Identification of candidate hub genes correlated with the pathogenesis, diagnosis, and prognosis of prostate cancer by integrated bioinformatics analysis
Introduction
Prostate cancer (PCa) has the second highest morbidity and mortality rates in men worldwide, succeeding lung cancer (1). Family history, race, and genetic factors are well-established risk factors for PCa (2). Men of African ancestry have the highest PCa incidence, followed by European and Asian men (3). The risk of PCa is correlated with increasing age; almost all PCa patients are over 50 years of age, with an average age of 66 years (4). In 2020, about 1,414,259 new cases of PCa and 375,304 associated deaths have occurred globally (5). By 2030, the number of new PCa cases worldwide is predicted to increase to 1,700,000 and lead to about 500,000 deaths (1).
Currently, typical clinical diagnosis methods for PCa include digital rectal examination (DRE), serum prostate specific antigen (PSA) level measurement, multiparametric magnetic resonance imaging (mpMRI), and trans-rectal ultrasound (TRUS) guided biopsy (6). However, each of these methods can only identify a proportion of cancers. For higher diagnosis efficiency, these methods are usually used in combination (7). Prostate-specific membrane antigen (PSMA) tagged PET/CT was reported to be a promising novel clinical imaging diagnostic method, but was more specific to advanced and metastatic disease than primary disease (8). Additionally, recent studies have revealed new biomarkers except the most widely used PSA, including prostate antigen 3 (PCA3), lncRNA, miRNA, and TMPRSS2:ERG fusion gene (9-11). An accurate pre-biopsy diagnosis method could reduce the number of unnecessary biopsies which would help prevent patients’ potential pain and risk related to the procedure (12). Meanwhile, molecular biomarkers provide added and worthy information about the biological mechanisms of PCa and can supplement existing clinicopathologic tools for prognosis (13). Therefore, further research that focuses on prospective molecular mechanisms associated with PCa may help to identify effective biomarkers, which could contribute to earlier diagnosis, prediction of prognosis and recurrence, and indication of potential therapeutic targets for patients.
With the rapid development of high-throughput sequencing technology, bioinformatics analysis has become a powerful tool in biomedical field for predicting disease-associated genes, disease subtypes, and disease treatment (14). The search for tumor-related genes and their related molecular mechanism has extensively involved the use of gene expression profile analyses in pursuit of discovering tumor-specific biomarkers, drug therapeutic targets, and prognosis predictors. However, due to the small sample sizes in individual studies and the use of different technological platforms, substantial inter-study variability and difficult statistical analyses have been generated (15). To solve this problem, integrated bioinformatics methods such as Robust Rank Aggregation (RRA), ImaGEO, minimum Redundancy Maximum Relevance (mRMR), support vector machine (SVM), and MetaDE, have been applied in various cancer studies, such as non-small cell lung cancer (NSCLC), cervical cancer, colorectal cancer, esophageal squamous cell carcinoma (ESCC) (16-21). These methods can integrate data from different independent studies and obtain more clinical samples for data mining, for ease of achieving more robust and accurate analysis. It’s worth noting that although numerous studies have already explored candidate gene biomarkers in PCa, most of these studies merely analyze individual dataset or utilize Venn diagram to directly combine the screened differentially expressed genes (DEGs) from different datasets, which may overlook some crucial biological information due to the high heterogeneity in PCa (22-27). Thus, we aim to suggest and improve the potential scarcity of studies on interaction-based analysis of DEGs in PCa.
In this study, 4 microarray datasets from Gene Expression Omnibus (GEO) database were analyzed. We innovatively combined 2 integrated bioinformatics method MetaQC/MetaDE and RRA to improve the efficiency and accuracy of DEGs screening. After 368 DEGs (120 upregulated and 248 downregulated) were detected, the Gene Ontology (GO) functional annotation and Kyoto Encyclopedia of Genes and Genomes (KEGG) pathway enrichment analysis of these genes were performed, and the protein-protein interaction (PPI) network of the DEGs was constructed; 11 hub genes were detected from the PPI network and after the survival and clinical attribute analysis, 4 of 11 hub genes CAV1, KRT5, SNAI2, MYLK show potential clinical diagnostic and prognostic value and could be used as novel candidate biomarkers and therapeutic targets for PCa. We present the following article in accordance with the STREGA reporting checklist (available at https://tcr.amegroups.com/article/view/10.21037/tcr-22-703/rc).
Methods
Microarray data
Gene expression datasets were screened for “prostate cancer” and “Homo sapiens”, and the study type was set as “expression profiling by array” in the GEO database (www.ncbi.nlm.nih.gov/geo/). The studies were selected based on the following inclusion criteria: (I) the datasets were from similar platforms of gene expression microarray and the gene family was denoted in detail; (II) the samples were collected from primary cancerous prostate tissues and normal prostates; (III) each dataset contains more than 10 samples. Eight GEO datasets with a total of 363 cases and 196 controls were selected from the GEO database (Table 1). Among them, GSE3325, GSE6956, GSE17951, GSE46602, GSE55945, and GSE69223 were based on the Affymetrix platform (Affymetrix; Thermo Fisher Scientific, Inc., Waltham, MA, USA). GSE32571 and GSE89194 were based on the Illumina platform (Illumina, Inc., San Diego, CA, USA). The original GSE3325 dataset contained 6 metastatic PCa tissue samples, which were removed for subsequent analysis. The study was conducted in accordance with the Declaration of Helsinki (as revised in 2013).
Table 1
GEO ID | Platform | Source DOI | Sample size | |
---|---|---|---|---|
Normal | Tumor | |||
GSE3325 | GPL570 | 10.1016/j.ccr.2005.10.001 | 6 | 13 |
GSE6956 | GPL571 | 10.1158/0008-5472.CAN-07-2608 | 20 | 69 |
GSE17951 | GPL570 | 10.1158/0008-5472.CAN-10-0021 | 45 | 109 |
GSE32571 | GPL6947 | 10.1007/s00109-012-0949-1 | 39 | 59 |
GSE46602 | GPL570 | 10.1038/srep16018 | 14 | 36 |
GSE55945 | GPL570 | 10.1158/1078-0432.CCR-09-0911 | 8 | 13 |
GSE69223 | GPL570 | 10.18632/oncotarget.6370 | 15 | 15 |
GSE89194 | GPL22571 | 10.1371/journal.pgen.1006477 | 49 | 49 |
GEO, Gene Expression Omnibus.
Data processing and quality control (QC)
Microarray raw data of the 8 datasets was downloaded via txt format from the corresponding platform. The original data of GSE3325, GSE6956, and GSE55945 was gathered by employing log2 transformation using the Limma Package (version 3.40.6) in R (http://www.bioconductor.org/packages/release/bioc/html/limma.html). For the five datasets GSE17951, GSE32571, GSE46602, GSE69223, and GSE89194, the original data was used since the gene expression data has already undergone log2 transformation. Then interquartile range (IQR) method in the MetaDE Package (version 1.0.5) was used to summarize the multiple probes to one intensity (28). The data QC step is vital for bioinformatics analysis, in order to assess the quality and consistency of the datasets and improve the reliability and accuracy of the results. The MetaQC method provides systematic quality assessment of microarray data across studies to decide inclusion/exclusion criteria for genomic meta-analysis. The QC steps were performed on these datasets by using the MetaQC package (version 0.1.13) in R and the datasets with low quality were filtered (28,29). The full method of data processing and QC step are shown in the Appendix 1.
Microarray meta-analysis for DEGs
The MetaDE package implements 12 major meta-analysis methods for differential expression analysis (28). The 4 selected datasets including 123 PCa samples and 76 normal prostate samples were merged into a new dataset by “MetaDE.merge” function in MetaDE package. After the merge, “MetaDE.rawdata” function was used to screen the DEGs, and Fisher’s exact test in the package was chosen as the meta-analysis method. The threshold for DEGs was false discovery rate (FDR) <0.01 and P value <0.01.
Screening of feature genes in each dataset and integration of DEGs by RRA method
For GSE32571, GSE46602, GSE55945, and GSE69223 datasets, the Limma R package was used to screen DEGs as well as adjusted P value <0.05 and |log2 fold change| >1 as the screening criteria for DEGs. The RRA R package was used to integrate the common DEGs of the 4 datasets. The RRA algorithm has been widely used for DEGs screening because of its robustness to noise and better enrichment results than other methods (30). Adjusted P value <0.05 and |log2FC| >1 were set as the screening criteria referring to the methods of previous similar studies (18,31).
Common DEGs screened by both RRA method and meta-analysis
The intersection of the DEGs identified by RRA and meta-analysis were taken to identify common DEGs of these two different methods. These common DEGs were used as the final version for succeeding GO, KEGG, and PPI analysis.
GO annotation and KEGG pathway enrichment analysis
GO annotation analysis provides explain and annotate of gene functions by three dimensions: cellular component (CC), molecular function (MF), and biological process (BP). Meanwhile, KEGG analysis provides the information of the biological pathways the genes participate in. GO annotation and KEGG pathway enrichment analysis of the identified DEGs were performed based on DAVID online database (https://david.ncifcrf.gov/tools.jsp) to characterize the functional roles of the DEGs (32,33). And the enrichment results were visualized by the ggplot2, GOplot, and tidyr R packages.
PPI network and modules analysis
Search Tool for the Retrieval of Interacting Genes/Proteins (STRING) database (https://string-db.org/) is widely used to analyze the interaction relationships between proteins. The PPI network of the DEGs was produced by STRING. Cytoscape software 3.7.1 was utilized to further analyze the PPI network. Hub genes play a crucial role in biological processes and affect the regulation of other genes and pathways. The Cytohubba plug-in tool provides 11 methods, MNC, DMNC, MCC, Degree, EPC, BottleNeck, EcCenticity, Betweenness, Closeness, Stress, Radiality to screen for hub genes from the PPI network (34). The 11 topological methods were intersected to identify the hub genes. Lastly, the Molecular Complex Detection (MCODE) plug-in tool was applied to explore notable modules in the PPI network.
Expression level analysis of the hub genes
The Gene Expression Profiling Interactive Analysis (GEPIA) online database (http://gepia.cancer-pku.cn/) was used to analyze and verify the mRNA expression level of the top hub genes between PCa samples and normal samples in The Cancer Genome Atlas Prostate Adenocarcinoma (TCGA PRAD) dataset (35). The Human Protein Atlas (HPA; https://www.proteinatlas.org/) database provides almost all of the human protein distribution information regarding organs, tissues, and cells. Based on the immunohistochemical data of normal prostate tissue and PCa tissue in the HPA database, the expression of the hub genes are tested in the protein level.
Methylation analysis
The DiseaseMeth 2.0 database (the human disease methylation database version 2.0; http://diseasemeth.edbc.org/) provides the information of 679,602 disease-gene associations from multiple technology platforms in 88 kinds of human diseases. However, most other related methylation databases only included information of methylated genes in specific kinds of diseases (36). MEXPRESS (http://mexpress.be) is also a online methylation database which integrate and visualize the association between clinical data from TCGA, gene expression, and DNA methylation (37,38). Based on the different advantages of these 2 datasets, the methylation level of hub genes in PCa and normal prostate tissues was analyzed via the DiseaseMeth 2.0 database, and the association between the gene expression level and DNA methylation status of the hub genes was analyzed using MEXPRESS.
The receiver operating characteristic (ROC) and clinical attribute analysis of the hub genes
ROC curve analysis was operated by the pROC R package (version 1.16.2) to predict the prospect of hub genes as diagnostic biomarkers (39). A ROC curve is a graphical plot that illustrates the diagnostic ability of a binary classifier as a function of its discrimination threshold. And ROC curve analysis has been well established in clinical diagnostic application for evaluating a marker’s capability of discriminating between individuals who experience disease onset and individuals who do not (40). Meanwhile, the ggstatsplot package in R (version 0.5.0; https://cran.r-project.org/package=ggstatsplot) was utilized to evaluate the correlation between the expression level of the hub genes and clinical features, such as pathological tumor stage (T stage), pathological lymph node metastasis stage (N stage), Gleason score, and biochemical recurrence (BCR) status. Survival analysis for hub genes was also assessed using survminer package (version 0.4.7; https://CRAN.R-project.org/package=survminer) and survival package (version 3.1-12; https://CRAN.R-project.org/package=survival). The clinical data was abstract from the TCGA PRAD dataset which contain RNA-sequencing of PCa tissue and clinical data of PCa patients. The tumor-node-metastasis (TNM) stage classification of TCGA PRAD dataset refers to the 7th edition American Joint Committee on Cancer (AJCC) system (41).
Statistical analysis
The MetaQC package in R was used to execute the QC step. The limma package, metaDE package and RRA package in R were used to screen DEGs. The functional enrichment research of DEGs were based on GO and KEGG analysis. The STRING database and Cytoscape were used to construct PPI network. ROC curve analysis was operated by the pROC R package to predict the prospect of hub genes as diagnostic biomarkers. The ggstatsplot package in R was utilized to evaluate the correlation between the expression level of the hub genes and clinical features and independent samples t-test or one-way analysis of variance (ANOVA) was used as appropriate. Survival analysis was performed by survminer and survival package in R. Survival plots were showed by the Kaplan-Meier method, and the significance was calculated by the log-rank test. P<0.05 was defined as statistically significant.
Results
QC of the microarray data
The QC results of the 8 microarray datasets are shown in Table 2 and Figure 1. The QC score and the principal component analysis (PCA) biplot indicated that the first 5 datasets, GSE55945, GSE32571, GSE89194, GSE46602 and GSE69223, were of high-quality and the last 3 datasets, GSE17951, GSE6956, GSE3325, were of low-quality according to the Rank of QC score and the positions in PCA plot (29). Thus, the first 5 datasets were selected for subsequent analyses. The datasets GSE55945, GSE32571, GSE46602 and GSE69223 were utilized for biomarker screening. And GSE89194, which contains paired and the largest sample sizes, ranking the second in the QC results, were utilized as validation set. The clinical and histopathological data of the patient cohorts in selected 5 datasets are listed in Table S1 (the information of GSE55945 is not available) (42-44).
Table 2
Dataset | Study | IQC | EQC | CQCg | CQCp | AQCg | AQCp | Rank |
---|---|---|---|---|---|---|---|---|
1 | GSE55945 | 8.17 | 2.74 | 72.33 | 140.58 | 15.48 | 58.78 | 2.83 |
2 | GSE32571 | 1.3 | 2.21 | 58.71 | 155.76 | 20.01 | 124.7 | 3.33 |
3 | GSE89194 | 0.18 | 1.63 | 76.32 | 169.97 | 19.2 | 113.38 | 3.33 |
4 | GSE46602 | 2.62 | 4.7 | 45.77 | 56.44 | 9.32 | 28.99 | 4.67 |
5 | GSE69223 | 3.54 | 1.48 | 20.93 | 92.79 | 8.83 | 65.4 | 5.00 |
6 | GSE17951 | 6.64 | 3.47 | 1.22 | 3.41 | 1.63 | 4.76 | 5.50 |
7 | GSE6956 | 6.03 | 2.26 | 0 | 158.69 | 0.03 | 0 | 5.50 |
8 | GSE3325 | 3.22 | 1.32 | 21.78 | 77.97 | 5.09 | 34.24 | 5.83 |
QC, quality control; IQC, internal QC; EQC, external QC; CQCg, consistency QC; CQCp, precision of CQCg; AQCg, accuracy QC; AQCp, precision of AQCg.
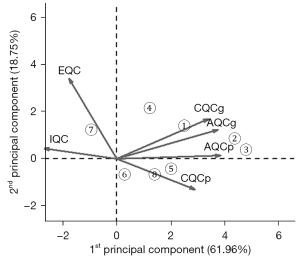
Microarray meta-analysis for DEGs in PCa
The 4 datasets, GSE55945, GSE32571, GSE46602 and GSE69223, containing 123 PCa samples and 76 normal samples, were utilized for the meta-analysis via MetaDE package. Using the threshold of FDR <0.01, a total of 2,778 DEGs were identified using the Fisher meta-analysis method in MetaDE package. Figure 2 shows the number of significant genes against different FDR threshold obtained from the MetaDE analysis.
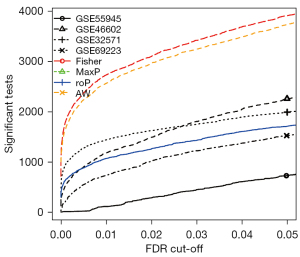
Identification of DEGs in each dataset and integration of DEGs in PCa
The DEGs were screened in each of the four datasets using the Limma package with adjusted P value <0.05 and |log2FC| >1. The GSE32571 dataset contained 292 DEGs, including 45 upregulated genes and 247 down regulated genes. The GSE46602 dataset contained 1316 DEGs, including 477 upregulated genes and 839 down regulated genes. The GSE69223 dataset had 1,371 DEGs, including 471 upregulated genes and 900 down regulated genes. The GSE55945 dataset contained 434 DEGs, including 156 upregulated genes and 278 down regulated genes. Figure 3 shows the DEGs volcano maps of the five datasets. The integrated DEGs were screened utilizing the RRA R package with adjusted P value <0.05 and |log2FC| >1, and 467 DEGs were identified, including 157 upregulated genes and 310 downregulated genes. The top 20 upregulated and downregulated genes according to adjusted P value are shown in Figure 4.
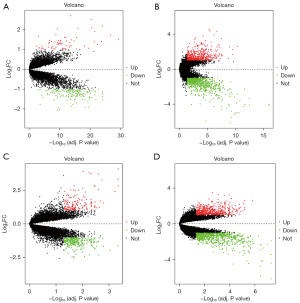
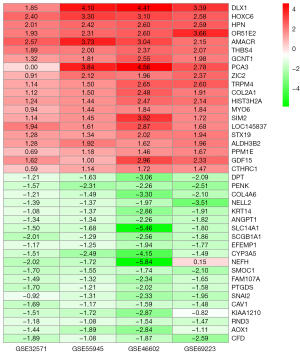
Identification of common DEGs screened by both RRA method and meta-analysis
The DEGs identified by RRA and meta-analysis were intersected to obtain the common DEGs. As a result, a total of 368 DEGs with 120 up-regulated DEGs and 248 down-regulated DEGs were selected. The 368 DEGs (available online: https://cdn.amegroups.cn/static/public/tcr-22-703-01.pdf) were used for following GO, KEGG, and PPI analyses.
GO functional enrichment analysis
GO functional enrichment analysis was performed for the upregulated and downregulated DEGs, respectively via DAVID. The GO functional annotation analysis has three parts: BP, CC, and MF. Figure 5 and Tables 3,4 showed the top 15 GO enrichment results with the statistically significant cut-off value as P value <0.05. The upregulated DEGs were principally enriched in lipid metabolic process (ontology: BP), extracellular exosome (ontology: CC) and RNA polymerase II transcription factor activity, and sequence-specific DNA binding (ontology: MF). The downregulated DEGs were principally enriched in cell adhesion (ontology: BP), cytoplasm (ontology: CC), and protein binding (ontology: MF).
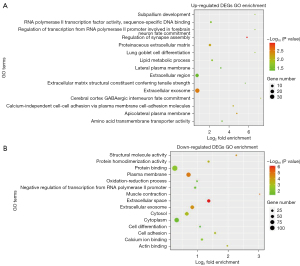
Table 3
Category | ID | Term | Count | P value |
---|---|---|---|---|
BP | GO:0006629 | Lipid metabolic process | 5 | 1.92e-02 |
BP | GO:0051963 | Regulation of synapse assembly | 3 | 1.14e-03 |
BP | GO:0016338 | Calcium-independent cell-cell adhesion via plasma membrane cell-adhesion molecules | 3 | 8.09e-03 |
BP | GO:0021893 | Cerebral cortex GABAergic interneuron fate commitment | 2 | 1.29e-02 |
BP | GO:0021544 | Subpallium development | 2 | 1.93e-02 |
BP | GO:0060480 | Lung goblet cell differentiation | 2 | 1.93e-02 |
BP | GO:0021882 | Regulation of transcription from RNA polymerase II promoter involved in forebrain neuron fate commitment | 2 | 1.93e-02 |
CC | GO:0070062 | Extracellular exosome | 30 | 3.23e-03 |
CC | GO:0005576 | Extracellular region | 17 | 3.99e-02 |
CC | GO:0005578 | Proteinaceous extracellular matrix | 7 | 6.52e-03 |
CC | GO:0016327 | Apicolateral plasma membrane | 3 | 4.88e-03 |
CC | GO:0016328 | Lateral plasma membrane | 3 | 4.28e-02 |
MF | GO:0000981 | RNA polymerase II transcription factor activity, sequence-specific DNA binding | 5 | 2.49e-02 |
MF | GO:0015171 | Amino acid transmembrane transporter activity | 3 | 3.70e-02 |
MF | GO:0030020 | Extracellular matrix structural constituent conferring tensile strength | 2 | 3.81e-02 |
GO, Gene Ontology; DEGs, differentially expressed genes; BP, biological process; CC, cellular component; MF, molecular function.
Table 4
Category | ID | Term | Count | P value |
---|---|---|---|---|
BP | GO:0007155 | Cell adhesion | 19 | 5.80e-05 |
BP | GO:0000122 | Negative regulation of transcription from RNA polymerase II promoter | 19 | 9.49e-03 |
BP | GO:0030154 | Cell differentiation | 15 | 4.37e-03 |
BP | GO:0001525 | Angiogenesis | 11 | 9.66e-04 |
BP | GO:0007399 | Nervous system development | 11 | 5.97e-03 |
CC | GO:0005737 | Cytoplasm | 83 | 2.62e-02 |
CC | GO:0005886 | Plasma membrane | 80 | 9.42e-05 |
CC | GO:0070062 | Extracellular exosome | 62 | 2.51e-05 |
CC | GO:0005829 | Cytosol | 60 | 5.84e-03 |
CC | GO:0005615 | Extracellular space | 53 | 7.74e-13 |
MF | GO:0005515 | Protein binding | 138 | 8.42e-03 |
MF | GO:0042803 | Protein homodimerization activity | 23 | 3.78e-04 |
MF | GO:0005509 | Calcium ion binding | 20 | 4.03e-03 |
MF | GO:0005198 | Structural molecule activity | 14 | 3.24e-05 |
MF | GO:0003779 | Actin binding | 12 | 1.44e-03 |
GO, Gene Ontology; DEGs, differentially expressed genes; BP, biological process; CC, cellular component; MF, molecular function.
Pathway enrichment analysis
The pathway enrichment analysis of the intersected DEGs was performed based on the KEGG database via DAVID, and the results are shown in Figure 6. These DEGs were principally enriched in the following pathways: the focal adhesion, drug metabolism—cytochrome P450, chemical carcinogenesis, glutathione metabolism, and metabolism of xenobiotics by cytochrome P450. Figure 7 showed the network graph of the DEGs drawn by program Cytoscape based on the KEGG enrichment results.
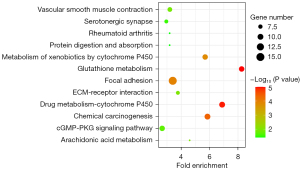

PPI network analysis and module analysis
The 368 DEGs were mapped into the PPI network via the STRING database, with a combined score of ≥0.4 as the cut-off value. Furthermore, the interaction results were analyzed by the Cytoscape plug-in tool MCODE to detect remarkable modules in the PPI network. A degree cutoff =2, Node Score cutoff =0.2, and K-core =2 were set as the advanced options. As a result, 11 functional modules were identified from the PPI network. The two modules with the highest score (module 1: MCODE score =8.00, module 2: MCODE score =6.70) were shown in Figure 8. GO and KEGG pathway enrichment of these genes in the two modules was performed, respectively. The GO enrichment results (Figure 9 and Table S2) showed that the genes in module 1 were most enriched with muscle contraction (ontology: BP), cytosol (ontology: CC) and structural constituent of muscle (ontology: MF); and genes in module 2 were most enriched with glutathione metabolic process (ontology: BP), extracellular region (ontology: CC) and glutathione transferase activity (ontology: MF). Meanwhile, the pathway enrichment results (Figure 10 and Table S3) showed that the genes in module 1 were principally enriched in vascular smooth muscle contraction, focal adhesion, and regulation of actin cytoskeleton. The genes in module 2 were principally enriched in chemical carcinogenesis, drug metabolism-cytochrome P450, and metabolism of xenobiotics by cytochrome P450.
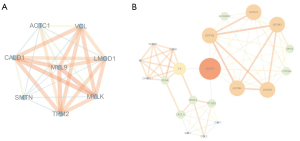
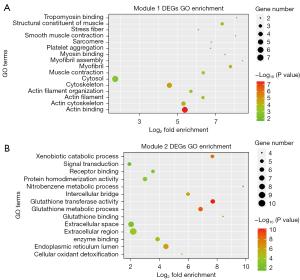
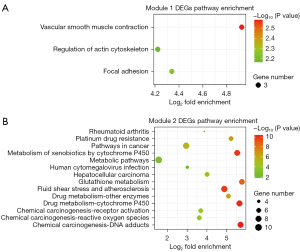
Screening of hub genes in PPI network
The top 25 hub genes were screened by the Cytohuba plug-in tool in Cytoscape according to the 11 topological algorithms respectively to address all different quantitative aspects of the interactions between the DEGs derived. 11 common hub genes that identified by at least 8 among 11 methods were identified, utilizing online Venn diagram tool (http://bioinformatics.psb.ugent.be/webtools/Venn/) (Table S4). Among the 11 hub genes, VEGFA, VCL, CAV1, KRT5, PTGS2, GJA1, SNAI2, CCL2, CXCL12, and MYLK were down-regulated, however, contrastingly, TWIST1 were up-regulated in primary PCa tissue (Table 5).
Table 5
Gene symbol | Full name | Log2FC |
---|---|---|
VEGFA | Vascular endothelial growth factor A | −1.04 |
VCL | Vinculin | −1.46 |
CAV1 | Caveolin 1 | −1.48 |
KRT5 | Keratin 5 | −2.25 |
PTGS2 | Prostaglandin-endoperoxide synthase 2 | −1.16 |
GJA1 | Gap junction protein alpha 1 | −1.03 |
TWIST1 | Twist family bHLH transcription factor 1 | 1.20 |
SNAI2 | Snail family transcriptional repressor 2 | −1.63 |
CCL2 | C-C motif chemokine ligand 2 | −1.11 |
CXCL12 | C-X-C motif chemokine ligand 12 | −1.27 |
MYLK | Myosin light chain kinase | −1.06 |
FC, fold change.
Expression level analysis of the hub genes
The GEPIA server (based on TCGA database) and HPA database were used to analyze and verify the expression of the 11 hub genes in PCa samples in both the levels of mRNA and protein. Based on the PRAD dataset in GEPIA (gene expression dataset of PCa in RNA level), the 8 of the 11 genes: VEGFA, VCL, CAV1, KRT5, PTGS2, GJA1, SNAI2, and MYLK were significantly downregulated (P value <0.001), and TWIST1 were significantly upregulated in PCa tissue (P value <0.001) (Figure 11). In the level of protein, based on the immunohistochemical data from the HPA database, CAV1, KRT5, GJA1, and SNAI2 also exhibited lower expression levels (Figure 12) in PCa tissue than normal tissue. But VEGFA, VCL, PTGS2, CXCL12, CCL2, and MYLK proteins exhibited inconsistent results in HPA database (the first 4 proteins showed both high and low expression levels in cancerous tissue and CCL2 and MYLK protein exhibited medium and low expression levels in both cancerous and normal tissue respectively). There is no data for the expression of the remaining TWIST1 protein in prostate tissue.
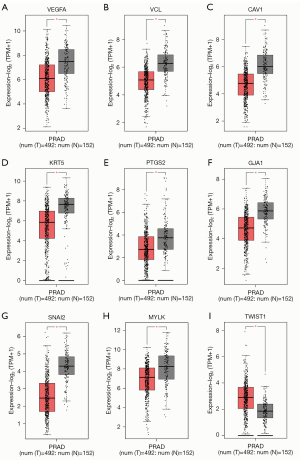

Association between methylation and expression of hub genes
The association between the expression levels of these 11 hub genes and their methylation status was explored in DiseaseMeth. The result showed that the average methylation levels of CAV1, CXCL12, GJA1, KRT5, MYLK, SNAI2, PTGS2, TWIST1 and VEGFA were significantly higher, and CCL2, VCL were significantly lower, in PCa than normal tissues (P value <0.05) (Figure 13). Meanwhile, the methylation analysis in MEXPRESS showed that numerous methylation sites existed in the DNA sequences of CAV1, CXCL12, GJA1, KRT5, MYLK, PTGS2, SNAI2, and VEGFA, which were negatively correlated with the expression levels of the hub genes. On the contrary, CCL2, TWIST1 and VCL showed positive results (Figure S1).
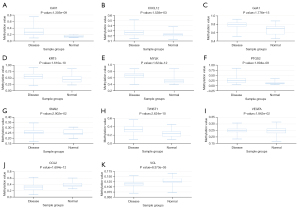
ROC and clinical attribute analysis of the hub genes
The GSE89194 dataset, ranking the second in the QC results, was used for ROC analysis because of containing paired samples and the largest sample size. Meanwhile, the RNA-seq and clinical data of the TCGA PRAD dataset were used to analyze the clinical diagnostic and prognostic value of the 11 hub genes. The ROC curves and the area under the curve (AUC) value in Figure 14 show that the gene expression level of 7 genes (VCL, CAV1, KRT5, GJA1, TWIST1, SNAI2 and MYLK) can clearly distinguish the cancer samples and normal samples. This suggests that these genes have potential as biomarkers for PCa. Figure 15 showed the relevance of the 11 hub genes with clinical attribute. The lower expression levels of the 4 downregulated genes, CAV1, KRT5, MYLK, and SNAI2, were significantly (P value <0.05) correlated with higher Gleason scores (CAV1: P value =0.002, KRT5: P value =0.001, SNAI2: P value =0.011, MYLK: P value <0.001), advanced pathological T stage (CAV1: P value <0.045, KRT5: P value =0.022, SNAI2: P value =0.016, MYLK: P value =0.016), and pathological N stage (CAV1: P value =0.01, KRT5: P value =0.045, SNAI2: P value =0.001, MYLK: P value =0.003). While the lower expression levels of CAV1, KRT5, and PTGS2 were associated with BCR status (CAV1: P value =0.048, KRT5: P value =0.024, PTGS2: P value =0.001). Moreover, the Kaplan-Meier survival curves (Figure 16) showed that lower expression of KRT5 and MYLK were significantly correlated with poor disease-free survival (KRT5: P value =0.023, MYLK: P value =0.0059). In summary, CAV1, KRT5, MYLK, and SNAI2 exhibit promising clinical diagnostic and prognostic value.
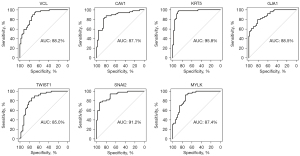

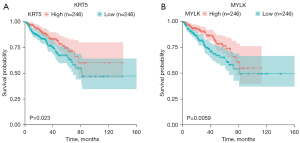
Discussion
In this study, we screened 368 common DEGs from four datasets (GSE32571, GSE55945, GSE46602, GSE69223) of PCa samples using a set of QC analysis “tools” and comparison of gene expression profiles. The GO enrichment analysis of the DEGs showed that the upregulated DEGs were majorly enriched in lipid metabolic process (ontology: BP), extracellular exosome (ontology: CC) and RNA polymerase II transcription factor activity, sequence-specific DNA binding (ontology: MF), and the downregulated DEGs were majorly enriched in cell adhesion (ontology: BP), cytoplasm (ontology: CC), and protein binding (ontology: MF). These processes are related to cell proliferation, adhesion, and metabolism, which indicated the processes changed significantly in PCa. Interestingly, KEGG pathway enrichment analysis of the DEGs also found the enrichment of similar processes: focal adhesion, chemical carcinogenesis, drug metabolism, and cytochrome-P450 pathways. These mutual confirmation result indicated boosting cell proliferation, cell movement and metabolism in the development of PCa cell. These changes were reported in other cancers and suggested the reliability of our screening methods (45-50).
The PPI network of DEGs in STRING and Cytoscape screened 11 hub genes: VEGFA, VCL, CAV1, KRT5, PTGS2, GJA1, TWIST1, SNAI2, CCL2, CXCL12 and MYLK. We validated the expression level of the 11 genes on both mRNA level based on GEPIA database and protein level based on HPA database. In the PRAD (prostate adenocarcinoma) dataset in the GEPIA database, VEGFA, VCL, CAV1, KRT5, PTGS2, GJA1, SNAI2, and MYLK were significantly downregulated, while TWIST1 were significantly upregulated in PCa tissue, which is in agreement with our results. And, in protein level according to the HPA dataset, CAV1, KRT5, GJA1, and SNAI2 exhibited lower expression levels in PCa tissue compared with normal prostate tissue, which is concordant with our research. However, the other 7 genes were not supported by HPA data. VEGFA, VCL, PTGS2, and CXCL12 exhibited both high and low protein expression levels in cancerous tissue, and CCL2 and MYLK proteins exhibited medium and low expression levels in both cancerous and normal tissue.
Numerous studies have reported that the elevated expression of VEGFA, VCL, PTGS2, and CXCL12 in cancers is associated with disease progression, tumor grade, metastasis, and prognosis (51-55). Although Zhu et al. reported that VCL expression level decreased as the tumor Gleason score increased, and Zheng et al. reported that downregulation of VCL suppressed tumor growth in vivo and VCL knockdown inhibited the migration, invasion, and movement and repressed colony formation and viability of PCa cells in vitro; our analysis revealed VEGFA, VCL, PTGS2, and CXCL12 expression was, actually, decreased in primary prostate tumor tissue (56,57).
VEGFA is a member of the VEGF family, which is involved in blood vessel development, homeostasis, and lymphatic vessel formation. VEGFA is a primary driver of angiogenesis and vasculogenesis. It is commonly accepted as promoter of tumor growth and motility and is upregulated in many forms of cancer (54). However, in our PCa study, VEGFA was significantly downregulated. This led us to further search other PCa datasets (not included in our screening datasets) in the GEO database, and we found that in GSE3325, a dataset specially for PCa progress, VEGFA was downregulated in primary PCa and upregulated in metastatic cancer comparing with benign PCa (58). This was consistent with our results. And in a large-scale analysis of the human transcriptome (GSE96), PCa and prostate normal tissue showed significant high expression compared to other samples from 36 human different tissues, except thyroid tissue. Comparing the PCa with prostate normal tissue, 2 of 5 patient samples showed significantly lower expression. 1 of 5 showed a significant high expression, and 2 of 5 exhibited nearly the same expression (59). Even though we do not know whether the high expression samples are from metastatic PCa in the dataset because of not denotation in the two datasets, combined with GEPIA result, we speculated that the VEGFA is possibly downregulated in primary PCa and upregulated in metastatic PCa, contrary to the expression in other cancers. Additionally, the cause maybe due to the high expression in normal prostate, and in the anormal condition of prostate, the expression is conversed correspondingly. However, the exact cause needs to be further studied experimentally.
VCL is an essential, ubiquitously expressed cytoskeletal protein that localizes to focal adhesions and adhesive junctions, and it plays a pivotal role in regulating cell adhesion, motility, and force transmission (55). Fagerberg et al. systematically analyzed the human tissue-specific expression in 95 human individuals representing 27 different tissues and found that, like VEGFA, VCL expression in normal prostate and endometrium were highly expressed, which is completely different from other normal issues (60). This may indicate a different expression pattern of VCL in PCa.
PTGS2, also as known as cyclooxygenase 2 (COX2), is an enzyme that catalyzes the conversion of arachidonic acid to prostaglandins, is often overexpressed in epithelial malignancies including breast, prostate, lung, kidney, ovary, and liver cancer and associated with worse disease progression (61). PTGS2 has been reported participating in cancer cell enhanced proliferation, migration, angiogenesis, inflammation, and metastatic dissemination in both PCa and colon cancer (51,52). Zhang reported that PTGS2 was over-expressed in PCa (62). Wang et al. found PTGS2 were lowly expressed in dasatinib resistant PCa cell lines and were highly expressed in dasatinib sensitive prostatic cancer cell lines, which may explain the conflicting results of PTGS2 expression in different PCa samples (63).
The CXCL12 is a member of the CXC family of chemokines that binds to CXCR4 and CXCR7 (64). CXCL12 can activate and induce the migration of hematopoietic progenitor cells, stem cells, endothelial cells, and most leukocytes. Additionally, it has been found to regulate inflammation, angiopoiesis, metastasis, and tumor growth, which indicates that CXCL12 is involved in cancer development and further metastasis (53). Expression level of CXCL12 and CXCR4 are increased in PCa and the CXCL12/CXCR4 axis participate in the metastasis of PCa (65). In a study of a microRNA-135b overexpression effects on PCa cell line (GSE57820), CXCL12 was found to be downregulated over time, whether microRNA-135b was overexpressed or not (66). In GSE56265, under the effects of lysophosphatidic acid, breast and PCa cell lines are both significantly down regulated relative to controls (67).
Prostate tumors are mostly multifocal and heterogeneous, and they fluctuate at different stages of tumor development and in different conditions. Our analysis indicated the complex status of PCa. Concurrently, we identified the robust biomarkers based on different sources of data. The results of these two aspects might provide references for future scientific research and clinical application.
DNA methylation status analysis via DiseaseMeth 2.0 and MEXPRESS database showed that CAV1, CXCL12, GJA1, VEGFA, KRT5, MYLK, PTGS2 and SNAI2 were methylated in PCa tissues compared to normal ones, which is in accord with the down-regulation of these 8 hub genes associated with PCa and examined in this study. It’s worth noting that TWIST1 were methylated, and VCL and CCL2 were demethylated, in PCa tissue, which is inconsistent with previous results in this study. And the methylation status of TWIST1 were positively correlated with the gene expression level. This suggests a more complex relationship between gene expression and DNA methylation status in PCa.
We further explored the diagnostic and prognostic value of the 11 hub genes. The ROC curve analysis showed that VCL, CAV1, KRT5, GJA1, SNAI2, TWIST1 and MYLK could be used to distinguish PCa tissue from normal prostate tissue sensitively and accurately. Additionally, we determined that CAV1, KRT5, SNAI2, and MYLK were negatively correlated with a higher Gleason score and advanced pathological T and N stages. Moreover, lower KRT5 and MYLK expression was significantly associated with poor disease-free survival, and lower KRT5 and PTGS2 expression was significantly related to BCR status of PCa patients. These outcomes suggest the efficacy of using the 4 genes to determine diagnosis and prognosis for PCa patients.
CAV1 is a carcinogenic membrane protein associated with endocytosis, extracellular matrix tissue, cholesterol distribution, cell migration and signal transduction. Previous studies have found that CAV1 is involved in liver cancer, colon cancer, breast cancer, kidney cancer, lung cancer and skin cancer etc., and acted as a promoter or inhibitor of cancer according to cancer type and progress (68-70). Multiple endogenous and exogenous agents, such as Chrysotobibenzyl, Cordycepin and Giantol, have been used to modulate CAV-1 expression to regulate lung cancer progression (71-73). KRT5 is one of the human keratin proteins, primarily expressed in epidermal basal keratinocytes (74). Cimpean et al. reported that the expression level of KRT5 is in correlation with the prognosis and TNM stage in head and neck squamous cell carcinomas (HNSCC) (75). And Ricciardelli et al. founded that K5 overexpression in serous ovarian cancer is associated with recurrence and chemotherapy resistance (76). SNAI2 encodes a zinc-finger protein of the Snail family of transcription factors, and plays an important part in epithelial-mesenchymal transition (EMT). Tian et al. reported that the miR-203/SNAI2 axis plays a role in regulating prostate tumor growth, migration, angiogenesis and stemness (77). Meanwhile, the dynamic expression of SNAI2 in PCa can predicts tumor progression and drug sensitivity, and loss of SNAI2 in PCa correlates with clinical response to androgen deprivation therapy (78,79). MYLK catalyzes the phosphorylation of myosin light chain and regulates the invasion and metastasis of some malignant tumors including lung cancer, colorectal cancer and breast cancer (22). Lin et al. found that MYLK promotes the progression of hepatocellular carcinoma by altering the cytoskeleton to enhance EMT (80). However, the specific role of these genes in the current therapeutic approaches in PCa is still indistinct and prospective experimental validation is required.
The limitations of our study were as follows: first, our results were not validated at further biological experimental level. Second, the sample size of the involved datasets were comparatively small, and the clinical tumor staging such as TNM stage and Gleason score of the selected samples was inconsistent, possibly ensured under different classification systems/years, which can affect the gene expression due to the high heterogeneity in PCa. Finally, our study only focused on the genes which were identified having significant expression level change between cancerous and non-cancer samples in multiple datasets. But we did not consider other characteristics like age, tumor classification and staging. Therefore, some underlying biological information may be neglected in our study.
Conclusions
In this study, we identified 368 DEGs and 11 hub genes as potential diagnostic biomarkers for PCa based on the integrated bioinformatics analysis. In the 11 hub genes, CAV1, KRT5, SNAI2, and MYLK gene expression level were significantly associated with specific clinical attributes, suggesting application prospects for these genes as biomarker candidates and therapeutic targets. However, these results are based on bioinformatic methods and need further experimental demonstration to reveal their contribution to the pathogenesis of PCa and to verify their feasibility as diagnostic and prognostic markers along with therapeutic targets.
Acknowledgments
Funding: This study was funded by
Footnote
Reporting Checklist: The authors have completed the STREGA reporting checklist. Available at https://tcr.amegroups.com/article/view/10.21037/tcr-22-703/rc
Peer Review File: Available at https://tcr.amegroups.com/article/view/10.21037/tcr-22-703/prf
Conflicts of Interest: All authors have completed the ICMJE uniform disclosure form (available at https://tcr.amegroups.com/article/view/10.21037/tcr-22-703/coif). The authors have no conflicts of interest to declare.
Ethical Statement: The authors are accountable for all aspects of the work in ensuring that questions related to the accuracy or integrity of any part of the work are appropriately investigated and resolved. The study was conducted in accordance with the Declaration of Helsinki (as revised in 2013).
Open Access Statement: This is an Open Access article distributed in accordance with the Creative Commons Attribution-NonCommercial-NoDerivs 4.0 International License (CC BY-NC-ND 4.0), which permits the non-commercial replication and distribution of the article with the strict proviso that no changes or edits are made and the original work is properly cited (including links to both the formal publication through the relevant DOI and the license). See: https://creativecommons.org/licenses/by-nc-nd/4.0/.
References
- Ferlay J, Soerjomataram I, Dikshit R, et al. Cancer incidence and mortality worldwide: sources, methods and major patterns in GLOBOCAN 2012. Int J Cancer 2015;136:E359-86. [Crossref] [PubMed]
- Gandaglia G, Leni R, Bray F, et al. Epidemiology and Prevention of Prostate Cancer. Eur Urol Oncol 2021;4:877-92. [Crossref] [PubMed]
- Kohaar I, Petrovics G, Srivastava S. A Rich Array of Prostate Cancer Molecular Biomarkers: Opportunities and Challenges. Int J Mol Sci 2019;20:1813. [Crossref] [PubMed]
- Ito K. Prostate cancer in Asian men. Nat Rev Urol 2014;11:197-212. [Crossref] [PubMed]
- Zhu Y, Mo M, Wei Y, et al. Epidemiology and genomics of prostate cancer in Asian men. Nat Rev Urol 2021;18:282-301. [Crossref] [PubMed]
- Noureldin M, Eldred-Evans D, Khoo CC, et al. Review article: MRI-targeted biopsies for prostate cancer diagnosis and management. World J Urol 2021;39:57-63. [Crossref] [PubMed]
- Grozescu T, Popa F. Prostate cancer between prognosis and adequate/proper therapy. J Med Life 2017;10:5-12. [PubMed]
- Parsi M, Desai MH, Desai D, et al. PSMA: a game changer in the diagnosis and treatment of advanced prostate cancer. Med Oncol 2021;38:89. [Crossref] [PubMed]
- García-Perdomo HA, Chaves MJ, Osorio JC, et al. Association between TMPRSS2:ERG fusion gene and the prostate cancer: systematic review and meta-analysis. Cent European J Urol 2018;71:410-9. [PubMed]
- Tomlins SA, Aubin SM, Siddiqui J, et al. Urine TMPRSS2:ERG fusion transcript stratifies prostate cancer risk in men with elevated serum PSA. Sci Transl Med 2011;3:94ra72. [Crossref] [PubMed]
- Adamaki M, Zoumpourlis V. Prostate Cancer Biomarkers: From diagnosis to prognosis and precision-guided therapeutics. Pharmacol Ther 2021;228:107932. [Crossref] [PubMed]
- Yu W, Zhou L. Early Diagnosis of Prostate Cancer from the Perspective of Chinese Physicians. J Cancer 2020;11:3264-73. [Crossref] [PubMed]
- Yan Y, Yeon SY, Qian C, et al. On the Road to Accurate Protein Biomarkers in Prostate Cancer Diagnosis and Prognosis: Current Status and Future Advances. Int J Mol Sci 2021;22:13537. [Crossref] [PubMed]
- Li A, He J, Zhang Z, et al. Integrated Bioinformatics Analysis Reveals Marker Genes and Potential Therapeutic Targets for Pulmonary Arterial Hypertension. Genes (Basel) 2021;12:1339. [Crossref] [PubMed]
- Song ZY, Chao F, Zhuo Z, et al. Identification of hub genes in prostate cancer using robust rank aggregation and weighted gene co-expression network analysis. Aging (Albany NY) 2019;11:4736-56. [Crossref] [PubMed]
- Giannos P, Kechagias KS, Gal A. Identification of Prognostic Gene Biomarkers in Non-Small Cell Lung Cancer Progression by Integrated Bioinformatics Analysis. Biology (Basel) 2021;10:1200. [Crossref] [PubMed]
- Giannos P, Kechagias KS, Bowden S, et al. PCNA in Cervical Intraepithelial Neoplasia and Cervical Cancer: An Interaction Network Analysis of Differentially Expressed Genes. Front Oncol 2021;11:779042. [Crossref] [PubMed]
- Ni M, Liu X, Wu J, et al. Identification of Candidate Biomarkers Correlated With the Pathogenesis and Prognosis of Non-small Cell Lung Cancer via Integrated Bioinformatics Analysis. Front Genet 2018;9:469. [Crossref] [PubMed]
- Giannos P, Triantafyllidis KK, Giannos G, et al. SPP1 in infliximab resistant ulcerative colitis and associated colorectal cancer: an analysis of differentially expressed genes. Eur J Gastroenterol Hepatol 2022;34:598-606. [Crossref] [PubMed]
- Hamzeh O, Alkhateeb A, Zheng JZ, et al. A Hierarchical Machine Learning Model to Discover Gleason Grade-Specific Biomarkers in Prostate Cancer. Diagnostics (Basel) 2019;9:219. [Crossref] [PubMed]
- Alkhateeb A, Rezaeian I, Singireddy S, et al. Transcriptomics Signature from Next-Generation Sequencing Data Reveals New Transcriptomic Biomarkers Related to Prostate Cancer. Cancer Inform 2019;18:1176935119835522. [Crossref] [PubMed]
- Liu S, Wang W, Zhao Y, et al. Identification of Potential Key Genes for Pathogenesis and Prognosis in Prostate Cancer by Integrated Analysis of Gene Expression Profiles and the Cancer Genome Atlas. Front Oncol 2020;10:809. [Crossref] [PubMed]
- Wang Y, Wang J, Tang Q, et al. Identification of UBE2C as hub gene in driving prostate cancer by integrated bioinformatics analysis. PLoS One 2021;16:e0247827. [Crossref] [PubMed]
- Zhang P, Qian B, Liu Z, et al. Identification of novel biomarkers of prostate cancer through integrated analysis. Transl Androl Urol 2021;10:3239-54. [Crossref] [PubMed]
- Liang X, Hu K, Li D, et al. Identification of Core Genes and Potential Drugs for Castration-Resistant Prostate Cancer Based on Bioinformatics Analysis. DNA Cell Biol 2020;39:836-47. [Crossref] [PubMed]
- A J, Zhang B, Zhang Z, et al. Novel Gene Signatures Predictive of Patient Recurrence-Free Survival and Castration Resistance in Prostate Cancer. Cancers (Basel) 2021;13:917.
- Cai J, Yang F, Chen X, et al. Signature Panel of 11 Methylated mRNAs and 3 Methylated lncRNAs for Prediction of Recurrence-Free Survival in Prostate Cancer Patients. Pharmgenomics Pers Med 2021;14:797-811. [Crossref] [PubMed]
- Wang X, Kang DD, Shen K, et al. An R package suite for microarray meta-analysis in quality control, differentially expressed gene analysis and pathway enrichment detection. Bioinformatics 2012;28:2534-6. [Crossref] [PubMed]
- Kang DD, Sibille E, Kaminski N, et al. MetaQC: objective quality control and inclusion/exclusion criteria for genomic meta-analysis. Nucleic Acids Res 2012;40:e15. [Crossref] [PubMed]
- Kolde R, Laur S, Adler P, et al. Robust rank aggregation for gene list integration and meta-analysis. Bioinformatics 2012;28:573-80. [Crossref] [PubMed]
- Gao X, Chen Y, Chen M, et al. Identification of key candidate genes and biological pathways in bladder cancer. PeerJ 2018;6:e6036. [Crossref] [PubMed]
- Huang da W. Sherman BT, Lempicki RA. Systematic and integrative analysis of large gene lists using DAVID bioinformatics resources. Nat Protoc 2009;4:44-57. [Crossref] [PubMed]
- Sherman BT, Hao M, Qiu J, et al. DAVID: a web server for functional enrichment analysis and functional annotation of gene lists (2021 update). Nucleic Acids Res 2022; Epub ahead of print. [Crossref] [PubMed]
- Chin CH, Chen SH, Wu HH, et al. cytoHubba: identifying hub objects and sub-networks from complex interactome. BMC Syst Biol 2014;8:S11. [Crossref] [PubMed]
- Tang Z, Li C, Kang B, et al. GEPIA: a web server for cancer and normal gene expression profiling and interactive analyses. Nucleic Acids Res 2017;45:W98-102. [Crossref] [PubMed]
- Xiong Y, Wei Y, Gu Y, et al. DiseaseMeth version 2.0: a major expansion and update of the human disease methylation database. Nucleic Acids Res 2017;45:D888-95. [Crossref] [PubMed]
- Koch A, Jeschke J, Van Criekinge W, et al. MEXPRESS update 2019. Nucleic Acids Res 2019;47:W561-5. [Crossref] [PubMed]
- Koch A, De Meyer T, Jeschke J, et al. MEXPRESS: visualizing expression, DNA methylation and clinical TCGA data. BMC Genomics 2015;16:636. [Crossref] [PubMed]
- Robin X, Turck N, Hainard A, et al. pROC: an open-source package for R and S+ to analyze and compare ROC curves. BMC Bioinformatics 2011;12:77. [Crossref] [PubMed]
- Kamarudin AN, Cox T, Kolamunnage-Dona R. Time-dependent ROC curve analysis in medical research: current methods and applications. BMC Med Res Methodol 2017;17:53. [Crossref] [PubMed]
- Cancer Genome Atlas Research Network. The Molecular Taxonomy of Primary Prostate Cancer. Cell 2015;163:1011-25. [Crossref] [PubMed]
- Kuner R, Fälth M, Pressinotti NC, et al. The maternal embryonic leucine zipper kinase (MELK) is upregulated in high-grade prostate cancer. J Mol Med (Berl) 2013;91:237-48. [Crossref] [PubMed]
- Mortensen MM, Høyer S, Lynnerup AS, et al. Expression profiling of prostate cancer tissue delineates genes associated with recurrence after prostatectomy. Sci Rep 2015;5:16018. [Crossref] [PubMed]
- Arredouani MS, Lu B, Bhasin M, et al. Identification of the transcription factor single-minded homologue 2 as a potential biomarker and immunotherapy target in prostate cancer. Clin Cancer Res 2009;15:5794-802. [Crossref] [PubMed]
- Aboubakar Nana F, Vanderputten M, Ocak S. Role of Focal Adhesion Kinase in Small-Cell Lung Cancer and Its Potential as a Therapeutic Target. Cancers (Basel) 2019;11:1683. [Crossref] [PubMed]
- Ok Atılgan A, Özdemir BH, Yılmaz Akçay E, et al. Association between focal adhesion kinase and matrix metalloproteinase-9 expression in prostate adenocarcinoma and their influence on the progression of prostatic adenocarcinoma. Ann Diagn Pathol 2020;45:151480. [Crossref] [PubMed]
- Xing P, Wang Y, Zhang L, et al. Knockdown of lncRNA MIR4435 2HG and ST8SIA1 expression inhibits the proliferation, invasion and migration of prostate cancer cells in vitro and in vivo by blocking the activation of the FAK/AKT/β catenin signaling pathway. Int J Mol Med 2021;47:93. [Crossref] [PubMed]
- Kolluru V, Tyagi A, Chandrasekaran B, et al. Profiling of differentially expressed genes in cadmium-induced prostate carcinogenesis. Toxicol Appl Pharmacol 2019;375:57-63. [Crossref] [PubMed]
- Tokizane T, Shiina H, Igawa M, et al. Cytochrome P450 1B1 is overexpressed and regulated by hypomethylation in prostate cancer. Clin Cancer Res 2005;11:5793-801. [Crossref] [PubMed]
- Gomez L, Kovac JR, Lamb DJ. CYP17A1 inhibitors in castration-resistant prostate cancer. Steroids 2015;95:80-7. [Crossref] [PubMed]
- Benelli R, Venè R, Ferrari N. Prostaglandin-endoperoxide synthase 2 (cyclooxygenase-2), a complex target for colorectal cancer prevention and therapy. Transl Res 2018;196:42-61. [Crossref] [PubMed]
- Garg R, Blando JM, Perez CJ, et al. COX-2 mediates pro-tumorigenic effects of PKCε in prostate cancer. Oncogene 2018;37:4735-49. [Crossref] [PubMed]
- Janssens R, Struyf S, Proost P. The unique structural and functional features of CXCL12. Cell Mol Immunol 2018;15:299-311. [Crossref] [PubMed]
- Lapeyre-Prost A, Terme M, Pernot S, et al. Immunomodulatory Activity of VEGF in Cancer. Int Rev Cell Mol Biol 2017;330:295-342. [Crossref] [PubMed]
- Lee HT, Sharek L, O'Brien ET, et al. Vinculin and metavinculin exhibit distinct effects on focal adhesion properties, cell migration, and mechanotransduction. PLoS One 2019;14:e0221962. [Crossref] [PubMed]
- Zhu LY, Zhong KB, Lu SX, et al. Vinculin and the androgen receptor in prostate cancer: expressions and correlations. Zhonghua Nan Ke Xue 2010;16:794-8. [PubMed]
- Zheng X, Xu H, Gong L, et al. Vinculin orchestrates prostate cancer progression by regulating tumor cell invasion, migration, and proliferation. Prostate 2021;81:347-56. [Crossref] [PubMed]
- Varambally S, Yu J, Laxman B, et al. Integrative genomic and proteomic analysis of prostate cancer reveals signatures of metastatic progression. Cancer Cell 2005;8:393-406. [Crossref] [PubMed]
- Su AI, Cooke MP, Ching KA, et al. Large-scale analysis of the human and mouse transcriptomes. Proc Natl Acad Sci U S A 2002;99:4465-70. [Crossref] [PubMed]
- Fagerberg L, Hallström BM, Oksvold P, et al. Analysis of the human tissue-specific expression by genome-wide integration of transcriptomics and antibody-based proteomics. Mol Cell Proteomics 2014;13:397-406. [Crossref] [PubMed]
- Ching MM, Reader J, Fulton AM. Eicosanoids in Cancer: Prostaglandin E2 Receptor 4 in Cancer Therapeutics and Immunotherapy. Front Pharmacol 2020;11:819. [Crossref] [PubMed]
- Zhang Z. MiR-124-3p Suppresses Prostatic Carcinoma by Targeting PTGS2 Through the AKT/NF-κB Pathway. Mol Biotechnol 2021;63:621-30. [Crossref] [PubMed]
- Wang XD, Reeves K, Luo FR, et al. Identification of candidate predictive and surrogate molecular markers for dasatinib in prostate cancer: rationale for patient selection and efficacy monitoring. Genome Biol 2007;8:R255. [Crossref] [PubMed]
- Sun X, Cheng G, Hao M, et al. CXCL12 / CXCR4 / CXCR7 chemokine axis and cancer progression. Cancer Metastasis Rev 2010;29:709-22. [Crossref] [PubMed]
- Adekoya TO, Richardson RM. Cytokines and Chemokines as Mediators of Prostate Cancer Metastasis. Int J Mol Sci 2020;21:4449. [Crossref] [PubMed]
- Aakula A, Leivonen SK, Hintsanen P, et al. MicroRNA-135b regulates ERα, AR and HIF1AN and affects breast and prostate cancer cell growth. Mol Oncol 2015;9:1287-300. [Crossref] [PubMed]
- David M, Sahay D, Mege F, et al. Identification of heparin-binding EGF-like growth factor (HB-EGF) as a biomarker for lysophosphatidic acid receptor type 1 (LPA1) activation in human breast and prostate cancers. PLoS One 2014;9:e97771. [Crossref] [PubMed]
- Senetta R, Stella G, Pozzi E, et al. Caveolin-1 as a promoter of tumour spreading: when, how, where and why. J Cell Mol Med 2013;17:325-36. [Crossref] [PubMed]
- Mahmood J, Zaveri SR, Murti SC, et al. Caveolin-1: a novel prognostic biomarker of radioresistance in cancer. Int J Radiat Biol 2016;92:747-53. [Crossref] [PubMed]
- Shi YB, Li J, Lai XN, et al. Multifaceted Roles of Caveolin-1 in Lung Cancer: A New Investigation Focused on Tumor Occurrence, Development and Therapy. Cancers (Basel) 2020;12:291. [Crossref] [PubMed]
- Petpiroon N, Bhummaphan N, Tungsukruthai S, et al. Chrysotobibenzyl inhibition of lung cancer cell migration through Caveolin-1-dependent mediation of the integrin switch and the sensitization of lung cancer cells to cisplatin-mediated apoptosis. Phytomedicine 2019;58:152888. [Crossref] [PubMed]
- Joo JC, Hwang JH, Jo E, et al. Cordycepin induces apoptosis by caveolin-1-mediated JNK regulation of Foxo3a in human lung adenocarcinoma. Oncotarget 2017;8:12211-24. [Crossref] [PubMed]
- Charoenrungruang S, Chanvorachote P, Sritularak B, et al. Gigantol, a bibenzyl from Dendrobium draconis, inhibits the migratory behavior of non-small cell lung cancer cells. J Nat Prod 2014;77:1359-66. [Crossref] [PubMed]
- Karantza V. Keratins in health and cancer: more than mere epithelial cell markers. Oncogene 2011;30:127-38. [Crossref] [PubMed]
- Cimpean AM, Balica RA, Doros IC, et al. Epidermal Growth Factor Receptor (EGFR) and Keratin 5 (K5): Versatile Keyplayers Defining Prognostic and Therapeutic Sub-classes of Head and Neck Squamous Cell Carcinomas. Cancer Genomics Proteomics 2016;13:75-81. [PubMed]
- Ricciardelli C, Lokman NA, Pyragius CE, et al. Keratin 5 overexpression is associated with serous ovarian cancer recurrence and chemotherapy resistance. Oncotarget 2017;8:17819-32. [Crossref] [PubMed]
- Tian X, Tao F, Zhang B, et al. The miR-203/SNAI2 axis regulates prostate tumor growth, migration, angiogenesis and stemness potentially by modulating GSK-3β/β-CATENIN signal pathway. IUBMB Life 2018;70:224-36. [Crossref] [PubMed]
- Cmero M, Kurganovs NJ, Stuchbery R, et al. Loss of SNAI2 in Prostate Cancer Correlates With Clinical Response to Androgen Deprivation Therapy. JCO Precis Oncol 2021;5. ePO. [Crossref] [PubMed]
- Mazzu YZ, Liao Y, Nandakumar S, et al. Dynamic expression of SNAI2 in prostate cancer predicts tumor progression and drug sensitivity. Mol Oncol 2022;16:2451-69. [Crossref] [PubMed]
- Lin J, He Y, Chen L, et al. MYLK promotes hepatocellular carcinoma progression through regulating cytoskeleton to enhance epithelial-mesenchymal transition. Clin Exp Med 2018;18:523-33. [Crossref] [PubMed]