Expression of NMU, PPBP and GNG4 in colon cancer and their influences on prognosis
Introduction
In 2021, colon cancer (CC) was the third leading cause of death worldwide (1). The incidence and mortality rates of CC were increasing in the last decade. Although the clinical management of patients with CC has improved after a series of rigorous treatments, due to the lack of effective posterior-line therapy, the 5-year overall survival remains low, which has become a major challenge for global health (2). Therefore, identifying the high risk patients so as to develop precise therapeutic strategy are in urgent need. To accomplish this goal, screening for novel and promising prognostic biomarkers for CC remains the priority.
Bioinformatics is a combination of biological and informatics methods to identify key disease-causing factors to facilitate the exploration of new treatment methods and ultimately solve challenging medical problems, such as cancer. There are a growing number of studies using public databases, such as The Cancer Genome Atlas (TCGA) (3) and Oncomine (4). Statistical tools, such as the R data analysis package and Cytoscape visualization software, provide researchers with more intuitive methods for bioinformatics analysis (5,6). Gene Ontology (GO) (7) and Kyoto Encyclopedia of Genes and Genomes (KEGG, http://www.genetic.jp/) (8) are widely regarded as useful tools for genetic analysis. The Database for Integrated Discovery, Visualization and Annotation (DAVID, http://david.abcc.ncifcrf.gov/) (9) contains comprehensive biological knowledge and a series of analysis tools that can be used to extract genetic biological information. These databases and bioinformatics tools provide much information for tumor research and contribute to precision medicine.
This study was carried out to identify the differentially expressed genes (DEGs) of human CC and analyze their relationships with clinical characteristics. We also evaluated the impact of these genes on clinical prognosis. We present the following article in accordance with the REMARK reporting checklist (available at https://tcr.amegroups.com/article/view/10.21037/tcr-22-1377/rc).
Methods
DEGs screening, signaling pathway enrichment and functional enrichment analysis
The study design is demonstrated in the flow diagram (Figure 1). All clinical and mRNA data of CC and tumor-adjacent tissues were obtained from the TCGA database (https://portal.gdc.cancer.gov) for DEG screening. R software was used to convert gene expression into numerical values, average repeated genes, filter low-expressed genes, and draw heat maps and volcano maps. A false discovery rate (FDR) <0.05 and fold change (|log2FC|) >2 were set as the criteria to screen statistically significant DEGs. The KEGG pathway enrichment and GO enrichment analyses of the hub genes were executed in the DAVID online tool (https://david.ncifcrf.gov). Statistical significance was defined with a P value beneath 5%.
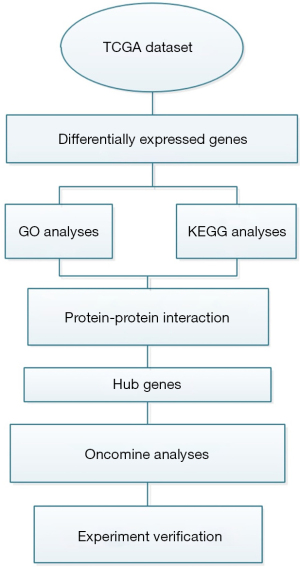
Protein-protein interaction (PPI) network construction
DEGs were imported into the STRING (10) online tool (https://string-db.org/) to assemble a PPI network. The criteria for protein interaction screening were set (confidence >0.9). The network was reconstructed by the degree algorithm of cytoHubba in Cytoscape software v3.7.1 (11) (http://www.cytoscape.org). This module was applied to obtain the top ten hub genes.
Oncomine database screening
To identify the overlapping key genes across the Oncomine (www.oncomine.org) and TCGA databases, the hub genes obtained from the cytoHubba module were searched in the Oncomine database for cross selection. Therefore, the genes screened out both in TCGA and Oncomine were obtained.
Cell culture and quantitative reverse transcription polymerase chain reaction (qRT-PCR)
Human normal colonic epithelial cells NCM460 (RRID:CVCL_0460) and human colorectal cancer cells RKO (AddexBio Cat# C0009012/374, RRID:CVCL_0504), SW480 (Abcam Cat# ab271146, RRID:CVCL_0546), SW620 (AddexBio Cat# C0009002/68, RRID:CVCL_0547), HCT116 (Abcam Cat# ab255451, RRID:CVCL_0291) and COLO678 (DSMZ Cat# ACC-194, RRID:CVCL_1129) were obtained from the Cell Bank of the National Collection of Authenticated Cell Cultures (Shanghai, China). All cell lines were cultured in high glucose DMEM containing 10% fetal bovine serum and 1% penicillin-streptomycin and maintained in a humidified atmosphere of 95% air and 5% CO2 at 37 ℃.
An EZ-press RNA purification kit was used to extract cellular RNA. A reverse transcription kit was used to synthesize cDNA, and qRT-PCR assays were performed on a LightCycler® 96 thermal cycler. The primer sequences are shown in Table S1. The reagents were purchased from EZBioscience (USA). The work was carried out according to the instructions.
Immunohistochemistry
Tissue microarray sections containing 69 CC samples and 55 adjacent tissue samples were purchased from Weiao Biotechnology (Shanghai, China). The clinical characteristics of the patients are shown in Table S2. Xylene was used for deparaffinization, and graded ethanol dilutions were used to rehydrate the tissue microarray sections. Following incubation at 4 ℃ overnight with rabbit anti-NMU, anti-PPBP, and anti-GNG4 primary antibodies, the peroxide sections were then blocked with 3% hydrogen. After incubation with secondary antibody at room temperature, sections were stained with diaminobenzidine (DAB) and counterstained with hematoxylin. Based on the proportion of positive cells and the integrated staining intensity, the staining scores were evaluated by two pathologists independently. The final score ranged from 0 to 12. The samples with scores of 6–12 were defined as high expression, while the samples with scores of 0–5 were defined as low expression.
Immunoblotting
Total proteins were extracted from the mentioned cells above with RIPA buffer (Beyotime, China) that contained protease and phosphatase inhibitors (Beyotime). Following centrifugation of protein lysates, a bicinchoninic acid assay kit (Pierce, USA) was used to determine the concentration of the supernatants. The final protein lysates were boiled at 100 ℃ for 5 min with 5× loading buffer (Beyotime) and separated on a 15% sodium dodecyl sulfate (SDS) polyacrylamide gel (Fdbio Science, China). The gel was transferred onto a polyvinylidene fluoride (PVDF) membrane (Bio-Rad, USA), which was incubated with methanol for 1 minute. The membrane was blocked in Tris-buffered saline containing 0.1% Tween 20 (TBST), which was added to 5% skimmed milk for 2 h at room temperature and incubated with antibodies against NMU (1:1,000, DF4238, Affinity Biosciences, USA), PPBP (1:1,000, DF6695, Affinity Biosciences, USA), GNG4 (1:1,000, DF9560, Affinity Biosciences, USA), and GAPDH (1:1,000, cat. No. 5174, Cell Signaling Technology, USA) at 4 ℃ overnight. After washing with TBST, the PVDF membrane was incubated with HRP conjugated goat anti-rabbit IgGs (1:10,000, Cell Signaling Technology) for 1 h. Enhanced chemiluminescence (ECL) substrate (Bio-Rad) was used for detection, and GelView 6000Pro (BLT, China) was used for digitizing immunoblots. Band densities were semiquantified by ImageJ 1.52.
Statistical analysis
Spearman correlation was used to analyze the relationship between different parameters. Overall survival was assessed by the Kaplan-Meier method. The area under the curve (AUC) and receiver operating curve (ROC) were applied to assess the accuracy of genes for predicting prognosis. The levels of relative gene expression were calculated using the comparative threshold cycle (2–ΔΔCt) method. Data were analyzed using SPSS 26.0, GraphPad Prism and R software. P<0.05 (bilateral) was considered statistically significant.
Ethical statement
The study was conducted in accordance with the Declaration of Helsinki (as revised in 2013). This article does not contain any studies with human participants or animals performed by any of the authors, and thus, there was no need for ethical approval.
Results
Identification of DEGs in CC
This study collected mRNA and clinical information of 480 CCs and 41 adjacent tissues from TCGA database. A total of 18,449 mRNAs were obtained, and 1,665 mRNAs were found to be differentially expressed, of which 911 were upregulated and 754 were downregulated (Figure 2A).
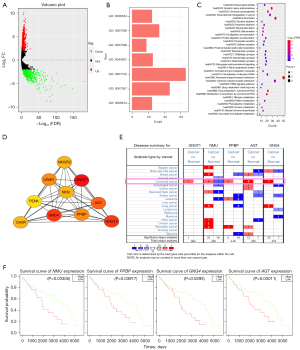
We then performed GO and KEGG enrichment analyses on 1,665 genes using the DAVID web tool. The GO analysis showed that DEGs were primarily involved in seven biological functions (Figure 2B), including nucleosome assembly, coagulation, digestion, cellular protein metabolism, cell adhesion, cell signal transduction and sodium ion transport. The KEGG analysis indicated that DEGs were involved in many biological pathways closely related to cancer progression, such as drug metabolism-cytochrome P450, neuroactive ligand-receptor interactions, alcoholism, chemical carcinogenesis, and metabolism of cytochrome P450 heterologous organisms (Figure 2C). These findings suggested that the DEGs participated extensively in the oncogenesis and progression of CC.
Identification of key genes for prognostic evaluation
We put the proteins encoded by DEGs into the STRING tool to construct a PPI network that was composed of 1,608 nodes and 3,084 edges (Figure S1A). After the PPI network was optimized using Cytoscape, the degree algorithm in cytoHubba was used to screen the top ten hub genes. The correlation between the hub genes (Figure 2D) and gene rankings (Table 1) was obtained. The top ten hub genes were then imported into the Oncomine database to search for key genes. GNGT1, NMU, PPBP, AGT, and GNG4 were found to be differentially expressed in colorectal cancer in the Oncomine database, while the expression of GNG13, LPAR1, NMUR2, CASR and PENK was not significant (Figure 2E).
Table 1
Rank | Name | Score |
---|---|---|
1 | GNGT1 | 106 |
2 | GNG13 | 81 |
2 | GNG4 | 81 |
4 | AGT | 68 |
5 | LPAR1 | 63 |
5 | PPBP | 63 |
7 | NMU | 62 |
7 | NMUR2 | 62 |
7 | CASR | 62 |
10 | PENK | 59 |
The clinical characteristics of the CC cohorts in the TCGA database were extracted, and survival analysis was performed with the five key genes (Figure 2F). Among these, the increased expression of NMU, PPBP, GNG4, and AGT indicated a poor prognosis (P<0.05), while the increased level of GNGT1 did not affect survival (P>0.05) (Figure S1B).
Expression of the key genes in CC cells and tissues
Quantitative RT-PCR was used to detect the mRNA expression levels of the key genes GNG4, NMU, PPBP and AGT in CC cells and normal colon epithelial cells. As shown in Figure 3A, the expression levels of NMU, GNG4, and PPBP mRNA in CC cells were significantly higher than those in normal colon epithelial cells (P<0.05), while the expression of AGT mRNA was similar among the different groups (P>0.05). Then, the protein expression levels of GNG4, PPBP and NMU in CC cells and normal epithelial cells were examined (Figure 3B). We found that both the mRNA and protein expression levels of GNG4, PPBP and NMU were increased in CC cells compared to normal epithelial cells.
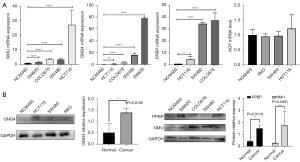
To confirm the expression of the 3 key genes in human CC, we detected the expression of GNG4, PPBP and NMU in a tissue microarray including 69 CC and 55 adjacent normal tissues by immunohistochemistry. The results showed that the positive staining of NMU and GNG4 was mainly located in the cytoplasm, while PPBP was mainly located in the cytoplasm and interstitium of CC cells. The score of each protein was calculated, and a scatter plot involving each case was drawn (Figure 4A-4C). The expression scores of NMU, GNG4 and PPBP in CC and adjacent tissues were 8.246±3.863 vs. 1.761±2.013 (P<0.0001), 7.667±3.677 vs. 2.109±2.034 (P<0.0001), and 5.667±5.011 vs. 3.364±3.335 (P<0.0001), respectively. These results suggested that NMU, GNG4 and PPBP were overexpressed in CC tissues compared with adjacent normal tissues.
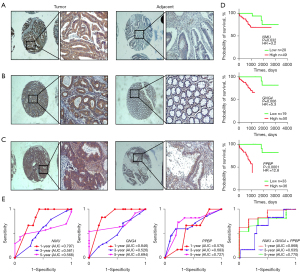
Prognostic biomarkers of CC
We then explored the correlation between the expression of NMU, GNG4, and PPBP and the clinical characteristics of the CC patients in the tissue microarray cohort (Table 2). The results showed that positive expression of PPBP was associated with lymph node metastasis, distant metastasis and advanced tumor stages (P<0.05).
Table 2
Clinical characteristics | No. | NMU | GNG4 | PPBP | |||||||||||
---|---|---|---|---|---|---|---|---|---|---|---|---|---|---|---|
Low N=20 | High N=49 | r | P | Low N=19 | High N=50 | r | P | Low N=33 | High N=36 | r | P | ||||
Gender | |||||||||||||||
F | 35 | 9 | 26 | −0.073 | 0.550 | 10 | 25 | 0.024 | 0.848 | 18 | 17 | 0.073 | 0.550 | ||
M | 34 | 11 | 23 | 9 | 25 | 15 | 19 | ||||||||
Age | |||||||||||||||
<65 | 24 | 6 | 18 | −0.064 | 0.600 | 7 | 17 | 0.027 | 0.828 | 11 | 13 | −0.029 | 0.812 | ||
≥65 | 45 | 14 | 31 | 12 | 33 | 22 | 23 | ||||||||
Pathological type | |||||||||||||||
Poor | 66 | 18 | 48 | −0.177 | 0.146 | 17 | 49 | −0.187 | 0.124 | 31 | 35 | −0.080 | 0.511 | ||
Well | 3 | 2 | 1 | 2 | 1 | 2 | 1 | ||||||||
Node metastasis | |||||||||||||||
No | 46 | 15 | 31 | 0.113 | 0.356 | 16 | 30 | 0.229 | 0.058 | 26 | 20 | 0.246 | 0.041* | ||
Yes | 23 | 5 | 18 | 3 | 20 | 7 | 16 | ||||||||
Distant metastasis | |||||||||||||||
No | 61 | 20 | 41 | 0.231 | 0.056 | 18 | 43 | 0.122 | 0.318 | 32 | 29 | 0.256 | 0.034* | ||
Yes | 8 | 0 | 8 | 1 | 7 | 1 | 7 | ||||||||
TNM stage | |||||||||||||||
I/II | 44 | 15 | 29 | 0.149 | 0.221 | 15 | 29 | 0.195 | 0.109 | 25 | 19 | 0.239 | 0.048* | ||
III/IV | 25 | 5 | 20 | 4 | 21 | 8 | 17 |
*, statistically significant (P<0.05). TNM, tumor-node-metastasis.
We used the Kaplan-Meier method to validate the relevance between the expression of NMU, GNG4, and PPBP and the overall survival of 69 CC cases (Figure 4D). At the significance level of 5%, the results of univariate analysis showed that the survival time of patients with high NMU, GNG4, PPBP expression and distant metastasis was shorter than that of the patients with low expression, and without distant metastasis (Table 3, NMU HR =3.5, P=0.019; GNG4 HR =3.1, P=0.034; PPBP HR =12.0, P<0.0001, distant metastasis HR =3.5, P=0.018). Moreover, the multivariate analysis showed that high NMU, GNG4 expression and low grade of differentiation would shorten the survival time of CC patients (Table 4). The results indicated that NMU, GNG4 and PPBP were adverse prognostic biomarkers of CC.
Table 3
Variables | Univariate analysis | ||
---|---|---|---|
HR | 95% CI | P value | |
GNG4 expression* | 3.1 | 1.2–8.0 | 0.034* |
PPBP expression* | 12.0 | 4.7–30.8 | <0.0001* |
NMU expression* | 3.5 | 1.4–9.0 | 0.019* |
Sex | 1.9 | 0.7–4.8 | 0.183 |
Age (≥65) | 1.1 | 0.4–2.8 | 0.913 |
Grade | 2.4 | 0.3–19.5 | 0.230 |
Node metastasis | 1.9 | 0.7–5.1 | 0.181 |
Distant metastasis | 3.5 | 0.6–19.8 | 0.018* |
TNM stage | 1.7 | 0.6–4.7 | 0.231 |
*, statistically significant (P<0.05). HR, hazard ratio; CI, confidence interval; TNM, tumor-node-metastasis.
Table 4
Variables | Multivariate analysis | ||||||||
---|---|---|---|---|---|---|---|---|---|
HR | 95% CI | P value | HR | 95% CI | P value | HR | 95% CI | P value | |
GNG4 expression* | 10.3 | 1.1–97.0 | 0.041* | ||||||
NMU expression* | 13.3 | 1.5–120.5 | 0.021* | ||||||
PPBP expression* | – | – | 0.570 | ||||||
Sex | 2.5 | 0.9–7.4 | 0.090 | 3.0 | 1.0–8.7 | 0.048 | 2.4 | 0.8–7.1 | 0.110 |
Age (≥65) | 1.4 | 0.5–3.8 | 0.543 | 1.5 | 0.5–4.0 | 0.463 | 1.4 | 0.5–3.9 | 0.483 |
Grade | 14.9 | 1.8–120.7 | 0.011* | 17.6 | 2.2–140.7 | 0.007* | 19.6 | 2.2–168.1 | 0.007* |
Node metastasis | – | – | 0.925 | – | – | 0.925 | – | – | 0.931 |
Distant metastasis | 3.1 | 0.8–12.8 | 0.115 | 2.6 | 0.6–10.7 | 0.186 | 1.9 | 0.4–7.8 | 0.390 |
TNM stage | – | – | 0.925 | – | – | 0.926 | – | – | 0.931 |
HR, hazard ratio; CI, confidence interval; TNM, tumor-node-metastasis.
Subsequently, we drew the ROC to evaluate the sensitivity and specificity of NMU, GNG4 and PPBP in predicting the survival of CC (Figure 4E). The combination of these three key genes predicted the overall survival of CC patients with AUCs of 0.868 (1 year), 0.635 (3 years) and 0.770 (5 years) respectively. These results suggested that the combination model surpassed the single biomarker in predicting the prognosis of CC.
Discussion
Although a substantial number of studies have declared that biomarkers are related to CC, only a few markers showed prognostic value (12-16). In this study, we explore the potential molecules that affect the prognosis of patients with CC through a combination of bioinformatics and experimental validation. A total of 1,665 CC-related DEGs were identified. Among these, NMU, PPBP and GNG4 were found associated to the prognosis of CC. Moreover, the expression levels of NMU, PPBP and GNG4 in CC cells and tissue microarray were validated. The expression levels of NMU, PPBP and GNG4 were upregulated in CC cells and tissues, which were consistent with the findings in the TCGA database. These biomarkers may help improve risk stratification, treatment decisions, and prognosis prediction for patients with CC.
NMU is a member of the neuroprotein family that has a highly conserved sequence neuropeptide. NMU is mainly found in the pituitary gland and gastrointestinal tract (17). The “U” in NMU derives from its ability to stimulate strong contractions in the rat uterine smooth muscle (18). It has been revealed that NMU played an important role in regulating immunity, regulating feeding behavior, controlling circadian rhythms and balancing energy metabolism (19). Besides, previous studies have shown that as a neuropeptide, NMU is also related to poor survival in cancers, especially colorectal cancer (13,14,20-24).
PPBP was highly expressed in lymph node and peritoneal metastasis specimens of gastric cancer, which could be associated with the CXCR2 signaling pathway (25). Interference with the CXCR2/PPBP signaling pathway might be a new option for reversing the resistance of CC patients with liver metastases to conversion therapy (26). In addition, Kinouchi et al. found that the abnormal expression of PPBP in peripheral blood cells contributed to the diagnosis of renal cell carcinoma (RCC) (27).
Previous studies of GNG4 mainly focused on the nervous system, suggesting that GNG4 was related to cognitive decline and glioblastoma (28,29). However, recent studies found that GNG4 could be an adverse marker of rectal cancer and gallbladder cancer (30-32). Angiotensinogen (AGT) deficiency is related to inflammatory bowel disease and the development of cancer (33). Studies of AGT in CC have mainly focused on the field of liver metastasis (33-36). In the current study, however, we were not able to validate the differential expression of AGT in CC cells.
Although there were some bioinformatics analyses exploring the DEGs in CC using Gene Expression Omnibus (GEO) or TCGA or both databases (13-16,37) for diagnostic and/or prognostic purposes, overlapping genes were identified consistent with our study, and seldom did they verify their findings with cells and tissue cohorts. Our study made up for this deficiency and focused on the prognostic value of these biomarkers. Nevertheless, the pathophysiological roles and the mechanisms of these 3 key genes participating in the oncogenesis and progression of CC remain to be clarified.
In conclusion, our study found that the combination model of NMU, PPBP and GNG4 expression predicted the overall survival of patients with CC at 1 and 5 years with high accuracy. They may serve as reliable prognostic biomarkers for CC.
Acknowledgments
Funding: This work was supported by
Footnote
Reporting Checklist: The authors have completed the REMARK reporting checklist. Available at https://tcr.amegroups.com/article/view/10.21037/tcr-22-1377/rc
Conflicts of Interest: All authors have completed the ICMJE uniform disclosure form (available at https://tcr.amegroups.com/article/view/10.21037/tcr-22-1377/coif). All authors report that this work was supported by the Key Laboratory of Malignant Tumor Molecular Mechanism and Translational Medicine of Guangzhou Bureau of Science and Information Technology (No. 2013,163) and the Key Laboratory of Malignant Tumor Gene Regulation and Target Therapy of Guangdong Higher Education Institutes (No. KLB09001). The authors have no other conflicts of interest to declare.
Ethical Statement: The authors are accountable for all aspects of the work in ensuring that questions related to the accuracy or integrity of any part of the work are appropriately investigated and resolved. The study was conducted in accordance with the Declaration of Helsinki (as revised in 2013). This article does not contain any studies with human participants or animals performed by any of the authors, and thus, there was no need for ethical approval.
Open Access Statement: This is an Open Access article distributed in accordance with the Creative Commons Attribution-NonCommercial-NoDerivs 4.0 International License (CC BY-NC-ND 4.0), which permits the non-commercial replication and distribution of the article with the strict proviso that no changes or edits are made and the original work is properly cited (including links to both the formal publication through the relevant DOI and the license). See: https://creativecommons.org/licenses/by-nc-nd/4.0/.
References
- Siegel RL, Miller KD, Fuchs HE, et al. Cancer Statistics, 2021. CA Cancer J Clin 2021;71:7-33. [Crossref] [PubMed]
- Bray F, Ferlay J, Soerjomataram I, et al. Global cancer statistics 2018: GLOBOCAN estimates of incidence and mortality worldwide for 36 cancers in 185 countries. CA Cancer J Clin 2018;68:394-424. [Crossref] [PubMed]
- Hutter C, Zenklusen JC. The Cancer Genome Atlas: Creating Lasting Value beyond Its Data. Cell 2018;173:283-5. [Crossref] [PubMed]
- Rhodes DR, Yu J, Shanker K, et al. ONCOMINE: a cancer microarray database and integrated data-mining platform. Neoplasia 2004;6:1-6. [Crossref] [PubMed]
- Ivanov AA, Revennaugh B, Rusnak L, et al. The OncoPPi Portal: an integrative resource to explore and prioritize protein-protein interactions for cancer target discovery. Bioinformatics 2018;34:1183-91. [Crossref] [PubMed]
- Phuong T, Nhung N. Predicting gene function using similarity learning. BMC Genomics 2013;14:S4. [Crossref] [PubMed]
- Harris MA, Clark J, Ireland A, et al. The Gene Ontology (GO) database and informatics resource. Nucleic Acids Res 2004;32:D258-61. [Crossref] [PubMed]
- Arakawa K, Kono N, Yamada Y, et al. KEGG-based pathway visualization tool for complex omics data. In Silico Biol 2005;5:419-23. [PubMed]
- Dennis G Jr, Sherman BT, Hosack DA, et al. DAVID: Database for Annotation, Visualization, and Integrated Discovery. Genome Biol 2003;4:3. [Crossref] [PubMed]
- Franceschini A, Szklarczyk D, Frankild S, et al. STRING v9.1: protein-protein interaction networks, with increased coverage and integration. Nucleic Acids Res 2013;41:D808-15. [Crossref] [PubMed]
- Shannon P, Markiel A, Ozier O, et al. Cytoscape: a software environment for integrated models of biomolecular interaction networks. Genome Res 2003;13:2498-504. [Crossref] [PubMed]
- Kadoch C, Hargreaves DC, Hodges C, et al. Proteomic and bioinformatic analysis of mammalian SWI/SNF complexes identifies extensive roles in human malignancy. Nat Genet 2013;45:592-601. [Crossref] [PubMed]
- Gong B, Kao Y, Zhang C, et al. Identification of Hub Genes Related to Carcinogenesis and Prognosis in Colorectal Cancer Based on Integrated Bioinformatics. Mediators Inflamm 2020;2020:5934821. [Crossref] [PubMed]
- Chen L, Lu D, Sun K, et al. Identification of biomarkers associated with diagnosis and prognosis of colorectal cancer patients based on integrated bioinformatics analysis. Gene 2019;692:119-25. [Crossref] [PubMed]
- Liang B, Li C, Zhao J. Identification of key pathways and genes in colorectal cancer using bioinformatics analysis. Med Oncol 2016;33:111. [Crossref] [PubMed]
- Zhao ZW, Fan XX, Yang LL, et al. The identification of a common different gene expression signature in patients with colorectal cancer. Math Biosci Eng 2019;16:2942-58. [Crossref] [PubMed]
- Domin J, Polak JM, Bloom SR. The distribution and biological effects of neuromedins B and U. Ann N Y Acad Sci 1988;547:391-403. [Crossref] [PubMed]
- Raddatz R, Wilson AE, Artymyshyn R, et al. Identification and characterization of two neuromedin U receptors differentially expressed in peripheral tissues and the central nervous system. J Biol Chem 2000;275:32452-9. [Crossref] [PubMed]
- Teranishi H, Hanada R. Neuromedin U, a Key Molecule in Metabolic Disorders. Int J Mol Sci 2021;22:4238. [Crossref] [PubMed]
- Wang X, Chen X, Zhou H, et al. The Long Noncoding RNA, LINC01555, Promotes Invasion and Metastasis of Colorectal Cancer by Activating the Neuropeptide, Neuromedin U. Med Sci Monit 2019;25:4014-24. [Crossref] [PubMed]
- Wang YP, Chen C, Li LX, et al. Neuromedin U expression related to the occurrence of laryngeal carcinoma and the regional lymph node metastasis. Lin Chung Er Bi Yan Hou Tou Jing Wai Ke Za Zhi 2016;30:811-4. [PubMed]
- Li Q, Han L, Ruan S, et al. The prognostic value of neuromedin U in patients with hepatocellular carcinoma. BMC Cancer 2020;20:95. [Crossref] [PubMed]
- Przygodzka P, Papiewska-Pajak I, Bogusz H, et al. Neuromedin U is upregulated by Snail at early stages of EMT in HT29 colon cancer cells. Biochim Biophys Acta 2016;1860:2445-53. [Crossref] [PubMed]
- Zhang JJ, Hong J, Ma YS, et al. Identified GNGT1 and NMU as Combined Diagnosis Biomarker of Non-Small-Cell Lung Cancer Utilizing Bioinformatics and Logistic Regression. Dis Markers 2021;2021:6696198. [Crossref] [PubMed]
- Yamamoto Y, Kuroda K, Sera T, et al. The Clinicopathological Significance of the CXCR2 Ligands, CXCL1, CXCL2, CXCL3, CXCL5, CXCL6, CXCL7, and CXCL8 in Gastric Cancer. Anticancer Res 2019;39:6645-52. [Crossref] [PubMed]
- Desurmont T, Skrypek N, Duhamel A, et al. Overexpression of chemokine receptor CXCR2 and ligand CXCL7 in liver metastases from colon cancer is correlated to shorter disease-free and overall survival. Cancer Sci 2015;106:262-9. [Crossref] [PubMed]
- Kinouchi T, Uemura M, Wang C, et al. Expression level of CXCL7 in peripheral blood cells is a potential biomarker for the diagnosis of renal cell carcinoma. Cancer Sci 2017;108:2495-502. [Crossref] [PubMed]
- Bonham LW, Evans DS, Liu Y, et al. Neurotransmitter Pathway Genes in Cognitive Decline During Aging: Evidence for GNG4 and KCNQ2 Genes. Am J Alzheimers Dis Other Demen 2018;33:153-65. [Crossref] [PubMed]
- Pal J, Patil V, Mondal B, et al. Epigenetically silenced GNG4 inhibits SDF1α/CXCR4 signaling in mesenchymal glioblastoma. Genes Cancer 2016;7:136-47. [Crossref] [PubMed]
- Zhu D, Gu X, Lin Z, et al. High expression of PSMC2 promotes gallbladder cancer through regulation of GNG4 and predicts poor prognosis. Oncogenesis 2021;10:43. [Crossref] [PubMed]
- Zhao H, Sheng D, Qian Z, et al. Identifying GNG4 might play an important role in colorectal cancer TMB. Cancer Biomark 2021;32:435-50. [Crossref] [PubMed]
- Lv QY, Zou HZ, Xu YY, et al. Expression levels of chemokine (C-X-C motif) ligands CXCL1 and CXCL3 as prognostic biomarkers in rectal adenocarcinoma: evidence from Gene Expression Omnibus (GEO) analyses. Bioengineered 2021;12:3711-25. [Crossref] [PubMed]
- Kuniyasu H. Multiple roles of angiotensin in colorectal cancer. World J Clin Oncol 2012;3:150-4. [Crossref] [PubMed]
- Zhou L, Luo Y, Sato S, et al. Role of two types of angiotensin II receptors in colorectal carcinoma progression. Pathobiology 2014;81:169-75. [Crossref] [PubMed]
- Shimomoto T, Ohmori H, Luo Y, et al. Diabetes-associated angiotensin activation enhances liver metastasis of colon cancer. Clin Exp Metastasis 2012;29:915-25. [Crossref] [PubMed]
- Neo JH, Ager EI, Angus PW, et al. Changes in the renin angiotensin system during the development of colorectal cancer liver metastases. BMC Cancer 2010;10:134. [Crossref] [PubMed]
- Sun G, Li Y, Peng Y, et al. Identification of differentially expressed genes and biological characteristics of colorectal cancer by integrated bioinformatics analysis. J Cell Physiol 2019; Epub ahead of print. [Crossref] [PubMed]