The feasibility of MRI texture analysis in distinguishing glioblastoma, anaplastic astrocytoma and anaplastic oligodendroglioma
Introduction
High-grade gliomas are known as the most common brain malignant tumors in adults and can be pathologically divided into glioblastoma (GBM) (Grade IV), anaplastic astrocytoma (AA) (Grade III) and anaplastic oligodendroglioma (AO) (Grade III) according to the classification of the World Health Organization (WHO) in 2016 (1-5). Lacking specific blood biomarkers and suggestive symptoms, the subtypes of high-grade gliomas could only be distinguished with surgical biopsy (6-8). However, without enough information, individualized treatment and personalized prognostic prediction are unlikely to be introduced. Moreover, the following treatment that patients undertake after surgical resection depends on the accurate pathological diagnosis. The treatment of GBM patients is based on the maximal safe resection, supplemented by standard postoperative radiotherapy plus temozolomide, and then adjuvanted with temozolomide (2,9-11). Patients with AA are recommended to receive radiation therapy or temozolomide after surgery or biopsy (1,10,12,13). For patients with AO, postoperative radiotherapy combined with PCV (procarbazine, lomustine, and vincristine) is a common treatment option (1,5,10,12,14).
Since no specific biomarker for glioma has been identified, the radiological examination is essential in presurgical evaluation. Magnetic resonance imaging (MRI) is commonly used to preliminarily assess the grade of gliomas prior to operation (15-18). However, high-grade gliomas represent similar patterns on MRI, including irregular shape with enhancement shadows and edema rings around the tumor tissue (17-21). At present, doctors interpret images with subjective judgment according to their experiences. But some researchers have extracted quantitative information from images and tried to apply it in tumor diagnosis (20,22-25).
Texture analysis is a novel image processing method that extracts texture features from medical images to obtain quantitative descriptions of lesions (26-31). The texture parameters calculated could provide image information beyond human naked-eye evaluation, including the light intensity distribution and spatial color distribution in the image, quantitative analysis of spatial features, inter-pixel relationships and image greyscale features (32-35). Several studies have applied texture features and pattern recognition techniques in differential diagnosis and prognosis, such as discriminating GBM from single brain metastasis, differentiation of papillary renal cell carcinoma subtypes, and prediction of survival outcomes of pancreatic cancer patient (28,31,36,37). In this research, we adopted the texture analysis-based machine learning technology to establish diagnostic models to presurgically differentiate among GBM, AA and AO. We present the following article in accordance with the STARD reporting checklist (available at https://tcr.amegroups.com/article/view/10.21037/tcr-22-1390/rc).
Methods
Data sets
This was a retrospective, single-center study. In the neurosurgery department of our hospital, 150 patients diagnosed with pathologically diagnostic GBM, AA or AO were randomly selected from January 2015 to December 2018. The inclusion criteria for patients in this study were: (I) with detailed pathological reports; (II) with available high-quality preoperative magnetic resonance (MR) images. The exclusion criteria were: (I) with history of anti-tumor therapy before MR scans, such as chemotherapy or radiotherapy; (II) history of irrelevant brain diseases, such as hypertensive intracerebral hemorrhage or other types of brain tumors. A senior neuropathologist with 10 years of experience adjusted the pathological results based on 2016 WHO glioma classification criteria. We collected preoperative MR images though Picture Archiving and Communication Systems (PACS) system, and reviewed electronic medical records to collect clinical parameters, including gender, age, tumor size, and the time between surgery and MR scan. The study was conducted in accordance with the Declaration of Helsinki (as revised in 2013). The Committee of Sichuan University approved this retrospective study and exempted the need to obtain informed consent for this retrospective study (ID: 2021-S-851).
Sequence selection and MRI acquisition
In this study, we focused on traditional MRI sequences, including contrast-enhanced T1-weighted (T1C) sequence, T1-weighted sequence, T2-weighted sequence and fluid attenuated inversion recovery (FLAIR) sequence. We chose the T1C sequence for texture analysis because it could better visualize the anatomy of the brain and clearly depict the boundary of the tumor. All the images of the included patients were obtained from the radiology department of our hospital.
The 3.0T GE SIGNA MRI scanner was used for MR scanning with the following parameters: Flip angle =9°, TR/TE/TI =1,900/2.26/900 ms, slice thickness =1 mm, field of view =240×240 mm2 and data matrix = 256×256. Multi-directional data for the two-dimensional image of T1C sequence were collected during the interval time of 90–250 s, including axial, parasagittal, and coronal view. Gadopentetate dimeglumine (0.1 mmol/kg) was used as the contrast agent. Figure 1 showed three examples of contrast-enhanced MRI images.
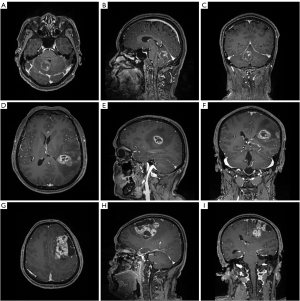
Texture feature extraction
Under the supervision of the senior radiologist, two researchers used LIFEx software to extract texture features from MR images (38). Preprocessing with spatial resampling, intensity discretization and intensity rescaling was performed for all MR images in LIFEx. Region of interest (ROI) was drawn along the boundaries of the tumor tissue on each slice. The primary tumor site was separated from the edema zone around the tumor and the surrounding tissue with different enhancement imaging patterns. Then the software could automatically generate 3D texture features within ROI with randomly selected 64 pixels. From the original images, 40 texture features were extracted, involving 9 first-order features derived from histogram, shape, and conventional statistics, and 31 second-order features derived from gray-level co-occurrence matrix (GLCM), gray-level run length matrix (GLRLM), neighborhood gray-level different matrix (NGLDM), and gray-level zone length matrix (GLZLM).
Establishment of classification model
In order to improve diagnostic performance and avoid over-fitting, texture features should be selected first with suitable methods. Unsure the suitable selection methods, we employed two feature selection methods in training dataset, including distance correlation (DC) and least absolute shrinkage and selection operator (LASSO). The selected features were listed in Table 1. Then the selected parameters were individually introduced into linear discriminant analysis (LDA) algorithm and support vector machine (SVM) algorithm, and four diagnostic models were built based on this principle. Each algorithm was used without hyperparameter tuning.
Table 1
Selection method | Selected features |
---|---|
DC | SHAPE_Volume (mL), GLRLM_LGRE, GLRLM_HGRE, GLRLM_SRLGE, |
GLRLM_SRHGE, GLZLM_LGZE, GLZLM_HGZE, GLZLM_SZLGE | |
LASSO | MinValue, meanValue, stdValue, maxValue, GLCM_Contrast, GLRLM_HGRE |
GLRLM_SRHGE, GLRLM_LRHGE, GLRLM_GLNU, GLRLM_RLNU, GLZLM_LZE | |
GLZLM_HGZE, GLZLM_SZHGE, GLZLM_LZLGE, GLZLM_LZHGE, GLZLM_GLNU | |
GLZLM_ZLNU |
DC, distance correlation; LASSO, least absolute shrinkage and selection operator.
With a ratio of 4:1, patients were randomly assigned to training and validation groups to evaluate the realistic performance of models and reduce over fitting. To assess the diagnostic capabilities of trained models, they were then applied to the validation group. In order to obtain the more accurate performance of models, the training group and the validation group both conducted a repetitive cycle of 100 different data distributions. The workflow from texture features extraction to machine learning modeling was shown in Figure 2.
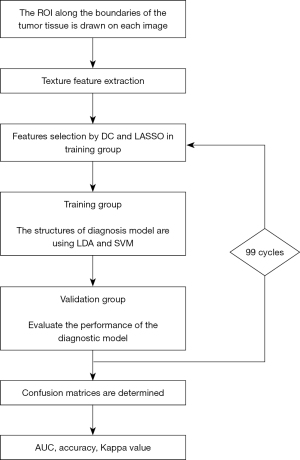
Statistical analysis
In order to evaluate the discriminative ability of models, accuracy, Kappa value, and area under receiver operating characteristic curve (AUC) were calculated for both the training group and the validation group. Function distribution and two-dimensional projection of function were drawn for visual analysis. All statistical analyses of this study were performed by SPSS software (version 21; IBM, Chicago). And the machine learning algorithms were programmed using scikit-learn package and Python Programming Language.
Results
Patient characteristics
A total of 150 patients were enrolled in this study. For the all patients involved in this research, the mean age was 44.93 years old, and the gender ratio of participants was 75:75. A total number of 50 patients were pathologically diagnosed with GBM, 50 patients were diagnosed with AA and 50 patients were diagnosed with AO, respectively. Table 2 showed the patient’s age, gender, tumor size and days between MR scan and surgery.
Table 2
Characteristics | Grade IV glioma | Grade III glioma | P value | ||
---|---|---|---|---|---|
GBM (N=50) | AA (N=50) | AO (N=50) | |||
Age (years) | 46.7 [15–70] | 41.2 [18–67] | 46.9 [16–74] | 0.117 | |
Gender, n (%) | 0.353 | ||||
Male | 22 (44.00) | 29 (58.00) | 24(48.00) | ||
Female | 28 (56.00) | 21 (42.00) | 26 (52.00) | ||
Maximum diameter (cm) | 27.8 | 31.5 | 31.7 | 0.093 | |
Days between MR scan and surgery | 6.4 | 7.2 | 7.8 | 0.058 |
The data are expressed as means or n (%). GBM, glioblastoma; AA, anaplastic astrocytoma; AO, anaplastic oligodendroglioma; MR, magnetic resonance.
Diagnostic ability
AUCs, Kappa values and accuracies of each model were summarized in Table 3. In the validation group, the accuracies of LDA-based models were 76.0% and 74.3%, while for SVM-based model were 58.0% and 63.3%, respectively.
Table 3
Models | Training group | Validation group | ||||||||||
---|---|---|---|---|---|---|---|---|---|---|---|---|
GBM | AA | AO | Kappa value | GBM | AA | AO | Kappa value | AUC | ||||
GBM | AA | AO | ||||||||||
LDA | ||||||||||||
DC | 97.4% | 71.3% | 60.5% | 0.643 (accuracy =76.3%) | 87.0% | 83.0% | 55.4% | 0.639 (accuracy =76.0%) | 0.999 | 0.834 | 0.865 | |
LASSO | 99.0% | 77.8% | 61.0% | 0.685 (accuracy =79.0%) | 87.0% | 85% | 47.8% | 0.613 (accuracy =74.3%) | 0.945 | 0.790 | 0.821 | |
SVM | ||||||||||||
DC | 100.0% | 17.3% | 64.7% | 0.407 (accuracy =60.4%) | 100.0% | 11.0% | 59.8% | 0.365 (accuracy =58.0%) | 1.00 | 0.725 | 0.821 | |
LASSO | 100.0% | 8.8% | 83.1% | 0.457 (accuracy =63.8%) | 100.0% | 3.0% | 85.9% | 0.451 (accuracy =63.3%) | 0.992 | 0.830 | 0.839 |
GBM, glioblastoma; AA, anaplastic astrocytoma; AO, anaplastic oligodendroglioma; AUC, area under the ROC curve; LDA, linear discriminate analysis; SVM, support vector machine; DC, distance correlation; LASSO, least absolute shrinkage and selection operator; ROC, receiver operating characteristic.
DC + LDA achieved the best performance with the accuracy of 76.0% in the validation group. The AUC and accuracy of GBM were 0.999 and 87.0% respectively, indicating that this diagnostic model had the strong ability to distinguish GBM from AA and AO. However, the AUCs of AA and AO were 0.834 and 0.865 respectively, suggesting that the diagnostic ability in differentiation between AA and AO was relatively poor and led to a decrease in the overall accuracy.
Figure 3 showed the relationship between the canonical discriminative functions of the GBM, AA and AO groups and for each of group centroids in DC + LDA model. It can be observed from the overlap that the DC + LDA model had a good ability in distinguishing GBM from the other two tumors, but cannot clearly differentiate AA from AO. Figure 4 showed a DC + LDA model for one of the 100 independent validation cycles, which conformed for distribution of canonical functions. The obvious shifts of LDA function can be observed, with a negative shift for GBM and positive shifts for AA and AO.
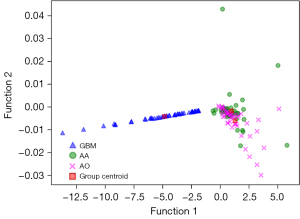
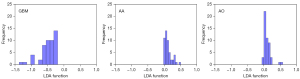
Discussion
Accurate classification of high-grade gliomas is significantly valuable in clinic because it is related to the treatment strategy, treatment response and prognosis of the patient. MR scan is the most important presurgical radiological examination. However, high-grade gliomas always represent similar image patterns, leading to inaccurate diagnosis in naked-eye assessment. Advanced MR technology, such as diffusion tensor imaging (DTI), also represented limited diagnostic value (39,40). Nowadays, the diagnosis of three subtypes of tumors mainly depends on histological and molecular pathology examination, requiring the tissue sample collected in the surgery or stereotactic biopsy. Therefore, we hope to maximize the utility of preoperative MR images to noninvasively identify the subtypes of high-grade gliomas.
Texture analysis, one branch of radiomics, can provide the spatial information of histological heterogeneity beyond visual assessment. Several studies have shown that texture parameters can reflect tumor edema, hemorrhage, necrosis, exudation, calcification, which provides a viable mechanism for the identification of GBM, AA and AO (36,41,42). Moreover, quantitative features extracted from the lesion image can be analyzed with novel computer technology, such as machine learning and deep learning algorithms (43,44). Previous studies suggested that machine learning based on texture analysis could be applied to the diagnosis and classification of brain tumors, such as low-grade gliomas from high-grade gliomas, GBM from primal central never system lymphoma (32,33,35,41,45,46). In previous researches, the best AUC of texture analysis-based models in distinguishing low and high-grade gliomas was 0.90 (46), for another, texture analysis on conventional preoperative MRI images can accurately predict early malignant transformation of low-grade glioma with AUC of 0.96 (35). The results suggested that machine learning-model had potential in improving accuracy of early diagnosis and reducing clinical workload. However, researches on high-grade gliomas were limited and most previous studies focused on distinguishing between two types of tumors (47-50). For this reason, we employed radiomics-based machine learning technology to distinguish among GAM, AA and AO, and this multi-classification study was more suitable for clinical requirements.
Feature selection is a measure of statistics dependence between two random variables or two random vectors of arbitrary, which can test each feature, measure the relationship between the feature and the response variable, and discard the bad features according to the evaluation. As an important process of machine learning, feature selection is able to reduce the irrelevant or redundant features, simplify and improve accuracy of the model. Our results also indicated that selection methods may affect the model performance. According to the mechanism, the selection methods for feature can be divided into three types: (I) Filter Method selects the features of data sets before the training of learners; (II) Wrapper Method selects the feature selection based on the results of machine learning, and its performance is better than filter method; (III) Embedded Method automatically selects the features during the training of learners (51). Two selection methods with different mechanisms were applied in this study: DC as the representative of Filter Method; LASSO as the representative of Embedded Method. The mutual features selected with both DC and LASSO indicated that they may play an important role in classification. The results suggested that DC can select the most sensitive and specific features. GLRLM_HGRE, GLRLM_SRHGE, and GLZLM_HGZE were the most frequently selected features, which are related to the size of homogenous grey-level runs for each grey level. They calculated the distribution of the high and low grey level runs in ROI, indicating that the heterogeneity of the tumor made the grey pixels of the image change. Therefore, it is reasonable to consider that this MRI feature is closely related to these texture features.
With LDA/SVM classifiers, four models were established in the current study. LDA is a classical classification algorithm and has a good performance in many previous studies (52-54). The results showed that the DC + LDA model had the promising diagnostic performance, especially in differentiation of grade III glioma (AA and AO) from grade IV glioma (GBM) with AUC more than 0.99. AA and AO have high consistency in growth and invasion, so features extracted from image also share statistical similarity (17-19,21,55), which may lead to the model’s not particularly satisfactory performance in predicting AA and AO, with the AUC of 0.834 and 0.865. However, the diagnosis model still had the recognition ability beyond the expectation and would be optimized in the subsequent research. In our study, we also found that the accuracy of LDA was higher than that of SVM. This may be due to the sensitivity of SVM feature selection, and SVM usually only supports binary classification algorithm, but has some shortcomings in multi classification problem.
Our study has some limitations. First, it was a retrospective, single-center survey with inherent selection bias. Second, the results were severely limited by patient sample size, and a larger sample size was required to improve our preliminary study. Third, the computer technology is developing rapidly in recent years, and novel classifiers were not evaluated in the current research. Fourth, we only used conventional MR images in this study, and the combination of other MRI sequences or advanced technology [such as magnetic resonance spectroscopy (MRS) and positron emission computed tomography (PET)] may provide more valuable parameters to improve performance. Last and foremost, diagnostic models were not validated in external datasets. Different MR scanners and imaging protocols may lead to uncertainty of our results. However, given the analysis protocols and image processing software we used were open-source, future researchers could repeat and verify our results.
Conclusions
In this study, we extracted texture features from the contrast-enhanced MRI images and analyzed the features using five machine learning models. The results showed that MRI texture analysis with LDA algorithm had the potential to distinguish GBM, AA and AO. The DC + LDA model had the best diagnostic performance in this study. Multi-center studies including larger patient cohort and more machine learning classifiers combined with other MRI sequences are warranted to verify our results.
Acknowledgments
Funding: This study has received funding from
Footnote
Reporting Checklist: The authors have completed the STARD reporting checklist. Available at https://tcr.amegroups.com/article/view/10.21037/tcr-22-1390/rc
Data Sharing Statement: Available at https://tcr.amegroups.com/article/view/10.21037/tcr-22-1390/dss
Conflicts of Interest: All authors have completed the ICMJE uniform disclosure form (available at https://tcr.amegroups.com/article/view/10.21037/tcr-22-1390/coif). All authors report that this study has received funding from 1·3·5 project for disciplines of excellence—Clinical Research Incubation Project, West China Hospital, Sichuan University (No. 2020HXFH036). The authors have no other conflicts of interest to declare.
Ethical Statement: The authors are accountable for all aspects of the work in ensuring that questions related to the accuracy or integrity of any part of the work are appropriately investigated and resolved. The study was conducted in accordance with the Declaration of Helsinki (as revised in 2013). The Committee of Sichuan University approved this retrospective study and exempted the need to obtain informed consent for this retrospective study (ID: 2021-S-851).
Open Access Statement: This is an Open Access article distributed in accordance with the Creative Commons Attribution-NonCommercial-NoDerivs 4.0 International License (CC BY-NC-ND 4.0), which permits the non-commercial replication and distribution of the article with the strict proviso that no changes or edits are made and the original work is properly cited (including links to both the formal publication through the relevant DOI and the license). See: https://creativecommons.org/licenses/by-nc-nd/4.0/.
References
- Kim YZ, Kim CY, Lim J, et al. The Korean Society for Neuro-Oncology (KSNO) Guideline for WHO Grade III Cerebral Gliomas in Adults: Version 2019.01. Brain Tumor Res Treat 2019;7:63-73. [Crossref] [PubMed]
- Kim YZ, Kim CY, Lim J, et al. The Korean Society for Neuro-Oncology (KSNO) Guideline for Glioblastomas: Version 2018.01. Brain Tumor Res Treat 2019;7:1-9. [Crossref] [PubMed]
- Nabors LB, Portnow J, Ammirati M, et al. NCCN Guidelines Insights: Central Nervous System Cancers, Version 1.2017. J Natl Compr Canc Netw 2017;15:1331-45. [Crossref] [PubMed]
- Rasmussen BK, Hansen S, Laursen RJ, et al. Epidemiology of glioma: clinical characteristics, symptoms, and predictors of glioma patients grade I-IV in the the Danish Neuro-Oncology Registry. J Neurooncol 2017;135:571-9. [Crossref] [PubMed]
- Weller M, van den Bent M, Tonn JC, et al. European Association for Neuro-Oncology (EANO) guideline on the diagnosis and treatment of adult astrocytic and oligodendroglial gliomas. Lancet Oncol 2017;18:e315-29. [Crossref] [PubMed]
- Diamandis P, Aldape KD. Insights From Molecular Profiling of Adult Glioma. J Clin Oncol 2017;35:2386-93. [Crossref] [PubMed]
- Reifenberger G, Wirsching HG, Knobbe-Thomsen CB, et al. Advances in the molecular genetics of gliomas - implications for classification and therapy. Nat Rev Clin Oncol 2017;14:434-52. [Crossref] [PubMed]
- Frosina G. Radiotherapy of High-Grade Gliomas: First Half of 2021 Update with Special Reference to Radiosensitization Studies. Int J Mol Sci 2021;22:8942. [Crossref] [PubMed]
- Stupp R, Brada M, van den Bent MJ, et al. High-grade glioma: ESMO Clinical Practice Guidelines for diagnosis, treatment and follow-up. Ann Oncol 2014;25:iii93-101. [Crossref] [PubMed]
- Weller M, van den Bent M, Hopkins K, et al. EANO guideline for the diagnosis and treatment of anaplastic gliomas and glioblastoma. Lancet Oncol 2014;15:e395-403. [Crossref] [PubMed]
- Yue Q, Gao X, Yu Y, et al. An EGFRvIII targeted dual-modal gold nanoprobe for imaging-guided brain tumor surgery. Nanoscale 2017;9:7930-40. [Crossref] [PubMed]
- Sanai N, Berger MS. Surgical oncology for gliomas: the state of the art. Nat Rev Clin Oncol 2018;15:112-25. [Crossref] [PubMed]
- Caccese M, Padovan M, D’Avella D, et al. Anaplastic Astrocytoma: State of the art and future directions. Crit Rev Oncol Hematol 2020;153:103062. [Crossref] [PubMed]
- Bou Zerdan M, Assi HI. Oligodendroglioma: A Review of Management and Pathways. Front Mol Neurosci 2021;14:722396. [Crossref] [PubMed]
- Aquino D, Gioppo A, Finocchiaro G, et al. MRI in Glioma Immunotherapy: Evidence, Pitfalls, and Perspectives. J Immunol Res 2017;2017:5813951. [Crossref] [PubMed]
- Heßelmann V, Mager AK, Goetz C, et al. Accuracy of High-Field Intraoperative MRI in the Detectability of Residual Tumor in Glioma Grade IV Resections. Rofo 2017;189:519-26. [Crossref] [PubMed]
- Nandu H, Wen PY, Huang RY. Imaging in neuro-oncology. Ther Adv Neurol Disord 2018;11:1756286418759865. [Crossref] [PubMed]
- Thust SC, Heiland S, Falini A, et al. Glioma imaging in Europe: A survey of 220 centres and recommendations for best clinical practice. Eur Radiol 2018;28:3306-17. [Crossref] [PubMed]
- Ideguchi M, Kajiwara K, Goto H, et al. MRI findings and pathological features in early-stage glioblastoma. J Neurooncol 2015;123:289-97. [Crossref] [PubMed]
- Kickingereder P, Neuberger U, Bonekamp D, et al. Radiomic subtyping improves disease stratification beyond key molecular, clinical, and standard imaging characteristics in patients with glioblastoma. Neuro Oncol 2018;20:848-57. [Crossref] [PubMed]
- Hu LS, Hawkins-Daarud A, Wang L, et al. Imaging of intratumoral heterogeneity in high-grade glioma. Cancer Lett 2020;477:97-106. [Crossref] [PubMed]
- Hansen MR, Pan E, Wilson A, et al. Post-gadolinium 3-dimensional spatial, surface, and structural characteristics of glioblastomas differentiate pseudoprogression from true tumor progression. J Neurooncol 2018;139:731-8. [Crossref] [PubMed]
- Hu LS, Ning S, Eschbacher JM, et al. Radiogenomics to characterize regional genetic heterogeneity in glioblastoma. Neuro Oncol 2017;19:128-37. [Crossref] [PubMed]
- Young RJ, Gupta A, Shah AD, et al. Potential utility of conventional MRI signs in diagnosing pseudoprogression in glioblastoma. Neurology 2011;76:1918-24. [Crossref] [PubMed]
- Flies CM, van Leuken KH, Ten Voorde M, et al. Conventional MRI Criteria to Differentiate Progressive Disease From Treatment-Induced Effects in High-Grade (WHO Grade 3-4) Gliomas. Neurology 2022;99:e77-88. [Crossref] [PubMed]
- Narchi H, Mahmoud-Ghoneim D, Skinner A, et al. Texture analysis of periventricular echogenicity on neonatal cranial ultrasound predicts periventricular leukomalacia. J Neonatal Perinatal Med 2013;6:117-24. [Crossref] [PubMed]
- Bisdas S, Shen H, Thust S, et al. Texture analysis- and support vector machine-assisted diffusional kurtosis imaging may allow in vivo gliomas grading and IDH-mutation status prediction: a preliminary study. Sci Rep 2018;8:6108. [Crossref] [PubMed]
- Soni N, Priya S, Bathla G. Texture Analysis in Cerebral Gliomas: A Review of the Literature. AJNR Am J Neuroradiol 2019;40:928-34. [Crossref] [PubMed]
- Chen X, Cheng G, Yang X, et al. Exploring the Value of Features of Lung Texture in Distinguishing Between Usual and Nonspecific Interstitial Pneumonia. Acad Radiol 2022;S1076-6332(22)00335-X. Epub ahead of print. [Crossref] [PubMed]
- Malik N, Geraghty B, Dasgupta A, et al. MRI radiomics to differentiate between low grade glioma and glioblastoma peritumoral region. J Neurooncol 2021;155:181-91. [Crossref] [PubMed]
- Dastmalchian S, Kilinc O, Onyewadume L, et al. Radiomic analysis of magnetic resonance fingerprinting in adult brain tumors. Eur J Nucl Med Mol Imaging 2021;48:683-93. [Crossref] [PubMed]
- Fan Y, Chen C, Zhao F, et al. Radiomics-Based Machine Learning Technology Enables Better Differentiation Between Glioblastoma and Anaplastic Oligodendroglioma. Front Oncol 2019;9:1164. [Crossref] [PubMed]
- Tian Z, Chen C, Fan Y, et al. Glioblastoma and Anaplastic Astrocytoma: Differentiation Using MRI Texture Analysis. Front Oncol 2019;9:876. [Crossref] [PubMed]
- Wang S, Meng M, Zhang X, et al. Texture analysis of diffusion weighted imaging for the evaluation of glioma heterogeneity based on different regions of interest. Oncol Lett 2018;15:7297-304. [Crossref] [PubMed]
- Zhang S, Chiang GC, Magge RS, et al. Texture analysis on conventional MRI images accurately predicts early malignant transformation of low-grade gliomas. Eur Radiol 2019;29:2751-9. [Crossref] [PubMed]
- Yun G, Kim YH, Lee YJ, et al. Tumor heterogeneity of pancreas head cancer assessed by CT texture analysis: association with survival outcomes after curative resection. Sci Rep 2018;8:7226. [Crossref] [PubMed]
- Vendrami CL, Velichko YS, Miller FH, et al. Differentiation of Papillary Renal Cell Carcinoma Subtypes on MRI: Qualitative and Texture Analysis. AJR Am J Roentgenol 2018;211:1234-45. [Crossref] [PubMed]
- Nioche C, Orlhac F, Boughdad S, et al. LIFEx: A Freeware for Radiomic Feature Calculation in Multimodality Imaging to Accelerate Advances in the Characterization of Tumor Heterogeneity. Cancer Res 2018;78:4786-9. [Crossref] [PubMed]
- Cuccarini V, Erbetta A, Farinotti M, et al. Advanced MRI may complement histological diagnosis of lower grade gliomas and help in predicting survival. J Neurooncol 2016;126:279-88. [Crossref] [PubMed]
- Hyare H, Thust S, Rees J. Advanced MRI Techniques in the Monitoring of Treatment of Gliomas. Curr Treat Options Neurol 2017;19:11. [Crossref] [PubMed]
- Alcaide-Leon P, Dufort P, Geraldo AF, et al. Differentiation of Enhancing Glioma and Primary Central Nervous System Lymphoma by Texture-Based Machine Learning. AJNR Am J Neuroradiol 2017;38:1145-50. [Crossref] [PubMed]
- Kim JH, Ko ES, Lim Y, et al. Breast Cancer Heterogeneity: MR Imaging Texture Analysis and Survival Outcomes. Radiology 2017;282:665-75. [Crossref] [PubMed]
- Han L, Kamdar MR. MRI to MGMT: predicting methylation status in glioblastoma patients using convolutional recurrent neural networks. Pac Symp Biocomput 2018;23:331-42. [Crossref] [PubMed]
- Kebir S, Weber M, Lazaridis L, et al. Hybrid 11C-MET PET/MRI Combined With "Machine Learning" in Glioma Diagnosis According to the Revised Glioma WHO Classification 2016. Clin Nucl Med 2019;44:214-20. [Crossref] [PubMed]
- Ditmer A, Zhang B, Shujaat T, et al. Diagnostic accuracy of MRI texture analysis for grading gliomas. J Neurooncol 2018;140:583-9. [Crossref] [PubMed]
- Skogen K, Schulz A, Dormagen JB, et al. Diagnostic performance of texture analysis on MRI in grading cerebral gliomas. Eur J Radiol 2016;85:824-9. [Crossref] [PubMed]
- Skogen K, Schulz A, Helseth E, et al. Texture analysis on diffusion tensor imaging: discriminating glioblastoma from single brain metastasis. Acta Radiol 2019;60:356-66. [Crossref] [PubMed]
- Li X, Zhu H, Qian X, et al. MRI Texture Analysis for Differentiating Nonfunctional Pancreatic Neuroendocrine Neoplasms From Solid Pseudopapillary Neoplasms of the Pancreas. Acad Radiol 2020;27:815-23. [Crossref] [PubMed]
- Li NY, Shi B, Chen YL, et al. The Value of MRI Findings Combined With Texture Analysis in the Differential Diagnosis of Primary Ovarian Granulosa Cell Tumors and Ovarian Thecoma-Fibrothecoma. Front Oncol 2021;11:758036. [Crossref] [PubMed]
- Sahin S, Yildiz G, Oguz SH, et al. Discrimination between non-functioning pituitary adenomas and hypophysitis using machine learning methods based on magnetic resonance imaging-derived texture features. Pituitary 2022;25:474-9. [Crossref] [PubMed]
- Rodriguez-Galiano VF, Luque-Espinar JA, Chica-Olmo M, et al. Feature selection approaches for predictive modelling of groundwater nitrate pollution: An evaluation of filters, embedded and wrapper methods. Sci Total Environ 2018;624:661-72. [Crossref] [PubMed]
- Xie T, Wang Z, Zhao Q, et al. Machine Learning-Based Analysis of MR Multiparametric Radiomics for the Subtype Classification of Breast Cancer. Front Oncol 2019;9:505. [Crossref] [PubMed]
- Han Y, Ma Y, Wu Z, et al. Histologic subtype classification of non-small cell lung cancer using PET/CT images. Eur J Nucl Med Mol Imaging 2021;48:350-60. [Crossref] [PubMed]
- Chen C, Ou X, Wang J, et al. Radiomics-Based Machine Learning in Differentiation Between Glioblastoma and Metastatic Brain Tumors. Front Oncol 2019;9:806. [Crossref] [PubMed]
- van Dijken BRJ, van Laar PJ, Holtman GA, et al. Diagnostic accuracy of magnetic resonance imaging techniques for treatment response evaluation in patients with high-grade glioma, a systematic review and meta-analysis. Eur Radiol 2017;27:4129-44. [Crossref] [PubMed]