Prognostic biomarkers of pancreatic cancer identified based on a competing endogenous RNA regulatory network
Introduction
Pancreatic cancer is a relatively uncommon disease but has shown an increased incidence from 0.5% to 1.0% in the US as of 2021 (1). Pancreatic cancer is expected to become the second leading cause of cancer-related death by 2030 (2). Pancreatic ductal adenocarcinoma accounts for most (90%) pancreatic tumors, including acinar carcinoma, pancreaticoblastoma, and pancreatic neuroendocrine tumors (1). Pancreatic ductal adenocarcinoma is also the third leading cause of cancer mortality in the US and seventh leading cause worldwide (3). Most patients suffering from advanced pancreatic cancer exhibit nonspecific symptoms, providing no effective screening for radical surgery. The median age at diagnosis is 71 years in the US (4); only 10–15% of patients exhibit localized resectable pancreatic disease at the time of discovery, 30–35% of patients have vascular involvement leading to mostly local unresectable disease, and 50% of patients exhibit distant metastasis (1). Currently, on the molecular typing of pancreatic cancer, many studies based on proteomics, genomics and transcriptomics have provided new insights into the molecular typing of pancreatic cancer (5-7). Although the molecular classification of pancreatic cancer is not as involved in guiding clinical treatment as breast cancer and colorectal cancer, there are many molecular classifications that are relatively mature and significantly related to prognosis (6-9) Therefore, in-depth exploration of the underlying mechanisms of pancreatic ductal adenocarcinoma and identification of effective prognostic indicators are important for clinical treatment decisions and patient management.
Recent studies revealed that numerous non-coding and coding RNA molecules exist in many tumor tissues, with long non-coding RNAs (lncRNAs), microRNAs (miRNAs), and mRNAs as the most abundant (10-12). The competing endogenous RNA (ceRNA) hypothesis was first proposed by Salmena et al. (13), who suggested that any gene containing miRNA response elements can be combined with miRNA through the miRNA response elements, thereby inhibiting the effect of miRNAs and indirectly regulating the expression of protein-coding genes. ceRNA is a cross-regulatory network that mediates malignant tumor cell phenotypes, including proliferation and inhibition, autophagy, infinite growth, induction of angiogenesis and angiogenic mimicry, and immune escape (14,15). Dong et al. found that the lncRNA GAS5 significantly reduced ovarian cancer cell proliferation and invasion by acting as a ceRNA and suppressing miR-96-5p expression, thereby providing a therapeutic strategy for ovarian cancer treatment (16). Further studies of ceRNAs will contribute to the development of prognostic prediction models. For example, Wang et al. identified eight prognostic biomarkers for prostate cancer: lncRNA LINC01082, miRNA hsa-miR-133a-3p, and the genes TTLL12, PTGDS, GAS6, CYP27A1, PKP3, and ZG16B. These authors then developed a predictive model through weighted gene co-expression network analysis of the ceRNA network (17). Analyzing the ceRNA regulatory network of “lncRNA-miRNA-mRNA” in malignant tumors can provide a new basis and targets for tumor diagnosis, which has substantial value for clinical applications. However, despite the growing body of research on ceRNA in various tumors, such as hepatocellular carcinoma (18) and breast cancer (19), research on pancreatic cancer is lacking.
In this study, we analyzed and compared the expression levels of lncRNA, miRNA, and mRNA in pancreatic adenocarcinoma (PAAD)-related tissues using The Cancer Genome Atlas (TCGA). By constructing a ceRNA regulatory network, we identified key prognostic factors significantly related to PAAD, which were used to construct a model for predicting the survival prognosis of patients. We present the following article in accordance with the TRIPOD reporting checklist (available at https://tcr.amegroups.com/article/view/10.21037/tcr-22-709/rc).
Methods
Data processing
First, we downloaded transcriptome data, including mRNA, lncRNA, and miRNA, and obtained the corresponding clinical data from TCGA (training dataset, https://gdc-portal.nci.nih.gov/). Based on the complete transcriptome data, we screened 165 samples, which included 161 PAAD samples and four adjacent normal samples. We used the Gene Expression Omnibus (https://www.ncbi.nlm.nih.gov/) (20) as the test dataset and obtained transcriptome data and clinical data for 65 cancer tissues from GSE62452 (21). The study was conducted in accordance with the Declaration of Helsinki (as revised in 2013).
Identification of differentially expressed genes (DEGs)
Limma’ package (Version 3.34.0, https://bioconductor.org/packages/release/bioc/html-/limma.html) (22) in R software was used to screen DEGs, including mRNAs, lncRNAs, and miRNAs, between PAAD tissues and adjacent normal tissues from patients with PAAD. The screening conditions were false discovery rate <0.05 and |log2 fold-change (FC)| >0.5. The ‘pheatmap’ package (Version 1.0.8, https://cran.r-project.org/package=pheatmap) (23) in R software was used to conduct unsupervised two-way hierarchical clustering based on Euclidian distances.
Biological network analysis of ceRNA
Biological networks reflect the relationships between genes or between genes and other functions or pathways. Biological network analysis can improve the understanding of how complex interactive networks of genes affect diseases and reveal key genes for further genetic diagnosis and targeted therapy. First, we searched the relationship between differentially expressed lncRNAs and differentially expressed miRNAs using the DIANA-LncBase database (version 3.0, https://diana.e-ce.uth.gr/lncbasev3) (24), retaining only the pairs in which the two expression levels differed in the opposite direction. We then used the StarBase database (version 2.0, http://starbase.sysu.edu.cn/) (25) to search for the target genes regulated by differentially expressed miRNAs, and mapped the differentially expressed mRNAs screened in the previous step to the regulated target genes, retaining only the negative expression levels of miRNA and mRNA. Cytoscape software (version 3.6.1, https://cytoscape.org/) (26) was used to visualize the ceRNA network of lncRNA-miRNA-mRNA. Finally, we performed functional enrichment analysis for differentially expressed mRNAs using the DAVID database (version 6.8, http://metascape.org/) (27), including Gene Oncology and the Kyoto Encyclopedia of Genes and Genomes.
Construction of a prediction model based on the ceRNA network
According to previous ceRNA networks, we performed univariate and multivariate Cox regression analysis on all mRNA-associated genes using the ‘survival’ package in R software (The R Project for Statistical Computing, Vienna, Austria) (28) to identify genes significantly associated with overall survival in TCGA training dataset. Based on genes previously identified as significantly associated with overall survival, we performed least absolute shrinkage and selection operator (LASSO) regression analysis using the ‘lars’ package (29) in R software to identify the optimum combination of genes. Furthermore, the risk score for each patient was calculated using the following formula:
Here Coefgenes represents the LASSO coefficient of the target gene and Exp genes represents the expression level of the target gene in TCGA dataset.
Estimation of the predictive ability of the prediction model
According to the median risk score, we divided all patients from TCGA dataset into high- and low-risk groups. We then plotted Kaplan-Meier survival curves to analyze the difference in survival prognosis between high- and low-risk groups. Receiver operating characteristic (ROC) curves were generated to estimate the predictive power of the model. The GSE62452 dataset was used to validate the predictive ability of the prognostic model.
Screening of clinical factors significantly associated with overall survival
To identify significant prognostic factors for patients with PAAD, we combined traditional prognostic factors and prediction models and performed univariate and multivariate Cox regression analyses. Based on the potential prognostic factors, we plotted decision curves and analyzed the correlation between clinical factors and the prognostic signature.
Examine the relationship between different subtype categories and risk groups
In the PAAD samples we included in the analysis, cluster analysis was performed based on 8 characteristic factors to obtain different subtype categories, and then the relationship between different subtype categories and risk groups was investigated. Afterwards, the correlation between different subtypes and survival prognosis was investigated in subtypes 1 and 2 obtained in the TCGA training set and the GSE62425 validation data set, respectively.
Examine the relationship between tumor purity and risk grouping
We use the estimate package in R3.6.1 to evaluate the TumorPurity of the TCGA and GSE62452 dataset samples respectively, and then use t-test to compare the differences in the TumorPurity in the samples of different risk groups.
Statistical analyses
R software (V.3.6.1) was utilized for data analysis. The significance between the two groups was identified using Wilcox test. The survival time differences between the two risk groups were estimated using Kaplan-Meier curves and log-rank test. The area under the ROC curve (AUC) was calculated to assess the risk signature’s accuracy. Independent factors of OS were determined using univariate along with multivariate Cox regression analyses. P<0.05 was the cut-off of statistical significance.
Results
Identification of DEGs in TCGA dataset
According to the platform annotation information provided in the downloaded data, 18,497 mRNAs, 2,528 lncRNAs, and 2,036 miRNAs were annotated in the dataset; the expression-level data are shown in https://cdn.amegroups.cn/static/public/tcr-22-709-1.xlsx and https://cdn.amegroups.cn/static/public/tcr-22-709-2.xlsx. The ‘Limma’ package was used to screen DEGs that met the threshold conditions, and 636 DEGs were obtained, including 71 lncRNAs, 25 miRNAs and 540 mRNAs. The list of DEGs is shown in https://cdn.amegroups.cn/static/public/tcr-22-709-3.xlsx, and the distribution of DEGs is shown in Figure 1A. The bidirectional hierarchical clustering heat map based on the expression levels of DEGs is shown in Figure 1B.
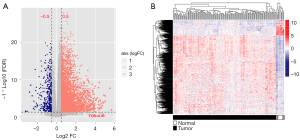
Construction of ceRNA and functional enrichment analysis
We searched the DIANA-LncBase database for interactions between the differentially expressed lncRNAs and miRNAs obtained in the first step, retaining only the linkage pairs with opposite expression differences, and obtained 56 linkage pairs (https://cdn.amegroups.cn/static/public/tcr-22-709-4.xlsx). We searched for target genes regulated by differentially expressed miRNAs using the StarBase database and identified mRNAs obtained in the first step that were significantly differentially expressed from the regulated target genes. We retained only the pairs showing negative correlations between miRNA and mRNA expression levels, and obtained 594 linkage pairs (https://cdn.amegroups.cn/static/public/tcr-22-709-5.xlsx). Next, we constructed a ceRNA regulatory network of lncRNA-miRNA-mRNA after comprehensively analyzing the differentially expressed lncRNAs, miRNAs, and mRNAs screened in this study, as shown in Figure 2. Furthermore, mRNAs in the ceRNA regulatory network were analyzed for functional enrichment, including Gene Ontology biological process and Kyoto Encyclopedia of Genes and Genomes signaling pathway enrichment. Twenty-six significantly related Gene Ontology biological processes and five Kyoto Encyclopedia of Genes and Genomes signaling pathways were obtained, as shown in Table 1; the visualization graphs are shown in Figure 3.
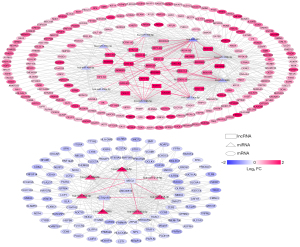
Table 1
Category | Term | Count | P value |
---|---|---|---|
Biology process | GO:0006811~ion transport | 9 | 1.14E-03 |
GO:0007584~response to nutrient | 7 | 1.31E-03 | |
GO:0006955~immune response | 16 | 3.96E-03 | |
GO:0045087~innate immune response | 16 | 4.79E-03 | |
GO:0035725~sodium ion transmembrane transport | 6 | 6.79E-03 | |
GO:0016477~cell migration | 9 | 7.34E-03 | |
GO:0006805~xenobiotic metabolic process | 6 | 8.94E-03 | |
GO:0006954~inflammatory response | 14 | 9.53E-03 | |
GO:0002250~adaptive immune response | 8 | 1.09E-02 | |
GO:0000281~mitotic cytokinesis | 4 | 1.15E-02 | |
GO:0050900~leukocyte migration | 7 | 1.50E-02 | |
GO:0030277~maintenance of gastrointestinal epithelium | 3 | 1.57E-02 | |
GO:0042102~positive regulation of T cell proliferation | 5 | 1.66E-02 | |
GO:0006952~defense response | 5 | 2.38E-02 | |
GO:0034162~toll-like receptor 9 signaling pathway | 3 | 2.42E-02 | |
GO:0014056~regulation of acetylcholine secretion, neurotransmission | 2 | 3.24E-02 | |
GO:0001808~negative regulation of type IV hypersensitivity | 2 | 3.24E-02 | |
GO:0030334~regulation of cell migration | 5 | 3.28E-02 | |
GO:0045089~positive regulation of innate immune response | 3 | 3.42E-02 | |
GO:0031295~T cell costimulation | 5 | 3.87E-02 | |
GO:0032753~positive regulation of interleukin-4 production | 3 | 4.16E-02 | |
GO:0030148~sphingolipid biosynthetic process | 4 | 4.34E-02 | |
GO:0007352~zygotic specification of dorsal/ventral axis | 2 | 4.82E-02 | |
GO:0009595~detection of biotic stimulus | 2 | 4.82E-02 | |
GO:0008645~hexose transport | 2 | 4.82E-02 | |
GO:0050718~positive regulation of interleukin-1 beta secretion | 3 | 4.95E-02 | |
KEGG pathway | hsa04380:Osteoclast differentiation | 9 | 1.50E-03 |
hsa05140:Leishmaniasis | 5 | 3.06E-02 | |
hsa04726:Serotonergic synapse | 6 | 3.78E-02 | |
hsa04725:Cholinergic synapse | 6 | 3.78E-02 | |
hsa04724:Glutamatergic synapse | 6 | 4.17E-02 |
GO, Gene Ontology; KEGG, Kyoto Encyclopedia of Genes and Genomes.
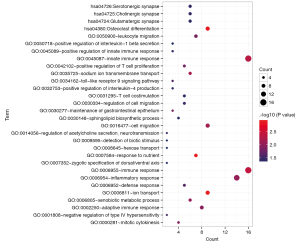
Screening for optimal gene combinations for construction of the prediction model
According to the mRNAs in the previously constructed ceRNA network, 118 genes significantly correlated with prognosis were first screened by univariate Cox regression (https://cdn.amegroups.cn/static/public/tcr-22-709-6.xlsx). This partial mRNAs significantly correlated with prognosis were screened using multivariate Cox regression analysis, and 21 mRNAs independently significantly correlated with prognosis were obtained (https://cdn.amegroups.cn/static/public/tcr-22-709-7.xlsx). LASSO regression was used to screen the genes that were significantly associated with prognosis to identify optimal gene combinations. The parameter map of LASSO screening optimized mRNA is shown in Figure 4A. Eight genes were obtained, and their prognostic information is shown in Figure 4B. The expression levels of eight genes in TCGA dataset are shown in Figure S1.
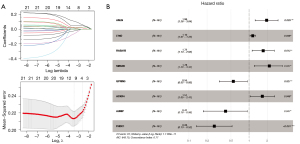
Based on the LASSO regression coefficients of the eight genes and their expression levels in TCGA training dataset, the following formula for the value-at-risk calculation was constructed:
(The 95% confidence interval of RS model is shown in https://cdn.amegroups.cn/static/public/tcr-22-709-8.xlsx).
For the eight optimized mRNA markers obtained through screening, the association between high-value (expression level was higher than or equal to the median expression value) and low-value (expression levels below the median expression value) sample groups of each gene expression level and survival prognosis was assessed using the Kaplan-Meier curve method (Figure 5). The high-risk group showed a shorter overall survival time compared to the low-risk group for five genes (ANLN, LY6D, RAB27B, SMAD6, and AUNIP), and the low-risk group showed a shorter overall survival time compared to the high-risk group for three genes (GPRIN3, ACKR4, and FHDC1). The median OS of each group is shown in https://cdn.amegroups.cn/static/public/tcr-22-709-8.xlsx.
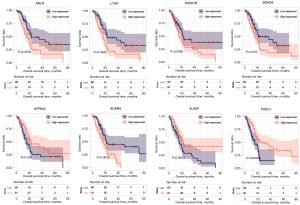
Assessment and comparison of model predictive ability
According to the above formula, we obtained the risk values for each sample in TCGA database (training set); the distribution of risk values versus overall survival time is shown in Figure 6A. Then, samples in TCGA database were divided into high- and low-risk groups based on the median risk values. The results of Kaplan-Meier survival analysis showed that patients in the high-risk group had a significantly worse survival prognosis than those in the low-risk group (P<0.0001). The receiver operating characteristic curve showed that the areas under the curve were 0.929, 0.895, and 0.884 at 1, 3, and 5 years, respectively (shown in Figure 6B), indicating that the eight-gene prediction model had good predictive ability. The 1-, 3-, and 5-year prognostic line graphs based on genetic prognostic features are shown in Figure 6C.
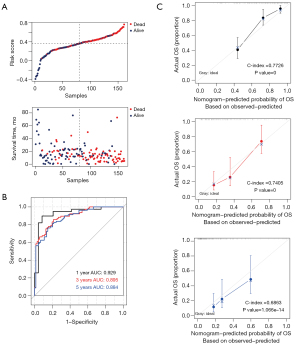
Additionally, samples from the GSE62452 dataset (validation set) were used to assess the stability of the model. Figure 7A shows the relationship between the risk values and survival prognosis for samples from the GSE62452 dataset. Kaplan-Meier survival curves confirmed that patients in the high-risk group had a significantly shorter overall survival time than those in the low-risk group (Figure 7B). Moreover, the areas under the curves at 1, 3, and 5 years were 0.843, 0.802, and 0.791, respectively, confirming the stability of the model. The 1-, 3-, and 5-year prognostic line graphs based on genetic prognostic features are shown in Figure 7C.
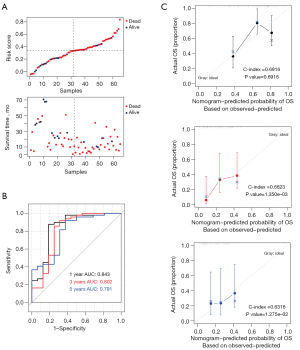
We plotted the Kaplan-Meier curve using the survival package in R3.6.1 software to evaluate the correlation between high and low groups and actual disease prognosis information. The Kaplan-Meier curve of each dataset is shown in Figure 8. In the training and validation data sets, there was a significant correlation between the different risk groups obtained after the samples were predicted based on the RS model and actual prognosis. The RS scores and groupings in the training and validation datasets are shown in https://cdn.amegroups.cn/static/public/tcr-22-709-9.xlsx and https://cdn.amegroups.cn/static/public/tcr-22-709-10.xlsx, respectively. And the clinical information of each symbol in the training dataset is shown in https://cdn.amegroups.cn/static/public/tcr-22-709-11.xlsx.
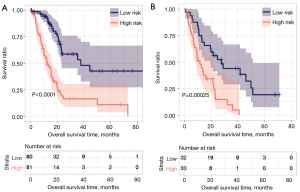
Confirmation of independent prognostic factors
To confirm the independent prognostic factors of pancreatic cancer, we performed univariate and multifactorial Cox regression analyses, as shown in Table 2. We screened three independent prognostic factors: pathological stage, targeted molecular therapy, and the predictive model. We segmented pancreatic cancer samples in TCGA database according to the pathological stage (Figure 9A), and analyzed the correlation between the pathological stage, targeted molecular therapy, and predictive model, respectively (Figure 9B). Then we segmented pancreatic cancer samples in TCGA database according to targeted molecular therapy (Figure 9C), and analyzed the correlation between the pathological stage, targeted molecular therapy, and predictive model, respectively (Figure 9D). The results indicate that high-risk patients with pancreatic cancer had significantly shorter prognostic OS times compared to those of low-risk patients, regardless of whether they received targeted molecular therapy (without target molecular therapy, P<0.0001; with target molecular therapy, P=0.0012). In patients with pathological stages I–II, the prognostic overall survival time of patients with a high RS was significantly shorter than that of patients with low-risk values (P<0.0001).
Table 2
Variables | Univariate analysis | Multivariate analysis | |||||
---|---|---|---|---|---|---|---|
HR | 95% CI | P value | HR | 95% CI | P value | ||
Age (mean ± SD) | 1.030 | 1.008–1.053 | 6.34E-03 | 1.022 | 0.998–1.045 | 6.34E–02 | |
Gender (male/female) | 0.912 | 0.583–1.395 | 6.42E-01 | – | – | – | |
Pathologic_M (M0/M1) | 1.859 | 0.434–7.857 | 3.92E-01 | – | – | – | |
Pathologic_N (N0/N1) | 2.063 | 1.201–3.544 | 5.33E-03 | 1.567 | 0.781–3.141 | 2.06E–01 | |
Pathologic_T (T1/T2/T3/T4) | 1.771 | 1.110–2.822 | 8.67E-03 | 1.109 | 0.508–2.421 | 7.95E–01 | |
Pathologic_stage (I/II/III/IV) | 1.558 | 1.033–2.356 | 3.55E-02 | 1.781 | 1.055–3.005 | 3.07E–02 | |
Chronic pancreatitis history (yes/no) | 1.101 | 0.522–2.317 | 8.01E-01 | – | – | – | |
Diabetes history (yes/no) | 0.906 | 0.506–1.614 | 7.38E-01 | – | – | – | |
Alcohol history (yes/no) | 1.124 | 0.698–1.810 | 6.29E-01 | – | – | – | |
Tobacco history (never/reform/current) | 1.203 | 0.842–1.719 | 3.09E-01 | – | – | – | |
Tumor recurrence (yes/no) | 1.635 | 0.981–2.727 | 6.46E-02 | – | – | – | |
Radiation therapy (yes/no) | 0.513 | 0.286–0.918 | 1.66E-02 | 0.742 | 0.387–1.422 | 3.68E-01 | |
Targeted molecular therapy (yes/no) | 0.492 | 0.311–0.780 | 2.07E-03 | 0.374 | 0.215–0.651 | 5.07E-04 | |
RS prediction model (high/low) | 4.071 | 2.480–6.681 | 2.19E-09 | 4.137 | 2.397–7.140 | 3.39E-07 |
HR, hazard ratio; CI, confidence interval; SD, standard deviation; RS, risk score.
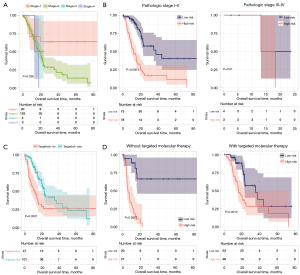
Analysis of decision curves
For the three prognostic factors, we plotted decision curves for the prognostic factors alone, as well as by integrating the three prognostic factors, to compare the effects of different prognostic factors on survival prognosis (Figure 10). The results indicate that the predictive model had the greatest influence on survival.
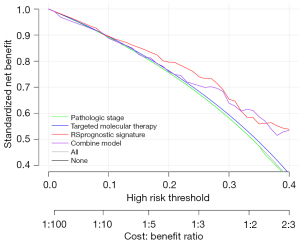
Correlation analysis between prognostic factors and clinical factors
We use the cor function in R3.6.1 software to calculate the correlation coefficient between the expression level of the characteristic factors used to construct the RS model in TCGA dataset and the clinical information corresponding to each sample. The correlation heatmap is shown in Figure S2, and the correlation coefficient and P value are shown in https://cdn.amegroups.cn/static/public/tcr-22-709-12.xlsx.
Transcriptome subtypes of pancreatic cancer in risk groups
In the TCGA training set and the GSE62425 validation dataset, we all performed clustering based on 8 characteristic factors, and obtained different subtypes, subtype 1 and 2 (Figure S3A,S3B), It can be seen from the figure that the expression level distribution of the 8 genes is relatively consistent. Then, the correlation between different subtypes and survival prognosis was investigated in subtypes 1 and 2 obtained in the TCGA training set and GSE62425 validation data set, respectively (Figure S3C,S3D). The results show that the results in the TCGA training set and the GSE62425 validation data set are consistent, subtype1 has a better prognosis, and then a heat map is displayed based on the information of the samples in the subtype, as shown in Figure S3E,S3F. For the data information of this part, please see the https://cdn.amegroups.cn/static/public/tcr-22-709-13.xlsx and https://cdn.amegroups.cn/static/public/tcr-22-709-14.xlsx.
Tumor purity by risk group
The relationship between tumor purity and risk grouping is shown in Figure S4. The tumor purity in the high-risk group was higher than that in the low-risk group in both TCGA (P=0.005852) and GSE62452 (P=0.000249) dataset. For the data results, please refer to the https://cdn.amegroups.cn/static/public/tcr-22-709-15.xlsx and https://cdn.amegroups.cn/static/public/tcr-22-709-16.xlsx.
Discussion
In the past decade, precision medicine has profoundly changed the prospect of some malignant tumor treatments, leading to a gradual increase in the global cancer survival rate. However, pancreatic cancer is a highly malignant tumor of the digestive system, and the rate of early diagnosis remains low. Moreover, the histological characteristics of pancreatic cancer, i.e., poor blood supply and rich fibrous connective tissue, hinder its systemic treatment; thus, it is particularly important to identify biomarkers for the early identification of pancreatic cancer. It is also necessary to improve the understanding of the genetic and molecular background of pancreatic cancer and identify new therapeutic targets. Studies aimed at identifying these biomarkers have increased in recent years.
In this study, we screened differentially expressed lncRNAs, miRNAs, and mRNAs between pancreatic cancer tissues and adjacent normal tissues. Key mRNAs were identified as candidate genes to construct a prediction model using a ceRNA interaction network. Univariate and multivariate analyses of the candidate genes were performed to construct a model for predicting the prognosis of patients with pancreatic cancer. The model’s predictive ability was verified using an external dataset, which revealed its high accuracy and stability.
Our prognostic model was generated based on eight genes: ANLN, FHDC1, LY6D, SMAD6, ACKR4, RAB27B, AUNIP, and GPRIN3. ANLN encodes an actin-binding protein that plays an important role in cell growth and migration. Wang et al. showed that ANLN was significantly regulated in pancreatic cancer tissues and cell lines, and found that high expression of ANLN was associated with some clinicopathological features, including tumor growth, metastasis, and poor prognosis (30). FHDC1 is a key gene in malignant transformation induced by microcystin-LR (L: lysine, R: arginine, MC-LR) in the human hepatocyte L02 cell line (31). Moreover, FHDC1 methylation can be used as a prognostic factor for the survival and recurrence of lung adenocarcinoma (32). Previous studies showed that LY6D is upregulated in pancreatic cancer tissues and is a likely molecular target for predicting the survival outcomes of patients with pancreatic cancer (33,34). LY6D is also associated with other tumors, such as prostate cancer (35), non-small cell lung cancer (36), and breast carcinoma (37). SMAD6 is overexpressed in surgically resected samples of pancreatic ductal adenocarcinoma (38,39). SMAD6 can override TGF-β-induced growth inhibition in vitro (40). Furthermore, overexpression of SMAD6 aggravates cerulein-induced chronic pancreatic fibrosis, which progresses to pancreatic cancer (41,42). ACKR4, a receptor for C-C type chemokines, can predict lymph node metastasis and prognosis in patients with various cancers such as breast cancer (43), colorectal cancer (44), gastric cancer (45), and cervical squamous cell cancer (46). RAB27B and RAB27A, members of the Rab family of small GTPases, play important roles in cell invasion, proliferation, and apoptosis, as well as in chemotherapy resistance in pancreatic cancer (47). Furthermore, Zhao et al. found that increased expression of RAB27B was significantly associated with perineural and vascular invasion in pancreatic cancer, as well as in distant metastasis. Some patients with pancreatic cancer showing high RAB27B expression exhibit significantly poorer overall survival (48). AUNIP plays a key role in the cell cycle and DNA damage repair which is suggested to be a candidate diagnostic and prognostic biomarker for various malignant tumors, such as oral squamous cell carcinoma (49), hepatocellular carcinoma (50), lung adenocarcinoma (50), and estrogen receptor-positive breast cancer (51). Zhou et al. reported that GPRIN3 can be used as a novel target for gastric cancer treatment, as it represses the Wnt/β-catenin signaling pathway for gastric cancer treatment (52).
In daily clinical practice, the TNM staging system is one of the most important indicators for predicting prognosis in patients with cancer (53) but is not always accurate (54) and may predict quite different outcomes for patients with the same clinical tumor stage (55). Therefore, there is an urgent need to identify new prognostic indicators to supplement the TNM staging system. With continuous advances in medical technology, the mechanisms of tumorigenesis and its progression are becoming increasingly clear. Therefore, genes that are closely related to tumor development can be used as molecular targets for the treatment and evaluation of patient prognosis via the widespread application of public tumor databases. For example, Jiang et al. used 14 ferroptosis-associated genes to construct a prognostic signature for patients with pancreatic cancer and confirmed its usefulness for predicting survival and guiding personalized immunotherapy (56). Similarly, Abou Khouzam et al. constructed an eight-gene hypoxia signature associated with overall survival in patients with pancreatic cancer and verified its applicability for characterizing the pancreatic tumor microenvironment and potentially guiding hypoxia-mediated therapeutic strategies (57).
Post-transcriptional regulation of genes is not a simple interaction between miRNA-mRNA silencing mechanisms but rather a complex regulatory network. Many lncRNA molecules are rich in miRNA-binding sites, which act as miRNA sponges in cells, thereby releasing the regulation of miRNA on their target genes and changing the expression levels of target genes. This mechanism of action is known as the ceRNA mechanism. Prognostic models of many malignant tumors have been established using bioinformatic techniques. For example, Zhang et al. revealed the potential regulatory axes and prognostic biomarkers of hepatocellular carcinoma by constructing a disease-specific lncRNA-miRNA-mRNA regulatory network (18). Studies based on the ceRNA hypothesis have revealed the functions of some lncRNAs in cancer. Wang et al. indicated that lncRNA FAL1 acts as a ceRNA and promotes the proliferation, migration, and invasion of colorectal cancer cells via regulation of the miR-637/NUPR1 pathway (58). Additionally, LINC00657 plays an oncogenic role in esophageal squamous cell carcinoma by targeting miR-615-3p and JunB (59). Moreover, lncRNA TP73-AS1 promotes non-small cell lung cancer progression by competitively sponging miR-449a/EZH2 (60).
Few studies have been performed to construct a prediction model based on the ceRNA network in patients with pancreatic cancer and verify its accuracy and stability using the Gene Expression Omnibus dataset. The developed eight-gene prediction model can effectively and independently predict the survival prognosis of patients with pancreatic cancer, enabling clinicians to make better treatment decisions. Moreover, the eight genes identified in this study are important potential diagnostic and therapeutic targets. However, the present study had some limitations. As the data used in this study are retrospective, further prospective and large-scale studies are needed to verify the accuracy of our predictive model. Additionally, our findings are based on bioinformatic analysis; therefore, some conclusions and results should be validated in experimental studies.
Acknowledgments
Funding: This work was supported by
Footnote
Reporting Checklist: The authors have completed the TRIPOD reporting checklist. Available at https://tcr.amegroups.com/article/view/10.21037/tcr-22-709/rc
Peer Review File: Available at https://tcr.amegroups.com/article/view/10.21037/tcr-22-709/prf
Conflicts of Interest: All authors have completed the ICMJE uniform disclosure form (available at https://tcr.amegroups.com/article/view/10.21037/tcr-22-709/coif). The authors have no conflicts of interest to declare.
Ethical Statement: The authors are accountable for all aspects of the work in ensuring that questions related to the accuracy or integrity of any part of the work are appropriately investigated and resolved. The study was conducted in accordance with the Declaration of Helsinki (as revised in 2013).
Open Access Statement: This is an Open Access article distributed in accordance with the Creative Commons Attribution-NonCommercial-NoDerivs 4.0 International License (CC BY-NC-ND 4.0), which permits the non-commercial replication and distribution of the article with the strict proviso that no changes or edits are made and the original work is properly cited (including links to both the formal publication through the relevant DOI and the license). See: https://creativecommons.org/licenses/by-nc-nd/4.0/.
References
- Siegel RL, Miller KD, Fuchs HE, et al. Cancer Statistics, 2021. CA Cancer J Clin 2021;71:7-33. [Crossref] [PubMed]
- Rahib L, Smith BD, Aizenberg R, et al. Projecting cancer incidence and deaths to 2030: the unexpected burden of thyroid, liver, and pancreas cancers in the United States. Cancer Res 2014;74:2913-21. [Crossref] [PubMed]
- Wong MCS, Jiang JY, Liang M, et al. Global temporal patterns of pancreatic cancer and association with socioeconomic development. Sci Rep 2017;7:3165. [Crossref] [PubMed]
- Rawla P, Sunkara T, Gaduputi V. Epidemiology of Pancreatic Cancer: Global Trends, Etiology and Risk Factors. World J Oncol 2019;10:10-27. [Crossref] [PubMed]
- Collisson EA, Bailey P, Chang DK, et al. Molecular subtypes of pancreatic cancer. Nat Rev Gastroenterol Hepatol 2019;16:207-20. [Crossref] [PubMed]
- Sinkala M, Mulder N, Martin DP. Integrative landscape of dysregulated signaling pathways of clinically distinct pancreatic cancer subtypes. Oncotarget 2018;9:29123-39. [Crossref] [PubMed]
- Moffitt RA, Marayati R, Flate EL, et al. Virtual microdissection identifies distinct tumor- and stroma-specific subtypes of pancreatic ductal adenocarcinoma. Nat Genet 2015;47:1168-78. [Crossref] [PubMed]
- Bailey P, Chang DK, Nones K, et al. Genomic analyses identify molecular subtypes of pancreatic cancer. Nature 2016;531:47-52. [Crossref] [PubMed]
- Collisson EA, Sadanandam A, Olson P, et al. Subtypes of pancreatic ductal adenocarcinoma and their differing responses to therapy. Nat Med 2011;17:500-3. [Crossref] [PubMed]
- Tay Y, Rinn J, Pandolfi PP. The multilayered complexity of ceRNA crosstalk and competition. Nature 2014;505:344-52. [Crossref] [PubMed]
- Ko CC, Hsieh YY, Yang PM. Long Non-Coding RNA MIR31HG Promotes the Transforming Growth Factor β-Induced Epithelial-Mesenchymal Transition in Pancreatic Ductal Adenocarcinoma Cells. Int J Mol Sci 2022;23:6559. [Crossref] [PubMed]
- Corradi C, Gentiluomo M, Gajdán L, et al. Genome-wide scan of long noncoding RNA single nucleotide polymorphisms and pancreatic cancer susceptibility. Int J Cancer 2021;148:2779-88. [Crossref] [PubMed]
- Salmena L, Poliseno L, Tay Y, et al. A ceRNA hypothesis: the Rosetta Stone of a hidden RNA language? Cell 2011;146:353-8. [Crossref] [PubMed]
- Rosano S, Parab S, Noghero A, et al. Long Non-Coding RNA LINC02802 Regulates In Vitro Sprouting Angiogenesis by Sponging microRNA-486-5p. Int J Mol Sci 2022;23:1653. [Crossref] [PubMed]
- Li X, Li Z, Liu P, et al. Novel CircRNAs in Hub ceRNA Axis Regulate Gastric Cancer Prognosis and Microenvironment. Front Med (Lausanne) 2021;8:771206. [Crossref] [PubMed]
- Dong Q, Long X, Cheng J, et al. LncRNA GAS5 suppresses ovarian cancer progression by targeting the miR-96-5p/PTEN axis. Ann Transl Med 2021;9:1770. [Crossref] [PubMed]
- Zhang T, Wang Y, Dong Y, et al. Identification of Novel Diagnostic Biomarkers in Prostate Adenocarcinoma Based on the Stromal-Immune Score and Analysis of the WGCNA and ceRNA Network. Dis Markers 2022;2022:1909196. [Crossref] [PubMed]
- Zhang Q, Sun L, Zhang Q, et al. Construction of a disease-specific lncRNA-miRNA-mRNA regulatory network reveals potential regulatory axes and prognostic biomarkers for hepatocellular carcinoma. Cancer Med 2020;9:9219-35. [Crossref] [PubMed]
- Paci P, Colombo T, Farina L. Computational analysis identifies a sponge interaction network between long non-coding RNAs and messenger RNAs in human breast cancer. BMC Syst Biol 2014;8:83. [Crossref] [PubMed]
- Barrett T, Suzek TO, Troup DB, et al. NCBI GEO: mining millions of expression profiles--database and tools. Nucleic Acids Res 2005;33:D562-6. [Crossref] [PubMed]
- Yang S, He P, Wang J, et al. A Novel MIF Signaling Pathway Drives the Malignant Character of Pancreatic Cancer by Targeting NR3C2. Cancer Res 2016;76:3838-50. [Crossref] [PubMed]
- Ritchie ME, Phipson B, Wu D, et al. limma powers differential expression analyses for RNA-sequencing and microarray studies. Nucleic Acids Res 2015;43:e47. [Crossref] [PubMed]
- Wang L, Cao C, Ma Q, et al. RNA-seq analyses of multiple meristems of soybean: novel and alternative transcripts, evolutionary and functional implications. BMC Plant Biol 2014;14:169. [Crossref] [PubMed]
- Paraskevopoulou MD, Vlachos IS, Karagkouni D, et al. DIANA-LncBase v2: indexing microRNA targets on non-coding transcripts. Nucleic Acids Res 2016;44:D231-8. [Crossref] [PubMed]
- Li JH, Liu S, Zhou H, et al. starBase v2.0: decoding miRNA-ceRNA, miRNA-ncRNA and protein-RNA interaction networks from large-scale CLIP-Seq data. Nucleic Acids Res 2014;42:D92-7. [Crossref] [PubMed]
- Shannon P, Markiel A, Ozier O, et al. Cytoscape: a software environment for integrated models of biomolecular interaction networks. Genome Res 2003;13:2498-504. [Crossref] [PubMed]
- Huang da W. Sherman BT, Lempicki RA. Systematic and integrative analysis of large gene lists using DAVID bioinformatics resources. Nat Protoc 2009;4:44-57. [Crossref] [PubMed]
- Huang da W. Sherman BT, Lempicki RA. Bioinformatics enrichment tools: paths toward the comprehensive functional analysis of large gene lists. Nucleic Acids Res 2009;37:1-13. [Crossref] [PubMed]
- Goeman JJ. L1 penalized estimation in the Cox proportional hazards model. Biom J 2010;52:70-84. [PubMed]
- Wang A, Dai H, Gong Y, et al. ANLN-induced EZH2 upregulation promotes pancreatic cancer progression by mediating miR-218-5p/LASP1 signaling axis. J Exp Clin Cancer Res 2019;38:347. [Crossref] [PubMed]
- Chen HQ, Zhao J, Li Y, et al. Gene expression network regulated by DNA methylation and microRNA during microcystin-leucine arginine induced malignant transformation in human hepatocyte L02 cells. Toxicol Lett 2018;289:42-53. [Crossref] [PubMed]
- Wang R, Zhu H, Yang M, et al. DNA methylation profiling analysis identifies a DNA methylation signature for predicting prognosis and recurrence of lung adenocarcinoma. Oncol Lett 2019;18:5831-42. [Crossref] [PubMed]
- Li Z, Hu C, Yang Z, et al. Bioinformatic Analysis of Prognostic and Immune-Related Genes in Pancreatic Cancer. Comput Math Methods Med 2021;2021:5549298. [Crossref] [PubMed]
- Xu D, Wang Y, Zhang Y, et al. Systematic Analysis of an Invasion-Related 3-Gene Signature and Its Validation as a Prognostic Model for Pancreatic Cancer. Front Oncol 2021;11:759586. [Crossref] [PubMed]
- Barros-Silva JD, Linn DE, Steiner I, et al. Single-Cell Analysis Identifies LY6D as a Marker Linking Castration-Resistant Prostate Luminal Cells to Prostate Progenitors and Cancer. Cell Rep 2018;25:3504-3518.e6. [Crossref] [PubMed]
- Lu Y, Lemon W, Liu PY, et al. A gene expression signature predicts survival of patients with stage I non-small cell lung cancer. PLoS Med 2006;3:e467. [Crossref] [PubMed]
- Mayama A, Takagi K, Suzuki H, et al. OLFM4, LY6D and S100A7 as potent markers for distant metastasis in estrogen receptor-positive breast carcinoma. Cancer Sci 2018;109:3350-9. [Crossref] [PubMed]
- Singh P, Srinivasan R, Wig JD, et al. A study of Smad4, Smad6 and Smad7 in Surgically Resected Samples of Pancreatic Ductal Adenocarcinoma and Their Correlation with Clinicopathological Parameters and Patient Survival. BMC Res Notes 2011;4:560. [Crossref] [PubMed]
- Singh P, Wig JD, Srinivasan R, et al. A comprehensive examination of Smad4, Smad6 and Smad7 mRNA expression in pancreatic ductal adenocarcinoma. Indian J Cancer 2011;48:170-4. [Crossref] [PubMed]
- Kleeff J, Maruyama H, Friess H, et al. Smad6 suppresses TGF-beta-induced growth inhibition in COLO-357 pancreatic cancer cells and is overexpressed in pancreatic cancer. Biochem Biophys Res Commun 1999;255:268-73. [Crossref] [PubMed]
- Miyamoto T, Nakamura H, Nagashio Y, et al. Overexpression of Smad6 exacerbates pancreatic fibrosis in murine caerulein-induced chronic pancreatic injuries. Pancreas 2010;39:385-91. [Crossref] [PubMed]
- Lin H, Dong B, Qi L, et al. Inhibitory Smads suppress pancreatic stellate cell activation through negative feedback in chronic pancreatitis. Ann Transl Med 2021;9:384. [Crossref] [PubMed]
- Mohammed MM, Shaker O, Ramzy MM, et al. The relation between ACKR4 and CCR7 genes expression and breast cancer metastasis. Life Sci 2021;279:119691. [Crossref] [PubMed]
- Zhu Y, Tang W, Liu Y, et al. CCX-CKR expression in colorectal cancer and patient survival. Int J Biol Markers 2014;29:e40-8. [Crossref] [PubMed]
- Zhu Z, Sun Z, Wang Z, et al. Prognostic impact of atypical chemokine receptor expression in patients with gastric cancer. J Surg Res 2013;183:177-83. [Crossref] [PubMed]
- Hou T, Liang D, Xu L, et al. Atypical chemokine receptors predict lymph node metastasis and prognosis in patients with cervical squamous cell cancer. Gynecol Oncol 2013;130:181-7. [Crossref] [PubMed]
- Li J, Jin Q, Huang F, et al. Effects of Rab27A and Rab27B on Invasion, Proliferation, Apoptosis, and Chemoresistance in Human Pancreatic Cancer Cells. Pancreas 2017;46:1173-9. [Crossref] [PubMed]
- Zhao H, Wang Q, Wang X, et al. Correlation Between RAB27B and p53 Expression and Overall Survival in Pancreatic Cancer. Pancreas 2016;45:204-10. [Crossref] [PubMed]
- Yang Z, Liang X, Fu Y, et al. Identification of AUNIP as a candidate diagnostic and prognostic biomarker for oral squamous cell carcinoma. EBioMedicine 2019;47:44-57. [Crossref] [PubMed]
- Ma C, Kang W, Yu L, et al. AUNIP Expression Is Correlated With Immune Infiltration and Is a Candidate Diagnostic and Prognostic Biomarker for Hepatocellular Carcinoma and Lung Adenocarcinoma. Front Oncol 2020;10:590006. [Crossref] [PubMed]
- Oh JH, Lee JY, Kim KH, et al. Elevated GCN5 expression confers tamoxifen resistance by upregulating AIB1 expression in ER-positive breast cancer. Cancer Lett 2020;495:145-55. [Crossref] [PubMed]
- Zhou W, Ding X, Jin P, et al. miR-6838-5p Affects Cell Growth, Migration, and Invasion by Targeting GPRIN3 via the Wnt/β-Catenin Signaling Pathway in Gastric Cancer. Pathobiology 2020;87:327-37. [Crossref] [PubMed]
- Gao G, Yu Z, Zhao X, et al. Immune classification and identification of prognostic genes for uveal melanoma based on six immune cell signatures. Sci Rep 2021;11:22244. [Crossref] [PubMed]
- Luo P, Yang X, Huang S, et al. Syntenin overexpression in human lung cancer tissue and serum is associated with poor prognosis. BMC Cancer 2020;20:159. [Crossref] [PubMed]
- Chang SL, Lee SW, Yang SF, et al. Expression and prognostic utility of SSX2IP in patients with nasopharyngeal carcinoma. APMIS 2020;128:287-97. [Crossref] [PubMed]
- Jiang P, Yang F, Zou C, et al. The construction and analysis of a ferroptosis-related gene prognostic signature for pancreatic cancer. Aging (Albany NY) 2021;13:10396-414. [Crossref] [PubMed]
- Abou Khouzam R, Rao SP, Venkatesh GH, et al. An Eight-Gene Hypoxia Signature Predicts Survival in Pancreatic Cancer and Is Associated With an Immunosuppressed Tumor Microenvironment. Front Immunol 2021;12:680435. [Crossref] [PubMed]
- Wang L, Jiang F, Xia X, et al. LncRNA FAL1 promotes carcinogenesis by regulation of miR-637/NUPR1 pathway in colorectal cancer. Int J Biochem Cell Biol 2019;106:46-56. [Crossref] [PubMed]
- Sun Y, Wang J, Pan S, et al. LINC00657 played oncogenic roles in esophageal squamous cell carcinoma by targeting miR-615-3p and JunB. Biomed Pharmacother 2018;108:316-24. [Crossref] [PubMed]
- Zhang L, Fang F, He X. Long noncoding RNA TP73-AS1 promotes non-small cell lung cancer progression by competitively sponging miR-449a/EZH2. Biomed Pharmacother 2018;104:705-11. [Crossref] [PubMed]