Bioinformatics analysis and identification of genes and pathways involved in patients with Wilms tumor
Introduction
Wilms tumor, or nephroblastoma, is an embryonic malignant tumor in children which accounts for almost 6% of all pediatric cancers (1,2). The tumor was first reported in 1814 and its detailed pathological description was made by Wilms in 1899. Wilms tumor is mainly seen in children under 5 years of age, while 90% of new cases occur in those less than 3 years of age (3). Although the current cure rate approaches 85% the early stage of the disease lack specific symptoms, which often delays both diagnosis and optimal treatment (4,5). Moreover, about 25% of Wilms tumor patients will face chronic health problems for many years after diagnosis and treatment (6,7). Therefore, it is of great significance to explore the molecular mechanism of Wilms tumor, such as genes and pathways, to improve its diagnosis, prediction, and treatment. In this study we sought to detail specific biomarkers for Wilms tumor by identifying DEGs between it and normal tissues.
Gene chipping, or gene profiling, is an important technology platform in the field of life sciences which has been used for more than 10 years. It is an effective means of screening differentially expressed genes (DEGs), which has the advantages of high throughput and fast measurement (8). Gene chipping has been widely used in gene expression analysis, gene discovery, gene mutation and polymorphism analysis, genome library mapping, disease diagnosis and prediction, drug screening, gene sequencing, and other fields. The development of high throughput microarray hybridization and sequencing technology has generated a great deal of genetic data, and biological information has rapidly expanded into the ocean of data. To adapt to this kind of high throughput gene expression data and the increasing need for data sharing, a variety of databases have been generated, including the national biological information technology center (NCBI) and the high throughput Gene Expression Omnibus (GEO) database. These public databases store the world’s largest high throughput molecular abundance data. Many valuable clues for new research have been discovered through integrating and re-analyzing these databases, and in recent years, many microarray data analysis studies have been published. For example, Zhang et al. (9) used bioinformatics methods to detect the gene expression profile data GSE13601 and revealed the potential mechanism of tongue squamous cell carcinoma, while Long et al. (10) reported that by analyzing the gene expression profile data GSE19804, the DEGs and hub genes enhanced the cognitive of the occurrence and development of lung cancer. The GEO and TCGA databases were used by Zhang et al. (11) to identify key genes and microRNAs in patients with Wilms tumor and Li et al. (12) to screen immune-related prognostic genes in clear cell renal tumors. At present, there is no reliable biomarker for Wilms tumor and the molecular mechanism of its occurrence and development have not been fully clarified.
In this study, the microarray profiles GSE66450, GSE73209, and GSE11151 were selected and collected from the GEO database and analyzed using bioinformatics methods. The purpose of this study was to find common DEGs, analyze them with Gene Ontology (GO) and Kyoto Encyclopedia of Genes and Genomes (KEGG) methods, and construct a protein-protein interaction (PPI) network. These data sets may help to improve the understanding of Wilms tumor and have a significant impact on its diagnosis and treatment. The purpose of this study is to find out potential biomarkers for the treatment of Wilms tumor. The combination of multiple chips can greatly increase the accuracy of screening hub genes. We present the following article in accordance with the STREGA reporting checklist (available at https://tcr.amegroups.com/article/view/10.21037/tcr-22-1847/rc).
Methods
Microarray data information and DEGs identification
The Wilms tumor gene expression profile of GSE66405, GSE73209, and GSE11151 were obtained from the GEO database (https://www.ncbi.nlm.nih.gov/geo/), and the raw expression data were downloaded as a series matrix file. The platform information of GSE66405 microarray data was as follows: GPL17077, Agilent-039494 Sure Print G3 Human GE v2 8×60K Microarray 039381 (probe name version) (submission date: 02 Mar 2016) (13). The platform information of GSE73209 microarray data was as follows: GPL10558, Illumina HumanHT-12 V4.0 expression bead chip (Submission date: 18 Sep 2016) (14), and for GSE11151 microarray data was GPL570 (HG-U133_plus_2) Affymetrix Human Genome U133 plus 2.0 Array (submission date: 11 Apr 2008) (15-17). The Affy installation package (library “affy” in R, https://www.r-project.org/) of R software was used for data correction and normalization of each chip. Applying the “limma” package (18) (https://bioconductor.org/packages/limma/) in the R statistics environment, DEGs were then extracted from GSE66405, GSE73209, and GSE11151, respectively. FDR ≤0.05 and |log2 fold change (FC)| ≥1 were set as the thresholds for DEGs. Finally, the “Robust Rank Aggregate” package (19) in R language was applied to identify common DEGs among those from the expression profile data sets. The study was conducted in accordance with the Declaration of Helsinki (as revised in 2013).
Construction of PPI network
The online database STRING (available online: http://string-db.org) (20) was employed to analyze the PPI network, and after obtaining the common DEGs with a combined score >0.04 were extracted.
Module analysis and hub genes selection
Subsequently, we used the Molecular Complex Detection (MCODE) tool with a cutoff MCODE score >5 in Cytoscape software (version 3.7.2) analysis of the PPI network. In addition, according to the Closeness method, identify hub genes using the CytoHubba plugin, where genes of the top 10 degrees were considered hub genes in the PPI network.
Functional enrichment analysis
For further functional analysis, the package “clusterProfiler” in R software was used to conduct KEGG and GO enrichment analysis on the common DEGs. Moreover, KEGG and GO pathway analyses of modules genes were conducted with the “clusterprofiler” package in R. For pathway analysis, P<0.05 was seemed as the significant gene functionals, and the results were visualized using the “ggplot2” and “GOplot” packages in R software.
Gene set enrichment analysis (GSEA)
GSEA v4.01.0 software was downloaded from the GSEA home website (http://www.gsea-msigdb.org/gsea/index.jsp) and the software implemented in a Java environment. GSEA was run using c2.cp.kegg.v.7.2.symbols.gmt (Curated) gene sets database, with 1,000 number of permutations, and GSEA FDR <0.05 was considered statistically significant. Multiple GSEA results were visualized using the R software.
Statistical analysis
All statistical analyses were completed in R software. The DEGs were analyzed via the limma package. The Wilcoxon non-parametric test was used for compare differences in two groups, and the correlation analyses were conducted using Spearman’s method. A P value less than 0.05 was considered statistically significant.
Results
Identification of DEGs in Wilms tumor
The GSE66405 data included 28 Wilms tumor and 4 normal tissues, the GSE73209 data had 32 Wilms tumor and 6 normal tissues, and the GSE11151 data included 4 Wilms tissues and 5 normal tissues (Table 1). Before data analysis, the R bioconductor “affy” package was used for the normalization of Affymetrix data (Figure 1). The identification of DEGs of microarray data after normalization was performed via “Limma” package in R. From the expression profile datasets GSE66405, GSE73209, and GSE11151, 3,092 (1,270 up- and 1,822 down-regulated genes), 620 (129 up- and 491 down-regulated genes), and 3,567 (1,698 up- and 1,869 down-regulated genes) DEGs were extracted, respectively. The heatmaps and volcano plots of the DEGs were generated using R language (Figure 2), with its “Robust Rank Aggreg” package used to analyze the common DEGs from the three profile datasets. A total of 474 common DEGs were identified, including 82 up- and 392 down-regulated genes in Wilms tumor tissues, compared to normal tissues. The package in R language was used to draw the heat map of the top 20 up- and top 20 down-regulated DEGs from the common DEGs (Figure 3).
Table 1
GSE ID | Platform | Tumor | Normal | Year |
---|---|---|---|---|
GSE66405 | GPL17077 | 28 | 4 | 2016 |
GSE73209 | GPL10558 | 32 | 6 | 2016 |
GSE11151 | GPL570 | 4 | 5 | 2008 |
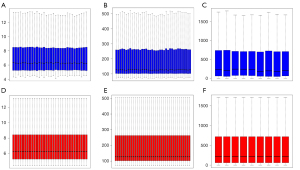
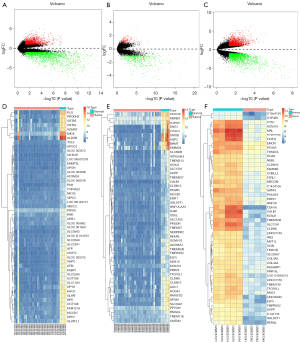
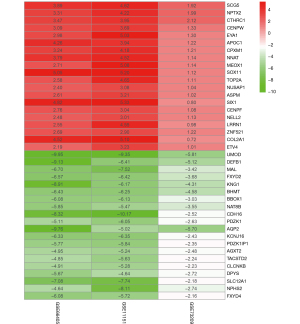
PPI network analysis results
To further highlight connections between the common DEGs, the STRING database was used. A total of 474 common DEGs (82 up- and 392 down-regulated genes) were filtered into the DEGs PPI network complex (Figure 4).
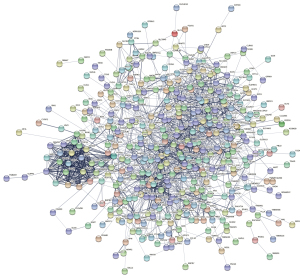
Modules and hub genes analysis
Using the MCODE app in Cytoscape software with a score ≥5, the five most significant modules were aggregated and extracted from the PPI network. Module 1 contained 23 nodes and 244 edges with a score of 22.182; module 2 contained 11 nodes and 50 edges with an MCODE score of 10.000; module 3 contained 11 nodes and 45 edges with an MCODE score of 9.000; module 4 contained eight nodes and 26 edges with a score of 7.429; and module 5 contained 26 nodes and 66 edges with a score of 5.280, respectively (Figure 5). Subsequently, with the using of cytoHubba we identify the top 10 hub genes of the PPI network, which included ALB, CDH1, EGF, AQP2, REN, SLC2A2, SPP1, UMOD, NPHS2, and FOXM1 (Figure 6A). By the final step, with a degree of 75, ALB was identified as the highest degree protein in the network (Figure 6B).
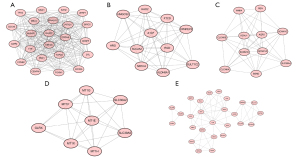
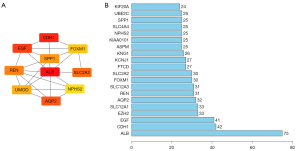
GO and KEGG analysis in Wilms tumor
To acquire a more comprehensive and in-depth understanding of the selected DEGs, KEGG pathways and GO pathways enrichment were analyzed in R software. GO enrichment analysis was classified into three aspects: biological process (BP), molecular function (MF), and cellular component (CC). The top 30 KEGG and GO pathway enrichments are shown (Figure 7). Moreover, to further investigate the biological functions of the DEGs in all the modules, KEGG and GO analysis were performed. DEGs in the modules were enriched in five KEGG pathways, including mineral absorption, glutathione metabolism, protein digestion and absorption, aldosterone-regulated sodium reabsorption, and platinum drug resistance (Figure 8A). GO enrichment analysis of the genes in modules showed 10 GO terms (GO:0006882, GO:0098754, GO:0055069, GO:0071280, GO:0010043, GO:0010273, GO:1990169, GO:0061687, GO:0097501, GO:0000280) represented significantly (Figure 8B).
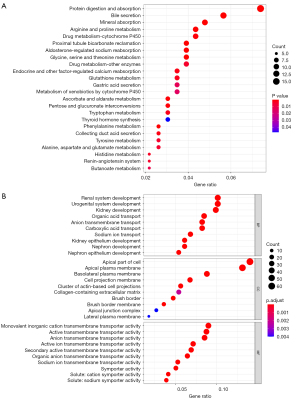
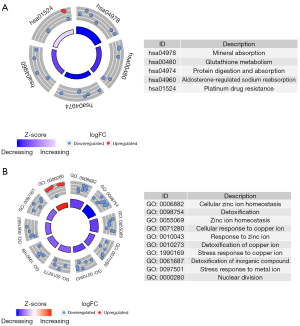
GSEA enrichment analysis
After determining ALB was the most related protein in the PPI network, we used the GSEA enrichment analysis method to detect the signal pathway related to the ALB gene in the expression profile data. In GSE66405, GSEA results showed apoptosis, the chemokine signaling pathway, FC epsilon RI signaling pathway, FC gamma R mediated phagocytosis, Mark signaling pathway, Neurotrophin signaling pathway, Nod-like receptor signaling pathway, Notch signaling pathway, Rig I like receptor signaling pathway, Toll-like receptor signaling pathway, and VEGF signaling pathway were correlated with ALB expression (Figure 9A). In GSE73209, GSEA results showed allograft rejection, the B cell receptor signaling pathway, Cell adhesion molecules cams, Chemokine signaling pathway, FC epsilon RI signaling pathway, Glycolysis gluconeogenesis, GRAFT versus HOST disease, Regulation of autophagy, RIG I like receptor signaling pathway, and T cell receptor signaling pathway were correlated with ALB expression (Figure 9B).
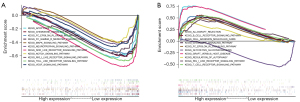
Discussion
In the present study, three Wilms tumor series matrix files, GSE66405, GSE73209, and GSE11151, were collected from the GEO online database. Moreover, all data were normalized before been analyzed into DEGs. Based on the “limma” package in R language, we extracted 3,092, 620, and 3,567 DEGs from the datasets GSE66405, GSE73209, and GSE11151, respectively. Using RobustRankAggregate packages in R language, we further detected 474 common DEGs between Wilms tumor tissue and normal tissue. As a comprehensive database that stores large amounts of genetic data, GEO has been widely used for bioinformatics analysis, and recently, massive genetic studies of cancer have been published (21-23). Several studies have reported the analysis of Wilms tumor using the GEO database, including Zhang et al. (11) who showed the key genes, miRNA, and mRNA-miRNA regulatory networks may contribute to further comprehending of the molecular mechanisms underlying the progress of the disease. The bioinformatics analysis from the GEO database by Zong et al. showed miR-30d was low-expressed in Wilms tumor and could induce apoptosis and inhibit proliferation, invasion, and migration by mediating Sox4 (24). However, there are few articles in Wilms tumor research utilizing multiple expression profiling data for analysis. Therefore, our study has some notable strengths, by analyzing the three GEO expression profiles.
After obtaining the 474 common DEGs, GO and KEGG analysis were performed via the “clusterProfiler” package, while STRING database analysis revealed the PPI network. Utilizing the MCODE app in Cytoscape software, five modules that might serve an important role in the development of Wilms tumor were detected. Studies have demonstrated the TGF-β/Smad signaling pathway plays a key role in Wilms tumor progression (25), while Li et al. showed S1P/S1P1 signaling stimulates cell migration and invasion in the disease (26). Therefore, our KEGG and GO results suggest these signaling pathways may serve a crucial role in Wilms tumor progression.
Previous studies have demonstrated genetic changes could accelerate the progression of Wilms tumor (27,28). In 1990, Wilms tumor 1 (WT1) was the first gene to be implicated in tumorigenesis and as a strong candidate predisposition gene for the disease (29,30). Recent medical studies confirmed WT1, located at chromosome 11p13, is mutated or absent in 15% of cases (31,32), and in a recent study, WT1 mutations activated cell cycle genes and promoted proliferation of Wilms tumor (33). Some researchers have reported relevant studies that WT1 was high-expressed in many types of cancer, including breast cancer (34), myeloproliferative neoplasms (35), ovarian cancer (36), and endometrial cancer (37). In this study, we constructed a PPI network and identified the top 10 hub genes via cytoHubba as ALB, CDH1, EGF, AQP2, REN, SLC2A2, SPP1, UMOD, NPHS2, and FOXM1. ALB is involved in maintaining human homeostasis and is closely related to many physiological and pathological processes. In pediatric tumors, the ALB gene was detected to be positive in hepatoblastoma and fibrolamellar hepatocellular carcinoma using immunohistochemistry and in situ hybridization, and the sensitivity and specificity of the situ hybridization was 100% (38). Cadherin 1 (CDH1) is related to cell adhesion and is regarded as an invasion-suppressor gene (39), and adecrease in its expression has been shown to be solely responsible for pancreatic cancer metastasis (40). In cardiovascular progenitor cells, WT1 binds to promoters of CDH1 to inhibit its activity and promotes EMT (41). EGF, an epidermal growth factor, stimulates not only cell growth but also cell migration in cancer cells (42,43), and is a potential target gene of WT1 in kidney development (44). Moreover, significant expression of EGF may play a role in promoting proliferation of Wilms tumor, perhaps by an autocrine mechanism (45). Aquaporin 2 (AQP2) is the arginine vasopressin regulated water channel of the kidney collecting duct, and several studies have shown it plays a key role in nephrogenic diabetes insipidus (46-49). Niu et al. have shown that overexpression of AQP2 in pheochromocytoma was positively correlated with tumor size, indicated that AQP2 has the potential to be a diagnostic immunohistochemical biomarker for that disease (50). In 1990, a report have revealed that Renin gene (REN) was high-expressed in Wilms tumor (51), and using in situ hybridization, McKenzie et al. detected REN mutations in 9 of 12 tumor tissues (52). Knockdown of WT1 protein by siRNA significantly increased the expression of renin mRNA, while overexpression reduced REN expression (53). Previous GWAS have identified the rs8192675 in SLC2A2 as being significantly associated with metformin response (54,55). The C allele of rs8192675 in the intron of SLC2A2 was associated with a 0.17% greater metformin-induced reduction in hemoglobin A1c (HbA1c) in 10,577 patients (55), while the expression level of SLC2A2 in hepatocellular carcinoma was higher than part of the family members, suggesting it as a novel prognostic biomarker for that disease (56). The SPP1 gene codes for secreted phosphoprotein-1 (SPP1) protein, also named osteopontin (OPN). SPP1 is highly expressed in a variety of human cancers and involve in many physiological and pathological processes, including cell proliferation, invasion, survival, and tumor metastasis (57,58). In hepatocellular carcinomas, elevated SPP1 levels were able to effectively increase growth and metastasis (59), and were also higher in cervical cancer (60). Higher SPP1 expressed levels were associated with poor disease-free survival (DFS) and overall survival (OS) in cervical cancer patients (61), while in prostate cancer, SPP1 was involved in tumor recurrence and metastasis progression by mediating the Smad4/PTEN pathway (62). Uromodulin (UMOD), also known as Tamm-Horsfall protein, encodes the uromodulin glycoprotein. Under normal physiologic conditions, UMOD is the most abundant protein found in urine, and may be a factor involved in the pathogenic process of kidney disease (63). Remarkably, uromodulin urinary levels in patients after renal transplantation were associated with donor UMOD rs12917707 genotype (64). Mutations in NPHS2 were found worldwide in nephrotic syndrome patients from different countries and ethnic origins (65-67), and more than 100 mutations in NPHS2 have been identified in patients with the disease (68). By integration of cistromic and transcriptomic analyses, NPHS2 was identified as a target gene of WT1 in podocyte differentiation and maintenance (69). FOXM1 (forkhead box M1) is a member of the forkhead box family, and its dysregulation can significantly contribute to tumorigenesis and cancer progression. Overexpression of FOXM1 has been observed in many human cancers including breast cancer (70), pancreatic cancer (71), and hepatocellular carcinoma (72), and was shown to correlate with a poor prognosis in lung cancer patients (73). Knockdown of FOXM1 expression could inhibit cervical cancer cells migration, invasion, and promote sensitivity to cisplatin (74), while its knockdown alone could inhibit cell proliferation and invasion and induce apoptosis in esophageal squamous cell carcinoma (75). The hub genes in the present findings have been published in several articles and are of potential value in the study of Wilms tumor. From the perspective of epigenetics, cancer is the result of result of gene imbalance, methylation, acetylation and phosphorylation are the causes of gene dysregulation (76,77). We seeked potential targets via researched dysregulated genes for the prevention and treatment of nephroblastoma.
One interesting finding in this study was that the hub gene ALB was the most associated protein in the PPI network, compared with other hub genes. Therefore, we performed GSEA analysis in expression profiling data GSE66405 and GSE73209 for examples with high ALB expression and low ALB expression, and the results provide a theoretical basis for the study of ALB genes in Wilms tumor. Unfortunately, the expression profile data GSE11151 was in error due to insufficient examples of the dataset. In addition, the limitations of this study are lack of the validation of expression level and functional research on the main target genes.
Conclusions
Our study displayed robust gene signatures in Wilms tumor and dysregulation of the pathways was closely associated with the development and progression of the disease. The hub genes ALB, CDH1, EGF, AQP2, REN, SLC2A2, SPP1, UMOD, NPHS2, and FOXM1 may play an important role in diagnosing and treating Wilms tumor.
Acknowledgments
We acknowledge the GEO database for providing their platforms and the contributors for uploading their meaningful datasets. We thank all the teachers and students who have helped us in this research.
Funding: The study was supported by Baise Scientific Research and Technology Development Plan (Nos. 20193125 and 20213212).
Footnote
Reporting Checklist: The authors have completed the STREGA reporting checklist. Available at https://tcr.amegroups.com/article/view/10.21037/tcr-22-1847/rc
Conflicts of Interest: All authors have completed the ICMJE uniform disclosure form (available at https://tcr.amegroups.com/article/view/10.21037/tcr-22-1847/coif). The authors have no conflicts of interest to declare.
Ethical Statement: The authors are accountable for all aspects of the work in ensuring that questions related to the accuracy or integrity of any part of the work are appropriately investigated and resolved. The study was conducted in accordance with the Declaration of Helsinki (as revised in 2013).
Open Access Statement: This is an Open Access article distributed in accordance with the Creative Commons Attribution-NonCommercial-NoDerivs 4.0 International License (CC BY-NC-ND 4.0), which permits the non-commercial replication and distribution of the article with the strict proviso that no changes or edits are made and the original work is properly cited (including links to both the formal publication through the relevant DOI and the license). See: https://creativecommons.org/licenses/by-nc-nd/4.0/.
References
- Mavinkurve-Groothuis AM, van den Heuvel-Eibrink MM, Tytgat GA, et al. Treatment of relapsed Wilms tumour (WT) patients: experience with topotecan. A report from the SIOP Renal Tumour Study Group (RTSG). Pediatr Blood Cancer 2015;62:598-602. [Crossref] [PubMed]
- Gleason JM, Lorenzo AJ, Bowlin PR, et al. Innovations in the management of Wilms' tumor. Ther Adv Urol 2014;6:165-76. [Crossref] [PubMed]
- Gooskens SL, Segers H, Pritchard-Jones K, et al. The Clinical Relevance of Age at Presentation in Nephroblastoma 2016.
- Baskaran D. Extrarenal teratoid wilms' tumor in association with horseshoe kidney. Indian J Surg 2013;75:128-32. [Crossref] [PubMed]
- Malogolowkin M, Spreafico F, Dome JS, et al. Incidence and outcomes of patients with late recurrence of Wilms' tumor. Pediatr Blood Cancer 2013;60:1612-5. [Crossref] [PubMed]
- Luu DT, Duc NM, Tra My TT, et al. Wilms' Tumor in Horseshoe Kidney. Case Rep Nephrol Dial 2021;11:124-8. [Crossref] [PubMed]
- Perrino CM, Wang JF, Chen AC, et al. Adult wilms' tumor metastatic to the lung: endobronchial ultrasound-guided fine needle aspiration biopsy. Diagn Cytopathol 2014;42:950-5. [Crossref] [PubMed]
- Vogelstein B, Papadopoulos N, Velculescu VE, et al. Cancer genome landscapes. Science 2013;339:1546-58. [Crossref] [PubMed]
- Zhang H, Liu J, Fu X, et al. Identification of Key Genes and Pathways in Tongue Squamous Cell Carcinoma Using Bioinformatics Analysis. Med Sci Monit 2017;23:5924-32. [Crossref] [PubMed]
- Long T, Liu Z, Zhou X, et al. Identification of differentially expressed genes and enriched pathways in lung cancer using bioinformatics analysis. Mol Med Rep 2019;19:2029-40. [Crossref] [PubMed]
- Zhang L, Gao X, Zhou X, et al. Identification of key genes and microRNAs involved in kidney Wilms tumor by integrated bioinformatics analysis. Exp Ther Med 2019;18:2554-64. [Crossref] [PubMed]
- Li J, Cao J, Li P, et al. A Bioinformatic Analysis of Immune-Related Prognostic Genes in Clear Cell Renal Cell Carcinoma Based on TCGA and GEO Databases. Int J Gen Med 2022;15:325-42. [Crossref] [PubMed]
- Ludwig N, Werner TV, Backes C, et al. Combining miRNA and mRNA Expression Profiles in Wilms Tumor Subtypes. Int J Mol Sci 2016;17:475. [Crossref] [PubMed]
- Karlsson J, Valind A, Jansson C, et al. Aberrant epigenetic regulation in clear cell sarcoma of the kidney featuring distinct DNA hypermethylation and EZH2 overexpression. Oncotarget 2016;7:11127-36. [Crossref] [PubMed]
- Yusenko MV, Ruppert T, Kovacs G. Analysis of differentially expressed mitochondrial proteins in chromophobe renal cell carcinomas and renal oncocytomas by 2-D gel electrophoresis. Int J Biol Sci 2010;6:213-24. [Crossref] [PubMed]
- Yusenko MV, Zubakov D, Kovacs G. Gene expression profiling of chromophobe renal cell carcinomas and renal oncocytomas by Affymetrix GeneChip using pooled and individual tumours. Int J Biol Sci 2009;5:517-27. [Crossref] [PubMed]
- Yusenko MV, Kuiper RP, Boethe T, et al. High-resolution DNA copy number and gene expression analyses distinguish chromophobe renal cell carcinomas and renal oncocytomas. BMC Cancer 2009;9:152. [Crossref] [PubMed]
- Guo Y, Bao Y, Ma M, et al. Identification of Key Candidate Genes and Pathways in Colorectal Cancer by Integrated Bioinformatical Analysis. Int J Mol Sci 2017;18:722. [Crossref] [PubMed]
- Kolde R, Laur S, Adler P, et al. Robust rank aggregation for gene list integration and meta-analysis. Bioinformatics 2012;28:573-80. [Crossref] [PubMed]
- Franceschini A, Szklarczyk D, Frankild S, et al. STRING v9.1: protein-protein interaction networks, with increased coverage and integration. Nucleic Acids Res 2013;41:D808-15. [Crossref] [PubMed]
- Zhang C, Zhang Z, Sun N, et al. Identification of a costimulatory molecule-based signature for predicting prognosis risk and immunotherapy response in patients with lung adenocarcinoma. Oncoimmunology 2020;9:1824641. [Crossref] [PubMed]
- Rahman MH, Rana HK, Peng S, et al. Bioinformatics and machine learning methodologies to identify the effects of central nervous system disorders on glioblastoma progression. Brief Bioinform 2021;22:bbaa365. [Crossref] [PubMed]
- Wu X, Lv D, Cai C, et al. A TP53-Associated Immune Prognostic Signature for the Prediction of Overall Survival and Therapeutic Responses in Muscle-Invasive Bladder Cancer. Front Immunol 2020;11:590618. [Crossref] [PubMed]
- Zong S, Zhao J, Liu L. miR-30d Induced Apoptosis by Targeting Sox4 to Inhibit the Proliferation, Invasion and Migration of Nephroblastoma. Onco Targets Ther 2020;13:7177-88. [Crossref] [PubMed]
- Shi Q, Wu H, Li Y, et al. Inhibition of Wilms' Tumor Proliferation and Invasion by Blocking TGF-β Receptor I in the TGF-β/Smad Signaling Pathway. Biomed Res Int 2020;2020:8039840. [Crossref] [PubMed]
- Li MH, Sanchez T, Yamase H, et al. S1P/S1P1 signaling stimulates cell migration and invasion in Wilms tumor. Cancer Lett 2009;276:171-9. [Crossref] [PubMed]
- Treger TD, Chowdhury T, Pritchard-Jones K, et al. The genetic changes of Wilms tumour. Nat Rev Nephrol 2019;15:240-51. [Crossref] [PubMed]
- Mahamdallie S, Yost S, Poyastro-Pearson E, et al. Identification of new Wilms tumour predisposition genes: an exome sequencing study. Lancet Child Adolesc Health 2019;3:322-31. [Crossref] [PubMed]
- Call KM, Glaser T, Ito CY, et al. Isolation and characterization of a zinc finger polypeptide gene at the human chromosome 11 Wilms' tumor locus. Cell 1990;60:509-20. [Crossref] [PubMed]
- Gessler M, Poustka A, Cavenee W, et al. Homozygous deletion in Wilms tumours of a zinc-finger gene identified by chromosome jumping. Nature 1990;343:774-8. [Crossref] [PubMed]
- Florio F, Cesaro E, Montano G, et al. Biochemical and functional interaction between ZNF224 and ZNF255, two members of the Kruppel-like zinc-finger protein family and WT1 protein isoforms. Hum Mol Genet 2010;19:3544-56. [Crossref] [PubMed]
- Charlton J, Pritchard-Jones K. WT1 Mutation in Childhood Cancer. Methods Mol Biol 2016;1467:1-14. [Crossref] [PubMed]
- Busch M, Schwindt H, Brandt A, et al. Classification of a frameshift/extended and a stop mutation in WT1 as gain-of-function mutations that activate cell cycle genes and promote Wilms tumour cell proliferation. Hum Mol Genet 2014;23:3958-74. [Crossref] [PubMed]
- Loeb DM, Evron E, Patel CB, et al. Wilms' tumor suppressor gene (WT1) is expressed in primary breast tumors despite tumor-specific promoter methylation. Cancer Res 2001;61:921-5. [PubMed]
- Cottin L, Riou J, Boyer F, et al. WT1 gene is overexpressed in myeloproliferative neoplasms, especially in myelofibrosis. Blood Cells Mol Dis 2019;75:35-40. [Crossref] [PubMed]
- Liu Z, Yamanouchi K, Ohtao T, et al. High levels of Wilms' tumor 1 (WT1) expression were associated with aggressive clinical features in ovarian cancer. Anticancer Res 2014;34:2331-40. [PubMed]
- Ohno S, Dohi S, Ohno Y, et al. Immunohistochemical detection of WT1 protein in endometrial cancer. Anticancer Res 2009;29:1691-5. [PubMed]
- Chen DA, Koehne de Gonzalez A, Fazlollahi L, et al. In situ hybridisation for albumin RNA in paediatric liver cancers compared with common immunohistochemical markers. J Clin Pathol 2021;74:98-101. [Crossref] [PubMed]
- Yoshiura K, Kanai Y, Ochiai A, et al. Silencing of the E-cadherin invasion-suppressor gene by CpG methylation in human carcinomas. Proc Natl Acad Sci U S A 1995;92:7416-9. [Crossref] [PubMed]
- von Burstin J, Eser S, Paul MC, et al. E-cadherin regulates metastasis of pancreatic cancer in vivo and is suppressed by a SNAIL/HDAC1/HDAC2 repressor complex. Gastroenterology 2009;137:361-71, 371.e1-5.
- Martínez-Estrada OM, Lettice LA, Essafi A, et al. Wt1 is required for cardiovascular progenitor cell formation through transcriptional control of Snail and E-cadherin. Nat Genet 2010;42:89-93. [Crossref] [PubMed]
- Lee E, Pandey NB, Popel AS. Lymphatic endothelial cells support tumor growth in breast cancer. Sci Rep 2014;4:5853. [Crossref] [PubMed]
- Yin M, Li X, Tan S, et al. Tumor-associated macrophages drive spheroid formation during early transcoelomic metastasis of ovarian cancer. J Clin Invest 2016;126:4157-73. [Crossref] [PubMed]
- Kim HS, Kim MS, Hancock AL, et al. Identification of novel Wilms' tumor suppressor gene target genes implicated in kidney development. J Biol Chem 2007;282:16278-87. [Crossref] [PubMed]
- Ghanem MA, Van Der Kwast TH, Den Hollander JC, et al. Expression and prognostic value of epidermal growth factor receptor, transforming growth factor-alpha, and c-erb B-2 in nephroblastoma. Cancer 2001;92:3120-9. [Crossref] [PubMed]
- Gao C, Higgins PJ, Zhang W. AQP2: Mutations Associated with Congenital Nephrogenic Diabetes Insipidus and Regulation by Post-Translational Modifications and Protein-Protein Interactions. Cells 2020;9:2172. [Crossref] [PubMed]
- Saglar Ozer E, Moeller HB, Karaduman T, et al. Molecular characterization of an aquaporin-2 mutation causing a severe form of nephrogenic diabetes insipidus. Cell Mol Life Sci 2020;77:953-62. [Crossref] [PubMed]
- Ando F, Mori S, Yui N, et al. AKAPs-PKA disruptors increase AQP2 activity independently of vasopressin in a model of nephrogenic diabetes insipidus. Nat Commun 2018;9:1411. [Crossref] [PubMed]
- Sohara E, Rai T, Yang SS, et al. Pathogenesis and treatment of autosomal-dominant nephrogenic diabetes insipidus caused by an aquaporin 2 mutation. Proc Natl Acad Sci U S A 2006;103:14217-22. [Crossref] [PubMed]
- Niu D, Bai Y, Yao Q, et al. AQP2 as a diagnostic immunohistochemical marker for pheochromocytoma and/or paraganglioma. Gland Surg 2020;9:200-8. [Crossref] [PubMed]
- Lindop GB, Duncan K, Millan DW, et al. Renin gene expression in nephroblastoma. J Pathol 1990;161:93-7. [Crossref] [PubMed]
- McKenzie KJ, Ferrier RK, Howatson AG, et al. Demonstration of renin gene expression in nephroblastoma by in situ hybridization. J Pathol 1996;180:71-3. [Crossref] [PubMed]
- Steege A, Fähling M, Paliege A, et al. Wilms' tumor protein (-KTS) modulates renin gene transcription. Kidney Int 2008;74:458-66. [Crossref] [PubMed]
- Rathmann W, Strassburger K, Bongaerts B, et al. A variant of the glucose transporter gene SLC2A2 modifies the glycaemic response to metformin therapy in recently diagnosed type 2 diabetes. Diabetologia 2019;62:286-91. [Crossref] [PubMed]
- Zhou K, Yee SW, Seiser EL, et al. Variation in the glucose transporter gene SLC2A2 is associated with glycemic response to metformin. Nat Genet 2016;48:1055-9. [Crossref] [PubMed]
- Kim YH, Jeong DC, Pak K, et al. SLC2A2 (GLUT2) as a novel prognostic factor for hepatocellular carcinoma. Oncotarget 2017;8:68381-92. [Crossref] [PubMed]
- Anborgh PH, Mutrie JC, Tuck AB, et al. Pre- and post-translational regulation of osteopontin in cancer. J Cell Commun Signal 2011;5:111-22. [Crossref] [PubMed]
- Waller AH, Sanchez-Ross M, Kaluski E, et al. Osteopontin in cardiovascular disease: a potential therapeutic target. Cardiol Rev 2010;18:125-31. [Crossref] [PubMed]
- Ye QH, Qin LX, Forgues M, et al. Predicting hepatitis B virus-positive metastatic hepatocellular carcinomas using gene expression profiling and supervised machine learning. Nat Med 2003;9:416-23. [Crossref] [PubMed]
- Thomas A, Mahantshetty U, Kannan S, et al. Expression profiling of cervical cancers in Indian women at different stages to identify gene signatures during progression of the disease. Cancer Med 2013;2:836-48. [Crossref] [PubMed]
- Cho H, Hong SW, Oh YJ, et al. Clinical significance of osteopontin expression in cervical cancer. J Cancer Res Clin Oncol 2008;134:909-17. [Crossref] [PubMed]
- Ding Z, Wu CJ, Chu GC, et al. SMAD4-dependent barrier constrains prostate cancer growth and metastatic progression. Nature 2011;470:269-73. [Crossref] [PubMed]
- Devuyst O, Pattaro C. The UMOD Locus: Insights into the Pathogenesis and Prognosis of Kidney Disease. J Am Soc Nephrol 2018;29:713-26. [Crossref] [PubMed]
- Reznichenko A, Böger CA, Snieder H, et al. UMOD as a susceptibility gene for end-stage renal disease. BMC Med Genet 2012;13:78. [Crossref] [PubMed]
- Baylarov R, Senol O, Atan M, et al. NPHS2 gene mutations in azerbaijani children with steroid-resistant nephrotic syndrome. Saudi J Kidney Dis Transpl 2020;31:144-9. [Crossref] [PubMed]
- Zaki M, El-Shaer S, Rady S, et al. Analysis of NPHS2 Gene Mutations in Egyptian Children with Nephrotic Syndrome. Open Access Maced J Med Sci 2019;7:3145-8. [Crossref] [PubMed]
- Berdeli A, Mir S, Yavascan O, et al. NPHS2 (podicin) mutations in Turkish children with idiopathic nephrotic syndrome. Pediatr Nephrol 2007;22:2031-40. [Crossref] [PubMed]
- Caridi G, Perfumo F, Ghiggeri GM. NPHS2 (Podocin) mutations in nephrotic syndrome. Clinical spectrum and fine mechanisms. Pediatr Res 2005;57:54R-61R. [Crossref] [PubMed]
- Dong L, Pietsch S, Tan Z, et al. Integration of Cistromic and Transcriptomic Analyses Identifies Nphs2, Mafb, and Magi2 as Wilms' Tumor 1 Target Genes in Podocyte Differentiation and Maintenance. J Am Soc Nephrol 2015;26:2118-28. [Crossref] [PubMed]
- Abdeljaoued S, Bettaieb I, Nasri M, et al. Overexpression of FOXM1 Is a Potential Prognostic Marker in Male Breast Cancer. Oncol Res Treat 2017;40:167-72. [Crossref] [PubMed]
- Xia JT, Wang H, Liang LJ, et al. Overexpression of FOXM1 is associated with poor prognosis and clinicopathologic stage of pancreatic ductal adenocarcinoma. Pancreas 2012;41:629-35. [Crossref] [PubMed]
- Sun H, Teng M, Liu J, et al. FOXM1 expression predicts the prognosis in hepatocellular carcinoma patients after orthotopic liver transplantation combined with the Milan criteria. Cancer Lett 2011;306:214-22. [Crossref] [PubMed]
- Liu YQ, Guo RH, Liu LK, et al. Correlation between expression of forkhead box M1 (FOXM1) and clinicopathological features and prognosis in patients with non-small cell lung cancer (NSCLC). Zhonghua Zhong Liu Za Zhi 2011;33:426-30. [PubMed]
- Poudyal D, Herman A, Adelsberger JW, et al. A novel microRNA, hsa-miR-6852 differentially regulated by Interleukin-27 induces necrosis in cervical cancer cells by downregulating the FoxM1 expression. Sci Rep 2018;8:900. [Crossref] [PubMed]
- Zang W, Wang T, Wang Y, et al. Knockdown of long non-coding RNA TP73-AS1 inhibits cell proliferation and induces apoptosis in esophageal squamous cell carcinoma. Oncotarget 2016;7:19960-74. [Crossref] [PubMed]
- Nam AS, Chaligne R, Landau DA. Integrating genetic and non-genetic determinants of cancer evolution by single-cell multi-omics. Nat Rev Genet 2021;22:3-18. [Crossref] [PubMed]
- Liu J, Qian C, Cao X. Post-Translational Modification Control of Innate Immunity. Immunity 2016;45:15-30. [Crossref] [PubMed]
(English Language Editor: B. Draper)