Construction and validation of prognostic prediction established on N6-methyladenosine related genes in cervical squamous cell carcinoma
Introduction
Cervical cancer (CESC) is the second leading reason for cancer death in women aged 20 to 39 years, which took the lives of 4,138 women in 2018; this means the equal of 11 women per day, half of whom were aged ≤58 years at death (1). The current treatment for CESC includes radiation therapy (RT) or concurrent chemoradiation therapy (CRT), which could harvest cures in 80% of women with the early-stage situation (stages I–II) and 60% of women with stage III situation (2). Recurrent, progressive, and pathologic process CESC carries a poor prognosis limited treatment progress (3). About 1/3 of these patients responded to systemic therapy, bringing the estimated overall survival (OS) approximately 1 year (4). Thus, profoundly determining the molecular mechanisms of CESC development would benefit numerous CESC patients.
RNA modifications play an essential role in linking individual development and cell fate decision as critical regulators in driving tumourigenesis, long-term survival, and therapy resistance (5). In cellular RNAs, researchers have found over one hundred styles of chemical modifications. The most plethoric internal transformation is N6-methyladenosine (m6A), and classification of proteins that writing, reading, and erasing this, and different characters have unconcealed functions for RNA alteration in closely all facet of the RNA lifecycle, in addition as in various cellular, biological process, and cancer development (6,7). Three regulators regulate m6A: writers, readers, and erasers, frequently occurring close stop codons and 3' untranslated regions (3'UTRs) (8). By modulating the fate of targeted RNA, m6A also can affect the tumor-associated immune cell infiltration and the efficacy of immunotherapy (9-12). Many cancers, including CESC, have been regulated through upregulating or down-regulating m6A RNA methylation regulators (13-17). Some researchers have reported the prognostic prediction of m6A-related genes in tumors (18,19). Nevertheless, m6A modification together with its regulators may play the exact opposite role in different tumor types and predictive modeling of m6A RNA methylation genes in CESC is still imperfect (20-22). We hope to discover the correlation between CESC’s prognosis and tumor immune microenvironment and m6A-related genes.
This research aimed to find the differential m6A RNA methylation regulators in CESC by analyzing transcriptome data from The Cancer Genome Atlas (TCGA) and Gene Expression Omnibus (GEO) databases. We developed two subtypes using Consensus clustering and found that different subtypes would predict clinical outcomes and distinguish immune microenvironment, immune score estimate, and PD-L1 expression. Meanwhile, we generated a five-gene predictive model by Least Absolute Shrinkage and Selection Operator (LASSO) Cox analysis and effectively accomplished and confirmed it in the TCGA and GEO validation cohorts. We discovered the relationship between the predictive model and the infiltration of immune cells in CESC. Finally, recommend a successful predictive model that could be a hypothetical model to predict survival outcomes with high integrity and trustworthiness for CESC patients. We present the following article in accordance with the TRIPOD reporting checklist (available at https://tcr.amegroups.com/article/view/10.21037/tcr-22-881/rc).
Methods
Data acquisition
We downloaded transcriptome data and clinical characteristics from the TCGA database (https://cancergenome.nih.gov/). The platform was Illumina HiSeq 2000 RNA Sequencing. We normalized the gene expression level data by log2(FPKM+1) and performed analysis consistent with the publication guidelines of the TCGA. Once corresponding with the clinical information of samples, the standard control samples and tumor samples with clinical prognostic details were retained, including 294 samples (3 control and 291 tumor samples). We used the 294 samples as the training dataset.
At the same time, the microarray dataset GSE44001 (14) and GSE7803 (23) was selected and downloaded from the GEO database. They aimed to validate the prediction potentials for cancer recurrence among the gene set prognostic and clinical predictive models to see whether the quantitative genetic method will have an essential prophetic role in primary CESC patients. The detection platform was Illumina HumanHT-12 WG-DASL V4.0 R2 expression beadchip. We apply the 300 patients as the validation cohort for this study.
The study was conducted in accordance with the Declaration of Helsinki (as revised in 2013).
Identification of differentially expressed m6A genes
We calculated and extracted the expression of m6A related genes from the TCGA and GEO databases, including methylated genes (METTL3, METTL14, METTL15, RBM15, RBM15B, WTAP, VIRMA, KIAA1429, and ZC3H13), demethylated genes (FTO and ALKBH5), and m6A binding and effector proteins (YTHDC1, YTHDC2, YTHDF1, YTHDF2, YTHDF3, RBMX, IGF2BP1, IGF2BP2, IGF2BP3, HNRNPA2B1, and HNRNPC). Then the differences in expression levels of the m6A gene in TCGA tumor samples and control samples were compared using the R 3.6.1 intergroup t-test. And the screening threshold was defined as the significant P value <0.05, and we retain the significantly different m6A related genes. We used heatmap version 1.0.8 (24) to perform hierarchical clustering based on the centered Pearson correlation algorithm, which shows the differential expression of related genes.
Subtype analysis based on DE m6A genes
We used ConsensusClusterPlus package version 1.54.0 (25) in R 3.6.1 to analyze tumor subtypes based on the significantly differentially expressed (DE) m6A genes. Based on the CESC subtypes, we used the Kaplan-Meier curve method in R3.6.1 survival pack version 2.41-1 (26) to evaluate the correlation of survival prognosis among different subtypes. Then we statistically analyzed the clinical information of different subtypes and compared the distribution by chi-square test.
Examination of the immune microenvironment in different subtypes
We used CIBERSORT (27) to calculate the proportion of immune cells in the tumor samples. Then the intergroup t-test function in R 3.6.1 was handled to evaluate the differences in the ratio of different subtypes of immune cells.
The immune score was premeditated using an estimate package from R3.6.1 (28). Then the distribution differences of immune scores among different subtypes were compared using the intergroup t-test function in R3.6.1. The subtype with a high immune score was distinguished as high tumor immune microenvironment (high TIME). The subtype with a low immune score was characterized as low tumor immune microenvironment (low TIME). Then based on the gene expression levels detected in all the samples, KEGG signaling pathways connected with different TIME were selected using gene set enrichment analysis (GSEA) (29).
Additionally, we extracted the expression level of the PD-L1 gene in tumor samples. The expression level of PD-L1 in tumor and control groups and different subtypes were compared using the R 3.6.1 intergroup t-test.
Construction of predictive risk prediction model
Based on the significantly DE m6A genes, we performed LASSO regression analysis with the lars package version 1.2 (30) in R3.6.1 to screen the optimized m6A gene combination. Then, according to the LASSO coefficient of each element in the optimized gene combination and the expression level in the TCGA dataset, we constructed the risk score (RS) model as follows:
Risk score (RS) = ∑Coef genes ×Exp genes
Where Coef genes represent the LASSO coefficient of the target gene, and Exp genes represent the expression level in the TCGA dataset.
Validation RS prognostic risk prediction model
We used the RS model to calculate the RS values in the TCGA training and GEO validation cohorts. And we divided the samples into high and low risks according to the RS median value.
We used The Kaplan-Meier curve method of survival package version 2.41-1 (31) in R3.6.1 to evaluate the correlation and prognosis between different groups. Then, we used univariate and multivariate Cox regression analysis in the TCGA dataset to investigate whether RS was an independent predictor. Finally, the intergroup t-test in R3.6.1 was used to investigate the differences in the distribution of RS values in different target clinical factors.
Correlation between prognosis characteristics and immunity based on DE m6A genes
Relied on the expression level of TCGA tumor samples, we applied the online tools Tumor IMmune Estimation Resource (TIMER) (32) to analyze the related immune cells.
Statistical analysis
We applied the chi-square test to compare the clinicopathological features. We check the difference by using The Student’s t-test (two-tailed). Univariate and multivariate Cox regression analyses are accustomed to establishing the independent predictor for CESC. The OS was compared by Kaplan-Meier method and log-rank test. We perform Statistical analysis using GraphPad Prism 8 software (GraphPad Software, Inc.). All statistical tests were two-sided. Asterisks denote statistical significance. The analysis flow chart is shown in Figure 1.
Results
Comparative analysis of m6A gene expression level
We identified 8 DE m6A genes between CESC and normal tissues in the TCGA database. The different expressions of these genes between CESC and normal tissues were displayed by a heatmap (Figure 2A). The mRNA expressions of YTHDF1-2, HNRNPA2B1, RBM15, IGF2BP1-3 were significantly increased, and FTO was down-regulated in CESC compared with normal tissues (Figure 2B). We found no significant difference in other m6A regulators.
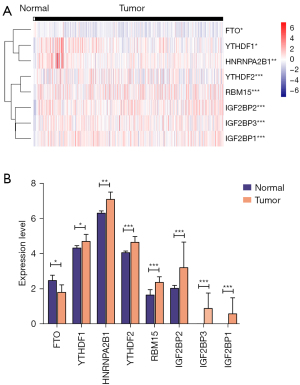
Consensus clustering identified two subtypes based on DE m6A genes
Based on the 8 DE m6A genes, we divided the CESC in the TCGA database into several clusters. We calculate the difference between clusters with the clustering index “k” increased from 2 to 9, And found k=2 was the best clustering index to obtain the most significant difference (Figure 3A,3B). CESC would be divided into two subtypes, subtypes 1 and 2 (Figure 3C). Subtypes 1 and 2 separately include 113 and 178 tumor tissues.
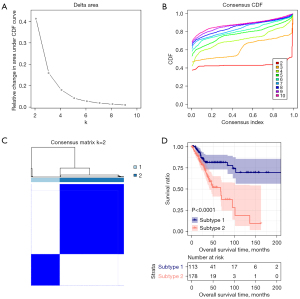
Meanwhile, we found significantly different survival prognosis information between the two subtypes by Kaplan-Meier survival analysis (Figure 3D). The OS of subtype 1 was markedly more prolonged than subtype 2, with significant differences in age and recurrence and no other clinical information. The clinical information distribution of tissues in different subtypes was displayed in Table 1.
Table 1
Characteristics total cases | N of case 291 | Subtype | P value | |
---|---|---|---|---|
Subtype 1 (N=113) | Subtype 2 (N=178) | |||
Age (years) | 4.35E-02 | |||
≤60 | 237 | 85 | 152 | |
>60 | 54 | 28 | 26 | |
Pathologic M | 3.14E-01 | |||
M0 | 107 | 42 | 65 | |
M1 | 10 | 6 | 4 | |
Pathologic N | 7.42E-01 | |||
N0 | 128 | 51 | 77 | |
N1 | 55 | 20 | 35 | |
Pathologic T | 5.30E-02 | |||
T1 | 137 | 47 | 90 | |
T2 | 67 | 30 | 37 | |
T3 | 16 | 9 | 7 | |
T4 | 10 | 1 | 9 | |
Pathologic stage | 1.91E-01 | |||
Stage I | 159 | 59 | 100 | |
Stage II | 64 | 25 | 39 | |
Stage III | 41 | 22 | 19 | |
Stage IV | 21 | 6 | 15 | |
Neoplasm histologic grade | 7.10E-01 | |||
G1 | 18 | 9 | 9 | |
G2 | 129 | 52 | 77 | |
G3 | 117 | 47 | 70 | |
Recurrence | 8.69E-03 | |||
Yes | 30 | 5 | 25 | |
No | 217 | 91 | 126 |
Relationships of the two subtypes with the immune microenvironment
To explore the relationships of the two subtypes with the immune microenvironment, we estimated the fraction of immune cells in the CESC by the CIBERSORT algorithm. The results showed that different subtypes were infiltrated with various immune cells (Figure 4A): B cell memory (Figure 4B), T cell CD8+ (Figure 4C), Macrophage M0 (Figure 4D) were meaningfully increased in subtype 1, while Mast cell resting (Figure 4E), Eosinophil (Figure 4F), and Neutrophil (Figure 4G) were significantly infiltrated in subtype 2. And there is no significant difference identified with other immune cells. Then, the immune scores of these two subtypes were performed by estimate package. We identified subtypes 1 and 2 to low and high TIME (tumor immune microenvironment) groups according to the different immune scores (Figure 5A-5C).
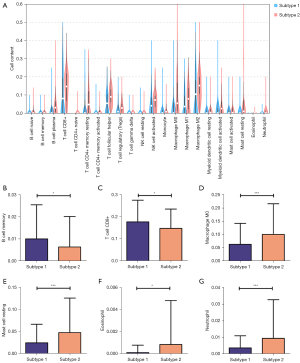
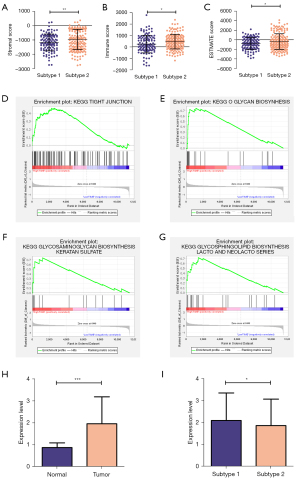
Furthermore, we conducted GSEA to explore the critical KEGG pathway with varying TIME scores. The results showed that various KEGG pathways enriched in the high TIME group. For example, tight junction (Figure 5D), O-glycan biosynthesis (Figure 5E), glycosaminoglycan biosynthesis keratan sulfate (Figure 5F), glycosphingolipid biosynthesis Lacto and Neolacto series (Figure 5G). Encouraged by the above results, we doubted any association between different subtypes and immune checkpoint molecules. Interestingly, we found the expression level of PD-L1 was positively related to tumor tissues and subtypes (Figure 5H,5I).
Development of a predictive risk prediction model with DE m6A genes
Results above have revealed that the DE m6A genes were related to prognosis and immune microenvironment. Next, we developed a predictive risk prediction model with DE m6A genes. We used LASSO Cox regression analysis to calculate the 8 DE m6A genes (Figure 6A,6B). We finally selected five genes to construct the risk signature, and the coefficient of IGF2BP1, IGF2BP2, HNRNPA2B1, YTHDF1, RBM15 were 0.023558929, 0.021148829, 0.045035491, −0.106566550, and −0.001037932. And we further validated the expressions of the genes in GSE7803, the expressions of IGF2BP2, HNRNPA2B1, YTHDF1, RBM15 were similar as the TCGA and the IGF2BP1 wasn’t dected in the GSE7803 (Figure S1). Then we calculated the risk score for each tumor tissue with the formula: risk score = (0.023558929) * Exp IGF2BP1 + (0.021148829) * Exp IGF2BP2 + (0.045035491) * Exp HNRNPA2B1 + (-0.106566550) * Exp YTHDF1 + (−0.001037932) * Exp RBM15. We display the RS score distribution and clinical survival information distribution of samples in TCGA training and GSE44001 validation databases (Figure 6C-6F), divided into low- and high-risk groups based on the median risk score. Meanwhile, we used the time-dependent receiver operating characteristic (ROC) curve to test the specificity and sensitivity of the predictive signature. In the TCGA training cohort, the area under the curve (AUC) at 1, 3, and 5 years was 0.819, 0.861, and 0.849 (Figure 6G). And in the GSE44001 validation cohort, the AUC at 1, 3, and 5 years were 0.708, 0.737, and 0.718 (Figure 6H). All the results suggested the predictive risk prediction model performed well.
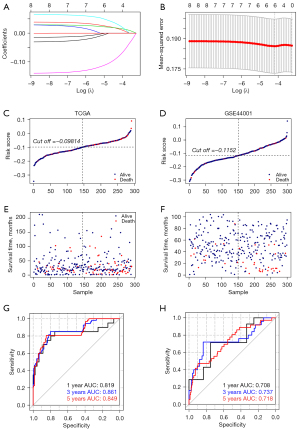
Association between DE m6A genes risk scores and clinical characteristics
Next, we evaluated the association between the risk scores and the clinical features. The Kaplan-Meier survival analysis showed the risk scores associated with the disease-free survival in the TCGA training and GSE44001 validation cohorts (Figure 7A,7B), indicating that the risk scores may be an independent prognostic tool. To further evaluate the independent predictive for CESC patients, we used the univariate and multivariate Cox regression models dealing with the factors including RS status, age, pathologic TNM, pathologic stage, neoplasm histologic grade, and tumor recurrence. We found Pathologic T, tumor recurrence, and RS status was significantly associated with survival in univariate and multivariate Cox analyses (Table 2). Then we analyze the differences of each independent prognostic clinical factor in RS distribution. And T3 has significant differences with T1 and T4 in RS scores; samples with recurrence got higher RS scores than those without recurrence (Figure 7C,7D).
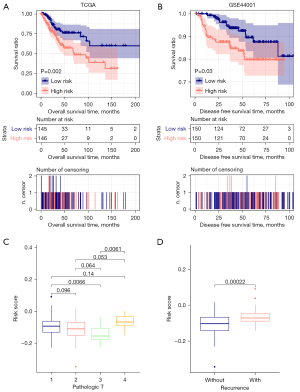
Table 2
Clinical characteristics | Uni-variable cox | Multi-variable cox | |||
---|---|---|---|---|---|
HR (95% CI) | P value | HR (95% CI) | P value | ||
Age (years) | 1.017 (0.999–1.035) | 6.00E-02 | – | – | |
Pathologic M (M0/M1) | 3.671 (0.229–10.96) | 1.26E-01 | – | – | |
Pathologic N (N0/N1/N2/N3) | 2.808 (0.409–5.596) | 2.19E-01 | – | – | |
Pathologic T (T1/T2/T3/T4) | 1.833 (1.371–2.452) | 2.31E-05 | 1.952 (1.016–3.752) | 4.48E-02 | |
Pathologic stage (I/II/III/IV) | 1.488 (1.195–1.852) | 2.86E-04 | 0.991 (0.536–1.832) | 9.77E-01 | |
Neoplasm histologic grade (G1/G2/G3) | 0.993 (0.643–1.535) | 9.76E-01 | – | – | |
Tumor recurrence (yes/no) | 4.791 (2.753–8.338) | 9.35E-10 | 5.377 (2.492–11.598) | 1.80E-05 | |
RS status (high/low) | 2.148 (1.306–3.534) | 1.83E-03 | 1.942 (1.183–4.270) | 9.88E-03 |
RS, risk score; TNM, tumor-node-metastasis; HR, hazard ratio; CI, confidence interval.
Correlation between prognosis characteristics and immunity based on DE m6A genes
Based on the mRNA expression level of TCGA tumor samples, the correlation of five DE m6A genes was used to construct the RS predictive model. The abundance of six immune cell infiltration was analyzed using the online tool TIMER. Different DE m6A genes could influence additional immune cell infiltration. IGF2BP1-2 significantly correlated with B cell, CD8+ T cell, and macrophage (Figure 8A,8B). HNRNPA2B1 has shown no significant correlation with immune cell infiltration (Figure 8C). YTHDF1 correlated considerably with CD8+ T cell and neutrophil (Figure 8D). RBM15 is significantly associated with CD4+ T cells (Figure 8E). At the same time, we analyzed the differences in the abundance of different cells under different copy number variations of the DE m6A genes (Figure 8F-8J). The above results showed that the five-gene predictive risk prediction model might reveal OS via immune cells infiltration.
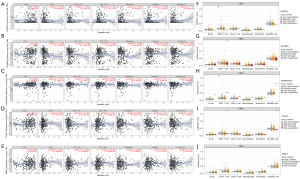
Discussion
Recently, m6A has played multifunctional roles in physiological and pathological processes and gained more and more attention. To reveal the epigenetic regulatory role and identify the influence on immune microenvironment and immunotherapy. It may help provide insight into the interactions of m6A-related genes in the anti-CESC immune response and develop an appropriate treatment plan. Using Consensus Cluster analysis, the current study demonstrated that CESC tissues in the TCGA could be divided into two subtypes by 8 DE m6A genes. Importantly, we identified a series of immune cells related to the different prognosis subtypes; the immune scores and the expression of the PD-L1 were different in the two subtypes. To further develop a predictive risk prediction model with DE m6A genes, we created a formula: risk score = (0.023558929) * Exp IGF2BP1 + (0.021148829) * Exp IGF2BP2 + (0.045035491) * Exp HNRNPA2B1 + (−0.106566550) * Exp YTHDF1 + (−0.001037932) * Exp RBM15. And we found risk scores may be an independent prognostic tool associated with the Pathologic T and tumor recurrence according to our model. Then, we found IGF2BP1-2 significantly correlated with B cell, CD8+ T cell, and macrophage; YTHDF1 correlated considerably with CD8+ T cell and neutrophil; RBM15 is significantly associated with CD4+ T cells. These data highlight an essential role of the predictive risk prediction model in prediction for CESC patients.
The expression of methyltransferases, demethylases, and binding proteins regulated the level of m6A methylation. Previous studies have demonstrated that the presentation of the RNA m6A modification proteins is associated with poor patient prognosis (33). Recent studies reveal that the m6A modification played a non-negligible role in the diversity and complexity of the tumor microenvironment (TME) (34,35). The TME plays an essential role in cancer progression and significantly affects responsiveness to immunotherapy (9). Alteration of the m6A modification in tumor cells influences the infiltration, activation, and effector functions of infiltrated immune cells in the TME (36). Nowadays, several studies have evaluated the function of m6A regulators in CESC. Zhang et al. found that YTHDC2 with Missense mutation could cause a different prognosis in CESC (37). Zhu et al. performed m6A regulators may be essential factors for phenotypic modifications of immune-related genes and thus affecting TME (38). Pan (20) and Wu (21) have analyzed the expression of m6A RNA methylation in CESC, but they didn’t get the perfect predictive model and missed the influence in TME. Zhang evaluated N6-Methyladenosine-Related lncRNAs as potential biomarkers for the prognoses prediction in CESC (22). In CESC, the part of the m6A methylation remains to learn.
In the current study, a five-gene predictive risk prediction model of IGF2BP1, IGF2BP2, HNRNPA2B1, YTHDF1, RBM15 was advanced and established decent presentation for predicting the survival of CESC. Additionally, we validated the model with the validation cohort GSE44001. Patients with relatively higher risk scores might have a shorter survival time, requiring more frequent examinations and favorable treatment. Meanwhile, we found two subtypes have a significant difference in the expression of the PD-L1. The expression or copy number variations of the DE m6A genes would influence the infiltration of the immune cells. All the results indicate that the m6A modification might regulate the immunotherapy response.
The IGF2BPs are essential in mRNA transport, alternative splicing, and m6A methylation (39). Our study found IGF2BP1 and IGF2BP2 expression significantly different between the CESC and normal tissues, also contained in our predictive risk prediction model. In recent years, Liu found IGF2BP1 could be an independent predictor for prognosis and the situation of tumor immunity in lung adenocarcinoma immunotherapy (40). And IGF2BP2 participated in metabolic diseases and cancer prognosis, including diabetes, breast cancer, esophageal adenocarcinoma, lung cancer, and many others (41). But IGF2BP functions in CESC were unclear, including in tumorigenesis, tumor development, and tumor immunity. Zhu found HNRNPA2B1 targeted EMT via the LINE-1/TGF-β1/Smad2/Slug signaling pathway to promote tumorigenesis and metastasis of oral squamous cell carcinoma (42). Furthermore, RBM15 regulated TMBIM6 stability through IGF2BP3-dependent to facilitate laryngeal squamous cell carcinoma progression (43). In CESC, more studies need to discover the function of m6A modification.
In conclusion, our study showed that m6A modification is a significant association with the survival and immunity of CESC. And we also a conducted DE m6A RNA methylation-based predictive risk prediction model effectively predicting the prognosis of CESC patients, which helped to understand the function of m6A RNA modification in CESC. However, more studies are still needed to confirm the different RNA modifications in the CESC.
Acknowledgments
We thank The Cancer Genome Atlas (TCGA) project, Gene Expression Omnibus (GEO), CIBERSORT, and Tumor Immune Estimation Resource (TIMER) databases and their contributors for the valuable public datasets used in this study.
Funding: None.
Footnote
Reporting Checklist: The authors have completed the TRIPOD reporting checklist. Available at https://tcr.amegroups.com/article/view/10.21037/tcr-22-881/rc
Peer Review File: Available at https://tcr.amegroups.com/article/view/10.21037/tcr-22-881/prf
Conflicts of Interest: All authors have completed the ICMJE uniform disclosure form (available at https://tcr.amegroups.com/article/view/10.21037/tcr-22-881/coif). The authors have no conflicts of interest to declare.
Ethical Statement: The authors are accountable for all aspects of the work in ensuring that questions related to the accuracy or integrity of any part of the work are appropriately investigated and resolved. The study was conducted in accordance with the Declaration of Helsinki (as revised in 2013).
Open Access Statement: This is an Open Access article distributed in accordance with the Creative Commons Attribution-NonCommercial-NoDerivs 4.0 International License (CC BY-NC-ND 4.0), which permits the non-commercial replication and distribution of the article with the strict proviso that no changes or edits are made and the original work is properly cited (including links to both the formal publication through the relevant DOI and the license). See: https://creativecommons.org/licenses/by-nc-nd/4.0/.
References
- Siegel RL, Miller KD, Fuchs HE, et al. Cancer Statistics, 2021. CA Cancer J Clin 2021;71:7-33. Erratum in: CA Cancer J Clin 2021;71:359. [Crossref] [PubMed]
- Koh WJ, Abu-Rustum NR, Bean S, et al. Cervical Cancer, Version 3.2019, NCCN Clinical Practice Guidelines in Oncology. J Natl Compr Canc Netw 2019;17:64-84. [Crossref] [PubMed]
- Cohen AC, Roane BM, Leath CA 3rd. Novel Therapeutics for Recurrent Cervical Cancer: Moving Towards Personalized Therapy. Drugs 2020;80:217-27. [Crossref] [PubMed]
- Monk BJ, Sill MW, Burger RA, et al. Phase II trial of bevacizumab in the treatment of persistent or recurrent squamous cell carcinoma of the cervix: a gynecologic oncology group study. J Clin Oncol 2009;27:1069-74. [Crossref] [PubMed]
- Delaunay S, Frye M. RNA modifications regulating cell fate in cancer. Nat Cell Biol 2019;21:552-9. [Crossref] [PubMed]
- Roundtree IA, Evans ME, Pan T, et al. Dynamic RNA Modifications in Gene Expression Regulation. Cell 2017;169:1187-200. [Crossref] [PubMed]
- Frye M, Harada BT, Behm M, et al. RNA modifications modulate gene expression during development. Science 2018;361:1346-49. [Crossref] [PubMed]
- He L, Li H, Wu A, et al. Functions of N6-methyladenosine and its role in cancer. Mol Cancer 2019;18:176. [Crossref] [PubMed]
- Li X, Ma S, Deng Y, et al. Targeting the RNA m6A modification for cancer immunotherapy. Mol Cancer 2022;21:76. [Crossref] [PubMed]
- Xiong J, He J, Zhu J, et al. Lactylation-driven METTL3-mediated RNA m6A modification promotes immunosuppression of tumor-infiltrating myeloid cells. Mol Cell 2022;82:1660-77.e10. [Crossref] [PubMed]
- Liu Z, Wang T, She Y, et al. N6-methyladenosine-modified circIGF2BP3 inhibits CD8+ T-cell responses to facilitate tumor immune evasion by promoting the deubiquitination of PD-L1 in non-small cell lung cancer. Mol Cancer 2021;20:105. [Crossref] [PubMed]
- Wan W, Ao X, Chen Q, et al. METTL3/IGF2BP3 axis inhibits tumor immune surveillance by upregulating N6-methyladenosine modification of PD-L1 mRNA in breast cancer. Mol Cancer 2022;21:60. [Crossref] [PubMed]
- Lan Q, Liu PY, Haase J, et al. The Critical Role of RNA m6A Methylation in Cancer. Cancer Res 2019;79:1285-92. [Crossref] [PubMed]
- Zhou S, Bai ZL, Xia D, et al. FTO regulates the chemo-radiotherapy resistance of cervical squamous cell carcinoma (CSCC) by targeting β-catenin through mRNA demethylation. Mol Carcinog 2018;57:590-7. [Crossref] [PubMed]
- Li Z, Peng Y, Li J, et al. N6-methyladenosine regulates glycolysis of cancer cells through PDK4. Nat Commun 2020;11:2578. [Crossref] [PubMed]
- Wang Q, Guo X, Li L, et al. N6-methyladenosine METTL3 promotes cervical cancer tumorigenesis and Warburg effect through YTHDF1/HK2 modification. Cell Death Dis 2020;11:911. [Crossref] [PubMed]
- Ma X, Li Y, Wen J, et al. m6A RNA methylation regulators contribute to malignant development and have a clinical prognostic effect on cervical cancer. Am J Transl Res 2020;12:8137-46. [PubMed]
- Guan K, Liu X, Li J, et al. Expression Status And Prognostic Value Of M6A-associated Genes in Gastric Cancer. J Cancer 2020;11:3027-40. [Crossref] [PubMed]
- Li Y, Qi D, Zhu B, et al. Analysis of m6A RNA Methylation-Related Genes in Liver Hepatocellular Carcinoma and Their Correlation with Survival. Int J Mol Sci 2021;22:1474. [Crossref] [PubMed]
- Pan J, Xu L, Pan H. Development and Validation of an m6A RNA Methylation Regulator-Based Signature for Prognostic Prediction in Cervical Squamous Cell Carcinoma. Front Oncol 2020;10:1444. [Crossref] [PubMed]
- Wu H, Dong H, Fu Y, et al. Expressions of m6A RNA methylation regulators and their clinical predictive value in cervical squamous cell carcinoma and endometrial adenocarcinoma. Clin Exp Pharmacol Physiol 2021;48:270-8. [Crossref] [PubMed]
- Zhang H, Kong W, Zhao X, et al. N6-Methyladenosine-Related lncRNAs as potential biomarkers for predicting prognoses and immune responses in patients with cervical cancer. BMC Genom Data 2022;23:8. [Crossref] [PubMed]
- Zhai Y, Kuick R, Nan B, et al. Gene expression analysis of preinvasive and invasive cervical squamous cell carcinomas identifies HOXC10 as a key mediator of invasion. Cancer Res 2007;67:10163-72. [Crossref] [PubMed]
- Wang L, Cao C, Ma Q, et al. RNA-seq analyses of multiple meristems of soybean: novel and alternative transcripts, evolutionary and functional implications. BMC Plant Biol 2014;14:169. [Crossref] [PubMed]
- Zhang X, Ren L, Yan X, et al. Identification of immune-related lncRNAs in periodontitis reveals regulation network of gene-lncRNA-pathway-immunocyte. Int Immunopharmacol 2020;84:106600. [Crossref] [PubMed]
- Wang P, Wang Y, Hang B, et al. A novel gene expression-based prognostic scoring system to predict survival in gastric cancer. Oncotarget 2016;7:55343-51. [Crossref] [PubMed]
- Newman AM, Liu CL, Green MR, et al. Robust enumeration of cell subsets from tissue expression profiles. Nat Methods 2015;12:453-7. [Crossref] [PubMed]
- Hu D, Zhou M, Zhu X. Deciphering Immune-Associated Genes to Predict Survival in Clear Cell Renal Cell Cancer. Biomed Res Int 2019;2019:2506843. [Crossref] [PubMed]
- Subramanian A, Tamayo P, Mootha VK, Mukherjee S, Ebert BL, Gillette MA, Paulovich A, Pomeroy SL, Golub TR, et al. Gene set enrichment analysis: a knowledge-based approach for interpreting genome-wide expression profiles. Proc Natl Acad Sci U S A 2005;102:15545-50. [Crossref] [PubMed]
- Li R, Chang C, Tanigawa Y, et al. Fast Numerical Optimization for Genome Sequencing Data in Population Biobanks. Bioinformatics 2021;37:4148-55. [Crossref] [PubMed]
- Qin R, Cao L, Ye C, et al. A novel prognostic prediction model based on seven immune-related RNAs for predicting overall survival of patients in early cervical squamous cell carcinoma. BMC Med Genomics 2021;14:49. [Crossref] [PubMed]
- Li T, Fan J, Wang B, et al. TIMER: A Web Server for Comprehensive Analysis of Tumor-Infiltrating Immune Cells. Cancer Res 2017;77:e108-10. [Crossref] [PubMed]
- Lan Q, Liu PY, Bell JL, et al. The Emerging Roles of RNA m6A Methylation and Demethylation as Critical Regulators of Tumorigenesis, Drug Sensitivity, and Resistance. Cancer Res 2021;81:3431-40. [Crossref] [PubMed]
- Zhang B, Wu Q, Li B, et al. m6A regulator-mediated methylation modification patterns and tumor microenvironment infiltration characterization in gastric cancer. Mol Cancer 2020;19:53. [Crossref] [PubMed]
- Zhao BS, Roundtree IA, He C. Post-transcriptional gene regulation by mRNA modifications. Nat Rev Mol Cell Biol 2017;18:31-42. Erratum in: Nat Rev Mol Cell Biol 2018;19:808. [Crossref] [PubMed]
- Gu Y, Wu X, Zhang J, et al. The evolving landscape of N6-methyladenosine modification in the tumor microenvironment. Mol Ther 2021;29:1703-15. [Crossref] [PubMed]
- Zhang C, Guo C, Li Y, et al. The role of YTH domain containing 2 in epigenetic modification and immune infiltration of pan-cancer. J Cell Mol Med 2021;25:8615-27. [Crossref] [PubMed]
- Zhu J, Xiao J, Wang M, et al. Pan-Cancer Molecular Characterization of m6A Regulators and Immunogenomic Perspective on the Tumor Microenvironment. Front Oncol 2021;10:618374. [Crossref] [PubMed]
- Korn SM, Ulshöfer CJ, Schneider T, et al. Structures and target RNA preferences of the RNA-binding protein family of IGF2BPs: An overview. Structure 2021;29:787-803. [Crossref] [PubMed]
- Liu J, Li Z, Cheang I, et al. RNA-Binding Protein IGF2BP1 Associated With Prognosis and Immunotherapy Response in Lung Adenocarcinoma. Front Genet 2022;13:777399. [Crossref] [PubMed]
- Wang J, Chen L, Qiang P. The role of IGF2BP2, an m6A reader gene, in human metabolic diseases and cancers. Cancer Cell Int 2021;21:99. [Crossref] [PubMed]
- Zhu F, Yang T, Yao M, et al. HNRNPA2B1, as a m6A Reader, Promotes Tumorigenesis and Metastasis of Oral Squamous Cell Carcinoma. Front Oncol 2021;11:716921. [Crossref] [PubMed]
- Wang X, Tian L, Li Y, et al. RBM15 facilitates laryngeal squamous cell carcinoma progression by regulating TMBIM6 stability through IGF2BP3 dependent. J Exp Clin Cancer Res 2021;40:80. [Crossref] [PubMed]