A nomogram and risk classification model predicts prognosis in Chinese esophageal squamous cell carcinoma patients
Introduction
Esophageal cancer, predominantly histological type—esophageal squamous cell carcinoma (ESCC), ranked eighth in morbidity and sixth in mortality worldwide in 2020 according to Cancer Today-IARC data. A series of genomic studies of ESCC have been published since 2014 (1-3). Some studies about the relevance of gene mutations in prognosis have been investigated (4,5). However, investigations were confined to single gene mutations, such as ZNF750 and EP300 mutations (6,7). Additionally, other genes were found to be correlated with prognosis (8). Additionally, some studies based on gene expression to make prediction models have been published (9,10). Gene expression is a continuous variable that is commonly used in prediction models. Continuous variables can better obtain the cutoff value. In Professor Cui’s study, they successfully constructed a new 3 autophagy-related gene prognostic model based on their real sequencing data and GSE53624 dataset (11). After rigorous validation, the area under the curve (AUC) value was good, and the prediction model showed potential to improve the ability of individualized prognosis prediction in ESCC (11).
In the clinic, we can easily obtain esophageal biopsies and gene mutation status with the development of the sequencing technique. We cannot determine which gene mutation can better predict prognosis, including overall survival (OS) and progression-free survival (PFS). However, mutation models predicting prognosis have not been established. Thus, we sought to investigate several gene mutations as markers to discriminate prognosis based on mutation state. Notably, the gene mutation status is a categorical variable that is different from continuous variables such as gene expression.
In our previous study, whole-exome sequencing (WES) for 78 patients and targeted sequencing for 316 patients (78 included) were used to depict the landscape of ESCC (12). Detailed gene mutation and authentic clinical data of all sequenced samples were collected. In this study, we aimed to develop and validate a model based on ESCC mutation status to predict the OS and PFS of resected patients with ESCC. The established prognostic nomogram was based on the clinicopathological parameters and gene mutations. We present the following article in accordance with the TRIPOD reporting checklist (available at https://tcr.amegroups.com/article/view/10.21037/tcr-22-915/rc).
Methods
Patients
A total of 316 locally advanced patients with ESCC underwent esophagectomy at Fudan University Shanghai Cancer Center from September 2007 to June 2011. Of these, 312 patients with complete clinical and gene somatic mutation information were enrolled in the study, of which, 88.1% (275 patients) were male. The proportions of cigarette and alcohol consumption were 64.7% (202 patients) and 44.2% (138 patients), respectively. Middle and low differentiation occupied 56.6% (179 patients) and 32.4% (101 patients) in the dataset, respectively. More than half of the patients were stage II, accounting for 57.4% (179 patients), and 38.8% (121 patients) were stage III. Patients were further randomly stratified into a training cohort (156 patients) and validation cohort (156 patients) at a 1:1 ratio. The International Cancer Genome Consortium (ICGC) contains public data, including sequencing and clinical information of various cancers. We chose ESCA-CA, including 263 Chinese ESCC donors, as the external validation set.
Primary tumor tissues and corresponding adjacent non-tumor tissues (located 5 cm from the tumors) were collected from patients with ESCC who received radical operation at Fudan University Shanghai Cancer Center. Surgical tissues were snap frozen in liquid nitrogen immediately and stored at −80 ℃. The clinicopathological characteristics, including age, sex, cigarette consumption, alcohol use history, tumor location, differentiation, and tumor/node/metastasis (TNM) stage, were collected from inpatient medical records. The pathological features were evaluated independently by two separate pathologists according to the TNM staging system of the American Joint Committee on Cancer (AJCC 7th edition). All patients were followed up after primary treatment at intervals that increased from three months to one year until death. The study was conducted in accordance with the Declaration of Helsinki (as revised in 2013). The study was approved by ethics board of Fudan University Shanghai Cancer Center (No. 050432-4-1212B) and informed consent was taken from all the patients.
WES and targeted sequencing, data processing, and mutation calling
The methods have been described previously (12). Genomic DNA was extracted from the frozen tissues. Then, DNA was sheared to short fragments. DNA fragments were end repaired, and an adenylate blocker was added at the 3' ends. Adaptors with barcode sequences were then ligated to both ends of the fragments. E-Gel was used to select DNA fragments of the targeted size. Whole-exome capture was performed using the TruSeq Exome Enrichment kit (Illumina) according to the manufacturer’s protocol. We included 283 “cancer-related genes” in the target enrichment panel. Briefly, these genes included high-priority genes in COSMIC and genes related to drug sensitivity. Targeted gene enrichment was performed with TruSeq Custom Enrichment kits (Illumina).
Read pairs (FASTQ format) were trimmed and filtered with fastq-mcf. The resulting high-quality reads were aligned to the human reference genome (GRCh37/hg19) using Burrows-Wheeler Aligner (BWA 0.7.12). We then used several popular callers, including Muse, MuTect2, SomaticSniper, Radia, and VarScan2, to identify somatic point mutations. Only mutations reported by at least two callers were used in further analyses. Somatic mutations were annotated using Oncotator. MutSigCV (V1.4) was applied to identify significantly mutated genes with default covariate tables. Genes with q (FDR) <0.1 were significantly mutated. The WES and targeted-sequencing data have been deposited in the NCBI Sequence Read Archive (SRA) under Bioproject (accession number: PRJNA399748).
Statistical analysis
The least absolute shrinkage and selection (LASSO) method was used to screen high-dimensional data in the regression analysis with the highest efficiency and least redundancy based on the 283 mutated genes. LASSO is a popular machine learning algorithm that has been extensively utilized in medical studies (13-16). The coefficient of each variable in the regression model was recorded and used to calculate the risk score of each patient. The most significantly mutated genes were identified from the training cohort. The risk score of each patient was calculated via a linear combination of these gene mutation statuses. A multimarker classifier was identified to predict the OS and PFS of patients with ESCC in the training set. Progression was defined as the recurrence of the primary tumor, progression of local and regional lymph nodes, and distant metastasis. LASSO Cox regression model analysis with factors included in the optimal model was conducted by the ‘glmnet’ package using R software (17). The risk score of each patient was thus estimated by the identified model for further analysis.
The optimum cutoff point was defined using X-tile plots based on the balanced number and significant difference in survival between the compared groups overall. X-tile plots provide an assessment of every possible way of dividing a population into different subgroups. The X-tile software allows the user to move a cursor across the grid and provides an “on-the-fly” histogram of the resulting population subsets along with an associated Kaplan-Meier curve. Survival analysis was conducted with SPSS software. Kaplan-Meier curves and the log-rank method were used to compare survival between different groups. Receiver operating characteristic (ROC) analysis was used to investigate the prognostic performance of the gene mutation-based model. Univariate and multivariate Cox regression analyses were conducted to identify independent prognostic predictors. Cox regression coefficients were used to construct a nomogram for predicting the probability of OS and PFS. Calibration plots were also derived based on the regression analysis. Calibration plots that are drawn by observed probabilities against predicted probabilities calculated with the nomogram. The X-axis is the probability of positive prediction by the model, and the Y-axis is the probability of true positive. Decision curve analysis (DCA) was used to assess the clinical utility of the established nomogram. The graph shows the clinical net benefit according to various threshold probabilities. The X-axis is the threshold probability, and the Y-axis is the net benefit, which is the subtraction of the proportion of all patients who are false-positive from the proportion who are true-positive weighted by the relative harm of a false-positive and a false-negative result. The nomogram and calibration plots were constructed using the ‘rms’ R package. Statistical analysis was performed with R software (version 3.2.0). Statistical levels were two-sided, and statistical significance was set at 0.05.
Results
Population characteristics
The study flowchart is illustrated in Figure S1. In our previous study, 78 patients with ESCC received WES. As a validation cohort, another cohort of 316 patients, including 78, underwent targeted sequencing to confirm the WES results. Of 316 patients, 312 patients were chosen for the present study. The training and validation sets were randomized at a ratio of 1:1 using a random table. The clinical characteristics of the current analyzed patients and ICGC donors (public dataset as external validation) are summarized in Table 1. Clinical features of ICGC data mainly included age, sex, TNM stage, and survival time of donors. However, information on cigarette consumption, alcohol consumption history, tumor differentiation, tumor location, and progression status was not supplied.
Table 1
Parameters | OS analysis | PFS analysis | ||||||||||||
---|---|---|---|---|---|---|---|---|---|---|---|---|---|---|
Training set | Validation set | ICGC | Training set | Validation set | ||||||||||
Low | High | Low | High | Low | High | Low | High | Low | High | |||||
Sex | ||||||||||||||
Male | 20 (90.9) | 117 (87.3) | 15 (88.2) | 123 (88.5) | 136 (79.5) | 76 (82.6) | 93 (86.9) | 44 (89.8) | 90 (90.9) | 48 (84.2) | ||||
Female | 2 (9.1) | 17 (12.7) | 2 (11.8) | 16 (11.5) | 35 (20.5) | 16 (17.4) | 14 (13.1) | 5 (10.2) | 9 (9.1) | 9 (15.8) | ||||
Age | ||||||||||||||
≤50 | 2 (9.1) | 15 (11.2) | 2 (11.8) | 16 (11.5) | 19 (11.1) | 12 (13.0) | 13 (12.1) | 4 (8.2) | 12 (12.1) | 6 (10.5) | ||||
51–60 | 12 (54.5) | 58 (43.3) | 10 (58.8) | 61 (43.9) | 75 (43.9) | 38 (41.3) | 42 (39.3) | 28 (57.1) | 46 (46.5) | 25 (43.9) | ||||
>60 | 8 (36.4) | 61 (45.5) | 5 (29.4) | 62 (44.6) | 77 (45.0) | 42 (45.7) | 52 (48.6) | 17 (34.7) | 41 (41.4) | 26 (45.6) | ||||
Smoking | ||||||||||||||
Yes | 13 (59.1) | 89 (66.4) | 12 (70.6) | 88 (63.3) | 66 (61.7) | 36 (73.5) | 64 (64.6) | 36 (63.2) | ||||||
No | 9 (40.9) | 45 (33.6) | 5 (29.4) | 51 (36.7) | 41 (38.3) | 13 (26.5) | 35 (36.4) | 21 (36.8) | ||||||
Alcohol | ||||||||||||||
Yes | 13 (59.1) | 58 (43.3) | 9 (52.9) | 58 (41.7) | 46 (43.0) | 25 (51.0) | 45 (45.5) | 22 (38.6) | ||||||
No | 9 (40.1) | 76 (56.7) | 8 (47.1) | 81 (58.3) | 61 (57.0) | 24 (49.0) | 54 (54.5) | 35 (61.4) | ||||||
Family history | ||||||||||||||
Yes | 2 (9.1) | 25 (18.7) | 4 (23.5) | 19 (13.7) | 20 (18.7) | 7 (14.3) | 21 (21.2) | 52 (91.2) | ||||||
No | 20 (90.9) | 109 (81.3) | 11 (64.7) | 112 (80.6) | 87 (81.3) | 42 (85.7) | 78 (78.8) | 5 (8.8) | ||||||
T stage | ||||||||||||||
T1 | 2 (9.1) | 5 (3.7) | 1 (5.9) | 5 (3.6) | 12 (7.0) | 2 (2.2) | 5 (4.7) | 2 (4.1) | 6 (6.1) | 0 (0) | ||||
T2 | 11 (50.0) | 55 (41.0) | 9 (52.9) | 59 (42.4) | 49 (28.7) | 10 (10.9) | 44 (41.1) | 22 (44.9) | 46 (46.5) | 22 (38.6) | ||||
T3 | 9 (40.9) | 74 (55.2) | 7 (41.2) | 75 (54.0) | 110 (64.3) | 80 (87.0) | 58 (54.2) | 25 (51.0) | 47 (47.5) | 35 (61.4) | ||||
N stage | ||||||||||||||
N0 | 9 (40.9) | 45 (33.6) | 10 (58.8) | 50 (36.0) | 121 (70.8) | 29 (31.5) | 39 (36.4) | 15 (30.6) | 42 (42.4) | 18 (31.6) | ||||
N1 | 11 (50.0) | 55 (41.0) | 6 (35.3) | 59 (42.4) | 31 (18.1) | 31 (33.7) | 44 (41.1) | 22 (44.9) | 42 (42.4) | 23 (40.4) | ||||
N2 | 2 (9.1) | 24 (17.9) | 1 (5.9) | 19 (13.7) | 17 (9.9) | 27 (29.3) | 18 (16.8) | 8 (16.3) | 10 (10.1) | 10 (17.5) | ||||
N3 | 0 (0) | 10 (7.5) | 0 (0) | 11 (7.9) | 2 (1.2) | 5 (5.4) | 6 (5.6) | 4 (8.2) | 5 (5.1) | 6 (10.5) | ||||
Differentiation | ||||||||||||||
High | 1 (4.5) | 19 (14.2) | 4 (23.5) | 8 (5.8) | 10 (9.3) | 10 (20.4) | 7 (7.1) | 5 (8.8) | ||||||
Middle | 15 (68.2) | 72 (53.7) | 10 (58.8) | 82 (59.0) | 62 (57.9) | 25 (51.0) | 62 (62.6) | 30 (52.6) | ||||||
Low | 6 (27.3) | 43 (32.1) | 3 (17.6) | 49 (35.3) | 35 (32.7) | 14 (28.6) | 30 (30.3) | 22 (38.6) | ||||||
Site/location (7th) | ||||||||||||||
Upper thoracic | 7 (31.8) | 21 (15.7) | 4 (23.5) | 34 (24.5) | 22 (20.6) | 6 (12.2) | 26 (26.3) | 12 (21.1) | ||||||
Middle thoracic | 11 (50.0) | 85 (63.4) | 11 (64.7) | 79 (56.8) | 63 (58.9) | 33 (67.3) | 52 (52.5) | 38 (66.7) | ||||||
Low thoracic | 4 (18.2) | 28 (20.9) | 2 (11.8) | 26 (18.7) | 22 (20.6) | 10 (20.4) | 21 (21.2) | 7 (12.3) | ||||||
TNM stage | ||||||||||||||
I | 2 (9.1) | 6 (4.5) | 2 (11.8) | 2 (1.4) | 69 (40.4) | 4 (4.3) | 4 (3.7) | 4 (8.2) | 3 (3.0) | 1 (1.8) | ||||
II | 14 (63.6) | 71 (53.0) | 12 (70.6) | 82 (59.0) | 59 (34.5) | 24 (26.1) | 61 (57.0) | 24 (49.0) | 67 (67.7) | 27 (47.4) | ||||
III | 6 (27.3) | 57 (42.5) | 3 (17.6) | 55 (39.6) | 43 (25.1) | 64 (69.6) | 42 (39.3) | 21 (42.9) | 29 (29.3) | 29 (50.9) | ||||
Nerve invasion | ||||||||||||||
Yes | 1 (4.5) | 27 (20.1) | 1 (5.9) | 20 (14.4) | 21 (19.6) | 7 (14.3) | 13 (13.1) | 8 (14.0) | ||||||
No | 21 (95.5) | 107 (79.9) | 16 (94.1) | 119 (85.6) | 86 (80.4) | 42 (85.7) | 86 (86.9) | 49 (86.0) | ||||||
Vessel invasion | ||||||||||||||
Yes | 2 (9.1) | 26 (19.4) | 1 (5.9) | 30 (21.6) | 18 (16.8) | 10 (20.4) | 18 (18.2) | 13 (22.8) | ||||||
No | 20 (90.9) | 108 (80.6) | 16 (94.1) | 109 (78.4) | 89 (83.2) | 39 (79.6) | 81 (81.8) | 44 (77.2) |
Data are shown as n (%). OS, overall survival; PFS, progression free survival; ICGC, International Cancer Genome Consortium.
There were 80 events (deaths) over a median follow-up time of 35.5 months in the training set and 88 events in the validation set.
Development of the gene mutation-based prognostic model
We identified potential predictive prognostic markers, including OS and PFS, using the LASSO Cox regression model (Figure S2). Regarding OS, nine prognostic markers (ADCY8, ALK, ARID1A, CDK8, DICER1, EPC1, ERBB2, MED12, TSC1) in the training cohort were identified through regression analysis. These gene mutation types and frequencies are summarized in Table S1. According to the mutation status of the nine genes, we derived a formula to calculate risk score (Table S1). In this formula, wild type equals 0, and mutated type equals 1. The optimum cutoff value of the nine-gene mutation model was defined as −0.13 by the X-tile plot approach (Table S2).
Using this formula, patients in the training set were classified into low- and high-risk subgroups. Patients with a risk score of −0.13 or higher were divided into the high-risk group, whereas those with a risk score lower than −0.13 were enrolled in the low-risk group. According to the risk score, 156 patients in the training cohort were further stratified into a high-risk group (135 patients, 86.5%) and a low-risk group (21 patients, 13.5%). Patients with lower risk scores have better 5-year OS. The 5-year OS was 41.0% in the high-risk group and 67.5% in the low-risk group (P=0.02; Figure 1A). The difference was the same in both the whole cohort and the validation set. In the validation cohort, we classified patients into a high-risk group (139 patients, 89.1%) and low-risk group (17 patients, 10.9%). The 5-year OS was 38.8% for the high-risk group and 65.5% for the low-risk group (P=0.04; Figure 1B). In the whole cohort, the 5-year OS was 40% in the high-risk group (274 patients) and 56.4% in the low-risk group (38 patients) (P=0.004; Figure 1C).
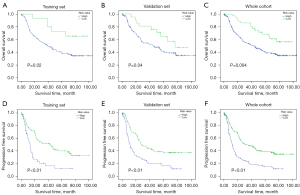
The survival difference was verified repeatedly in ICGC data (Figure S3A). The survival advantage was significant in the low-risk group (171 patients) compared to the high-risk group (92 patients, P<0.01). Additionally, a consistent result was verified in The Cancer Genome Atlas (TCGA) data (Figure S3B, P<0.01).
Regarding PFS, 21 gene mutation-based models were developed (Figure S2B). These gene mutation types, frequencies, and risk score formulas are summarized in Table S1. Then, we sought to set up different risk score groups according to the risk score. The cutoff risk value of the risk score was defined as 0.04 through X-tile (Table S2). There were 207 patients in the low-risk group with a risk value <0.04 (range, −0.91 to 0). A total of 105 patients were divided into a high-risk group with a risk value >0.04. Additionally, the training and validation sets were randomized at a ratio of 1:1. The patient characteristics are shown in Table 1. In the training set, the 3-year PFS was 16.2% in the high-risk group (49 patients) and 52.1% in the low-risk group (107 patients). PFS was significantly better in the low-risk group than in the high-risk group in the training set (P<0.01, Figure 1D). The result was identified in both the validation set and the whole cohort. In the validation set, the 3-year PFS was 21.9% in the high-risk group (57 patients) and 43.8% in the low-risk group (99 patients) (P<0.01, Figure 1E). In the whole cohort, the 3-year PFS was 19.7% in the high-risk group (105 patients) and 48.4% in the low-risk group (207 patients) (P<0.01, Figure 1F).
Genes expression in the prediction model
Seventy-four paired ESCC tumor and adjacent normal tissues were subjected to RNA-Seq. In the OS prediction model, expression of ALK, CDK8, EPC1, and ERBB2 was significantly decreased in tumor tissue (P<0.05). The expression differences of the other four genes were not significant. In the PFS prediction model, significantly increased expression of MAP3K13, TSHR, PMS1, MSH2, PMS2, AP3B2, PTCH1, FANCF, and PIK3CA was observed in tumor tissue in comparison to normal tissue, while significantly decreased expression of CS, EPC1, LAMA2, ALK, ERCC5, and PDZRN4 was observed in tumor tissue. Other gene expression was not significant. The expression of all genes included in the prediction model is summarized in Table S3. The top two genes with upregulated expression in tumors were MSH2 and AP3B2, and the top two genes with downregulated expression were PDZRN4 and LAMA2 (Figure S4).
Predictive value of the established model
To determine whether the prognostic prediction model was an independent variable in comparison with other clinicopathological features, univariate and multivariate Cox regression analyses were performed. In univariate analysis, stage, risk score, sex, differentiation, and vessel invasion were found to be influencing factors of OS, while other clinicopathological factors showed no statistically significant differences (Figure 2A). Multivariate analysis showed that only stage and mutation-based classifiers remained independent predictors of OS (Figure 2B). Moreover, the time-dependent ROC curve showed that the area under the ROC curve was 0.599, which was similar to that of the TNM stage (AUC =0.654). Furthermore, the combination of the gene mutation-based prediction model and TNM stage showed better performance for predicting OS than TNM stage alone (P=0.00013). Thus, the gene mutation-based model could add prognostic value to TNM stage in predicting OS (Figure S5A). In ICGC data, the gene-mutation model was better, with an area under the ROC of 0.654 in the prediction of OS. In combination with stage, the prediction efficiency reached 0.672 (Figure S5B).
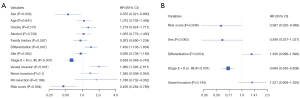
Only stage, risk score, and vessel invasion were found to be significant in PFS univariate analysis (P<0.05) (Figure 3A). In multivariate analysis, stage and risk score were independent factors of PFS (P<0.01, Figure 3B). In ROC curve analysis, the AUC of the gene mutation-based model was 0.590, and the TNM stage value was 0.654. Combined with the risk score and TNM stage analysis, a better prediction of PFS was observed with AUC =0.694 (Figure S5C).
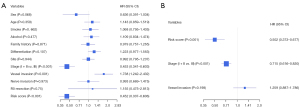
Nomogram construction and its clinical utility
To establish an applicable method to predict OS and PFS probabilities, we constructed a nomogram plot integrating gene mutations and multiple clinicopathological features. The nomogram was generated based on multivariate analysis. The independent predictors of multiple analyses, including sex, vessel invasion, differentiation, risk score, and stage, were included in the prediction model for OS (Figure 4A). A nomogram model based on the ICGC database, including stage and risk score, is shown in Figure 4B. The independent predictors of multiple analyses, including vessel invasion, risk score, and stage, were included in the prediction model for PFS (Figure 4C). In all analyses, the risk model showed better predictive value and high consistency.
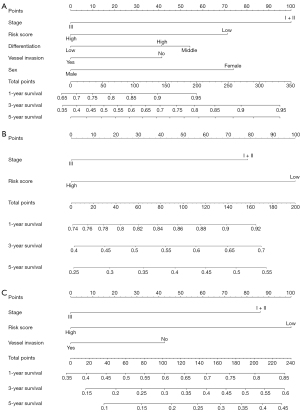
The calibration curve was also investigated and showed favorable consistency between the predicted OS and PFS and the actual observation (Figure 5A,5B). In survival analysis of external validation set—ICGC data, good consistency was also shown between prediction and actual events (Figure 5C). DCA was used to assess the potential clinical application of the mutation-based model by quantifying the net benefits. In the current analysis, DCA exhibited satisfactory positive net benefits of the nomogram at the threshold probabilities for 5-year OS and PFS (Figure 6A,6B). The benefit was verified repeatedly in ICGC validation (Figure 6C). These results indicated that our 9-gene-based and 21-gene-based prediction models performed well and were capable of distinguishing different patients with ESCC with high or low risk of survival and PFS.
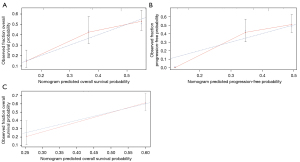

Discussion
Previous studies have demonstrated the genetic landscape and many different single prognostic biomarkers for ESCC (2,3,6). TNM stage is mainly used to assess the prognosis of ESCC; however, more accurate prediction models integrating additional genomic and clinical parameters are needed. Some risk score systems have been estimated for ESCC, such as the 6-IHC marker-based classifier model (18). However, it was based on IHC markers, not gene mutation status. Another classifier was a single gene as a prognostic biomarker (19). Thus, we sought to develop a postoperative prediction model that would practically promote prognostic value in comparison with a single biomarker.
In the present study, gene mutation information came from WES and target sequencing samples to maximize model accuracy. Additionally, a large sample size of 316 patients with ESCC were enrolled for analysis. Using the LASSO method, we established a 9-gene-based and 21-gene-based prediction model for ESCC prognosis. This prognostic model was successfully validated in either the internal or external validation cohorts, which indicated excellent power to classify patients with ESCC into different risk subgroups.
Based on this prognostic model, patients with ESCC could be divided into high- and low-risk subgroups. In the current analysis, we observed that the high-risk subgroup had poor clinical outcomes, either OS or PFS, compared to those of the low-risk subgroup. Therefore, this prediction model may help identify patients with ESCC with poor prognosis and make appropriate clinical follow-up plans to prevent disease recurrence and progression.
In OS and PFS prediction models, CDK8 occurred repeatedly and was identified as correlated with suppression of ESCC (20). CDK8 regulates several transcription factors implicated in cancer (21,22). Regarding ARID1A, immunohistochemistry performed on an independent archival cohort demonstrated that ARID1A protein loss decreased from normal squamous epithelium to EAC. Enhanced cell growth, proliferation, and invasion were observed upon ARID1A knockdown in EAC cells (23). ERBB2 amplification variants mainly give rise to attention in breast cancer and head and neck tumors (24). Mutation is also correlated with worse prognosis in cancers (25). ADCY8 catalyzes the formation of cyclic AMP from ATP. Increased expression of ADCY8 plays an important role in tumor differentiation (26). TSC1 is a tumor suppressor gene that encodes the growth inhibitory protein hamartin. TSC1 mutation reduces drug sensitivity and selectivity in bladder cancer (27).
The conventional prognostic factors of patients with ESCC who undergo surgical resection include TNM stage, differentiation, and metastatic lymph node status and number (7). To assess whether the gene mutation-based model can be an independent factor of prognosis, we performed univariate and multivariate Cox regression analyses. In the entire cohort, the prediction model displayed an independent correlation with OS and PFS after adjusting for sex, TNM stage, tumor differentiation, and vessel invasion.
To evaluate the prediction accuracy of the gene mutation-based model, we performed time-dependent ROC analysis and calculated AUCs at different cutoff times. The ROC receiver either in OS or PSF analysis was not above 0.75 or not satisfactory, as in other studies (28,29). The reason may be that the model is based on gene mutation status, which is a categorical variable. The wild type is defined as 0, and the mutant status as 1. The risk score mode based on binary variables may not be more satisfactory than that based on continuous variables. Additionally, the AUC analysis focused mainly on the predictive accuracy of the model. In AUC analysis, false-positives and false negatives will be encountered. To find a way to maximize the net benefit in clinical utility, DCA is adopted. DCA incorporating doctors’ or patients’ preferences is a statistical method that can provide advice on whether patients could theoretically benefit from the chosen treatment (30). In the current study, favorable consistency was observed between the prediction model and actual observations in the calibration plot, which proved that the developed nomogram model was repeatable and reliable.
To the best of our knowledge, the present nomogram is the first one based on sequenced gene mutation status for predicting the survival of patients with ESCC after esophagectomy. Based on the scoring system, surgeons could conduct an individualized prediction of OS and PFS for different patients. Screening patients at high risk for poor prognosis might help to make rational physical examinations and follow-up periods.
Nevertheless, there are some limitations of the current study. This is a retrospective analysis which limits the established nomogram. The gene mutation status was based on WES. It is possible that some gene mutation statuses were not acquired through panel sequencing in the clinic. The gene mutation-based model failed to incorporate some high-frequency genes (e.g., TP53 mutation and NFE2L2 mutation). In future research, we will select the genes with a high mutation rate in the risk model and combine them with other genes with a high mutation rate (more than 15%) to construct a smaller gene panel that can be sequenced more easily in the clinic. Additionally, clinical information of the external validation set from ICGC was not complete. Further efforts to collect nationwide multicenter clinical data would increase the applicability of the prediction model.
In conclusion, we developed and validated the first nomogram model that integrated gene mutation status and clinical features, which could be helpful for better predicting the prognosis of resected patients with ESCC. With this prediction model, patients were stratified into different subgroups that would be given individualized follow-up options.
Acknowledgments
We would like to thank AJE for polishing the manuscript.
Funding: This study was financially supported by
Footnote
Reporting Checklist: The authors have completed the TRIPOD reporting checklist. Available at https://tcr.amegroups.com/article/view/10.21037/tcr-22-915/rc
Data Sharing Statement: Available at https://tcr.amegroups.com/article/view/10.21037/tcr-22-915/dss
Conflicts of Interest: All authors have completed the ICMJE uniform disclosure form (available at https://tcr.amegroups.com/article/view/10.21037/tcr-22-915/coif). The authors have no conflicts of interest to declare.
Ethical Statement: The authors are accountable for all aspects of the work in ensuring that questions related to the accuracy or integrity of any part of the work are appropriately investigated and resolved. The study was conducted in accordance with the Declaration of Helsinki (as revised in 2013). The study was approved by ethics board of Fudan University Shanghai Cancer Center (No. 050432-4-1212B) and informed consent was taken from all the patients.
Open Access Statement: This is an Open Access article distributed in accordance with the Creative Commons Attribution-NonCommercial-NoDerivs 4.0 International License (CC BY-NC-ND 4.0), which permits the non-commercial replication and distribution of the article with the strict proviso that no changes or edits are made and the original work is properly cited (including links to both the formal publication through the relevant DOI and the license). See: https://creativecommons.org/licenses/by-nc-nd/4.0/.
References
- Gao YB, Chen ZL, Li JG, et al. Genetic landscape of esophageal squamous cell carcinoma. Nat Genet 2014;46:1097-102. [Crossref] [PubMed]
- Lin DC, Hao JJ, Nagata Y, et al. Genomic and molecular characterization of esophageal squamous cell carcinoma. Nat Genet 2014;46:467-73. [Crossref] [PubMed]
- Qin HD, Liao XY, Chen YB, et al. Genomic Characterization of Esophageal Squamous Cell Carcinoma Reveals Critical Genes Underlying Tumorigenesis and Poor Prognosis. Am J Hum Genet 2016;98:709-27. [Crossref] [PubMed]
- Shigaki H, Baba Y, Watanabe M, et al. PIK3CA mutation is associated with a favorable prognosis among patients with curatively resected esophageal squamous cell carcinoma. Clin Cancer Res 2013;19:2451-9. [Crossref] [PubMed]
- Hu N, Kadota M, Liu H, et al. Genomic Landscape of Somatic Alterations in Esophageal Squamous Cell Carcinoma and Gastric Cancer. Cancer Res 2016;76:1714-23. [Crossref] [PubMed]
- Dai W, Ko JMY, Choi SSA, et al. Whole-exome sequencing reveals critical genes underlying metastasis in oesophageal squamous cell carcinoma. J Pathol 2017;242:500-10. [Crossref] [PubMed]
- Bi Y, Kong P, Zhang L, et al. EP300 as an oncogene correlates with poor prognosis in esophageal squamous carcinoma. J Cancer 2019;10:5413-26. [Crossref] [PubMed]
- Sawada G, Niida A, Uchi R, et al. Genomic Landscape of Esophageal Squamous Cell Carcinoma in a Japanese Population. Gastroenterology 2016;150:1171-82. [Crossref] [PubMed]
- Ueno H, Hirai T, Nishimoto N, et al. Prediction of lymph node metastasis by p53, p21(Waf1), and PCNA expression in esophageal cancer patients. J Exp Clin Cancer Res 2003;22:239-45. [PubMed]
- Kihara C, Tsunoda T, Tanaka T, et al. Prediction of sensitivity of esophageal tumors to adjuvant chemotherapy by cDNA microarray analysis of gene-expression profiles. Cancer Res 2001;61:6474-9. [PubMed]
- Cui H, Weng Y, Ding N, et al. Autophagy-Related Three-Gene Prognostic Signature for Predicting Survival in Esophageal Squamous Cell Carcinoma. Front Oncol 2021;11:650891. [Crossref] [PubMed]
- Deng J, Chen H, Zhou D, et al. Comparative genomic analysis of esophageal squamous cell carcinoma between Asian and Caucasian patient populations. Nat Commun 2017;8:1533. [Crossref] [PubMed]
- Liu Z, Guo C, Li J, et al. Somatic mutations in homologous recombination pathway predict favourable prognosis after immunotherapy across multiple cancer types. Clin Transl Med 2021;11:e619. [Crossref] [PubMed]
- Liu Z, Lu T, Li J, et al. Development and clinical validation of a novel six-gene signature for accurately predicting the recurrence risk of patients with stage II/III colorectal cancer. Cancer Cell Int 2021;21:359. [Crossref] [PubMed]
- Liu Z, Guo C, Dang Q, et al. Integrative analysis from multi-center studies identities a consensus machine learning-derived lncRNA signature for stage II/III colorectal cancer. EBioMedicine 2022;75:103750. [Crossref] [PubMed]
- Liu Z, Lu T, Li J, et al. Clinical Significance and Inflammatory Landscape of aNovel Recurrence-Associated Immune Signature in Stage II/III Colorectal Cancer. Front Immunol 2021;12:702594. [Crossref] [PubMed]
- Friedman J, Hastie T, Tibshirani R. Regularization Paths for Generalized Linear Models via Coordinate Descent. J Stat Softw 2010;33:1-22. [Crossref] [PubMed]
- Meng J, Zhang J, Xiu Y, et al. Prognostic value of an immunohistochemical signature in patients with esophageal squamous cell carcinoma undergoing radical esophagectomy. Mol Oncol 2018;12:196-207. [Crossref] [PubMed]
- Kawasaki Y, Okumura H, Uchikado Y, et al. Nrf2 is useful for predicting the effect of chemoradiation therapy on esophageal squamous cell carcinoma. Ann Surg Oncol 2014;21:2347-52. [Crossref] [PubMed]
- Chattopadhyay I, Singh A, Phukan R, et al. Genome-wide analysis of chromosomal alterations in patients with esophageal squamous cell carcinoma exposed to tobacco and betel quid from high-risk area in India. Mutat Res 2010;696:130-8. [Crossref] [PubMed]
- Wei R, Kong L, Xiao Y, et al. CDK8 regulates the angiogenesis of pancreatic cancer cells in part via the CDK8-β-catenin-KLF2 signal axis. Exp Cell Res 2018;369:304-15. [Crossref] [PubMed]
- siRNA-mediated silencing of CDK8 inhibits proliferation and growth in breast cancer cells Retraction. Int J Clin Exp Pathol 2018;11:1836. [PubMed]
- Streppel MM, Lata S, DelaBastide M, et al. Next-generation sequencing of endoscopic biopsies identifies ARID1A as a tumor-suppressor gene in Barrett's esophagus. Oncogene 2014;33:347-57. [Crossref] [PubMed]
- Brase JC, Schmidt M, Fischbach T, et al. ERBB2 and TOP2A in breast cancer: a comprehensive analysis of gene amplification, RNA levels, and protein expression and their influence on prognosis and prediction. Clin Cancer Res 2010;16:2391-401. [Crossref] [PubMed]
- Ping Z, Siegal GP, Harada S, et al. ERBB2 mutation is associated with a worse prognosis in patients with CDH1 altered invasive lobular cancer of the breast. Oncotarget 2016;7:80655-63. [Crossref] [PubMed]
- Orchel J, Witek L, Kimsa M, et al. Expression patterns of kinin-dependent genes in endometrial cancer. Int J Gynecol Cancer 2012;22:937-44. [Crossref] [PubMed]
- Woodford MR, Hughes M, Sager RA, et al. Mutation of the co-chaperone Tsc1 in bladder cancer diminishes Hsp90 acetylation and reduces drug sensitivity and selectivity. Oncotarget 2019;10:5824-34. [Crossref] [PubMed]
- Li Y, Ge D, Gu J, et al. A large cohort study identifying a novel prognosis prediction model for lung adenocarcinoma through machine learning strategies. BMC Cancer 2019;19:886. [Crossref] [PubMed]
- Liang W, Zhang L, Jiang G, et al. Development and validation of a nomogram for predicting survival in patients with resected non-small-cell lung cancer. J Clin Oncol 2015;33:861-9. [Crossref] [PubMed]
- Vickers AJ, Elkin EB. Decision curve analysis: a novel method for evaluating prediction models. Med Decis Making 2006;26:565-74. [Crossref] [PubMed]