homozygous Mutation of the TYMP Gene. Clin Nutr Res 2015;4:132-6. [Crossref] [PubMed]
- Liekens S, Bronckaers A, Pérez-Pérez MJ, et al. Targeting platelet-derived endothelial cell growth factor/thymidine phosphorylase for cancer therapy. Biochem Pharmacol 2007;74:1555-67. [Crossref] [PubMed]
- Pacitti D, Levene M, Garone C, et al. Mitochondrial Neurogastrointestinal Encephalomyopathy: Into the Fourth Decade, What We Have Learned So Far. Front Genet 2018;9:669. [Crossref] [PubMed]
- Grimminger PP, Schneider PM, Metzger R, et al. Low thymidylate synthase, thymidine phosphorylase, and dihydropyrimidine dehydrogenase mRNA expression correlate with prolonged survival in resected non-small-cell lung cancer. Clin Lung Cancer 2010;11:328-34. [Crossref] [PubMed]
- Saito K, Khan K, Yu SZ, et al. The predictive and therapeutic value of thymidine phosphorylase and dihydropyrimidine dehydrogenase in capecitabine (Xeloda)-based chemotherapy for head and neck cancer. Laryngoscope 2009;119:82-8. [Crossref] [PubMed]
- Kumagai Y, Tachikawa T, Higashi M, et al. Thymidine phosphorylase and angiogenesis in early stage esophageal squamous cell carcinoma. Esophagus 2018;15:19-26. [Crossref] [PubMed]
- Wang L, Huang X, Chen Y, et al. Prognostic value of TP/PD-ECGF and thrombocytosis in gastric carcinoma. Eur J Surg Oncol 2012;38:568-73. [Crossref] [PubMed]
- Koumarianou A, Tzeveleki I, Mekras D, et al. Prognostic markers in early-stage colorectal cancer: significance of TYMS mRNA expression. Anticancer Res 2014;34:4949-62. [PubMed]
- Li W, Yue H. Thymidine phosphorylase: A potential new target for treating cardiovascular disease. Trends Cardiovasc Med 2018;28:157-71. [Crossref] [PubMed]
- Furukawa T, Tabata S, Yamamoto M, et al. Thymidine phosphorylase in cancer aggressiveness and chemoresistance. Pharmacol Res 2018;132:15-20. [Crossref] [PubMed]
- Zhang Q, Zhang Y, Hu X, et al. Thymidine phosphorylase promotes metastasis and serves as a marker of poor prognosis in hepatocellular carcinoma. Lab Invest 2017;97:903-12. [Crossref] [PubMed]
- Yang XR, Xu Y, Yu B, et al. High expression levels of putative hepatic stem/progenitor cell biomarkers related to tumour angiogenesis and poor prognosis of hepatocellular carcinoma. Gut 2010;59:953-62. [Crossref] [PubMed]
- Slager EH, Honders MW, van der Meijden ED, et al. Identification of the angiogenic endothelial-cell growth factor-1/thymidine phosphorylase as a potential target for immunotherapy of cancer. Blood 2006;107:4954-60. [Crossref] [PubMed]
- Cerami E, Gao J, Dogrusoz U, et al. The cBio cancer genomics portal: an open platform for exploring multidimensional cancer genomics data. Cancer Discov 2012;2:401-4. [Crossref] [PubMed]
- Fusco MJ, West HJ, Walko CM. Tumor Mutation Burden and Cancer Treatment. JAMA Oncol 2021;7:316. [Crossref] [PubMed]
- Gilson P, Merlin JL, Harlé A. Detection of Microsatellite Instability: State of the Art and Future Applications in Circulating Tumour DNA (ctDNA). Cancers (Basel) 2021;13:1491. [Crossref] [PubMed]
- Yoshihara K, Shahmoradgoli M, Martínez E, et al. Inferring tumour purity and stromal and immune cell admixture from expression data. Nat Commun 2013;4:2612. [Crossref] [PubMed]
- Newman AM, Liu CL, Green MR, et al. Robust enumeration of cell subsets from tissue expression profiles. Nat Methods 2015;12:453-7. [Crossref] [PubMed]
- Reinhold WC, Sunshine M, Liu H, et al. CellMiner: a web-based suite of genomic and pharmacologic tools to explore transcript and drug patterns in the NCI-60 cell line set. Cancer Res 2012;72:3499-511. [Crossref] [PubMed]
- Wu T, Dai Y. Tumor microenvironment and therapeutic response. Cancer Lett 2017;387:61-8. [Crossref] [PubMed]
- Wang Y, Wei Q, Chen Y, et al. Identification of Hub Genes Associated With Sensitivity of 5-Fluorouracil Based Chemotherapy for Colorectal Cancer by Integrated Bioinformatics Analysis. Front Oncol 2021;11:604315. [Crossref] [PubMed]
- Marangoni E, Laurent C, Coussy F, et al. Capecitabine Efficacy Is Correlated with TYMP and RB1 Expression in PDX Established from Triple-Negative Breast Cancers. Clin Cancer Res 2018;24:2605-15. [Crossref] [PubMed]
- Kobashi N, Matsumoto H, Zhao S, et al. The Thymidine Phosphorylase Imaging Agent 123I-IIMU Predicts the Efficacy of Capecitabine. J Nucl Med 2016;57:1276-81. [Crossref] [PubMed]
- Li S, Yang H, Li K, et al. Thymidine phosphorylase promotes angiogenesis and tumour growth in intrahepatic cholangiocarcinoma. Cell Biochem Funct 2020;38:743-52. [Crossref] [PubMed]
- Naoe M, Ogawa Y, Morita J, et al. Expression of the fluoropyrimidine-metabolizing enzymes in bladder cancers as measured by the Danenberg tumor profile. Oncol Res 2009;18:153-62. [Crossref] [PubMed]
- Zizzo N, Passantino G, D'alessio RM, et al. Thymidine Phosphorylase Expression and Microvascular Density Correlation Analysis in Canine Mammary Tumor: Possible Prognostic Factor in Breast Cancer. Front Vet Sci 2019;6:368. [Crossref] [PubMed]
- Takebayashi Y, Natsugoe S, Baba M, et al. Thymidine phosphorylase in human esophageal squamous cell carcinoma. Cancer 1999;85:282-9. [Crossref] [PubMed]
- Liu M, Pan Q, Xiao R, et al. A cluster of metabolism-related genes predict prognosis and progression of clear cell renal cell carcinoma. Sci Rep 2020;10:12949. [Crossref] [PubMed]
- Biagioni A, Staderini F, Peri S, et al. 5-Fluorouracil Conversion Pathway Mutations in Gastric Cancer. Biology (Basel) 2020;9:265. [Crossref] [PubMed]
- Peri S, Biagioni A, Versienti G, et al. Enhanced Vasculogenic Capacity Induced by 5-Fluorouracil Chemoresistance in a Gastric Cancer Cell Line. Int J Mol Sci 2021;22:7698. [Crossref] [PubMed]
- Ruckhäberle E, Karn T, Engels K, et al. Prognostic impact of thymidine phosphorylase expression in breast cancer--comparison of microarray and immunohistochemical data. Eur J Cancer 2010;46:549-57. [Crossref] [PubMed]
- Saif MW, Hashmi S, Bell D, et al. Prognostication of pancreatic adenocarcinoma by expression of thymidine phosphorylase/dihydropyrimidine dehydrogenase ratio and its correlation with survival. Expert Opin Drug Saf 2009;8:507-14. [Crossref] [PubMed]
- Nakashima M, Nakano T, Ametani Y, et al. Expression of thymidine phosphorylase as an effect prediction factor for uterine cervical squamous cell carcinoma after radiotherapy: an immunohistochemical study. Int J Gynecol Cancer 2006;16:1309-13. [Crossref] [PubMed]
- Huang X, Wang L, Chen Y, et al. Poor Prognosis Associated with High Levels of Thymidine Phosphorylase and Thrombocytosis in Patients with Renal Cell Carcinoma. Urol Int 2017;98:162-8. [Crossref] [PubMed]
- Jardim DL, Goodman A, de Melo Gagliato D, et al. The Challenges of Tumor Mutational Burden as an Immunotherapy Biomarker. Cancer Cell 2021;39:154-73. [Crossref] [PubMed]
- Schrock AB, Ouyang C, Sandhu J, et al. Tumor mutational burden is predictive of response to immune checkpoint inhibitors in MSI-high metastatic colorectal cancer. Ann Oncol 2019;30:1096-103. [Crossref] [PubMed]
- Li Z, Jia Y, Zhu H, et al. Tumor mutation burden is correlated with response and prognosis in microsatellite-stable (MSS) gastric cancer patients undergoing neoadjuvant chemotherapy. Gastric Cancer 2021;24:1342-54. [Crossref] [PubMed]
- Samstein RM, Lee CH, Shoushtari AN, et al. Tumor mutational load predicts survival after immunotherapy across multiple cancer types. Nat Genet 2019;51:202-6. [Crossref] [PubMed]
- Dudley JC, Lin MT, Le DT, et al. Microsatellite Instability as a Biomarker for PD-1 Blockade. Clin Cancer Res 2016;22:813-20. [Crossref] [PubMed]
- André T, Shiu KK, Kim TW, et al. Pembrolizumab in Microsatellite-Instability-High Advanced Colorectal Cancer. N Engl J Med 2020;383:2207-18. [Crossref] [PubMed]
- Zhou R, Zhang J, Zeng D, et al. Immune cell infiltration as a biomarker for the diagnosis and prognosis of stage I-III colon cancer. Cancer Immunol Immunother 2019;68:433-42. [Crossref] [PubMed]
- Sui S, An X, Xu C, et al. An immune cell infiltration-based immune score model predicts prognosis and chemotherapy effects in breast cancer. Theranostics 2020;10:11938-49. [Crossref] [PubMed]
- Kawahara A, Hattori S, Akiba J, et al. Infiltration of thymidine phosphorylase-positive macrophages is closely associated with tumor angiogenesis and survival in intestinal type gastric cancer. Oncol Rep 2010;24:405-15. [Crossref] [PubMed]
- Nagaoka H, Iino Y, Takei H, et al. Platelet-derived endothelial cell growth factor/thymidine phosphorylase expression in macrophages correlates with tumor angiogenesis and prognosis in invasive breast cancer. Int J Oncol 1998;13:449-54. [Crossref] [PubMed]