Development of novel gene signatures for the risk stratification of prognosis and diagnostic prediction of osteosarcoma patients using bioinformatics analysis
Introduction
Osteosarcoma (OS) is a common malignant bone cancer in children and teenagers that originates from osteoblast cells. The majority of patients with early stage OS and localized tumors can be cured; however, the 5-year survival rate for OS patients with metastasis or recurrence is extremely low at 14% (1). About 30% of patients relapse within 2 years (2). Additionally, 80–90% of OS patients are diagnosed with a high grade, which poses a further significant challenge for OS treatment (3). Currently, biomarker identification has been applied in the individualized management of tumor patients, such as in the direction of therapy and prognosis. Thus, novel biomarkers for the early diagnosis and prognosis of OS urgently need to be identified to improve the management of OS.
Numerous factors have been reported to contribute to the progression of OS, including gender, age, and familial and genetic factors (4). Additionally, there is accumulating evidence that genetic factors have important implications in OS pathogenesis (5-7). Various genes are dysregulated and have been identified as promising biomarkers for detection or prognosis. For example, TGF-β (8,9) was recognized as a promising diagnostic marker in OS and it presented a higher level in serum of OS patients compared with healthy individuals. Ezrin (10,11) was identified as a diagnostic and prognostic marker in OS. It was up-regulated in high-grade OS patients and points to a worse prognosis. Moreover, Cyclin E1 (12), MMP-9 (13), HIF-1 (14) and APE1 (15) were identified as prognostic markers of OS, and their high expression were correlated with adverse prognosis. In addition, high-mobility group box 2 was found to be upregulated in OS and negatively associated with the survival of OS patients (16). Xi et al. demonstrated that the upregulation of Transducin (beta)-like 1 X-linked receptor 1 promotes the initiation and recurrence of OS (17). Recently, using high-throughput gene expression databases such as the Gene Expression Omnibus (GEO), The Cancer Genome Atlas (TCGA), and the Therapeutically Applicable Research to Generate Effective Treatments (TARGET) databases, researchers have detected various molecular signatures associated with OS prognosis. For example, Zhang et al. identified 2 metastasis- and recurrence-related genes in the GEO database (18). Although many biomarkers have been reported in OS, they have not improved the prognosis of this disease, which possibly due to the researches being flawed, too heterogeneous or lacking valid evaluation (19). Development of effective markers for diagnosis, prognosis and predicting recurrence of OS patients, may prove a much-needed strategy for early diagnosis and develop promising therapeutic targets. Thus, in the present study, we first screened out OS-associated microRNAs (miRNAs) in the Human microRNA Disease Database (HMDD). By comparing the targets of these miRNAs with the differentially expressed genes (DEGs) in response to OS, we identified OS-associated candidate genes. After conducting a comprehensive bioinformatics analysis, including univariate and multivariate Cox, and Kaplan-Meier (KM) analyses, we identified 3 prognosis-related genes in OS. The 3 genes were integrated into a prognostic index (risk-score model) for OS survival and recurrence stratification. Next, 2 of the 3 genes were found to be associated with OS diagnosis and incorporated into a logistics model for diagnostic prediction. We present the following article in accordance with the TRIPOD reporting checklist (available at https://tcr.amegroups.com/article/view/10.21037/tcr-22-1706/rc).
Methods
Research design
This study screened prognosis-related genes and constructed gene signatures for early diagnosis and prognostic prediction of OS based on the data obtained from public databases using a comprehensive bioinformatics analysis.
Selection of potential prognosis-related genes in OS
The HMDD, an online public repository, contains information about the miRNAs related to various human diseases. The study was conducted in accordance with the Declaration of Helsinki (as revised in 2013). In this study, 180 miRNAs related to OS and 67 miRNAs associated with the prognosis of OS were downloaded from the HMDD. A Venn diagram was used to compare the 180 miRNAs and the 67 miRNAs. Ultimately, 28 miRNA hub genes associated with prognosis of OS were identified. The target genes of the 28 miRNAs were predicted by TargetScan and miRDB. The DEGs related to OS were screened using 3 data sets from the GEO repository; that is, GSE16088 (which comprised 9 normal and 14 tumor samples), GSE36001 (which comprised 6 normal and 19 tumor samples), and GSE56001 (which comprised 6 normal and 6 tumor samples). The DEGs in each data set were identified using Limma package in R and integrated using the robust rank aggregation (RRA) method. Genes that had a |log2fold change (FC)| ≥0.5 and a P value <0.05 were recognized as the DEGs. The potential prognosis-related OS genes were identified by comparing the DEGs with the predicted target genes of the 28 miRNAs from TargetScan and miRDB.
Construction of a prognosis signature for OS survival and recurrence
The TARGET database was used to download the gene expression and clinical data for OS. There were 96 samples with complete clinical information for OS. A univariate Cox analysis was performed to detect the prognosis-associated genes. Next, the samples were divided into two groups (high- and low-expression groups) according to the median expression of the prognosis-associated gene. KM curves were plotted to analyze the prognostic value of the prognosis-associated genes. The proportional hazard (PH) assumption was assessed by calculating Pearson’s coefficients to determine whether the effect of the factor (here is the expression of the genes) on survival was independent of time (20). Genes that met the PH assumption were analyzed by forward and backward stepwise multivariate Cox regression, and 3 genes were ultimately identified.
Evaluation of the gene signature
Risk scores were calculated based on the expression of the core genes that met these criteria. The optimal cutoff of risk score was calculated using X-tile software, and the OS samples were then stratified into high- and low-risk groups. The “survivalROC” package in R was installed to plot the receiver operating characteristic (ROC) curves. The areas under the ROC curves (AUCs) were calculated to evaluate the prognostic value of the risk score for 3-, 5-, 10-, and 15-year survival. Univariate and multivariate Cox analyses were performed to examine the prognostic value of the risk score and X-tile classification. A nomogram was established by integrating the genes. The ROC and calibration curves were plotted to analyze the predictive performance of the nomogram. The gene expression and survival information of 53 OS samples in GSE21257 data set was downloaded to validate the nomogram.
Gene set enrichment analysis (GSEA)
To explore the biological function of the nomogram model, the Kyoto Encyclopedia of Genes and Genomes (KEGG) pathways were analyzed using the GSEA method in the TARGET database. When the P value was <0.05, a pathway was considered significantly enriched.
Construction of a signature for OS diagnosis
The GSE42352 data set (which comprised 15 normal and 103 tumor samples) was used to determine whether the 3 prognosis-associated genes could be also applied for OS diagnosis. A logistic regression was conducted to assess the diagnostic value of the genes. The goodness of fit for the logistic model was evaluated using the Akaike information criterion (AIC); a lower AIC value indicated a better fit. A nomogram was constructed for diagnosis, and the predictive performance was evaluated by the Hosmer-Lemeshow test. A principal component analysis (PCA) with a 95% confidence ellipse was performed to examine the discriminative performance of the diagnosis model. The GSE19276 (which comprised 5 normal and 44 tumor samples) and GSE36001 (which comprised 6 normal and 19 tumor samples) data sets were used to validate the diagnosis signature. The accuracy and diagnostic utility of the diagnosis signature were evaluated based upon the AUC.
Statistical analysis
KM analysis was performed for survival analysis with two-sided log-rank tests for comparison. Univariate and multivariate Cox analyses were performed to examine the prognostic value of the prognostic gene signature. Hazard ratios (HRs) and 95% confidence intervals (CIs) were calculated. A logistic regression was conducted to assess the diagnostic value of the genes and establish the diagnostic model. The accuracy and prognostic/diagnostic utility of the prognostic/diagnostic signatures were evaluated based upon the AUC as calculated by the “survivalROC” package in R. The value of AUC ranges from 0.5 to 1. The closer AUC is to 1.0, the higher the accuracy of the detection prognostic/diagnostic signature is. Statistical analyses were conducted in R version 4.0.4 (The R Foundation for Statistical Computing, Vienna, Austria) and SPSS 22.0 (IBM Corp., Armonk, NY, USA). A P value <0.05 was considered significant.
Results
Screening for OS-associated genes
We downloaded 180 miRNAs related to OS and 67 miRNAs related to survival from the HMDD database. After comparing the 2 types of miRNAs, 28 common miRNAs were obtained (see Figure 1A). To screen out the OS-associated genes, the following steps were performed. First, 3 data sets from the GEO database (i.e., GSE16088, GSE36001, and GSE56001) were downloaded to identify the DEGs (see Figure 1B). To minimize the batch effect, the RRA method was used to integrate the DEGs. A total of 1,770 DEGs were detected, including 1,019 upregulated and 751 downregulated genes that met the criteria of a |log2FC| ≥0.5 and a P value <0.05. Second, the target genes of the 28 miRNAs were predicted by miRDB and TargetScan. A total of 10,512 and 3,549 target genes were detected by miRDB and TargetScan, respectively. Third, the similarities between the 1,770 DEGs and the 2 groups of the target genes were analyzed by a Venn diagram. Ultimately, 267 intersected genes were identified, which were defined as the OS-associated genes (see Figure 1C).
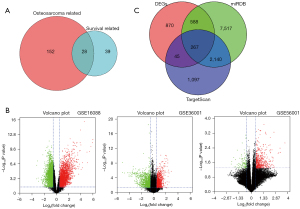
Construction of a prognostic model for OS
We used 96 OS samples with gene expression and complete survival information in the TARGET database to establish the prognostic model. A univariate Cox analysis was conducted to analyze the association between the 267 OS-associated genes and OS survival, and 34 genes were found to be associated with the outcomes of OS (P<0.05; see Figure S1). Subsequently, the prognostic value of the 34 genes were further analyzed by KM curves. The 96 OS samples were divided into high- and low-expression groups based on the cutoff of the median expression of each gene. The survival differences between the high- and low-expression groups of 14 genes were significant (P<0.05; see Figure S2). Next, the PH assumption was tested for the following multivariate Cox analysis. The PH assumptions for DEK and RH0BTB1 were refuted, as the scaled Schoenfeld residuals of the 2 genes were significantly associated with survival time. However, the PH assumption was supported for the remaining 12 genes. Next, 3 prognosis-associated genes were selected in the 12 genes by a stepwise multivariate Cox analysis. Finally, a prognostic signature comprised of 3 genes [i.e., signal transducer and activators of transcription factor 4 (STAT4), heat shock protein family E member 1 (HSPE1), and actin-related protein 2/3 complex subunit 5 (ARPC5)] was developed for OS (see Table 1).
Table 1
ID | Coef | P value | HR | Low 95% CI | High 95% CI |
---|---|---|---|---|---|
STAT4 | −0.0101 | 0.0112 | 0.9899 | 0.9822 | 0.9977 |
HSPE1 | 0.1698 | 0.1362 | 1.1851 | 0.9478 | 1.4818 |
ARPC5 | −0.0037 | 0.0526 | 0.9963 | 0.9926 | 1.00004 |
STAT4, signal transducer and activators of transcription factor 4; HSPE1, heat shock protein family E member 1; ARPC5, actin-related protein 2/3 complex subunit 5; Coef, coefficient; HR, hazard ratio; CI, confidence interval.
Evaluation of the 3-gene signature for OS survival
Based on the 3 prognosis-associated genes mentioned above, the risk score of each sample was calculated by summing the product of the expression level of each gene and its corresponding coefficient. The optimal cutoff value of the risk score was identified as 1.3 by X-title (see Figure 2A). Based on this cutoff value, 96 OS samples were stratified into the following 2 groups: (I) the high-risk group, containing 34 samples; and (II) the low-risk group, containing 62 samples. As Figure 2B shows, the number of deaths increased as the risk score increased. Additionally, the survival rate of the high-risk group was significantly lower than that of the low-risk group (P<0.05; see Figure 2C). Similar results were obtained after risk stratification based on the median risk score (P<0.05; see Figure 2D). Figure 2E shows the ROC curves for 1-, 3-, 5-, 10-, and 15-year survival for the prognostic model. The AUCs for 3-, 5-, 10-, and 15-year survival were all >0.7, which suggests that the model has excellent discriminative performance.
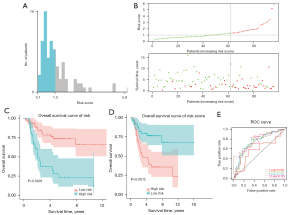
Next, to examine the prognostic value of the risk score and the risk stratification obtained by X-tile, we performed univariate and multivariate Cox analyses using these 2 factors and 2 clinical features (i.e., gender and metastasis status). As Figure 3A-3C show, risk score and risk stratification obtained by X-tile were independent prognostic factors for OS.
To examine the predictive performance of the 3-gene signature, a nomogram was developed by integrating the expression of the 3 prognosis-related genes (i.e., STAT4, HSPE1, and ARPC5) to predict 3-, 5-, 10-, and 15-year survival (see Figure 3D). In the nomogram, the point of each gene was obtained easily based on its expression level. The total points of 1 patient could then be calculated by summing up all the gene points. According to the nomogram, HSPE1 had the greatest effect on OS prognosis. In particular, the calibration curves for 3-, 5-, 10-, and 15-year survival had good consistency between the predicted and observed results, which indicated that the nomogram predicted OS survival accurately (see Figure 3E). All these results demonstrated the accurate predictive performance of the 3-gene signature for OS survival.
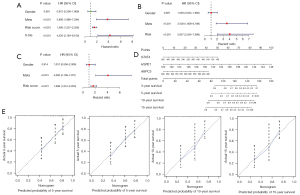
Validation of the 3-gene signature
An external data set GSE21257 comprising the gene expression and survival information of 53 OS samples was used to validate the prognostic signature. X-tile was used to divide the 53 samples into the following 2 groups based on risk score: (I) the high-risk group, containing 13 samples; and (II) the low-risk group, containing 40 samples. As Figure 4A shows, there were more deaths in the high-risk group than the low-risk group. The overall survival rate of the low-risk group was significantly higher than that of the high-risk group (P<0.05; see Figure 4B). Additionally, the AUCs were high (all >0.7) for the prediction of 1-, 3-, and 5-year survival, suggesting a good discriminative performance (see Figure 4C). The nomogram was developed to predict 1-, 3-, and 5-year survival (see Figure 4D). The high consistency of the observed and predicted results in the calibration curves indicated that the predictive performance of the 3-gene signature was good (see Figure 4E).
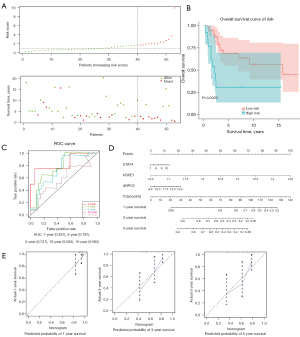
GSEA
To perform the biological annotation of the 3 genes, the GSEA method was used to explore the KEGG pathways enriched in the high- and low-risk groups in the TARGET database. A total of 34 signaling pathways were significantly enriched in the low-risk group (P<0.05; see Table S1). The top 3 pathways enriched with the most genes were the mitogen-activated protein kinase (MAPK) signaling pathway, the regulation of actin cytoskeleton pathway, and the calcium signaling pathway. Additionally, 1 signaling pathway was enriched in high-risk group; that is, the cytosolic DNA sensing pathway.
Construction of the 3-gene signature for recurrence prediction
Given the high rate of recurrence in OS, we next investigated whether the 3 genes could be used to predict OS recurrence in the TARGET database. In this database, the 76 OS samples with gene expression and complete recurrence data contained 32 cases with recurrence and 44 cases without recurrence. The 3 genes (i.e., STAT4, HSPE1, and ARPC5) were incorporated into a multivariate Cox regression model, and the coefficient for each gene is displayed in Table 2. The risk score for each patient was calculated, and the samples were then divided into high- (n=22) and low-risk (n=54) groups using X-tile. As Figure 5A shows, the number of patients with recurrence increased along with the risk score. As the KM curves in Figure 5B show, the high-risk patients had a significantly higher relapse rate than the low-risk patients (P<0.05). Additionally, the AUCs for predicting 3- and 10-year relapse were high (both >0.7), which shows the excellent predictive performance of our signature (see Figure 5C). Further, the univariate and multivariate Cox analyses revealed that risk stratification by X-tile was independently associated with the relapse of OS (P<0.05; see Figure 5D,5E). A nomogram was constructed to predict the 3- and 10-year relapse rate of OS patients (see Figure 5F). In the nomogram, HSPE1 had the greatest effect on the OS relapse rate. The calibration curves showed the high predictive reliability of the 3-gene signature for the 3- and 10-year relapse rates of OS (see Figure 5G).
Table 2
ID | Coef | P value | HR | Low 95% CI | High 95% CI |
---|---|---|---|---|---|
STAT4 | −0.005299372 | 0.117497645 | 0.994714644 | 0.988136313 | 1.00133677 |
HSPE1 | 0.135647621 | 0.187789355 | 1.145278251 | 0.935942617 | 1.401434498 |
ARPC5 | −0.000277364 | 0.888932242 | 0.999722675 | 0.99583872 | 1.003621778 |
STAT4, signal transducer and activators of transcription factor 4; HSPE1, heat shock protein family E member 1; ARPC5, actin-related protein 2/3 complex subunit 5; Coef, coefficient; HR, hazard ratio; CI, confidence interval.
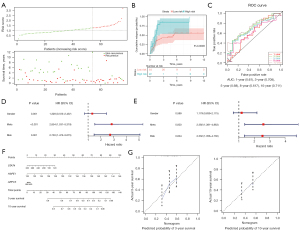
Construction of the 2-gene signature for OS diagnosis
The GSE42352 data set, which comprised 103 OS and 15 normal samples, was used to investigate whether the 3 genes could be used to develop a diagnostic model for OS. First, a logistic regression analysis was conducted to analyze the association between the 3 genes and a diagnosis of OS. STAT4 and HSPE1 were significantly associated with a diagnosis of OS (P<0.05), but no significant correlation was found between ARPC5 and a diagnosis of OS (P>0.05; see Table 3). Additionally, the AIC value for the 3 genes (42.389) was larger than that for the 2 genes (42.329). Thus, the 2 genes (STAT4 and HSPE1) were incorporated into the logistics model for OS diagnosis. The overdispersion occurred in the binomial logistic regression for the 2 genes. The quasibinomial logistic regression model of the 2 genes was thus constructed as the diagnosis signature for OS. The high AUC value (0.981) suggested that the 2-gene diagnosis signature for OS had a good discrimination (see Figure 6A).
Table 3
ID | Estimate | Standard error | Z value | Pr (>|z|) |
---|---|---|---|---|
ARPC5 | 0.7079 | 0.5551 | 1.275 | 0.2022 |
HSPE1 | 1.3956 | 0.6449 | 2.164 | 0.0304* |
STAT4 | −3.6393 | 0.8501 | −4.281 | 1.86e-05*** |
*P<0.05; ***P<0.01. ARPC5, actin-related protein 2/3 complex subunit 5; HSPE1, heat shock protein family E member 1; STAT4, signal transducer and activators of transcription factor 4.
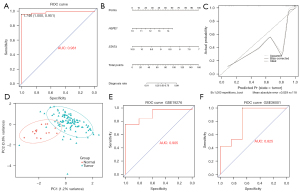
Next, a diagnosis nomogram model was established based on the expression of STAT4 and HSPE1 (see Figure 6B). The diagnostic rate was calculated using the points of the 2 genes. The calibration plot showed that the error between the predicted and actual value was 0.029, which showed the good predictive performance of our model (see Figure 6C). Subsequently, the confidence ellipse based on the PCA was plotted to evaluate the effectiveness of the diagnostic model. As Figure 6D shows, there was little overlap between the normal and tumor ellipses, and their centers were far apart. This demonstrated that the distributions of the principal components in the normal and tumor groups were respectively concentrated and differed from each other. The PCA results indicated that our diagnostic model could discriminate between tumor and normal patients effectively.
Next, the gene expression profiles from the GSE19276 (which comprised 5 normal and 44 tumor cases) and GSE36001 (which comprised 6 normal and 19 tumor cases) data sets were downloaded to validate our diagnostic model. A logistics regression model was used based on the expression of HSPE1 and STAT4. The ROC curves showed that our model had great discriminative power with high AUCs (0.905 in GSE19276 and 0.825 in GSE36001; see Figure 6E,6F). All the results showed the excellent predictive performance of the 2-gene diagnostic signature.
Discussion
OS is a malignant bone tumor that often occurs in children and young adults aged 10–19 years. Recurrence and metastasis severely decrease the survival time and the quality of life of OS patients. Given the importance of early detection and recurrence in OS prognosis, it is imperative that gene biomarkers for OS prognosis, diagnosis, and recurrence be identified.
In this study, the HMDD database and 3 microarray data sets were downloaded to screen OS-associated genes. After comparing the targets of the OS-associated miRNAs and DEGs related to OS, a total of 267 candidate genes associated with OS were identified. Subsequently, the univariate Cox and KM analyses revealed that the expression of 14 of the genes were significantly associated with the outcomes of OS patients. Finally, 3 genes (i.e., STAT4, HSPE1, and ARPC5) were selected based on the PH assumption and stepwise multivariate Cox regression, and we established a 3-gene prognostic signature for the survival and recurrence of OS. The reliability of the prognostic signature was then evaluated and validated by risk stratification and nomogram construction. Additionally, a logistic regression model integrating STAT4 and HSPE1 was analyzed and validated and found to be effective for OS diagnosis.
The 3 genes in the signature have been reported to be involved in the progression of various tumors in many studies. Some of the 7 members of the STAT family have been identified as biomarkers and targets for prognosis and immunotherapy for some cancers; for example, STAT3 and STAT5A in breast, lung, and prostate cancer, and STAT6 in lung cancer (21,22). Many studies have investigated the role of STAT members in OS but have focused on STAT3 and STAT5 (23-25). The role of STAT4 in cancer is controversial. Previous studies have reported that STAT4 promotes the metastasis of gastric and ovarian cancer (26,27). However, in these 2 cancers and breast cancer, the expression of STAT4 was shown to be positively associated with prognosis (28-30).
As critical chaperonins, members of the heat shock protein family play important roles in inhibiting the accumulation of misfolded proteins by promoting the refolding and degradation of these proteins (31). HSPE1 is one of the main constituents of chaperonin in mitochondria and has been proven to be involved in tumor development and progression (32-34). HSPE1 has been reported to be upregulated and inhibit the activation of T cells in ovarian cancer, which would support the immune escape of this cancer (34). Additionally, the plasma concentration of HSPE1 has been found to be higher in breast cancer patients than in controls (35).
The oncogenic role of ARPC5 has been investigated in many cancers, including head and neck squamous cell carcinoma, prostate cancer and multiple myeloma (36-38). ARPC5L, an important paralog of ARPC5, has been proven to directly regulate the antimetastatic effect of SI-83, a promising OS drug, by dephosphorylation (39).
To the best of our knowledge, no previous study has sought to uncover the associations of STAT4, HSPE1, and ARPC5 with the prognosis of OS. In our study, STAT4 and ARPC5 were found to be positive prognostic predictors for OS. However, we detected a negative association between HSPE1 expression and the survival outcome of OS patients. The clinical significance of these genes and their association in OS needs further investigations.
The significance of the signaling pathways has been investigated in OS. Takahashi et al. reported that actin cytoskeleton stimulated the differentiation of adipocytes and repressed the initiation of OS via depolymerization (40). The MAPK signaling pathway has been revealed to be involved in the autophagy and apoptosis of OS cells (41). Yang et al. found that calcium signaling positively regulates the transcription of caveolin-1, a transformation suppressor protein in OS cells (42). In our study, the GSEA results revealed that the MAPK signaling pathway, the regulation of actin cytoskeleton pathway, and the calcium signaling pathway were most enriched in the low-risk group, and the cytosolic DNA sensing pathway was significantly enriched in the high-risk group.
There are multiple studies on prognostic models for OS. For example, Zhang et al. constructed a prognostic model incorporating gene signature and clinical factors for OS patients with recurrence (43). Zhu et al. identified 7 energy metabolism-associated genes to predict the prognosis of OS patients (44). Another 4 immune-related gene prognostic signature was established to predict the survival of OS patients (45). All of these studies only identified gene signatures for survival prediction. Conversely, in the present study, in addition to survival prediction, we first established a gene prognostic signature for recurrence and diagnosis that had excellent predictive performance.
However, the limitations of our study should be noted. First, our signature was based on the retrospective analysis of cases in the TARGET and GEO databases. Thus, further clinical verifications are needed. Second, due to the limitation of the databases, some important clinical prognostic factors, such as surgery, age and grade, were not integrated to analyze the prognostic value of our signature. Third, due to the low incidence of OS, the number of the samples in the databases was relatively small, which may have led to selection bias in this study. Fourth, cell and animal experiments need to be conducted to verify the effects of the genes in our signature on OS.
Conclusions
In summary, 3 genes (STAT4, HSPE1, and ARPC5) were identified and incorporated in an accurate and reliable prognostic signature for the survival, recurrence, and diagnosis of OS by conducting a comprehensive bioinformatics analysis. Our signature is favorable for the individual management of OS patients and could be a promising therapeutic target for OS.
Acknowledgments
Funding: None.
Footnote
Reporting Checklist: The authors have completed the TRIPOD reporting checklist. Available at https://tcr.amegroups.com/article/view/10.21037/tcr-22-1706/rc
Conflicts of Interest: All authors have completed the ICMJE uniform disclosure form (available at https://tcr.amegroups.com/article/view/10.21037/tcr-22-1706/coif). The authors have no conflicts of interest to declare.
Ethical Statement: The authors are accountable for all aspects of the work, including ensuring that any questions related to the accuracy or integrity of any part of the work have been appropriately investigated and resolved. The study was conducted in accordance with the Declaration of Helsinki (as revised in 2013).
Open Access Statement: This is an Open Access article distributed in accordance with the Creative Commons Attribution-NonCommercial-NoDerivs 4.0 International License (CC BY-NC-ND 4.0), which permits the non-commercial replication and distribution of the article with the strict proviso that no changes or edits are made and the original work is properly cited (including links to both the formal publication through the relevant DOI and the license). See: https://creativecommons.org/licenses/by-nc-nd/4.0/.
References
- Hutanu D, Popescu R, Stefanescu H, et al. The Molecular Genetic Expression as a Novel Biomarker in the Evaluation and Monitoring of Patients With Osteosarcoma-Subtype Bone Cancer Disease. Biochem Genet 2017;55:291-9. [Crossref] [PubMed]
- Crompton BD, Goldsby RE, Weinberg VK, et al. Survival after recurrence of osteosarcoma: a 20-year experience at a single institution. Pediatr Blood Cancer 2006;47:255-9. [Crossref] [PubMed]
- Bielack S, Carrle D, Casali PG, et al. Osteosarcoma: ESMO clinical recommendations for diagnosis, treatment and follow-up. Ann Oncol 2009;20:137-9. [Crossref] [PubMed]
- Ottaviani G, Jaffe N. The etiology of osteosarcoma. Cancer Treat Res 2009;152:15-32. [Crossref] [PubMed]
- Morice S, Mullard M, Brion R, et al. The YAP/TEAD Axis as a New Therapeutic Target in Osteosarcoma: Effect of Verteporfin and CA3 on Primary Tumor Growth. Cancers (Basel) 2020;12:3847. [Crossref] [PubMed]
- Zhang L, Lv B, Shi X, et al. High Expression of N-Acetylgalactosaminyl-transferase 1 (GALNT1) Associated with Invasion, Metastasis, and Proliferation in Osteosarcoma. Med Sci Monit 2020;26:e927837. [PubMed]
- Chen H, Pan R, Li H, et al. CHRDL2 promotes osteosarcoma cell proliferation and metastasis through the BMP-9/PI3K/AKT pathway. Cell Biol Int 2021;45:623-32. [Crossref] [PubMed]
- Lamora A, Talbot J, Bougras G, et al. Overexpression of smad7 blocks primary tumor growth and lung metastasis development in osteosarcoma. Clin Cancer Res 2014;20:5097-112. [Crossref] [PubMed]
- Zhu ZQ, Tang JS, Gang D, et al. Antibody microarray profiling of osteosarcoma cell serum for identifying potential biomarkers. Mol Med Rep 2015;12:1157-62. [Crossref] [PubMed]
- Park HR, Cabrini RL, Araujo ES, et al. Expression of ezrin and metastatic tumor antigen in osteosarcomas of the jaw. Tumori 2009;95:81-6. [Crossref] [PubMed]
- Li H, Min D, Zhao H, et al. The Prognostic Role of Ezrin Immunoexpression in Osteosarcoma: A Meta-Analysis of Published Data. PLoS One 2013;8:e64513. [Crossref] [PubMed]
- Wei R, Thanindratarn P, Dean DC, et al. Cyclin E1 is a prognostic biomarker and potential therapeutic target in osteosarcoma. J Orthop Res 2020;38:1952-64. [Crossref] [PubMed]
- Liu Y, Wang Y, Teng Z, et al. Matrix metalloproteinase 9 expression and survival of patients with osteosarcoma: a meta-analysis. Eur J Cancer Care (Engl) 2017; [Crossref] [PubMed]
- Mizobuchi H, García-Castellano JM, Philip S, et al. Hypoxia markers in human osteosarcoma: an exploratory study. Clin Orthop Relat Res 2008;466:2052-9. [Crossref] [PubMed]
- Wang D, Luo M, Kelley MR. Human apurinic endonuclease 1 (APE1) expression and prognostic significance in osteosarcoma: enhanced sensitivity of osteosarcoma to DNA damaging agents using silencing RNA APE1 expression inhibition. Mol Cancer Ther 2004;3:679-86. [Crossref] [PubMed]
- Yang S, Ye Z, Wang Z, et al. High mobility group box 2 modulates the progression of osteosarcoma and is related with poor prognosis. Ann Transl Med 2020;8:1082. [Crossref] [PubMed]
- Xi X, Wu Q, Bao Y, et al. Overexpression of TBL1XR1 confers tumorigenic capability and promotes recurrence of osteosarcoma. Eur J Pharmacol 2019;844:259-67. [Crossref] [PubMed]
- Zhang Y, Zhao H, Xu W, et al. High Expression of PQBP1 and Low Expression of PCK2 are Associated with Metastasis and Recurrence of Osteosarcoma and Unfavorable Survival Outcomes of the Patients. J Cancer 2019;10:2091-101. [Crossref] [PubMed]
- Zamborsky R, Kokavec M, Harsanyi S, et al. Identification of Prognostic and Predictive Osteosarcoma Biomarkers. Med Sci (Basel) 2019;7:28. [Crossref] [PubMed]
- Grambsch PM, Therneau TM. Proportional hazards tests and diagnostics based on weighted residuals. Biometrika 1994;81:515-26. [Crossref]
- Guo L, Fang T, Jiang Y, et al. Identification of immune checkpoint inhibitors and biomarkers among STAT family in stomach adenocarcinoma. Am J Transl Res 2020;12:4977-97. [PubMed]
- Wu HT, Liu J, Li GW, et al. The transcriptional STAT3 is a potential target, whereas transcriptional STAT5A/5B/6 are new biomarkers for prognosis in human breast carcinoma. Oncotarget 2017;8:36279-88. [Crossref] [PubMed]
- Wu Z, Liu J, Hu S, et al. Serine/Threonine Kinase 35, a Target Gene of STAT3, Regulates the Proliferation and Apoptosis of Osteosarcoma Cells. Cell Physiol Biochem 2018;45:808-18. [Crossref] [PubMed]
- Liu Y, Liao S, Bennett S, et al. STAT3 and its targeting inhibitors in osteosarcoma. Cell Prolif 2021;54:e12974. [Crossref] [PubMed]
- Subramaniam D, Angulo P, Ponnurangam S, et al. Suppressing STAT5 signaling affects osteosarcoma growth and stemness. Cell Death Dis 2020;11:149. [Crossref] [PubMed]
- Zhou X, Xia Y, Su J, et al. Down-regulation of miR-141 induced by helicobacter pylori promotes the invasion of gastric cancer by targeting STAT4. Cell Physiol Biochem 2014;33:1003-12. [Crossref] [PubMed]
- Zhao L, Ji G, Le X, et al. An integrated analysis identifies STAT4 as a key regulator of ovarian cancer metastasis. Oncogene 2017;36:3384-96. [Crossref] [PubMed]
- Wang S, Yu L, Shi W, et al. Prognostic roles of signal transducers and activators of transcription family in human breast cancer. Biosci Rep 2018;38:BSR20171175. [Crossref] [PubMed]
- Nishi M, Batsaikhan BE, Yoshikawa K, et al. High STAT4 Expression Indicates Better Disease-free Survival in Patients with Gastric Cancer. Anticancer Res 2017;37:6723-9. [PubMed]
- Li S, Sheng B, Zhao M, et al. The prognostic values of signal transducers activators of transcription family in ovarian cancer. Biosci Rep 2017;37:BSR20170650. [Crossref] [PubMed]
- Doyle SM, Shorter J, Zolkiewski M, et al. Asymmetric deceleration of ClpB or Hsp104 ATPase activity unleashes protein-remodeling activity. Nat Struct Mol Biol 2007;14:114-22. [Crossref] [PubMed]
- Czarnecka AM, Campanella C, Zummo G, et al. Mitochondrial chaperones in cancer: from molecular biology to clinical diagnostics. Cancer Biol Ther 2006;5:714-20. [Crossref] [PubMed]
- Cappello F, David S, Rappa F, et al. The expression of HSP60 and HSP10 in large bowel carcinomas with lymph node metastase. BMC Cancer 2005;5:139. [Crossref] [PubMed]
- Akyol S, Gercel-Taylor C, Reynolds LC, et al. HSP-10 in ovarian cancer: expression and suppression of T-cell signaling. Gynecol Oncol 2006;101:481-6. [Crossref] [PubMed]
- Tsai CH, Chen YT, Chang YH, et al. Systematic verification of bladder cancer-associated tissue protein biomarker candidates in clinical urine specimens. Oncotarget 2018;9:30731-47. [Crossref] [PubMed]
- Xiong T, Luo Z. The Expression of Actin-Related Protein 2/3 Complex Subunit 5 (ARPC5) Expression in Multiple Myeloma and its Prognostic Significance. Med Sci Monit 2018;24:6340-8. [Crossref] [PubMed]
- Kinoshita T, Nohata N, Watanabe-Takano H, et al. Actin-related protein 2/3 complex subunit 5 (ARPC5) contributes to cell migration and invasion and is directly regulated by tumor-suppressive microRNA-133a in head and neck squamous cell carcinoma. Int J Oncol 2012;40:1770-8. [PubMed]
- Liu C, Liu R, Zhang D, et al. MicroRNA-141 suppresses prostate cancer stem cells and metastasis by targeting a cohort of pro-metastasis genes. Nat Commun 2017;8:14270. [Crossref] [PubMed]
- Bernardini G, Laschi M, Serchi T, et al. Proteomics and phosphoproteomics provide insights into the mechanism of action of a novel pyrazolo3,4-dpyrimidine Src inhibitor in human osteosarcoma. Mol Biosyst 2014;10:1305-12. [Crossref] [PubMed]
- Takahashi N, Nobusue H, Shimizu T, et al. ROCK Inhibition Induces Terminal Adipocyte Differentiation and Suppresses Tumorigenesis in Chemoresistant Osteosarcoma Cells. Cancer Res 2019;79:3088-99. [Crossref] [PubMed]
- Li C, Gao H, Feng X, et al. Ginsenoside Rh2 impedes proliferation and migration and induces apoptosis by regulating NF-κB, MAPK, and PI3K/Akt/mTOR signaling pathways in osteosarcoma cells. J Biochem Mol Toxicol 2020;34:e22597. [Crossref] [PubMed]
- Yang XY, Huang CC, Kan QM, et al. Calcium regulates caveolin-1 expression at the transcriptional level. Biochem Biophys Res Commun 2012;426:334-41. [Crossref] [PubMed]
- Zhang M, Liu Y, Kong D. Identifying biomolecules and constructing a prognostic risk prediction model for recurrence in osteosarcoma. J Bone Oncol 2020;26:100331. [Crossref] [PubMed]
- Zhu N, Hou J, Ma G, et al. Co-expression network analysis identifies a gene signature as a predictive biomarker for energy metabolism in osteosarcoma. Cancer Cell Int 2020;20:259. [Crossref] [PubMed]
- Cao M, Zhang J, Xu H, et al. Identification and Development of a Novel 4-Gene Immune-Related Signature to Predict Osteosarcoma Prognosis. Front Mol Biosci 2020;7:608368. [Crossref] [PubMed]
(English Language Editor: L. Huleatt)