Identification of prognostic miRNA-mRNA regulatory network in the progression of HCV-associated cirrhosis to hepatocellular carcinoma
Introduction
Hepatocellular carcinoma (HCC) is one of the primarily prevalent and fatal malignancies in the world today (1). Most patients with HCC do not benefit from surgical intervention, including resection, transplantation, and percutaneous ablation (2). Generally, the occurrence of HCC passes through three development stages: hepatitis, cirrhosis, and finally HCC (3). At each stage, gene mutations may occur resulting in additional circumvention of cell proliferation regulation and promoting HCC development (4). Researchers have found that monitoring HCC in patients with cirrhosis can improve patients' survival (5). In clinical practice, biomarkers indicating the progression of cirrhosis to HCC are very limited. Hence, it is essential to explore potential biomarkers that predict a high risk of HCC evolution in the cirrhotic stage.
Multiple pathogenic factors such as hepatitis C virus (HCV), parasites, primary biliary cholangitis, hepatitis B virus, and autoimmune hepatitis are contributing to the occurrence of HCC. In 2015, about 399,000 people with HCV chronic infection died from cirrhosis and HCC (6). Despite the promising efficacy of novel combination therapies based on direct-acting antivirals (DAAs), HCV-associated deaths are rising each year due to the high risk of HCC progression in patients with cirrhosis (7). Cirrhosis is a vital course in the progression of chronic HCV infection to HCC. Approximately 85–95% of HCC patients have suffered from cirrhosis before evolving into HCC, and about 2–4% of cirrhosis progressed into HCC each year (8).
MicroRNAs (miRNAs) are non-coding RNA with 22 nucleotides (9) and negatively regulate gene expression by inhibiting protein translation (10). The production of miRNAs usually passes through several stages, including primary miRNAs, precursor miRNAs, duplexes, and mature miRNAs (11). Notably, different miRNAs may originate from a single transcript and share similar biological functions. Different miRNAs may be responsible for regulating the same gene (12). Most miRNAs could target a variety of genes to be involved in diverse biological processes (13). Dysregulated miRNAs affect the progression of various cancers such as breast cancer, colorectal cancer, and HCC (14,15). For instance, miR-708 and miR-370 target specific genes to regulate HCC development (16), and circulatory miR-155 may serve as a potential non-invasive indicator of HCV-associated HCC (17). Besides, the expression levels of miRNAs could be regulated by transcription factors (18,19). To our knowledge, the miRNAs regulatory network in the progression of cirrhosis to HCC has rarely been reported.
Here, we explore the miRNAs and genes with altered expression levels between HCV-associated cirrhosis and HCC. A regulatory network consisting of intersection genes and their corresponding miRNAs was constructed. PABPC1 (Polyadenylate-binding protein 1), SLC2A9 (solute carrier gene family 2, member 9), and UHRF1 (ubiquitin-like with PHD and ring finger domains 1) constitute a prognostic model predicting the progression of HCV-associated cirrhosis to HCC. UHRF1 acts as an oncogene that promotes the proliferation and migration ability of HCC cells. We present the following article in accordance with the TRIPOD reporting checklist (available at https://tcr.amegroups.com/article/view/10.21037/tcr-22-989/rc).
Methods
GEO datasets
Datasets that included miRNA or mRNA expression profiles of clinical HCV-associated cirrhosis and HCC samples from the Gene Expression Omnibus database (GEO) were screened. Only those datasets that included more than 20 clinical tissue samples were collected. The GSE40744 dataset owned the miRNA expression profiles of 18 HCV-associated cirrhosis tissue samples and 9 HCV-associated HCC tissue samples. The GSE6764 dataset contained the mRNA expression information of 3 HCV-infected cirrhotic tissue samples and 35 HCV-infected HCC tissue samples. The GSE15654 dataset with the prognosis information of 216 HCV-associated early-stage cirrhosis patients was downloaded.
Identification of DE-miRNAs
To find out DE-miRNAs between cirrhosis and HCC among HCV-infected patients, the GSE40744 dataset was analyzed using the “Limma” package in R from the Bioconductor project. |Log2 fold change (FC)| >1.5 and P value <0.05 were selection criteria. Associated codes with R packages “pheatmap” and “ggplot2” were added to R software. The names of the miRNAs were converted using miRBase (http://www.mirbase.org/). The potential downstream genes of DE-miRNAs were identified by the miRNet online tool. The potential transcription factors were identified by the FunRich software.
Identification of DEGs
The DEGs between cirrhosis and HCC among HCV-infected patients in the GSE6764 dataset were identified by the “Limma” package. The “ggplot2” and “pheatmap” packages were applied to conduct Volcano plots and Heatmap, respectively. The criteria were P value <0.05 and |log2FC| >1. The DEGs were overlapped with potential target genes to select intersection ones using the Venn diagram. The construction of the miRNA-mRNA regulatory network was processed in Cytoscape software.
Gene Ontology (GO) and Kyoto Encyclopedia of Genes and Genomes (KEGG) analysis
GO and KEGG analysis on intersection genes were conducted using the “clusterProfiler” package in R. P value <0.05 was considered statistically different.
Prognostic model
The GSE15654 dataset was selected to identify risk genes in the progression of HCV-associated cirrhosis to HCC. Patients were equally distributed to two subsets at random. Firstly, in the training set, univariate Cox regression was performed on intersection genes to explore potential risk genes, which were then evaluated using LASSO regression and multivariate Cox regression analysis by the “glmnet” package. Next, the risk score for each patient was calculated (risk score = C1*expGene1 + C2*expGene2 + … + Cn*expGeneN, in which “exp” is the expression level of the gene, while Cn is the regression coefficient of the corresponding gene). Based on the median risk score, cirrhotic sufferers were partitioned into two groups using the R package (“surviminer”), and Kaplan-Meier analysis was executed to compare the HCC development time. The predictive value of the risk model was estimated by receiver operating characteristic (ROC) analysis using the “survivalROC” package in R. The cumulative risk of HCC development among cirrhotic patients showed the predictive capacity of the model. To verify the reliability and validity of the prognostic model, the same criteria and methods were used in the test set. P value <0.05 was considered statistically significant.
Clinical patients’ specimens
Volunteers were recruited from clinical patients undergoing surgical resection at Zhongshan Hospital of Fudan University in 2020, and 10 pairs of frozen HCV-associated cirrhosis and HCC tumor tissue samples were collected. The study fully complied with the Declaration of Helsinki (as revised in 2013), and was approved and supervised by the Ethics Committee of Zhongshan Hospital, Fudan University while it was conducted (Approval ID: 2019, No. 29). All patients provided informed consent. Patients included in this study meet the following criteria: (I) concomitant chronic HCV infection; (II) no other type of malignancy; (III) no other serious fatal disease; (IV) have not received a liver transplant.
The quantitative real-time polymerase chain reaction (qRT-PCR)
The qPCR experiment was carried out as previously reported (20). Complied with the manufacturer’s specifications (Vazyme, China), total RNA of patient liver tissues was extracted and mRNA reverse transcription was implemented. Primers of PABPC1, UHRF1, SLC2A9, and GAPDH were synthesized by Tingke Bio (China). The primers for PABPC1: Forward 5'-CCCAGCGCCCCCAGCTAC-3' and Reverse 5'-CACGTTCCGCGTCCGCC-3'. The primers for UHRF1: Forward 5'-CGACGGAGCGTACTCCCTAG-3' and Reverse 5'-TCATTGATGGGAGCAAAGCA-3'. The primers for SLC2A9: Forward 5'-TCAGGACAAGATCCCATACA-3' and Reverse 5'-TCTGACTGTTGGAAGAACTC-3'. The primers for GAPDH: Forward 5'-TGACTTCAACAGCGACACCCA-3' and Reverse 5'-CACCCTGTTGCTGTAGCCAAA-3'. The cDNA mixtures were amplified for 40 cycles in the ABI7500 real-time PCR detection system. Subsequently, the relative gene expression levels were normalized to GAPDH. MicroRNAs in the patient's liver tissues were isolated and converted to cDNA using the TaqMan MicroRNA Reverse Transcription Kit (Thermo Fisher, USA). The stem-loop primers and probes for miR-200b-3p, miR-200c-3p, miR-10a-5p, and RNU6B (housekeeping control) were purchased from Thermo Fisher (Assay ID: 002251, 002300, 000387, and 001093, respectively). The stem-loop primers for miR-466 were purchased from Ribobio (China). The qRT-PCR detection for miRNA was performed according to the TaqMan MicroRNA assay kit.
Cell lines
The cell lines including Huh7, HepG2, and 293T were obtained from the Shanghai Cell Bank of the Chinese Academy of Sciences and stored in liquid nitrogen in our laboratory. These cells were cultured in DMEM (C11995500BT, Gibco, USA) medium supplemented with 10% fetal bovine serum (10099-141, Gibco, USA) and 1% penicillin/streptomycin (TMS-AB2-C, Sigma, Germany). They all grew in good condition with normal morphology.
Western blot
Total cellular proteins were extracted using cell lysate buffer (HY-K1001, MedChemExpress, USA) at 4 ℃ for 30 min, then mixed with protein loading buffer and cooked at 100 ℃ for 10 min. Separation was performed by electrophoresis using 8% SDS-PAGE at a constant pressure of 90 V for 2 h (1658033, Bio-Rad, USA). The proteins were transferred to PVDF membranes (3010040001, Sigma, Germany) at a constant current of 350 mA for 90 min and then incubated with 1:1,000 diluted UHRF1 antibody (ab153972, Abcam, UK) and 1:5,000 diluted GAPDH antibody (5174, Cell Signaling, USA) at 4 ℃ for 12 h. After washing three times with TBST for 15 minutes each time, the membranes were incubated with 1:10,000 diluted secondary antibody (98164, Cell Signaling Technology, USA) for 1 h at room temperature. Finally, exposure and imaging were performed (Tanon, China).
Cell transfection and infection
The full-length cDNA of UHRF1 was assembled into an empty pCDH-CMV-MCS-EF1-GreenPuro (SBI) lentiviral vector to construct a plasmid overexpressing UHRF1. The shRNA (5'-GCCAGGTGGTCATGCTCAACTACAA-3') targeting UHRF1 was recombined into the pLKO.1 vector to construct a plasmid that knocked down UHRF1. When the density of 293T cells reached approximately 70%, the recombinant plasmid mixed with psPAX2 and pMD2.G was transfected into the cells using a transfection reagent (L3000-015, Invitrogen, USA). Mature infectious lentivirus in the cell supernatant was collected after 3 days. The lentivirus overexpressing UHRF1 or knocking down UHRF1 were then infected with HepG2 cells and Huh7 cells, respectively, and screened with puromycin (A1113802, Gibco, USA). The target monoclonal cell lines were expanded and tested by western blot.
CCK-8 assay
The HepG cells overexpressing UHRF1 and Huh7 cells knocking down UHRF1 and the respective control cells were inoculated into 96-well plates at a cell density of 103 cells/well. After the cells adhered to the wall, a mixture of CCK-8 (HY-K0301, MedChemExpress, USA) and DMEM was added, then incubated at 37 ℃ for 30 min. The absorbance of each group was observed at 450 nm (Bio-Rad, USA). Five wells were set up in each group and observed continuously for 6 days.
Colony formation assay
HCC cells were seeded in 6-well plates (800 cells/well) and cultured at 37 ℃ for 2 weeks, then unattached cells were removed with PBS. Cells were fixed with paraformaldehyde for 20 min, then colored with crystal violet (Solarbio, China) for 20 min, followed by washed with PBS, air-dried, and finally photographed.
Cell scratch assay
Cells in the logarithmic growth phase were inoculated into 6-well plates. When the cells were relatively dense, a sterile tip of 20 µL size was used to draw a straight line in a direction perpendicular to the cell layer to ensure that the width of the wound keep the same in each well. The detached cells were washed away with PBS, and the width the wound were observed and recorded (IX51, Olympus, Japan). The cells were then incubated at 37 ℃ for 48 h before photographing.
Statistical analysis
In this study, P<0.05 was regarded as statistical significance. The results of all functional experiments were analyzed by Student’s t-test and were represented as mean ± SD (GraphPad prism 8). Each experiment was repeated three times. All bioinformatics analyses unless otherwise noted were conducted in R software (version 3.5.3).
Results
39 DE-miRNAs were identified between HCV-associated cirrhosis and HCC
The detailed study protocol is shown in Figure 1. Taking |Log2FC| >1.5 and P value <0.05 as the selection criteria, 39 differentially expressed miRNAs (DE-miRNAs) between HCV-associated cirrhosis and HCC were identified within the GSE40744 dataset using “Limma” package. In HCV-associated HCC, there are 8 upregulated miRNAs (miR-130b-3p, miR-1269a, miR-224-5p, miR-452-5p, miR-224-3p, miR-184, miR-552, and miR-466) and 31 downregulated miRNAs (miR-130a-3p, miR-4269, miR-214-5p, miR-199a-5p, miR-200c-3p, miR-199b-3p, miR-199a-3p, miR-424-3p, miR-125a-5p, miR-886-3p, miR-139-3p, miR-10a-5p, miR-23a-5p, miR-29b-3p, miR-203, miR-214-3p, miR-503-5p, miR-27a-5p, miR-150-5p, miR-138-5p, miR-139-5p, miR-424-5p, miR-30a-3p, miR-31-5p, miR-451a, miR-200b-5p, miR-200a-3p, miR-134, miR-200b-3p, miR-708-5p, and miR-127-3p). These DE-miRNAs are drawn using Heatmap and Volcano plot (Figure 2A,2B). In addition, the top 10 potential upstream transcription factors of DE-miRNAs were identified by FunRich software (Figure 2C,2D).
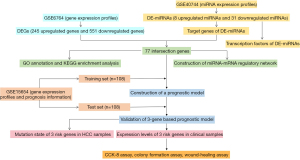
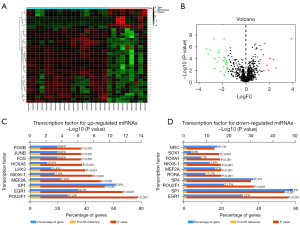
796 DEGs were identified between HCV-associated cirrhosis and HCC
The mRNA expression profiles of the GSE6764 dataset were performed to distinguish differentially expressed genes (DEGs) between HCV-associated cirrhosis and HCC with the criteria of |Log2FC| >1 and P value <0.05. 796 DEGs were identified, including 245 upregulated genes and 551 downregulated genes in HCV-associated HCC (Figure 3A,3B). The downstream target genes (1,080 downregulated and 3,744 upregulated) of 39 DE-miRNAs were predicated using the miRNet tool and then overlapped with the 796 DEGs. Twenty-five downregulated intersection genes and 52 upregulated intersection genes were found (Figure 3C,3D). The association between intersection genes and their corresponding DE-miRNAs was visualized by Cytoscape platform software to establish a main miRNA-mRNA regulatory network in which discrete miRNAs and genes were excluded (Figure 4A).
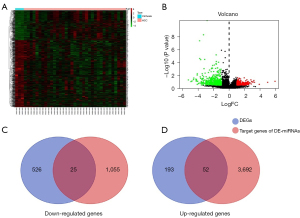
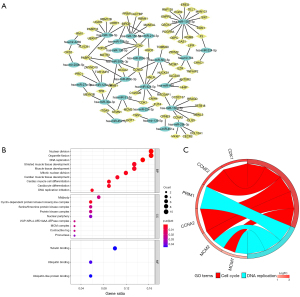
GO and KEGG enrichment analysis
GO analysis on 77 intersection genes was conducted for three categories. The enriched GO functions of these genes included regulation of nuclear division, organelle fusion, and DNA replication in biological processes, midbody and cyclin-dependent protein kinase holoenzyme complex in cellular components, and tubulin binding in molecular function (Figure 4B). KEGG analysis was performed and 77 intersection genes chiefly participated in the pathways of cell cycle and DNA replication (Figure 4C).
Construction of a prognostic model to indicate the risk of HCC development in patients with HCV-associated cirrhosis
To construct a prognostic model in the progression of HCV-associated cirrhosis to HCC, all patients (n=216) with early-stage cirrhosis in the GSE15654 dataset were randomly distributed to two subsets: 108 patients in the training set and 108 patients in the test set. There were scarcely any different clinical characteristics between the training set and the test set (Table 1). In the training set, we found that three risk genes, including PABPC1, SLC2A9, and UHRF1, were strongly associated with the occurrence of HCC after performing a univariate Cox regression analysis of 77 intersection genes. Then, these three risk genes were subjected to the least absolute shrinkage and selection operator (LASSO) (Figure 5A) and multivariate Cox regression analysis (Table 2). PABPC1, SLC2A9, and UHRF1 all retained independent prognostic values and could build up a prognostic model based on the regression coefficients. The risk score of each patient was worked out [risk score = (−0.69138*exp PABPC1) + (0.344947*exp UHRF1) + (−0.48720*exp SLC2A9)]; −0.69138, 0.344947, and −0.48720 are the regression coefficients of PABPC1, UHRF1, and SLC2A9, respectively; exp means the expression level of each gene). The median risk score of patients in the training set was 0.903, which served as a cut-off point for dividing the training set into two subgroups: 54 patients with higher risk scores and 54 patients with lower risk scores (Figure 5B). Later, Heatmap was drawn in terms of the expression levels of PABPC1, UHRF1, and SLC2A9 (Figure 5C). Patients with higher risk scores were more likely to develop HCC (Figure 5D). Most importantly, the area under the ROC curve (AUC) in 1, 3, 5, and 7 years were calculated and the values were 0.738, 0.815, 0.742, and 0.698, respectively (Figure 5E).
Table 1
Clinical characteristics | Training set (N=108) (%) | Testing set (N=108) (%) | Entire set (N=216) (%) | P value |
---|---|---|---|---|
Presence of varices | 0.4031 | |||
Yes | 24 (22.22) | 28 (27.18) | 52 (24.66) | |
No | 84 (77.78) | 75 (72.82) | 159 (75.34) | |
Bilirubin (mg/dL) | 0.4142 | |||
<1.0 | 57 (52.78) | 51 (47.22) | 108 (50.00) | |
≥1.0 | 51 (47.22) | 57 (52.78) | 108 (50.00) | |
Platelet | 0.0405 | |||
<100,000/mm3 | 57 (52.78) | 42 (38.89) | 99 (45.83) | |
≥100,000/mm3 | 51 (47.22) | 66 (61.11) | 117 (54.17) | |
Status | 0.2373 | |||
Death | 37 (34.26) | 29 (26.85) | 66 (30.56) | |
Alive | 71 (65.74) | 79 (73.15) | 150 (69.44) | |
Child | 0.7677 | |||
Yes | 32 (29.63) | 34 (31.48) | 66 (30.56) | |
No | 76 (70.37) | 74 (68.52) | 150 (69.44) | |
Liver cancer | 0.0538 | |||
Yes | 39 (36.11) | 26 (24.07) | 65 (30.09) | |
No | 69 (63.89) | 82 (75.93) | 151 (69.91) |
The Mann-Whitney U test, t-test, Fisher’s exact test, or Chi-square test was performed to compare the differences according to appropriate variables. P<0.05 is statistically significant.
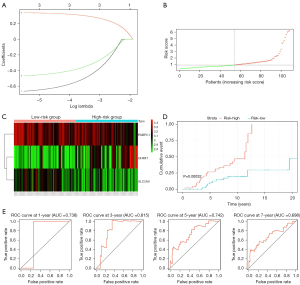
Table 2
ID | coef | HR | HR.95L | HR.95H | P value |
---|---|---|---|---|---|
PABPC1 | −0.69138 | 0.50088 | 0.27147 | 0.92414 | 0.02694 |
UHRF1 | 0.344947 | 1.41191 | 1.08456 | 1.83806 | 0.01037 |
SLC2A9 | −0.48720 | 0.61433 | 0.3893 | 0.98556 | 0.04335 |
PABPC1, Polyadenylate-binding protein 1; UHRF1, ubiquitin-like with PHD and ring finger domains; SLC2A9, solute carrier gene family 2, member 9; HR, hazard ratio.
Remarkable predictive efficacy of the prognostic model
To test the validity of the prognostic model consisting of PABPC1, SLC2A9, and UHRF1 for indicating the progression of HCV-associated cirrhosis to HCC, the same risk score calculation formula and grouping thresholds (risk score =0.903) as in the training set were used to divide the test set. 45 patients were enrolled in a low-risk group and 63 patients were enrolled in a high-risk group. Heatmap was drawn in terms of the expression levels of PABPC1, UHRF1, and SLC2A9 in the test set (Figure 6A). The cirrhotic patients with a higher risk score were more inclined to evolve into HCC (Figure 6B). AUC values in 1, 3, 5, and 7 years were 0.877, 0.683, 0.647, and 0.688, respectively (Figure 6C). Univariate and multivariate Cox regression analyses performed on the whole risk model demonstrated that it can be a prognostic factor for indicating the possibility of developing HCC in patients with cirrhosis (Figure 6D,6E).
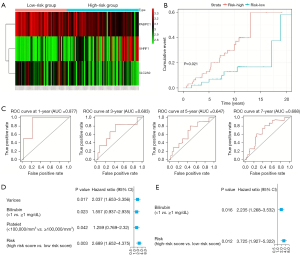
Mutations of risk genes in clinical HCC samples
The genetic mutations of PABPC1, UHRF1, and SLC2A9 in 353 clinical HCC samples were searched using the cBioPortal database. PABPC1 showed a 9% change, including 29 cases of amplification, 1 case of deep deletion, and 1 case of mutation. SLC2A9 showed a 0.6% change, including 1 case of amplification and 1 case of mutation. UHRF1 showed a 0.8% change, with 2 cases of amplification and 1 case of deep deletion (Figure 7).
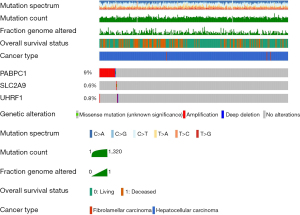
Expression levels of risk genes and their corresponding miRNAs in clinical HCV-associated cirrhosis and HCC samples
Ten pairs of clinical HCV-associated cirrhosis and HCC tissue samples were extracted to detect the expression levels of PABPC1, SLC2A9, and UHRF1 and their corresponding miRNAs by qRT-PCR. There were few significant differences in demographic and clinical characteristics such as age, sex, body mass index, liver-related biochemical parameters, HCV RNA titers, and HCV genotype between patients with HCV-associated cirrhosis and HCC, except for total bilirubin, aspartate aminotransferase (AST), and alpha-fetoprotein (AFP) (Table 3). According to the analysis of GSE40744 and GSE6764 datasets, miR-200b-3p, miR-200c-3p and miR-10a-5p targeted PABPC1, miR-466 targeted SLC2A9, and miR-10a-5p also targeted UHRF1. The mRNA levels of PABPC1 and UHRF1 were obviously increased while the mRNA level of SLC2A9 was distinctly decreased in HCV-associated HCC samples compared to that in HCV-associated cirrhotic samples (Figure 8A-8C). Correspondingly, the expression levels of miR-200b-3p, miR-200c-3p, and miR-10a-5p were downregulated while the expression level of miR-466 was upregulated in HCV-associated HCC samples (Figure 8D-8G).
Table 3
Characteristics | Cirrhosis (n=10) | HCC (n=10) | P value |
---|---|---|---|
Age (years) | 63±8.5 | 65±7.8 | n.s |
Gender (male/female) | 6/4 | 7/3 | n.s |
BMI (kg/m2) | 24.6±4.5 | 25.3±4.2 | n.s |
Diabetes mellitus (n) | 2 | 3 | n.s |
ALT (U/L) | 57.3±22.3 | 59.5±26.7 | n.s |
AST (U/L) | 50.2±24.5 | 57.9±20.9 | 0.02 |
ALP (U/L) | 179.5±40.1 | 231.4±57.9 | n.s |
Total bilirubin (mg/dL) | 1.15±0.75 | 1.20±0.83 | 0.01 |
Albumin (g/L) | 35.9±7.6 | 37.1±8.8 | n.s |
AFP (ng/mL) | 68±45.7 | 387±87.3 | 0.03 |
HCV RNA (log IU/mL) | 6.1±0.73 | 6.2±0.67 | n.s |
HCV genotype (n) | |||
1b | 6 | 7 | n.s |
2 | 2 | 2 | n.s |
3 | 1 | 1 | n.s |
6 | 1 | 0 | n.s |
DAA treatment (n) | 3 | 2 | n.s |
Pegylated interferon treatment (n) | 4 | 4 | n.s |
SVR (%) | 5 (71.4%) | 4 (66.7%) | n.s |
Results are shown as mean ± SD. Continuous variables between two groups were assessed with Mann-Whitney U test or t-test, and categorical variables were evaluated with χ2 test or Fisher’s exact test. HCV, hepatitis C virus; HCC, hepatocellular carcinoma; BMI, body mass index; ALT, alanine aminotransferase; AST, aspartate aminotransferase; ALP, alkaline phosphatase; AFP, alpha-fetoprotein; DAA, direct-acting antivirals; SVR, sustained virologic response; n.s, no statistical significance.
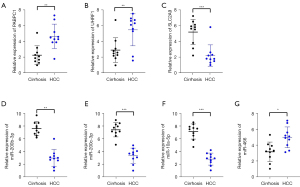
UHRF1 promotes proliferation and migration of HCC cells
To demonstrate whether UHRF1 promotes the development of HCV-associated HCC, we constructed the UHRF1-knockdown Huh7 cell line and the UHRF1-overexpressing HepG2 cell line (Figure 9A,9B). By CCK-8 and colony formation assays, we found that the proliferative capacity of UHRF1-knockdown Huh7 cells was reduced, while that of UHRF1-overexpressing HepG2 cells was increased (Figure 9C-9F). The wound-healing assay revealed that the function of migration and repair were impaired in UHRF1-knockdown Huh7 cells (Figure 9G).
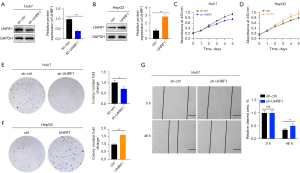
Discussion
In the research, 39 DE-miRNAs and 796 DEGs between cirrhosis and HCC in HCV-infected patients were identified in GSE40744 and GSE6764, respectively. Seventy-seven intersection genes obtained from the crossover of DEGs and potential target genes of DE-miRNAs mainly function in the cell cycle and DNA replication. Furthermore, PABPC1, UHRF1, and SLC2A9 form a prognostic model indicating the development of HCV-associated cirrhosis to HCC. Patients with HCV-associated cirrhosis who have a high-risk score are more likely to develop HCC. Compared to that in HCV-associated cirrhosis, PABPC1, UHRF1, and miR-466 are upregulated while SLC2A9, miR-200b-3p, miR-200c-3p, and miR-10a-5p are downregulated in HCV-associated HCC. These three risk genes and their four corresponding miRNAs make up a prognostic regulatory network in the progression of HCV-associated cirrhosis to HCC. UHRF1 promotes the proliferation and migration ability of HCC cells.
Despite several bioinformatics analyses being conducted to explore the miRNA regulatory network of HCC, pretty little research focused on the progression process of cirrhosis to HCC. This study noted that PABPC1, UHRF1, and SLC2A9 could be considered prognostic biomarkers indicating the potential risk of HCC development in patients with HCV-associated cirrhosis. In addition, PABPC1, UHRF1, SLC2A9, miR-200b-3p, miR-200c-3p, miR-10a-5p, and miR-466 may have important implications for the early diagnosis of HCC.
PABPC1, belonging to the PABPC gene family, is responsible for regulating mRNA metabolism by binding to the mRNA (21,22). Recent studies have found that PABPC1 participates in regulating diverse malignant tumors including gastric carcinoma (23), gliomas (24), endometrial cancer (25), etc. (26). Zhang et al. reported that overexpressed PABPC1 interplays with AGO2 to mediate gene silencing in HCC (27). Similarly, PABPC1 was expressed at a higher level in HCC compared to cirrhosis according to GSE6764. Interestingly, upregulated PABPC1 expression in cirrhotic patients did not imply an increased risk of HCC development. Herein, the expression level of PABPC1 from HCV-associated cirrhosis to HCC is not a linear model, and cirrhotic patients with lower expression of PABPC1 are more likely to enter into low-grade HCC, in which the expression level of PABPC1 is also low.
SLC2A9 initially was known as a fructose transporter. In recent years, SLC2A9 was identified as an important urate transportsome associated with hypouricemia (28). SLC2A9 could be regulated by the tumor suppressor p53, resulting in the reactive oxygen species (ROS) being reduced and cell proliferation being inhibited (29,30). SLC2A9 was lowly expressed in HCC (30), which was consistent with our validation results. Our prognostic model demonstrated that HCV-associated cirrhotic patients with lower expression of SLC2A9 are more likely to develop HCC.
UHRF1, a well-known epigenetic regulator, maintains DNA methylation during cell proliferation (31). As an oncogene, UHRF1 is overexpressed in HCC and negatively regulates the levels of tumor-suppressive lncRNA MEG3 (32,33). A higher expression level of UHRF1 in liver tissues induces the inactivation of TP53-mediated senescence, which causes non-programmatic cell proliferation (34). Similarly, in our research, a higher mRNA level of UHRF1 was detected in HCV-associated HCC than that in HCV-associated cirrhosis. UHRF1-knockdown Huh7 cells showed reduced proliferation, migration, and repair capacity. Therefore, UHRF1 serves as an oncogene that promotes HCC development.
It is estimated that almost 3/5 of human mRNAs could be regulated by miRNAs (35). Some miRNAs make an important role in hepatitis virus infection, such as miR-15b and miR-501, which directly facilitate viral replication (36,37). Moreover, miRNAs can serve as biomarkers for different clinical stages of liver disease. For instance, upregulated miR-29 expression is associated with the suppression of fibrosis/cirrhosis (38). The miR-199b and miR-244 participate in hepatocarcinogenesis (39). In our research, miR-200b-3p, miR-200c-3p, and miR-10a-5p are downregulated while miR-466 is upregulated in HCV-associated HCC samples. Specifically, miR-10a-5p can target PABPC1 to prevent HCC development while also fostering HCC development by targeting UHRF1. In the future study, we will explore how these indicators affect the development of HCV-associated HCC.
However, our study has some limitations. First, we only explored the differential expression levels of risk genes and their corresponding miRNAs in liver tissue samples from HCV-associated HCC and cirrhosis. However, exosomes contain a large amount of important informative materials, mediating the transfer of proteins, DNA, and RNA, contributing to the development of HCC. The abundant mRNA and miRNAs in exosomes may provide new biomarkers for the early diagnosis of HCC (40). We will subsequently examine the expression and function of these risk genes and miRNAs in blood exosomes. Second, experimental validation was not enough to fully demonstrate the regulatory functions of miRNAs and risk genes in the progression of HCV-associated cirrhosis to HCC. Finally, exploring gene signatures indicating the risk of HCC development in patients with cirrhosis is urgent, which could provide holistic insights for early diagnosis and prevention of HCC.
Conclusions
In summary, a 3-gene-based prognostic model for indicating the risk of HCV-associated cirrhosis developing into HCC is well established. PABPC1, UHRF1, SLC2A9, miR-200b-3p, miR-466, miR-200c-3p, and miR-10a-5p are quite likely to play a huge role in the progression of HCV-associated cirrhosis to HCC. UHRF1 serves as an oncogene to promote the proliferation and migration of HCC cells.
Acknowledgments
We thank Professor Weiguo Dong and doctor Binglu Huang (Renmin Hospital of Wuhan University) for their help in embellishing the language of the article.
Funding: This work was supported by
Footnote
Reporting Checklist: The authors have completed the TRIPOD reporting checklist. Available at https://tcr.amegroups.com/article/view/10.21037/tcr-22-989/rc
Conflicts of Interest: All authors have completed the ICMJE uniform disclosure form (available at https://tcr.amegroups.com/article/view/10.21037/tcr-22-989/coif). The authors have no conflicts of interest to declare.
Ethical Statement: The authors are accountable for all aspects of the work in ensuring that questions related to the accuracy or integrity of any part of the work are appropriately investigated and resolved. The study fully complied with the Declaration of Helsinki (as revised in 2013), and was approved and supervised by the Ethics Committee of Zhongshan Hospital, Fudan University while it was conducted (Approval ID: 2019, No. 29). All patients provided informed consent.
Open Access Statement: This is an Open Access article distributed in accordance with the Creative Commons Attribution-NonCommercial-NoDerivs 4.0 International License (CC BY-NC-ND 4.0), which permits the non-commercial replication and distribution of the article with the strict proviso that no changes or edits are made and the original work is properly cited (including links to both the formal publication through the relevant DOI and the license). See: https://creativecommons.org/licenses/by-nc-nd/4.0/.
References
- Heimbach JK, Kulik LM, Finn RS, et al. AASLD guidelines for the treatment of hepatocellular carcinoma. Hepatology 2018;67:358-80. [Crossref] [PubMed]
- Omata M, Cheng AL, Kokudo N, et al. Asia-Pacific clinical practice guidelines on the management of hepatocellular carcinoma: a 2017 update. Hepatol Int 2017;11:317-70. [Crossref] [PubMed]
- Borzio M, Fargion S, Borzio F, et al. Impact of large regenerative, low grade and high grade dysplastic nodules in hepatocellular carcinoma development. J Hepatol 2003;39:208-14. [Crossref] [PubMed]
- Müller M, Bird TG, Nault JC. The landscape of gene mutations in cirrhosis and hepatocellular carcinoma. J Hepatol 2020;72:990-1002. [Crossref] [PubMed]
- Choi DT, Kum HC, Park S, et al. Hepatocellular Carcinoma Screening Is Associated With Increased Survival of Patients With Cirrhosis. Clin Gastroenterol Hepatol 2019;17:976-987.e4. [Crossref] [PubMed]
- Loureiro D, Tout I, Narguet S, et al. miRNAs as Potential Biomarkers for Viral Hepatitis B and C. Viruses 2020;12:1440. [Crossref] [PubMed]
- Dash S, Aydin Y, Widmer KE, et al. Hepatocellular Carcinoma Mechanisms Associated with Chronic HCV Infection and the Impact of Direct-Acting Antiviral Treatment. J Hepatocell Carcinoma 2020;7:45-76. [Crossref] [PubMed]
- Kanwal F, Hoang T, Kramer JR, et al. Increasing prevalence of HCC and cirrhosis in patients with chronic hepatitis C virus infection. Gastroenterology 2011;140:1182-1188.e1. [Crossref] [PubMed]
- Ha M, Kim VN. Regulation of microRNA biogenesis. Nat Rev Mol Cell Biol 2014;15:509-24. [Crossref] [PubMed]
- Bartel DP. MicroRNAs: genomics, biogenesis, mechanism, and function. Cell 2004;116:281-97. [Crossref] [PubMed]
- Karmon AE, Cardozo ER, Rueda BR, et al. MicroRNAs in the development and pathobiology of uterine leiomyomata: does evidence support future strategies for clinical intervention? Hum Reprod Update 2014;20:670-87. [Crossref] [PubMed]
- O'Brien J, Hayder H, Zayed Y, et al. Overview of MicroRNA Biogenesis, Mechanisms of Actions, and Circulation. Front Endocrinol (Lausanne) 2018;9:402. [Crossref] [PubMed]
- Surajit P, Suhanya PV, Antara B. miRNA biogenesis, function and their therapeutic implications. Res J Biotechnol 2021;16:186-94. [Crossref]
- McGuire A, Brown JA, Kerin MJ. Metastatic breast cancer: the potential of miRNA for diagnosis and treatment monitoring. Cancer Metastasis Rev 2015;34:145-55. [Crossref] [PubMed]
- Abreu FB, Liu X, Tsongalis GJ. miRNA analysis in pancreatic cancer: the Dartmouth experience. Clin Chem Lab Med 2017;55:755-62. [Crossref] [PubMed]
- Hayes CN, Chayama K. MicroRNAs as Biomarkers for Liver Disease and Hepatocellular Carcinoma. Int J Mol Sci 2016;17:280. [Crossref] [PubMed]
- Ning S, Liu H, Gao B, et al. miR-155, miR-96 and miR-99a as potential diagnostic and prognostic tools for the clinical management of hepatocellular carcinoma. Oncol Lett 2019;18:3381-7. [Crossref] [PubMed]
- Mullany LE, Herrick JS, Wolff RK, et al. MicroRNA-transcription factor interactions and their combined effect on target gene expression in colon cancer cases. Genes Chromosomes Cancer 2018;57:192-202. [Crossref] [PubMed]
- Lu Y, Yue X, Cui Y, et al. MicroRNA-124 suppresses growth of human hepatocellular carcinoma by targeting STAT3. Biochem Biophys Res Commun 2013;441:873-9. [Crossref] [PubMed]
- Zhang G, Chen H, Guo Y, et al. Activation of Platelet NLRP3 Inflammasome in Crohn's Disease. Front Pharmacol 2021;12:705325. [Crossref] [PubMed]
- Tritschler F, Huntzinger E, Izaurralde E. Role of GW182 proteins and PABPC1 in the miRNA pathway: a sense of déjà vu. Nat Rev Mol Cell Biol 2010;11:379-84. [Crossref] [PubMed]
- Wu Y, Xu K, Qi H. Domain-functional analyses of PIWIL1 and PABPC1 indicate their synergistic roles in protein translation via 3'-UTRs of meiotic mRNAs. Biol Reprod 2018;99:773-88. [Crossref] [PubMed]
- Zhu J, Ding H, Wang X, et al. PABPC1 exerts carcinogenesis in gastric carcinoma by targeting miR-34c. Int J Clin Exp Pathol 2015;8:3794-802. [PubMed]
- Wang Q, Wang Z, Bao Z, et al. PABPC1 relevant bioinformatic profiling and prognostic value in gliomas. Future Oncol 2020;16:4279-88. [Crossref] [PubMed]
- Zhang L, Wan Y, Zhang Z, et al. IGF2BP1 overexpression stabilizes PEG10 mRNA in an m6A-dependent manner and promotes endometrial cancer progression. Theranostics 2021;11:1100-14. [Crossref] [PubMed]
- Takashima N, Ishiguro H, Kuwabara Y, et al. Expression and prognostic roles of PABPC1 in esophageal cancer: correlation with tumor progression and postoperative survival. Oncol Rep 2006;15:667-71. [Crossref] [PubMed]
- Zhang H, Sheng C, Yin Y, et al. PABPC1 interacts with AGO2 and is responsible for the microRNA mediated gene silencing in high grade hepatocellular carcinoma. Cancer Lett 2015;367:49-57. [Crossref] [PubMed]
- Vitart V, Rudan I, Hayward C, et al. SLC2A9 is a newly identified urate transporter influencing serum urate concentration, urate excretion and gout. Nat Genet 2008;40:437-42. [Crossref] [PubMed]
- Itahana Y, Han R, Barbier S, et al. The uric acid transporter SLC2A9 is a direct target gene of the tumor suppressor p53 contributing to antioxidant defense. Oncogene 2015;34:1799-810. [Crossref] [PubMed]
- Han X, Yang J, Li D, et al. Overexpression of Uric Acid Transporter SLC2A9 Inhibits Proliferation of Hepatocellular Carcinoma Cells. Oncol Res 2019;27:533-40. [Crossref] [PubMed]
- Bostick M, Kim JK, Estève PO, et al. UHRF1 plays a role in maintaining DNA methylation in mammalian cells. Science 2007;317:1760-4. [Crossref] [PubMed]
- Bronner C, Achour M, Arima Y, et al. The UHRF family: oncogenes that are drugable targets for cancer therapy in the near future? Pharmacol Ther 2007;115:419-34. [Crossref] [PubMed]
- Zhuo H, Tang J, Lin Z, et al. The aberrant expression of MEG3 regulated by UHRF1 predicts the prognosis of hepatocellular carcinoma. Mol Carcinog 2016;55:209-19. [Crossref] [PubMed]
- Ko E, Kim JS, Bae JW, et al. SERPINA3 is a key modulator of HNRNP-K transcriptional activity against oxidative stress in HCC. Redox Biol 2019;24:101217. [Crossref] [PubMed]
- Friedman RC, Farh KK, Burge CB, et al. Most mammalian mRNAs are conserved targets of microRNAs. Genome Res 2009;19:92-105. [Crossref] [PubMed]
- Dai X, Zhang W, Zhang H, et al. Modulation of HBV replication by microRNA-15b through targeting hepatocyte nuclear factor 1α. Nucleic Acids Res 2014;42:6578-90. [Crossref] [PubMed]
- Jin J, Tang S, Xia L, et al. MicroRNA-501 promotes HBV replication by targeting HBXIP. Biochem Biophys Res Commun 2013;430:1228-33. [Crossref] [PubMed]
- Roderburg C, Urban GW, Bettermann K, et al. Micro-RNA profiling reveals a role for miR-29 in human and murine liver fibrosis. Hepatology 2011;53:209-18. [Crossref] [PubMed]
- Gao P, Wong CC, Tung EK, et al. Deregulation of microRNA expression occurs early and accumulates in early stages of HBV-associated multistep hepatocarcinogenesis. J Hepatol 2011;54:1177-84. [Crossref] [PubMed]
- Sasaki R, Kanda T, Yokosuka O, et al. Exosomes and Hepatocellular Carcinoma: From Bench to Bedside. Int J Mol Sci 2019;20:1406. [Crossref] [PubMed]