Pan-cancer analysis identifies CDCA3 as a novel prognostic marker associated with immune infiltration in lung adenocarcinoma through bioinformatics analysis
Introduction
Lung cancer is a malignant tumor with a high incidence and mortality rate worldwide (1,2). In China, lung cancer is the most lethal tumor for men and the second most lethal tumor for women after breast cancer. In 2019, Chinese cancer registration data showed 787 thousand newly reported cases of lung cancer, with an incidence rate of 57.26/10 million. Moreover, there were 631 thousand deaths from lung cancer, with a mortality rate of 45.87/10 million (3,4). Thus, lung cancer is a serious threat to the health of Chinese residents. Lung cancer is divided into small cell lung cancer (SCLC) and non-small cell lung cancer (NSCLC) according to different pathological types. Lung adenocarcinoma (LUAD) is the major subtype of NSCLC, accounting for more than 40% of cases (5). Moreover, most patients are in the later stage of the LUAD when diagnosed. Although developments in biomarkers, targeted gene therapy, and immuno-oncology have improved the clinical management of patients with advanced NSCLC, the 5-year survival rate of patients with lung cancer is still less than 20%, and the survival rate after metastasis is less than 3% due to blood metastasis, lymph node metastasis, and drug resistance during treatment (4,6). This undoubtedly increases the difficulty of treatment for doctors. Thus, there is an urgent need to explore the pathogenesis of LUAD and identify biomarkers with good specificity and sensitivity.
Cell division cycle-associated protein-3 (CDCA3), also known as Tom-1, is located on human chromosome 12p13. This gene has a total length of 7,271 bp and contains 6 exons. The protein contains 286 coding amino acids (7) and is one of the key proteins necessary for the activation of cyclin dependent kinase 1 (CDK1)/cyclin B and the entry of cells into mitosis. The excessive proliferation of cells is related to an abnormal cell cycle and promotes the formation of malignant tumors. Therefore, this gene has important research value in the occurrence and development of tumors. Studies have reported that CDCA3 is involved in a variety of tumors, such as hepatocellular carcinoma (HCC) (8,9), gastric cancer (GC) (10), bladder cancer (BC) (11), and prostate cancer (PC) (12) In Hepatocellular Carcinoma, researchers pointed that CDCA3 was a novel prognostic biomarker and associated with immune infiltration. However, there was few study on the role of CDCA3 could act as a prognostic biomarkers in LUAD from bioinformatics Perspective. Thus, the role of CDCA3 in LUAD was discussed here (13).
In the first stage of this study, the different expression of CDCA3 in LUAD and normal tissues was assessed by bioinformatics. Then, the correlation between the expression of CDCA3 and clinicopathological parameters was analyzed. Independent prognostic analysis, single gene enrichment analysis, and functional exploration of CDCA3 were also carried out. In addition, the correlation between CDCA3 and the tumor microenvironment was analyzed and a competing endogenous RNA (ceRNA) network was constructed using The Cancer Genome Atlas (TCGA) database. Next, the mRNA and protein expression levels of CDCA3 were preliminarily verified by clinical tissue samples, and the results were found to be consistent with bioinformatics analysis. Our findings showed that the expression of CDCA3 was upregulated in multiple tumors, including LUAD, and related to clinical parameters such as tumor stage. High expression of CDCA3 was negatively correlated with overall survival (OS) and disease-free survival (DFS), which suggested that CDCA3 expression could be used as an independent prognostic factor for LUAD. Furthermore, Cell-type Identification by Estimating Relative Subsets of RNA Transcripts (CIBERSORT) analysis found that CDCA3 was correlated with 14 immune cells, and Tumor Immune Estimation Resource (TIMER) analysis found that CDCA3 was correlated with 4 immune cells. This research explored the relationship between CDCA3 and LUAD from multiple perspectives with the aim of providing a promising therapeutic target for the prevention and treatment of LUAD. We present the following article in accordance with the REMARK reporting checklist (available at https://tcr.amegroups.com/article/view/10.21037/tcr-22-1901/rc).
Methods
Data source
This study mainly through bioinformatics analysis to download mRNA expression data and survival and clinical information of 59 normal samples and 534 LUAD samples were extracted from the TCGA database. Among the 534 LUAD samples, a total of 476 LUAD samples with both survival and clinical information were used for the survival analysis. The GSE30219, GSE18842, and GSE31210 datasets were obtained from the Gene Expression Omnibus (GEO) database. The GSE30219 dataset containing 14 normal samples and 85 LUAD samples was used for the differential expression and survival analyses. The GSE18842 dataset containing 45 normal samples and 46 LUAD samples was used for the differential expression analysis. Moreover, the GSE31210 dataset containing 226 LUAD samples was used for the survival analysis.
The expression of CDCA3 in LUAD
The TIMER database (http://timer.cistrome.org/) and the Gene Expression Profiling Interactive Analysis (GEPIA) database (http://gepia.cancer-pku.cn/) were used to search the mRNA expression of CDCA3 in different cancers. Furthermore, the expression of CDCA3 was identified in normal and LUAD samples in the TCGA database and the GSE30219 and GSE18842 datasets.
Correlation between CDCA3 expression and clinicopathological characteristics
LUAD patients in the TCGA database were separated into 2 groups according to age (<60 and ≥60), gender (female and male), stage (stage I-II and stage III-IV), N (N0 and N+), and smoking status (<40 and ≥40). The expression of CDCA3 was then compared in these groups.
Independent prognostic analysis
OS and the DFS were investigated with respect to CDCA3 gene expression in the TCGA database and the GSE30219 and GSE31210 datasets. OS and DFS curves were drawn using the Kaplan-Meier method. To determine whether CDCA3 expression was significant with respect to other clinical characteristics, independent prognostic analysis was conducted using the TCGA database and the GSE30219 and GSE31210 datasets. The potential prognostic factors were screened by Cox analysis. Correlations between CDCA3 expression and survival and other clinical characteristics of patients were confirmed by using multivariate Cox analysis.
Single gene GSEA of CDCA3
Gene Set Enrichment Analysis (GSEA) was performed to analyze the Gene Ontology (GO) functions and Kyoto Encyclopedia of Genes and Genomes (KEGG) pathways enrichment of CDCA3 using the “clusterProfiler” package in R (The R Foundation for Statistical Computing, Vienna, Austria) (14). The correlation coefficients between the expression levels of all genes and the CDCA3 gene were calculated, and conducted GSEA according to the correlation coefficient.
Relationship between CDCA3 expression and immunity
The “estimate” (15) installation package in R language was used to evaluate the immune score, tumor purity, and stromal score of LUAD samples from the TCGA database. In addition, the differences between immune cell subtypes were explored using the CIBERSORT algorithm. Next, the relationship between the level of CDCA3 expression and immunity was explored in TIMER2.0 (http://timer.cistrome.org/).
CeRNA network construction
The differentially expressed mRNAs (DEmRNAs), long noncoding RNAs (DElncRNAs), and microRNAs (DEmiRNAs) were identified between LUAD and normal samples from the TCGA database by using the “limma” package (16) in R with |log2FC| >1 and P<0.05. Next, the target miRNAs of CDCA3 were predicted using the miRWalk database (http://mirwalk.umm.uni-heidelberg.de/). In accordance with ceRNA theory, miRNAs that negatively correlated with the expression trend of the CDCA3 were screened out. Then, the Starbase website (http://starbase.sysu.edu.cn/) was used to predict the target lncRNAs of miRNA. Similarly, lncRNAs that negatively correlated with the expression trend of the above miRNAs were selected. Finally, a ceRNA regulatory network was constructed using the miRNAs, lncRNAs, and mRNAs and was visualized using Cytoscape software (The Cytoscape Consortium).
Experimental verification
The study was conducted in accordance with the Declaration of Helsinki (as revised in 2013). The study was approved by the ethics committee of The Second Affiliated Hospital of Kunming Medical University (No. KYCS2022090), and informed consent was obtained from all patients. A total of 33 LUAD tissues and 33 normal tissues, which obtained from Department of thoracic surgery, the Second Affiliated Hospital of Kunming Medical University and were used for the immunohistochemistry (IHC) staining, mRNA expression, and western blotting (WB) of CDCA3. For IHC, LUAD tissue sections were detected with two-step detection kit (PV-9000, ZSGB-Bio, Beijing, China). Next, total RNA and complementary DNA (cDNA) synthesis were performed according to the manufacturer’s instructions. Gene expression was calculated by 2-∆∆CT method. The forward primer for CDCA3 was 5'-AGTACACGAGCAAGAAGCCATT-3', and the reverse primer for CDCA3 was 5'-GCAACAGGAGGATCAGACAGA-3'. For WB, whole cell lysates were prepared by RIPA buffer, and then proteins were separated with sodium dodecyl sulphate-polyacrylamide gel electrophoresis (SDS-PAGE) gel and transferred to polyvinylidene difluoride (PVDF) membranes. The proteins were incubated overnight with primary antibodies CDCA3 (1:100 dilution, bs-7894R, LS-Bio, Beijing, China) and glyceraldehyde 3-phosphate dehydrogenase (GAPDH; 1:100 dilution, bs-13282R, FineTest, Wuhan, China). Next, secondary antibodies (1:100 dilution, #31001, PIERCE, USA) were incubated, and the chemiluminescent signals were detected using an ECL-Plus detection kit (#131023-60-4, BOC Sciences, USA).
Statistical analyses
The CDCA3 expression of cancer and adjacent tissues was analyzed using the TIMER and GEPIA databases with P values and fold changes. The correlation between CDCA3 expression and clinicopathological characteristics was compared using the chi-square test or the chi-square goodness of fit test. Analysis of OS and DFS was performed using Kaplan-Meier curves, log-rank test.
The factors with (P<0.05) in the univariate Cox regression analysis results were added in the multivariate Cox regression analysis. The factors appearing in the multivariate Cox regression results were considered to be an independent prognostic factors.
In this study, all boxplots were drawn by the R package “ggplot2” (17), and significance was calculated by the wilcox.test function in R. P values <0.05 were considered statistically significant.
Results
The expression of CDCA3 in LUAD
To explore the correlation between the expression of CDCA3 and cancer, CDCA3 expression was estimated in different tumors and adjacent normal tissues. In the TIMER database, CDCA3 expression was higher in bladder urothelial carcinoma (BLCA), breast invasive carcinoma (BRCA), cervical squamous cell carcinoma (CESC), cholangiocarcinoma (CHOL), colon adenocarcinoma (COAD), esophageal carcinoma (ESCA), glioblastoma multiforme (GBM), head and neck squamous cell carcinoma (HNSC), HPV-positive head and neck squamous cell carcinoma (HNSC-HPV+), kidney renal clear cell carcinoma (KIRC), kidney renal papillary cell carcinoma (KIRP), liver hepatocellular carcinoma (LIHC), LUAD, lung squamous cell carcinoma (LUSC), pancreatic adenocarcinoma (PAAD), prostate adenocarcinoma (PRAD), rectum adenocarcinoma (READ), stomach adenocarcinoma (STAD), thyroid carcinoma (THCA), and uterine corpus endometrial carcinoma (UCEC) tumors than in normal samples (Figure 1A). In the GEPIA database, CDCA3 expression was higher in adrenocortical carcinoma (ACC), BLCA, BRCA, CESC, COAD, diffuse large B-cell lymphoma (DLBC), ESCA, GBM, HNSC, LIHC, LUAD, LUSC, ovarian cancer (OV), PAAD, READ, skin cutaneous melanoma (SKCM), STAD, thymoma (THYM), UCEC, and uterine carcinosarcoma (UCS) tumors than in normal samples (Figure 1B). Conversely, in the GEPIA database, CDCA3 expression was lower in acute myeloid leukemia (LAML) tumors than in normal samples (Figure 1B). Subsequently, CDCA3 expression was estimated between LUAD and normal samples in the TCGA database and the GSE30219 and GSE18842 datasets, and the expression levels of CDCA3 were found to be significantly higher in LUAD compared to those in normal samples (Figure 1C-1E).
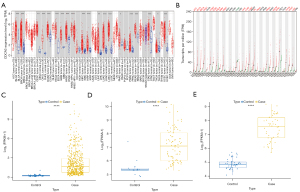
Correlation between CDCA3 expression and clinicopathological characteristics
To investigate the correlation between CDCA3 expression and clinicopathological characteristics, TCGA samples were separated into 2 groups according to clinicopathological characteristics. As shown in Figure 2, CDCA3 was significantly correlated with age (Figure 2A), gender (Figure 2B), smoking status (Figure 2C), stage (Figure 2D), and N (Figure 2E). However, no correlation was found between the expression of CDCA3 and T (Figure 2F) or M (Figure 2G).
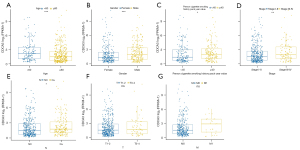
CDCA3 is an independent prognostic risk factor in LUAD
To further assess the prognostic potential of CDCA3 for LUAD, Kaplan-Meier survival analysis was performed using the TCGA database and the GSE30219 and GSE31210 datasets. According to the median expression of CDCA3 in LUAD, patients with LUAD were separated into high and low CDCA3 expression groups. The Kaplan-Meier plot showed that higher CDCA3 expression was related to poorer OS and DFS in TCGA OS P=0.002 (Figure 3A) and DFS P=0.014 (Figure 3B); GSE30219 OS P<0.001 (Figure 3C) and DFS P<0.001 (Figure 3D); and GSE31210 OS P<0.001 (Figure 3E) and DFS P<0.001 (Figure 3F). Moreover, an independent prognostic analysis of OS and DFS was conducted using the TCGA database and the GSE30219 and GSE31210 datasets to determine whether CDCA3 was an independent prognostic factor for LUAD. The results of the independent prognostic analysis of OS (Figure 4) and DFS (Figure 5) showed that CDCA3 could act as an independent prognostic factor. Univariate Cox analysis indicated that CDCA3 could serve as an independent prognostic factor for LUAD OS and DFS based on TCGA (Figures 4A,5A), GSE30219 (Figures 4C,5C), and GSE31210 (Figures 4E,5E). Multivariate Cox analysis showed that CDCA3 may be an independent prognostic factor for LUAD OS based on TCGA (Figure 4B) and for LUAD OS and DFS based on GSE30219 (Figures 4D,5D) and GSE31210 (Figures 4F,5F). Multivariate Cox analysis showed that CDCA3 expression may not be an independent prognostic factor for DFS based on TCGA (Figure 5B).
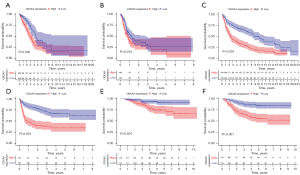
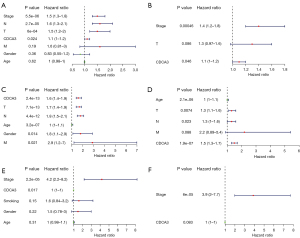
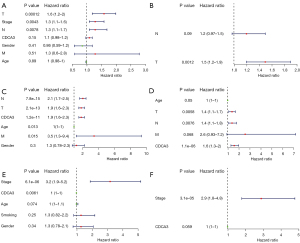
Single gene GSEA of CDCA3
GSEA was performed to explore the GO and KEGG enrichment of CDCA3. The top 10 significantly enriched GO terms were cell cycle checkpoint signaling, cell cycle G2 M phase transition, chromosome localization, chromosome segregation, DNA conformational change, DNA dependent DNA replication, DNA geometric change, DNA reorganization, and DNA repair (Figure 6A). The top 10 KEGG pathways were cell cycle, proteasome, spliceosome, DNA replication, oxidative phosphorylation, pyrimidine metabolism, Parkinson’s disease, oocyte meiosis, cell adhesion molecules (CAMs), and ASTHMA (Figure 6B). In these KEGGs, cell adhesion molecules (CAMs) and ASTHMA showed a downward trend, which means that the down regulated genes played a leading role in these two pathways.
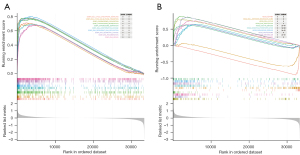
Relationship between CDCA3 expression and immunity
As shown in Figure 7, CDCA3 expression was negatively correlated with ImmuneScore (P=4.31e-07) (Figure 7A), StromalScore (P=5.72e-13) (Figure 7B), and EstimateScore (P=5.31e-11) (Figure 7C), but positively correlated with TumorPurity (P=4.83e-10) (Figure 7D). Furthermore, the landscape of LUAD immune infiltrations was obtained from 520 samples after filtering in the TCGA database (Figure 8A). As shown in Figure 8B,8C, plasma cells were negatively correlated with M2 macrophages. Additionally, CIBERSORT analysis found that among 22 types of immune cells, 14 immune cells were significantly different between the high and low CDCA3 expression groups (Figure 8D). Moreover, TIMER analysis showed that CDCA3 expression was significantly correlated with 4 types of cells, they were B cells, CD4+ T cells, macrophages, and dendritic cells (Figure 8E).
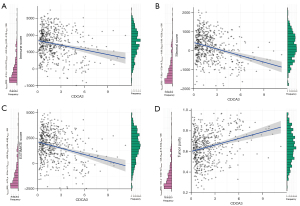
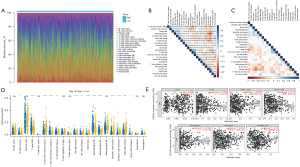
CeRNA network construction
Overall, 1969 DEmRNAs, 38 DElncRNAs, and 434 DEmiRNAs were selected between normal and LUAD samples in the TCGA database (Figure 9A-9C). Finally, a ceRNA network was constructed with CDCA3, 18 lncRNAs, and 7 miRNAs. The ceRNA network contained 26 nodes and 32 edges (Figure 9D).
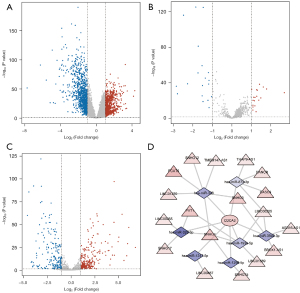
Experimental verification
To verify the reliability of the above results, 33 LUAD tissues and 33 normal tissues were used to test CDCA3 expression using IHC, real-time quantitative reverse transcriptase polymerase chain reaction (qRT-PCR), and WB. IHC and WB showed that the expression levels of CDCA3 in LUAD tissues were significantly higher than those in normal tissues (Figure 10A,10B). Furthermore, the mRNA and protein levels of CDCA3 were significantly higher in LUAD than in normal tissues (Figure 10C,10D). These experimental results were consistent with those of the bioinformatic analyses.
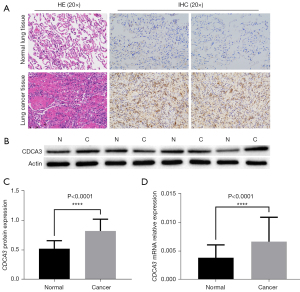
Discussion
LUAD is the most common pathological type of lung cancer. Tumor occurrence is inseparable from genetic factors, such as the molecular genetic basis of malignant transformation and tumor progression. In the last several years, studies on genes and proteins related to LUAD have reported valuable information about the occurrence of LUAD. Research has shown that CDCA3 is a significant oncogene in various tumors. In BC, specific types of transcription factors can activate the expression of CDCA3, which then promotes the proliferation of BC cells, such as kinesin family member 22 (KIF22) and KIF4A. Similarly, research has shown that CDCA3 promotes cell cycle progression, accelerates cell growth, and promotes BC cell migration (11,18,19). Moreover, CDCA3 is a biomarker of chemosensitivity in NSCLC. A high expression level of CDCA3 improves sensitivity to platinum chemotherapy, and upregulation of CDCA3 expression may enhance the sensitivity of tyrosine kinase inhibitor (TKI) in epidermal growth factor receptor (EGFR)-mutant lung cancer (13,20). KIRP and breast cancer present the same finding. CDCA3 not only participates in cell cycle regulation but also promotes doxorubicin resistance in breast cancer and enhances sensitivity to platinum-based chemotherapy in NCSLC (20-22). However, controversial outcomes still exist; for example, knockdown of CDCA3 in NCSLC reduces the proliferation of NCSLC cells by stagnating them in G2/M phase (23). In colorectal cancer (CRC) and PC, CDCA3 induces cell cycle arrest in the G1 phase (24,25). In addition, CDCA3 also affects the progression of GC through epigenetics. DNA hypomethylation promotes the expression of CDCA3 and the invasion and metastasis of GC cells by regulating the binding of Sp1 transcription factor (Sp1) to the CDCA3 promoter. Similarly, another study found that the overexpression of CDCA3 leads to the continuous activation of the Ras pathway and induces the abnormal activation of the extracellular signal-regulated kinase (ERK)/mitogen-activated protein kinase (MAPK) signaling pathway, which leads to the occurrence of GC (10,26).
The clinical application of immunotherapy has shifted the focus of tumor treatment from tumor cells to the tumor microenvironment (27). Based on CIBERSORT bioinformatics analysis, our study found that 14 of 22 types of immune cells had significant differences in the high and low CDCA3 expression groups, including memory B cells, activated mast cells (P<0.05), follicular helper T cells, activated NK cells (P<0.01), CD8 T cells, CD4 memory resting T cells, CD4 memory activated T cells, resting NK cells, monocytes, M0 macrophages, M1 macrophages, resting dendritic cells, activated dendritic cells, and resting mast cells (P<0.001). Previous studies have also reported a correlation between CDCA3 and immune cell infiltration in HCC involving B cells, neutrophils, monocytes, tumor-associated macrophages (TAMs), M1 and M2 macrophages, CD4+ T cells, CD8+ T cells, NK cells, and dendritic cells (8). In the TIMER database, we found a significant correlation between the expression of CDCA3 and 4 types of immune cells, namely B cells, CD4+ T cells, macrophages, and dendritic cells (P values were 7.17e-06, 1.55e-02, 1.31e-03, and 9.95e-03, respectively). This was consistent with our previous CIBERSORT results. Moreover, CDCA3 is also regulated by noncoding RNA, which affects the occurrence of tumors. CeRNA is a key regulatory mechanism (28). LncRNA or circular RNA (circRNA) compete for binding to miRNA, and then the transcription level of target genes regulated by miRNA are upregulated. Many studies have reported on this mechanism, such as circRNA-miRNA-CDCA3 or LncRNA-miRNA-CDCA3 (29). CirRNA hsa_circ_101555, hsa_circ_0005728, and Circ_0001421 via the miR-145-5p/CDCA3, miR-512-3p/CDCA3, and miR-4677-3p/CDCA3 axis is a ceRNA network that boosts proliferation and migration in HCC, BC, and LC (21,30,31). LncRNA LINC00707 accelerates the development and progression of BC by targeting miR-145/CDCA3 (22). Our study predicted the target non-coding RNAs, including 18 lncRNAs and 7 miRNAs, and then constructed a ceRNA network. Next, we verified the binding between target genes and the screened RNA through a series of experiments to explore the mechanism.
GO functional analysis indicated that CDCA3 may participate in processes such as cell division, apoptosis, DNA damage, and DNA repair and may participate in tumor progression through cell cycle G2 M phase transition, as shown in Figure 6A. This result is consistent with previous report (23). KEGG pathway analysis indicated that CDCA3 was involved in cell cycle, proteasome, spliceosome, and DNA replication pathways. Studies have shown that CDCA3 activates the nuclear factor kappa B (NF-KB)/cyclin D1 signaling pathway and promotes cancer cell proliferation in CRC and PC (25). In breast cancer and pancreatic cancer, CDCA3 plays a critical role in tumor progression through activation of the phosphoinositide 3-kinases (PI3K)/AKT pathway (7,32). The above pathways were included in our KEGG analysis results, which are consistent with previous reports.
In this study, we explored the expression of CDCA3 in LUAD from multiple angles through a bioinformatics analysis. First, we investigated the correlation between this gene and clinicopathological parameters. Next, we analyzed the independent prognosis and tumor microenvironment of LUAD and constructed a ceRNA network of CDCA3. Moreover, we preliminarily verified the bioinformatics results using relevant experiments. Generally speaking, our findings are consistent with previous results on CDCA3 in tumors and provide a theoretical basis for further exploration of the role of CDCA3 in the occurrence of LUAD. However, our study had some limitations. The function of CDCA3 requires further exploration in follow-up experiments in vivo and in vitro to confirm its role in the preliminary screening and early diagnosis of LUAD.
Conclusions
In brief, our results showed that the expression of CDCA3 was upregulated in a variety of cancers, including LUAD, and was significantly correlated with several clinical parameters. The high expression of CDCA3 was negatively correlated with OS and DFS. Univariate and multivariate Cox regression analysis indicated that CDCA3 could be used as an independent prognostic factor of LUAD. In addition, we also analyzed the relationship between CDCA3 and the tumor immune microenvironment and found that its expression was related to certain immune cells. The above results might provide a theoretical basis for further study.
Acknowledgments
Funding: This work was supported by the National Key Research and Development Program of China (No. 2017YFC0907902), the Natural Science Foundation of Yunnan Province (No. 2017FA039), and the Natural Science Foundation of Yunnan Province (No. 2017FE468-214).
Footnote
Reporting Checklist: The authors have completed the REMARK reporting checklist. Available at https://tcr.amegroups.com/article/view/10.21037/tcr-22-1901/rc
Data Sharing Statement: Available at https://tcr.amegroups.com/article/view/10.21037/tcr-22-1901/dss
Conflicts of Interest: All authors have completed the ICMJE uniform disclosure form (available at https://tcr.amegroups.com/article/view/10.21037/tcr-22-1901/coif). The authors have no conflicts of interest to declare.
(English Language: C. Gourlay)
Ethical Statement: The authors are accountable for all aspects of the work in ensuring that questions related to the accuracy or integrity of any part of the work are appropriately investigated and resolved. The study was conducted in accordance with the Declaration of Helsinki (as revised in 2013). The study was approved by the Ethics Committee of The Second Affiliated Hospital of Kunming Medical University (No. KYCS2022090) and informed consent was obtained from all patients.
Open Access Statement: This is an Open Access article distributed in accordance with the Creative Commons Attribution-NonCommercial-NoDerivs 4.0 International License (CC BY-NC-ND 4.0), which permits the non-commercial replication and distribution of the article with the strict proviso that no changes or edits are made and the original work is properly cited (including links to both the formal publication through the relevant DOI and the license). See: https://creativecommons.org/licenses/by-nc-nd/4.0/.
References
- Hu J, Chen Y, Li X, et al. THUMPD3-AS1 Is Correlated With Non-Small Cell Lung Cancer And Regulates Self-Renewal Through miR-543 And ONECUT2. Onco Targets Ther 2019;12:9849-60. [Crossref] [PubMed]
- Zou Y, Jing C, Liu L, et al. Serum microRNA-135a as a diagnostic biomarker in non-small cell lung cancer. Medicine (Baltimore) 2019;98:e17814. [Crossref] [PubMed]
- Miller KD, Nogueira L, Mariotto AB, et al. Cancer treatment and survivorship statistics, 2019. CA Cancer J Clin 2019;69:363-85. [Crossref] [PubMed]
- Gao S, Li N, Wang S, et al. Lung Cancer in People’s Republic of China. J Thorac Oncol 2020;15:1567-76. [Crossref] [PubMed]
- Hutchinson BD, Shroff GS, Truong MT, et al. Spectrum of Lung Adenocarcinoma. Semin Ultrasound CT MR 2019;40:255-64. [Crossref] [PubMed]
- Bade BC, Dela Cruz CS. Lung Cancer 2020: Epidemiology, Etiology, and Prevention. Clin Chest Med 2020;41:1-24. [Crossref] [PubMed]
- Xing C, Wang Z, Zhu Y, et al. Integrate analysis of the promote function of Cell division cycle-associated protein family to pancreatic adenocarcinoma. Int J Med Sci 2021;18:672-84. [Crossref] [PubMed]
- Wang Z, Chen S, Wang G, et al. CDCA3 Is a Novel Prognostic Biomarker Associated with Immune Infiltration in Hepatocellular Carcinoma. Biomed Res Int 2021;2021:6622437. [Crossref] [PubMed]
- Wu B, Huang Y, Luo Y, et al. The diagnostic and prognostic value of cell division cycle associated gene family in Hepatocellular Carcinoma. J Cancer 2020;11:5727-37. [Crossref] [PubMed]
- Zhang Y, Yin W, Cao W, et al. CDCA3 is a potential prognostic marker that promotes cell proliferation in gastric cancer. Oncol Rep 2019;41:2471-81. [Crossref] [PubMed]
- Li S, Liu X, Liu T, et al. Identification of Biomarkers Correlated with the TNM Staging and Overall Survival of Patients with Bladder Cancer. Front Physiol 2017;8:947. [Crossref] [PubMed]
- Gu P, Yang D, Zhu J, et al. Bioinformatics analysis of the clinical relevance of CDCA gene family in prostate cancer. Medicine (Baltimore) 2022;101:e28788. [Crossref] [PubMed]
- Sahin KB, Shah ET, Ferguson GP, et al. Elevating CDCA3 Levels Enhances Tyrosine Kinase Inhibitor Sensitivity in TKI-Resistant EGFR Mutant Non-Small-Cell Lung Cancer. Cancers (Basel) 2021;13:4651. [Crossref] [PubMed]
- Yu G, Wang LG, Han Y, et al. clusterProfiler: an R package for comparing biological themes among gene clusters. OMICS 2012;16:284-7. [Crossref] [PubMed]
- Du J, Tao Q, Liu Y, et al. Assessment of the targeted effect of Sijunzi decoction on the colorectal cancer microenvironment via the ESTIMATE algorithm. PLoS One 2022;17:e0264720. [Crossref] [PubMed]
- Smyth GK. Linear models and empirical bayes methods for assessing differential expression in microarray experiments. Stat Appl Genet Mol Biol 2004;3:Article3.
- Villanueva RAM, Chen ZJ. ggplot2: Elegant Graphics for Data Analysis (2nd ed.). Measurement: Interdisciplinary Research and Perspectives 2019;17:160-7.
- Li K, Li S, Tang S, et al. KIF22 promotes bladder cancer progression by activating the expression of CDCA3. Int J Mol Med 2021;48:211. [Crossref] [PubMed]
- Zheng P, Wu K, Gao Z, et al. KIF4A promotes the development of bladder cancer by transcriptionally activating the expression of CDCA3. Int J Mol Med 2021;47:99. [Crossref] [PubMed]
- Kildey K, Gandhi NS, Sahin KB, et al. Elevating CDCA3 levels in non-small cell lung cancer enhances sensitivity to platinum-based chemotherapy. Commun Biol 2021;4:638. [Crossref] [PubMed]
- Dou D, Ren X, Han M, et al. CircUBE2D2 (hsa_circ_0005728) promotes cell proliferation, metastasis and chemoresistance in triple-negative breast cancer by regulating miR-512-3p/CDCA3 axis. Cancer Cell Int 2020;20:454. [Crossref] [PubMed]
- Gao T, Ji Y. Long Noncoding RNA LINC00707 Accelerates Tumorigenesis and Progression of Bladder Cancer via Targeting miR-145/CDCA3 Regulatory Loop. Urol Int 2021;105:891-905. [Crossref] [PubMed]
- Adams MN, Burgess JT, He Y, et al. Expression of CDCA3 Is a Prognostic Biomarker and Potential Therapeutic Target in Non-Small Cell Lung Cancer. J Thorac Oncol 2017;12:1071-84. [Crossref] [PubMed]
- Qian W, Zhang Z, Peng W, et al. CDCA3 mediates p21-dependent proliferation by regulating E2F1 expression in colorectal cancer. Int J Oncol 2018;53:2021-33. [Crossref] [PubMed]
- Gu P, Zhang M, Zhu J, He X, Yang D. Suppression of CDCA3 inhibits prostate cancer progression via NF-κB/cyclin D1 signaling inactivation and p21 accumulation. Oncol Rep 2022;47:42. [Crossref] [PubMed]
- Yu J, Hua R, Zhang Y, et al. DNA hypomethylation promotes invasion and metastasis of gastric cancer cells by regulating the binding of SP1 to the CDCA3 promoter. J Cell Biochem 2020;121:142-51. [Crossref] [PubMed]
- Nadal E, Massuti B, Dómine M, et al. Immunotherapy with checkpoint inhibitors in non-small cell lung cancer: insights from long-term survivors. Cancer Immunol Immunother 2019;68:341-52. [Crossref] [PubMed]
- Fei X, Hu C, Wang X, et al. Construction of a Ferroptosis-Related Long Non-coding RNA Prognostic Signature and Competing Endogenous RNA Network in Lung Adenocarcinoma. Front Cell Dev Biol 2021;9:751490. [Crossref] [PubMed]
- Yang J, Hao R, Zhang Y, et al. Construction of circRNA-miRNA-mRNA network and identification of novel potential biomarkers for non-small cell lung cancer. Cancer Cell Int 2021;21:611. [Crossref] [PubMed]
- Gu X, Zhang J, Ran Y, et al. Circular RNA hsa_circ_101555 promotes hepatocellular carcinoma cell proliferation and migration by sponging miR-145-5p and regulating CDCA3 expression. Cell Death Dis 2021;12:356. [Crossref] [PubMed]
- Zhang K, Hu H, Xu J, et al. Circ_0001421 facilitates glycolysis and lung cancer development by regulating miR-4677-3p/CDCA3. Diagn Pathol 2020;15:133. [Crossref] [PubMed]
- Du WW, Yang W, Li X, et al. The Circular RNA circSKA3 Binds Integrin β1 to Induce Invadopodium Formation Enhancing Breast Cancer Invasion. Mol Ther 2020;28:1287-98. [Crossref] [PubMed]