Novel insight on predicting prognosis of gastric cancer based on inflammation
Introduction
Gastric cancer (GC), one of the most common digestive malignancies, is the third leading cause of malignancy-related deaths (1), and stomach adenocarcinoma (STAD) is its most common pathological subtype (2). Although early diagnosis, surgical resection, and adjuvant therapy have improved patient survival, the prognosis for advanced GC remains poor.
The role of inflammation in tumor initiation and malignant transformation has gained considerable research interest (3). The inflammatory microenvironment not only initiates and promotes oncogenic transformation, but also supports tumor progression, especially in GC (4,5). Chronic inflammation is associated with all processes of GC development, driving histopathologic changes from chronic gastritis to gastric atrophy, intestinal metaplasia, dysplasia, and GC finally (6). The hallmarks of cancer-related inflammation are mediating cancer progression via cytokines, chemokines, and innate immune cells. Inflammation has been added as the seventh hallmark of cancer, and inflammatory mediators can, therefore, be used prognostic markers (7). However, no previous studies have investigated the utility of inflammation-related genes (IRGs) in predicting the prognosis of GC patients after curative resection.
In this study, we aimed to identify an IRG related survival model to predict poor clinical outcomes of GC patients that may facilitate the evaluation of patient prognosis and the relationship between the tumor immune microenvironment and cancer stem cells and facilitate therapeutic decision-making. We present the following article in accordance with the TRIPOD reporting checklist (available at https://tcr.amegroups.com/article/view/10.21037/tcr-22-1042/rc).
Methods
Data collection
Figure 1 depicts the study workflow. The RNA-seq data from The Cancer Genome Atlas (TCGA) database (https://tcga-data.nci.nih.gov/tcga/) was used as the training set which comprised 375 STAD and 32 non-tumor tissue samples. The baseline information of STAD samples is summarized in Table S1. The independent datasets GSE62254 and GSE15459, downloaded from the Gene Expression Omnibus (GEO) database (https://www.ncbi.nlm.nih.gov/geo/), collectively comprising data of 500 STAD patients which were used as one of the test sets for constructing the gene signature (Figure 2A). We excluded the patients who were followed up <90 days in order to minimize the impact of the death from non-tumor-related reasons. The IRG sets (34 in total) were obtained from the gene sets database of Gene Ontology (GO) pathways at the gene set enrichment analysis (GSEA) website (https://www.gsea-msigdb.org/gsea/index.jsp) (8) (Table S2). The study was conducted in accordance with the Declaration of Helsinki (as revised in 2013).
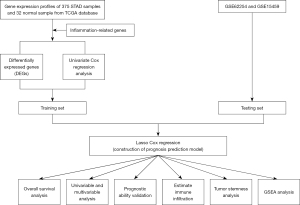
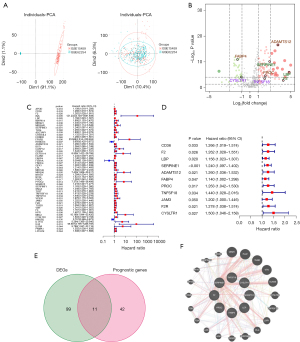
Screening for differentially expressed genes (DEGs)
The “limma” package were used to identify the DEGs between tumor and non-tumor samples by the cut-off criteria, which were set as false discovery rate (FDR)-adjusted P<0.05 and log2|fold change (FC)| >1.
Cox univariate survival analysis
We also use the “survival” package to perform Cox univariate survival analysis and the threshold was set as P<0.05. Hub IRGs, differentially expressed survival-related IRGs, which were used to analyze the gene-gene interaction by GeneMANIA database (https://genemania.org/).
Establishment of the risk model and determination of the prognostic value of the risk score
Least absolute shrinkage and selection operator (LASSO) regression was performed to ensure that there was no overfitting in the model based on the RNA-seq of hub IRGs, and a model with 8 selected hub IRGs was constructed to establish the risk score. The “glmnet” package was used to perform LASSO regression. The risk score for STAD samples were calculated in both training and test sets. Then, the STAD samples were divided into two subtypes, high- and low-risk subtypes. We constructed the ROC curves to evaluate the prediction efficiency of the risk model. To perform principal component analysis (PCA) and t-distribution stochastic neighbor embedding (t-SNE) analysis, the “Rtsne” package was used.
Independent prognostic factor analysis
Risk score and clinical data were integrated to analyze independent prognostic factors. Both univariate and multivariate analysis were conducted to evaluate the prognostic value of the model and clinical parameters. We performed analysis of variance (ANOVA) to compare clinical outcomes of patients with risk scores.
Determination of associations between immune infiltration and the prognostic model
We used single-sample GSEA (ssGSEA) algorithm (9) to quantify the tumor-infiltrating immune cells, immune-related pathways and immune-related functions in GC. Furthermore, we also used ESTIMATE algorithm to assess the stromal scores and immune scores and Spearman correlation analysis to identify the relationships with the model.
Correlation of cancer stemness with the risk model
In order to measure stem-cell-like features of tumor cells, we extracted tumor stemness features from transcriptomic and epigenetic of GC samples (10). Spearman correlation analysis was performed to identify the correlation between cancer stemness and model.
Correlation analysis on checkpoint gene markers and immunotherapy response
The correlationship between prognostic model and checkpoint gene expression was examined by Spearman correlation analysis. Checkpoint gene markers were acquired by a literature search (11-17). The web of Tumor Immune Dysfunction and Exclusion (TIDE) was used to predict the immunotherapy response.
GSEA
We downloaded hallmark gene sets “h.all.v7.2.symbols.gmt” from the GSEA database to analyze the two subtypes by the software of GSEA (version 4.1.0). The gene sets with FDR P<0.05 were considered to display significant differences.
Statistical analysis
All statistical analysis were performed with R software version 4.0.2. Statistical significance was set at P<0.05. The DEGs were identified by “limma” package and the statistical significance was set at adjusted P<0.05 and log2|FC| >1. By R package “survminer”, the optimal cutoffs for Kaplan-Meier survival analysis were determined. Independent t-test was employed to compare continuous variables among low- and high-risk groups, the chi-square test was utilized to test categorical data and Mann-Whitney test was employed to examine differences in immune infiltration between two groups.
Results
IRG identification
In total, 34 inflammation-related pathways were obtained in the GO pathway analysis at the GSEA website. We identified 525 IRGs related to these 34 pathways after removing duplicates (available online: https://cdn.amegroups.cn/static/public/tcr-22-1042-1.docx).
Gene expression and survival analysis
In total, 110 DEGs were identified, including 77 upregulated and 33 downregulated genes (Figure 2B). We identified 53 genes with prognostic value by using Cox univariate analysis (Figure 2C,2D). Of these, 11 were identified as DEGs (Figure 2E). The correlation network generated based on the RNA-seq profiles of survival-related genes is summarized in Figure 2F.
Construction of prognostic model using LASSO regression
The risk score was defined by coefficients which obtained after the LASSO regression (Figure 3A,3B), and the formula used was as follows: risk score = (0.03610 × F2 expression) + (0.06059 × LBP expression) + (0.13449 × SERPINE1 expression) + (0.07010 × ADAMTS12 expression) + (0.00392 × FABP4 expression) + (0.15878 × PROC expression) + (0.09276 × TNFSF18 expression) + (0.22928 × CYSLTR1 expression). We sorted the STAD samples into one low-risk and two high-risk subtypes based on the median risk score. Furthermore, no matter in the training or test sets, Kaplan-Meier analysis revealed that the high-risk score subtypes had a significantly shorter survival (Figure 3C,3D). ROC curves are shown in Figure 3E,3F. The areas under the ROC curve (AUCs) of the model for the prediction of 1-, 3-, and 5-year survival were 0.63, 0.68 and 0.68 in training sets, respectively and 0.65, 0.60, and 0.60 in the test set, respectively, indicating the potential robustness of this model in predicting the survival of STAD patients. STAD patients were sorted into two different risk groups with a relatively clear resolution, as shown in Figure 3G,3H.
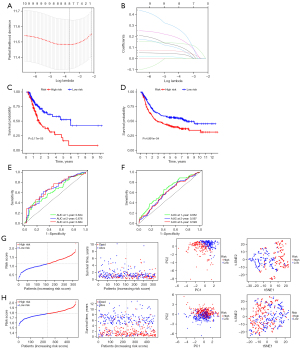
Integrated analysis of risk score and clinical parameters of STAD patients
By combining clinical data and risk scores of STAD patients in TCGA database, we obtained the comprehensive data which include 304 patients. Three factors (age, stage, and risk score) were filter from five parameters (age, gender, grade, stage, and risk score) by univariate Cox regression analysis, which correlated with the OS (P<0.005) in the training set (Figure 4A,4B). Furthermore, two factors (stage and risk score) were filtered in the test set (Figure 4C,4D). ANOVA results illustrated that the two groups were significantly different with respect to the clinical stage and survival status (Figure 4E). Moreover, patients with GC in the stage I were significantly related to a lower risk score in the TCGA and GEO database (Figure 5A,5B).
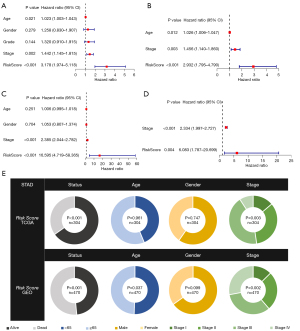
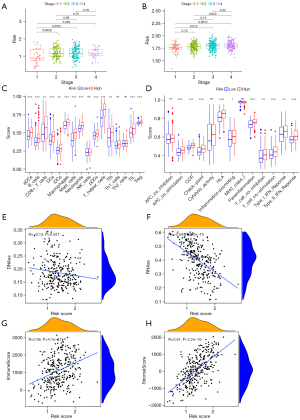
Relationship between risk score and tumor immune microenvironment
After ssGSEA, the activity or enrichment levels of immune cells, functions, or pathways in two groups in training sets were quantified. Compared with the low-risk group, the abundance of activated dendritic cells (aDCs), CD8+ T cells, macrophages, T follicular helper (Tfh), regulatory T cells (Tregs) and other 11 kinds of immune cells was significantly higher in the high-risk group (Figure 5C). In terms of immune function, antigen-presenting cell (APC) co-stimulation, check-point, Inflammation-promoting and other 6 kinds of immune function, were significantly enriched in in the high-risk group (Figure 5D).
Association of risk score with tumor stemness and immune score
RNA stemness score (RNAss) and DNA stemness score (DNAss) based on mRNA expression and DNA methylation pattern were used to evaluate tumor stemness. The model was negatively correlated with RNAss and DNAss (P<0.005), especially with RNAss (r=−0.54, Spearman, P<0.0001) (Figure 5E,5F).
We further explored the relationship between the expression levels of the genes in the model with the presence of the major components of infiltrating stromal cells and immune cells, which represented by stromal scores and immune score by ESTIMATE algorithm. The model shows positively correlationship with stromal scores (r=0.36, Spearman, P<0.001) and immune scores (r=0.61, Spearman, P<0.001) (Figure 5G,5H).
Relationship between risk score and immune checkpoints
We identified 47 checkpoints by literature search. In the Spearman analysis, the risk score correlated with 9 checkpoints (CD200, CD276, CD86, HAVCR2, LAIR1, NRP1, PDCD1LG2, TNFRSF4, and TNFSF4; r>0.3, Spearman, P<0.05) (Figure 6A,6B). According to TIDE algorithm, the high-risk group showed higher dysfunction, exclusion, and TIDE scores (P<0.001) (Figure 7A-7C).
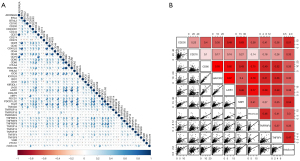
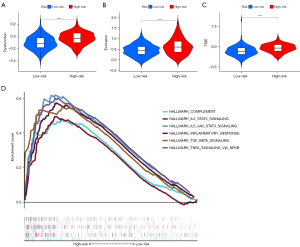
GSEA
GSEA results demonstrated that genes in the high-risk group were significant highly enriched in 16 pathways, and 6 of these were immune-related pathways (Figure 7D). Similarly, 5 pathways were significantly activated in the low-risk group.
Discussion
In this study, we established an inflammation-related prognostic model for GC. Inflammation contributes to aberrant biological behaviors, for example, uncontrolled cell growth, proliferation as well as invasion and angiogenesis. Chronic inflammation is a high-risk factor for progression from gastritis to GC. GC is a highly heterogeneous malignant tumor (18), and its molecular characteristics are also related to tumor biological behaviors. Thus, independent prognostic molecular markers or risk models related to inflammation in GC are urgently needed.
Many prognostic models have been previously constructed to predict the outcomes of GC patients, but few have been effective. Wan et al. established a prognostic model based on the immune microenvironment, and Wei et al. developed a lipid metabolism-related model for GC (19,20). These studies used various gene sets to construct the models but did not consider inflammatory factors. Overall, few studies have established an inflammation-related prognostic model. Although the preoperative inflammation-based Glasgow prognostic score (GPS) composed of the C-reactive protein (CRP) and albumin is a simple and useful prognostic factor for patients with GC (21), however, GPS is based on preoperative index but not postoperative specimen. Therefore, comprehensive consideration of GPS and the models based on postoperative gene expression which can improve assessment of prognosis and guide treatment of patients with GC in a routine clinical work. In this study, we first investigated 525 IRGs by a literature research and screened 53 inflammation-related DEGs with prognostic value. From these 53 genes, an 8-gene signature was identified using the LASSO regression and TCGA database and used to establish the inflammation-related model that could predict the prognosis of GC patients; the model was then validated by GEO database. Our analysis also revealed that patients with high-risk score had poor OS and changed with the stage. Therefore, the new model could help to identify high-risk patients and formulate efficient therapeutic plans for GC patients.
After constructing the risk assessment model, receiver operating characteristic (ROC) curve analysis, risk score distribution, PCA, and t-SNE analysis were used to analyze the prognostic ability of the model in both sets. STAD patients were sorted into high- and low-risk groups by the model. The risk score was then combined with the clinical parameters in the test and training sets, respectively. Risk score and stage were identified as independent prognostic factors in the both sets.
Immune checkpoint inhibitors (ICIs) exert antitumor effects by reversing tumor-evading immune surveillance. In clinical practice, the high expression of immune checkpoints has been used as an index to predict the immune response after immunotherapy. Thus, the relationship between the risk score and the immune checkpoint genes is worth exploring. Of the 47 checkpoints, 9 showed highly significant correlation with risk score and could potentially be used for predicting immunotherapy response. Interestingly, both TNFSF4 and its receptor TNFRSF4 showed significant correlation with the model. We further used the TIDE algorithm to predict immunotherapy response; this algorithm integrates data on two tumor immune escape mechanisms: the prevention of T cell infiltration in tumors with low cytotoxic T lymphocytes (CTL) level and induction of T cell dysfunction in tumors with high infiltration of CTL. The high-risk group had a higher TIDE score than the low. Furthermore, according to the dysfunction and exclusion scores, the high-risk group also showed a higher degree of T cell dysfunction and exclusion. All the results reflect the strong activation of immune escape mechanisms in the high-risk group.
Some biomarkers included in our model have been reported in other cancers, and most are already known to play a role in inflammation and immune infiltration. F2, LBP, ADAMTS12, and FABP4 are known to be involved in immune and inflammatory responses. For example, F2, also known as PT or RPRGL, promotes M1 macrophage polarization by regulating thrombin expression (22). It is associated with the expression of classical pro-inflammatory markers. LBP, which can act on different kinds of immune cells, is also involved in the regulation of immune and inflammatory responses by promoting the activation of macrophages and NK cells (23). FABP4 may be a prognostic biomarker in STAD and its expression correlated with immune infiltration, especially in M2 macrophages (24). By contrast, other target genes promote tumor progression in GC or other cancers. SERPINE1 has been previously identified as a marker of unfavorable prognosis in GC (25,26) and shown to facilitate tumor cell proliferation, migration, and invasion (27). ADAMTS12 is a potential biomarker for GC tumor microenvironment (TME) conversion, which influenced the immune activity of GC TME by macrophage and neutrophils (28)].
Our study has the following limitations. First, we did not analyze the underlying biological mechanisms. A functional analysis is therefore needed to obtain mechanistic details. Second, although the model was validated using GSE62254 and GSE15459, studies with larger populations and longer follow-up duration are needed to confirm the effectiveness and robustness of the model. However, this is the first study to establish inflammation-related prognostic scores in GC with a high predictive value, despite the limitations mentioned above. Moreover, our novel IRG signature could be beneficial for developing individualized treatments as well as improving the OS of GC patients.
Conclusions
Our study provided a good prognostic risk model for GCs patients. This model was validated in several datasets for the reliability and effectiveness. Further, this model was also involved in immunotherapy and which can bring us a novel insights of GCs patients.
Acknowledgments
Funding: This study was supported in part by
Footnote
Reporting Checklist: The authors have completed the TRIPOD reporting checklist. Available at https://tcr.amegroups.com/article/view/10.21037/tcr-22-1042/rc
Conflicts of Interest: All authors have completed the ICMJE uniform disclosure form (available at https://tcr.amegroups.com/article/view/10.21037/tcr-22-1042/coif). The authors have no conflicts of interest to declare.
Ethical Statement: The authors are accountable for all aspects of the work in ensuring that questions related to the accuracy or integrity of any part of the work are appropriately investigated and resolved. This study was conducted in accordance with the Declaration of Helsinki (as revised in 2013).
Open Access Statement: This is an Open Access article distributed in accordance with the Creative Commons Attribution-NonCommercial-NoDerivs 4.0 International License (CC BY-NC-ND 4.0), which permits the non-commercial replication and distribution of the article with the strict proviso that no changes or edits are made and the original work is properly cited (including links to both the formal publication through the relevant DOI and the license). See: https://creativecommons.org/licenses/by-nc-nd/4.0/.
References
- Siegel RL, Miller KD, Jemal A. Cancer statistics, 2019. CA Cancer J Clin 2019;69:7-34. [Crossref] [PubMed]
- Ajani JA, Lee J, Sano T, et al. Gastric adenocarcinoma. Nat Rev Dis Primers 2017;3:17036. [Crossref] [PubMed]
- Ni Z, Huang C, Zhao H, et al. PLXNC1: A Novel Potential Immune-Related Target for Stomach Adenocarcinoma. Front Cell Dev Biol 2021;9:662707. [Crossref] [PubMed]
- Mantovani A, Allavena P, Sica A, et al. Cancer-related inflammation. Nature 2008;454:436-44. [Crossref] [PubMed]
- Bernard V, Semaan A, Huang J, et al. Single-Cell Transcriptomics of Pancreatic Cancer Precursors Demonstrates Epithelial and Microenvironmental Heterogeneity as an Early Event in Neoplastic Progression. Clin Cancer Res 2019;25:2194-205. [Crossref] [PubMed]
- Coussens LM, Werb Z. Inflammation and cancer. Nature 2002;420:860-7. [Crossref] [PubMed]
- Mantovani A. Cancer: Inflaming metastasis. Nature 2009;457:36-7. [Crossref] [PubMed]
- Subramanian A, Tamayo P, Mootha VK, et al. Gene set enrichment analysis: a knowledge-based approach for interpreting genome-wide expression profiles. Proc Natl Acad Sci U S A 2005;102:15545-50. [Crossref] [PubMed]
- Barbie DA, Tamayo P, Boehm JS, et al. Systematic RNA interference reveals that oncogenic KRAS-driven cancers require TBK1. Nature 2009;462:108-12. [Crossref] [PubMed]
- Malta TM, Sokolov A, Gentles AJ, et al. Machine Learning Identifies Stemness Features Associated with Oncogenic Dedifferentiation. Cell 2018;173:338-54.e15. [Crossref] [PubMed]
- Tivol EA, Borriello F, Schweitzer AN, et al. Loss of CTLA-4 leads to massive lymphoproliferation and fatal multiorgan tissue destruction, revealing a critical negative regulatory role of CTLA-4. Immunity 1995;3:541-7. [Crossref] [PubMed]
- Seliger B, Marincola FM, Ferrone S, et al. The complex role of B7 molecules in tumor immunology. Trends Mol Med 2008;14:550-9. [Crossref] [PubMed]
- Harjunpää H, Guillerey C. TIGIT as an emerging immune checkpoint. Clin Exp Immunol 2020;200:108-19. [Crossref] [PubMed]
- Khan M, Arooj S, Wang H NK. Cell-Based Immune Checkpoint Inhibition. Front Immunol 2020;11:167. [Crossref] [PubMed]
- van der Leun AM, Thommen DS, Schumacher TN. CD8+ T cell states in human cancer: insights from single-cell analysis. Nat Rev Cancer 2020;20:218-32. [Crossref] [PubMed]
- Veldman J, Visser L, Berg AVD, et al. Primary and acquired resistance mechanisms to immune checkpoint inhibition in Hodgkin lymphoma. Cancer Treat Rev 2020;82:101931. [Crossref] [PubMed]
- Zhang C, Liu Y, Targeting NK. Cell Checkpoint Receptors or Molecules for Cancer Immunotherapy. Front Immunol 2020;11:1295. [Crossref] [PubMed]
- Zhang Y, Ma S, Niu Q, et al. Features of alternative splicing in stomach adenocarcinoma and their clinical implication: a research based on massive sequencing data. BMC Genomics 2020;21:580. [Crossref] [PubMed]
- Wan L, Tan N, Zhang N, et al. Establishment of an immune microenvironment-based prognostic predictive model for gastric cancer. Life Sci 2020;261:118402. [Crossref] [PubMed]
- Wei XL, Luo TQ, Li JN, et al. Development and Validation of a Prognostic Classifier Based on Lipid Metabolism-Related Genes in Gastric Cancer. Front Mol Biosci 2021;8:691143. [Crossref] [PubMed]
- McMillan DC. The systemic inflammation-based Glasgow Prognostic Score: a decade of experience in patients with cancer. Cancer Treat Rev 2013;39:534-40. [Crossref] [PubMed]
- López-Zambrano M, Rodriguez-Montesinos J, Crespo-Avilan GE, et al. Thrombin Promotes Macrophage Polarization into M1-Like Phenotype to Induce Inflammatory Responses. Thromb Haemost 2020;120:658-70. [Crossref] [PubMed]
- Abella V, Scotece M, Conde J, et al. Leptin in the interplay of inflammation, metabolism and immune system disorders. Nat Rev Rheumatol 2017;13:100-9. [Crossref] [PubMed]
- Guo Y, Wang ZW, Su WH, et al. Prognostic Value and Immune Infiltrates of ABCA8 and FABP4 in Stomach Adenocarcinoma. Biomed Res Int 2020;2020:4145164. [Crossref] [PubMed]
- Xu B, Bai Z, Yin J, et al. Global transcriptomic analysis identifies SERPINE1 as a prognostic biomarker associated with epithelial-to-mesenchymal transition in gastric cancer. PeerJ 2019;7:e7091. [Crossref] [PubMed]
- Li L, Zhu Z, Zhao Y, et al. FN1, SPARC, and SERPINE1 are highly expressed and significantly related to a poor prognosis of gastric adenocarcinoma revealed by microarray and bioinformatics. Sci Rep 2019;9:7827. [Crossref] [PubMed]
- McCann JV, Xiao L, Kim DJ, et al. Endothelial miR-30c suppresses tumor growth via inhibition of TGF-β-induced Serpine1. J Clin Invest 2019;129:1654-70. [Crossref] [PubMed]
- Hou Y, Xu Y, Wu D. ADAMTS12 acts as a tumor microenvironment related cancer promoter in gastric cancer. Sci Rep 2021;11:10996. [Crossref] [PubMed]