A multidimensional model incorporating radiomics score and liquid biopsy for the prediction of malignant and benign pulmonary nodules
Introduction
Lung cancer is currently one of the most common types of malignancies worldwide. Its overall incidence has declined in recent years due to the reduction in tobacco consumption and improvements in the relevant oncological treatment techniques. However, the mortality rate of lung cancer remains the highest of all cancers (1). Due to delayed diagnoses, most patients with lung cancer have reached advanced stages by diagnosis, resulting in a low 5-year overall survival rate (1,2), whereas patients with stage I lung cancer who undergo surgery have a 10-year survival rate of 92% (3). Therefore, the early screening and treatment of people at high risk for lung cancer can improve patient prognosis and reduce the risk of death from lung cancer (4,5). The benefits demonstrated in the National Lung Screening Trial have led to the widespread implementation of lung cancer screening worldwide (6). Although low-dose computed tomography (LDCT) can identify small pulmonary nodules (PNs) more effectively than can conventional X-rays, accurate distinction between benign and malignant nodules remains a challenge (7).
Pathological biopsy is the gold standard for diagnosing benign and malignant PNs (8). Although this method is accurate and effective, it has many limitations. An invasive approach is required for the acquisition of the standard tissue sample. The procedure is risky and painful for the patient and may cause complications such as spreading of the tumor and pneumothorax during the puncture process. Therefore, the development of a simple, reliable, and economical method to determine the nature of PNs has become an urgent clinical demand.
Radiomics has recently become the method of choice for analyzing medical images (9). As a quantitative analysis tool, radiomic features can extract and analyze image features from radiological images to diagnose disease more accurately and objectively and to predict treatment efficacy (10,11). Lambin et al. first introduced the concept of radiomic features in 2012, and since Aerts et al. first applied it to lung cancer in 2014, radiomic features have been used to predict pathological response to tumor treatment, lymph node metastasis, and disease diagnosis (8,12-14). Peikert et al. used a least absolute shrinkage and selection operator (LASSO) Cox regression model to screen 7 quantitative radiomic features, achieving a specificity of 85.6% and a sensitivity of 90.0% in differentiating benign from malignant PNs (15). Chen et al. retrospectively analyzed 75 PNs from 72 patients. They found that 76 features were significantly different between benign and malignant lesions and selected the 4 best radiomic features with the highest accuracy, achieving a sensitivity of 92.85% and a specificity of 72.73% (16). Overall then, it appears these models boast good sensitivity but low specificity. Hawkins et al. used a radiomics model to determine the malignancy risk of nodules at baseline and at 1- and 2-year follow ups, with areas under the receiver operating characteristic (ROC) curve (AUCs) of 0.83 and 0.75, respectively (17). A study showed that AUCs of 0.944 and 0.873, respectively, can be achieved using deep learning with extensive sample data to classify benign and malignant PNs during the first 2 years following diagnosis (18). Both of the abovementioned studies were based on National Lung Screening Trial data, in which the proportion of benign nodules is 96.4%, with only some nodules being pathologically confirmed; consequently, the model cannot be applied directly to the follow-up population of those with PNs and high malignancy rates (19).
In addition, liquid biopsy techniques have advanced rapidly in the last decade and have been applied to diagnosis and treatment in the clinical oncology setting (20,21). Previous findings have shown that a method based on the 4-color fluorescence in-situ hybridization (FISH) technique to identify circulating genetically abnormal cells (CACs) can be used to diagnose benign and malignant PNs (22,23). The diagnostic value of this method has been validated in a Chinese population (24), where an AUC of 0.823 was achieved for the diagnosis of PNs. In recent years, various blood markers have been developed for tumor liquid biopsy, and blood-based biomarkers can assist with tumor diagnosis. A multicenter clinical study included 192 patients with an operable lung mass, of whom 64% had stage I lung cancer, with 65 lung cancer-related gene coding regions being sequenced. The study found that the specificity of plasma circulating tumor DNA (ctDNA) detection was 96% (25). In 2017, Ooki et al. established a new cancer-specific methylation genome (a total of 6 investigated genes) to diagnose early-stage non-small cell lung cancer (NSCLC). The sensitivity of this methylation genome was 72.0%, with a specificity of 71.0% (26). Biomarkers have shown a reasonable specificity in the diagnosis of PNs, but their sensitivity has been disappointing. Nevertheless, due to several constraints, the collection and analysis process of novel biomarkers lacks standardization.
Due to the complex tumor microenvironment during tumor development, liquid biopsy or imaging histology alone may not be sufficient to accurately distinguish benign from malignant PNs. This study thus aimed to develop a model combining radiomics score (Rad-score) and liquid biopsy as a clinically valuable tool for differentiating benign and malignant PNs. We hypothesized that this model has the potential to improve the efficiency of decision-making, increase the prognostic accuracy for patients with lung cancer, and serve as an auxiliary clinical diagnostic tool. We present the following article in accordance with the TRIPOD reporting checklist (available at https://tcr.amegroups.com/article/view/10.21037/tcr-22-1755/rc).
Methods
Study design and participants
The study was conducted in accordance with the Declaration of Helsinki (as revised in 2013). The study was approved by the Institutional Ethics Committee for Clinical Research of Zhongda Hospital, affiliated to Southeast University (No. 2019ZDSYLL176-Y01), and each participant provided written informed consent prior to the research. This prospective study involved patients with PNs. The inclusion criteria were as follows: (I) single or multiple PNs detected using computed tomography (CT) or LDCT within the past month, (II) age ≥18 years, and (III) scheduled to undergo nonsurgical biopsy or surgery. The exclusion criteria were as follows: (I) women who were breastfeeding or pregnant and (II) a history of malignant tumors in the last 5 years.
Between January 2020 and December 2021, 180 patients diagnosed with PNs confirmed by an LDCT scan were recruited at Zhongda Hospital. All participants completed a demographic survey to obtain clinical information. In all, 156 patients with PNs who met the inclusion criteria completed the plasma sample collection and received a definite pathological diagnosis. All patients completed testing for traditional tumor markers, including carcinoembryonic antigen (CEA), CYFRA21-1, neuron-specific enolase (NSE), progastrin-releasing protein (ProGRP), squamous cell carcinoma antigen (SCC), and CAC detection. Participants underwent fine needle biopsy, fiberoptic bronchoscopy, or surgical resection followed by pathological examination. A flowchart of patient recruitment and processing is presented in Figure 1.
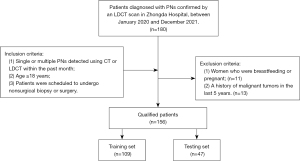
CAC detection
We selected a 4-color FISH assay which was developed at the University of Texas MD Anderson Cancer Center (23). This technology uses a 4-color FISH probe set to detect the chromosome abnormality of surfactant protein A at 10q22.3 and 3p22.1, which have been implicated in the early pathogenesis of lung cancer and are related to the internal control genes CEP10 and 3q29 (22). A sample of peripheral blood (10 mL) was collected preoperatively for the CAC assay using the Mononuclear Cell Chromosomal Abnormality Detection Kit (Zhuhai Sanmed Biotechnology Ltd., Zhuhai, China). The assay procedure was performed according to the manufacturer’s instructions (22).
CT image acquisition and segmentation
All patients underwent CT examination. The CT machine used was a Siemens SOMATOM Definition Flash system (Siemens Healthineers, Erlangen, Germany) with the following CT scan parameters: 150 effective mAs, 120 kV, beam collimation 128 mm × 0.6 mm, frame rotation time 0.5 s, pitch 1.2, and a reconstructed image slice thickness 0.625–1.25 mm.
In this study, all regions of interest (ROIs) of PNs were segmented using 3D Slicer version 4.11.20210226 (3D Slicer; National Institutes of Health, Bethesda, MD, USA). A radiologist with 5 years of experience performed the segmentation task twice manually. In all, 103 patients with multiple nodules were included in this study. The larger PNs were segmented. An interclass correlation coefficient (ICC) was used for analyzing intraobserver agreement (ICC >0.80). The image segmentation process is shown in Figure 2.
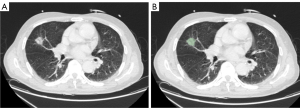
Radiomic feature extraction
Open-source software [FeAture Explorer (FAE) v. 0.0.3; Song et al., Shanghai, China] was used to extract and select radiomic features and then establish a radiomics model. The software automatically matched the original data with the corresponding ROIs and extracted 106 radiomics features. All texture features were calculated for 13 directions and merged using the 3D-average approach. Parameters of params.yaml for radiomics extraction are provided in website: https://cdn.amegroups.cn/static/public/tcr-22-1755-1.yaml.
Model building
At a ratio of 7:3, 156 eligible patients with PNs were randomly divided into a training set (n=109) and an internal test set (n=47). The synthetic minority oversampling technique (SMOTE) was used to remove the imbalance of the training data set. We applied principal component analysis (PCA) to the feature matrix. Before building the model, we used the Kruskal-Wallis test to select features and used logistic regression with LASSO constraint as the classifier. To conclude the model’s hyper-parameter, we applied 5-fold cross-validation on the training data set. Each participant’s Rad-score was calculated using the linear combination of the final selected features and their corresponding weights. The above processes were performed with FAEPro version 0.3.7 (FAE).
Statistical analysis
The SPSS 20.0 software (IBM Corp., Armonk, NY, USA), Python 3.7 (Python Software Foundation, Beaverton, OR, USA), and MedCalc 19.6.4 (MedCalc Software, Ostend, Belgium) were used for statistical analyses. Continuous data (age, diameter of the nodule, and Rad-score) were tested for normality and homogeneity of variance. For categorical data (gender, smoking history, and family history), the differences between the 2 groups were compared using independent samples t-tests. A P value <0.05 indicated statistical significance.
A multivariate logistic regression model was constructed using the established Rad-score and clinical data in the training set. Pathology results were used as the gold standard to generate the ROC curve. The ROC curve and AUC were used to evaluate the model’s performance in the training and test sets. DeLong’s test was applied to compare whether the differences in AUC between models were statistically significant.
Results
Patient characteristics
A total of 156 patients with PNs were included. The clinical characteristics of all enrolled patients are shown in Table 1. There were 59 (54.1%) patients in the training set and 26 (55.3%) in the test set with malignant PNs. Smoking history differed significantly between benign and malignant PNs in the training and test sets (P<0.001); whether the nodule was solid or not was significantly different between malignant and benign PNs (P<0.001), but benign and malignant nodules differed significantly in diameter (P<0.05).
Table 1
Characteristics | Training set (n=109) | Testing set (n=47) | |||||
---|---|---|---|---|---|---|---|
Benign | Malignant | P value | Benign | Malignant | P value | ||
Age (years) | 57.84±12.430 | 57.31±12.282 | 0.822 | 57.14±12.607 | 55.88±11.010 | 0.717 | |
Gender | 0.110 | 0.282 | |||||
Female | 17 (34%) | 29 (49.2%) | 8 (38.1%) | 14 (53.8%) | |||
Male | 33 (66%) | 30 (50.8%) | 13 (61.9%) | 12 (46.2%) | |||
Smoking history | <0.001 | <0.001 | |||||
Nonsmoker* | 44 (88%) | 24 (40.7%) | 19 (90.5%) | 5 (19.2%) | |||
Current or past smoker† | 6 (12%) | 35 (59.3%) | 2 (9.5%) | 21 (80.8%) | |||
Family history | 0.718 | 0.360 | |||||
No | 34 (68%) | 42 (71.2%) | 9 (42.9%) | 19 (73.1%) | |||
Yes | 16 (32%) | 17 (28.8%) | 12 (57.1%) | 7 (26.9%) | |||
Nodule count | 0.056 | 0.112 | |||||
Multiple | 36 (72%) | 32 (54.2%) | 18 (85.7%) | 17 (65.4%) | |||
Single | 14 (28%) | 27 (45.8%) | 3 (14.3%) | 9 (34.6%) | |||
Diameter of the nodule | 10.06±5.783 | 13.15±5.956 | 0.007 | 9.67±5.228 | 12.38±5.906 | 0.106 | |
Type of nodule | <0.001 | <0.001 | |||||
Subsolid | 12 (24%) | 45 (76.3%) | 7 (33.3%) | 20 (76.9%) | |||
Solid | 38 (76%) | 14 (23.7%) | 14 (66.7%) | 6 (23.1%) | |||
Nodule location | 0.718 | 0.016 | |||||
Non-upper lobe | 28 (56%) | 31 (52.5%) | 13 (61.9%) | 7 (26.9%) | |||
Upper lobe | 22 (44%) | 28 (47.5%) | 8 (38.1%) | 19 (73.1%) | |||
Cancer stage | – | – | |||||
IA1 | – | 41 (69.5%) | – | 19 (73.1%) | |||
IA2 | – | 11 (18.6%) | – | 4 (15.4%) | |||
IA3 | – | 7 (11.9%) | – | 3 (11.5%) | |||
Malignant subtypes | – | – | |||||
Adenocarcinoma | – | 57 (96.6%) | – | 26 (100.0%) | |||
Squamous cell carcinoma | – | 2 (3.4%) | – | – |
No. of patients (%) for categorical variable; mean ± standard deviation for continuous variables. *, current smokers were identified as those with 20 pack-years; †, past smokers were identified as those having a quit time of <15 years.
Biomarkers
All patients were tested for established tumor biomarkers and CACs. In both the training and test groups, the detection results of CACs in malignant PNs were significantly different from those in benign PNs (P<0.001). However, none of the established tumor biomarkers were significantly different between malignant and benign PNs (Table 2). When 3 was selected as the cutoff value (22), the sensitivity of CACs in diagnosing benign and malignant PNs was 83.1% while the specificity was 78.0% (Table 3). Table 3 shows that the AUC of CACs in diagnosing benign and malignant PNs was 0.805 [95% confidence interval (CI): 0.718–0.875], with a sensitivity of 83.1%. In the test set, the sensitivity of CACs was 73.1%.
Table 2
Characteristics | Training set (n=109) | Testing set (n=47) | |||||
---|---|---|---|---|---|---|---|
Benign | Malignant | P value | Benign | Malignant | P value | ||
CAC | <0.001 | <0.001 | |||||
Negative | 39 (78%) | 10 (16.9%) | 17 (81.0%) | 7 (26.9%) | |||
Positive | 11 (22%) | 49 (83.1%) | 4 (19.0%) | 19 (73.1%) | |||
CEA (ng/mL) | 0.906 | 0.877 | |||||
Negative | 49 (98%) | 58 (98.3%) | 20 (95.2%) | 25 (96.2%) | |||
Positive | 1 (2%) | 1 (1.7%) | 1 (4.8%) | 1 (3.8%) | |||
SCC (μg/L) | – | – | |||||
Negative | 50 (100%) | 59 (100%) | 21 (100%) | 26 (100%) | |||
Positive | 0 (0%) | 0 (0%) | 0 (0%) | 0 (0%) | |||
NSE (ng/mL) | 0.291 | 0.108 | |||||
Negative | 47 (94%) | 52 (88.1%) | 21 (100%) | 23 (88.5%) | |||
Positive | 3 (6%) | 7 (11.9%) | 0 (0%) | 3 (11.5%) | |||
Pro-GRP (pg/mL) | 0.189 | 0.364 | |||||
Negative | 50 (100%) | 57 (96.6%) | 21 (100%) | 25 (96.2%) | |||
Positive | 0 (0%) | 2 (3.4%) | 0 (0%) | 1 (3.8%) | |||
CYFRA21-1 (ng/mL) | 0.235 | 0.683 | |||||
Negative | 49 (98%) | 55 (93.2%) | 20 (95.2%) | 24 (92.3%) | |||
Positive | 1 (2%) | 4 (6.8%) | 1 (4.8%) | 2 (7.7%) |
Data are presented as n (%). CAC, circulating genetically abnormal cell; CEA, carcinoembryonic antigen; SCC, squamous cell carcinoma antigen; NSE, neuron-specific enolase; ProGRP, progastrin-releasing protein.
Table 3
Predictors | Training set (n=109) | Testing set (n=47) | |||||
---|---|---|---|---|---|---|---|
Sensitivity | Specificity | AUC (95% CI) | Sensitivity | Specificity | AUC (95% CI) | ||
Radiomics model | 0.831 | 0.800 | 0.844 (0.766–0.915) | 0.885 | 0.619 | 0.769 (0.611–0.901) | |
CAC | 0.831 | 0.780 | 0.805 (0.718–0.875) | 0.731 | 0.810 | 0.770 (0.624–0.880) | |
Model (CAC + type of nodule + smoking history + Rad-score) | 0.864 | 0.920 | 0.943 (0.881–0.978) | 0.846 | 0.952 | 0.960 (0.858–0.995) |
AUC, area under the ROC curve; CI, confidence interval; CAC, circulating genetically abnormal cell; Rad-score, radiomics score.
Radiomics model
In all, 105 radiomic features were extracted from the CT images of each patient with PNs. These radiomic features are described in website: https://cdn.amegroups.cn/static/public/tcr-22-1755-2.xlsx. The model based on 5 features achieved the highest AUC in the validation data set. The AUC was 0.804 (95% CI: 0.708–0.886). The AUC of the radiomics model was 0.844 (95% CI: 0.766–0.915) in the training set. This model had a sensitivity of 83.1% and a specificity of 80.0%. The ROC curves and the selected features are shown in Figure 3.
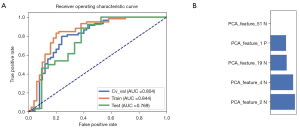
Building a multidimensional model
The Rad-score of each PN was calculated using the linear combination of radiomics features and their corresponding weights (available online: https://cdn.amegroups.cn/static/public/tcr-22-1755-3.xlsx). We selected the clinical characteristics with P<0.1 (smoking history, nodule count, diameter of the nodule, and type of nodule) and combined them with CACs and Rad-score to construct a multidimensional model using logistic regression analysis. The final multidimensional model incorporated 4 variables (P<0.05), the AUC of the multidimensional model was 0.943 (95% CI: 0.881–0.978), the sensitivity was 86.4%, and the specificity was 92.0% (Table 3 and Figure 4). Nonetheless, we tested the significance of AUCs with the DeLong’s test. The difference between CACs and the multidimensional model was significant (P<0.05). The model achieved stable performance in 5-fold cross-validation (Figure 5A). The calibration plot showed good agreement between prediction and observation (Figure 5B).
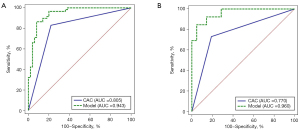
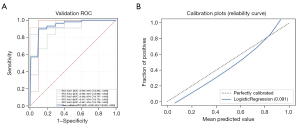
Discussion
This single-center prospective study constructed logistic regression models and tested their predictive power. A new model was established that combines clinical features, CACs, and Rad-score, and it achieved good diagnostic results in distinguishing benign and malignant PNs.
The diagnosis and treatment of PNs are not standardized, and a unified consensus has not yet been reached. Excessive surgical treatment of many PNs is not uncommon. Some authors have asserted that an ideal model for diagnosis and prediction should be comprehensive and include histology, serum markers, medical history (27), and other features in addition to radiomic features. However, it remains to be determined which features should be included in the feature selection of comprehensive models and whether these features improve diagnostic performance. It is also controversial whether a model combining imaging factors, radiomics, or other features is better than a single-factor model (28); the results of existing studies have been inconsistent.
Due to the epidemiological importance of lung cancer in different regions and across various ethnic groups, many different countries are actively conducting research on lung cancer. Despite the great potential of existing methods for the early diagnosis of lung cancer, there is no single method for this purpose that is routinely used for clinical detection. Therefore, clinical validation and application of existing biomarkers are especially important. Research is needed on combining promising biomarkers in different ways and using the most suitable combination for screening.
Compared with traditional CT scan images, radiomics reduces the influence of human error and can thus contribute greatly to distinguishing benign from malignant PNs. This study used LASSO regression to screen 5 stable features from 105 radiological features and to build a model. In the training set, the radiomics model had a sensitivity of 83.1% and a specificity of 80.0% (Table 3).
In this study, CACs were detected in the enrolled population, and the ROC curve was used to evaluate the diagnostic value of CACs. Accordingly, we combined the Rad-score, CACs, and clinical characteristics (type of nodule and smoking history) to construct a multidimensional model. Compared with the sensitivity of CACs, the sensitivity of the model was significantly improved (Table 3), which also verified the high sensitivity of radiomics. Compared with the classic Mayo model (AUC =0.8328) (29), the AUC =0.943 (95% CI: 0.881–0.978) of the multidimensional model was improved (Table 3). Among the clinical parameters, type of nodule and smoking history were identified as independent risk factors according to multivariate logistic regression. Although the incidence of lung cancer among nonsmoking women in China is increasing annually (30), smoking history is still a significant risk factor for lung cancer.
The multidimensional model showed that further combination, exploration, and validation of existing diagnostic methods is a promising research paradigm for diagnosing malignant PNs. However, this study has a few shortcomings: (I) we employed a prospective study design with a small data set. Some studies indicate that models based on small data sets that lack standardized scanning instruments and protocols are prone to overfitting and perform poorly in multicenter validation (27,31). (II) The ROIs of this study were manually delineated by radiologists, and thus specific human errors might have occurred. (III) The sample data came from a single-center medical institution, which might have led to biased results. (IV) The study lacked an external independent test set, and so the diagnostic effect of the model should be furthered verified using larger samples.
Conclusions
In this prospective study, we constructed a multidimensional predictive model for the identification of malignant PNs. The diagnostic performance of the model was better than that of existing biomarkers. The model has the advantages of noninvasiveness, simplicity, and efficiency and can be used as an auxiliary tool for the diagnosis of PNs. The combination and validation of multiple diagnostic methods are promising in the field of the early lung cancer diagnosis.
Acknowledgments
Funding: This work was supported by
Footnote
Reporting Checklist: The authors have completed the TRIPOD reporting checklist. Available at https://tcr.amegroups.com/article/view/10.21037/tcr-22-1755/rc
Data Sharing Statement: Available at https://tcr.amegroups.com/article/view/10.21037/tcr-22-1755/dss
Conflicts of Interest: All authors have completed the ICMJE uniform disclosure form (available at https://tcr.amegroups.com/article/view/10.21037/tcr-22-1755/coif). The authors have no conflicts of interest to declare.
Ethical Statement: The authors are accountable for all aspects of the work in ensuring that questions related to the accuracy or integrity of any part of the work are appropriately investigated and resolved. The study was conducted in accordance with the Declaration of Helsinki (as revised in 2013). The study was approved by the Institutional Ethics Committee for Clinical Research of Zhongda Hospital, affiliated to Southeast University (No. 2019ZDSYLL176-Y01), and each participant provided written informed consent prior to the research.
Open Access Statement: This is an Open Access article distributed in accordance with the Creative Commons Attribution-NonCommercial-NoDerivs 4.0 International License (CC BY-NC-ND 4.0), which permits the non-commercial replication and distribution of the article with the strict proviso that no changes or edits are made and the original work is properly cited (including links to both the formal publication through the relevant DOI and the license). See: https://creativecommons.org/licenses/by-nc-nd/4.0/.
References
- Siegel RL, Miller KD, Fuchs HE, et al. Cancer statistics, 2022. CA Cancer J Clin 2022;72:7-33. [Crossref] [PubMed]
- Alberg AJ, Brock MV, Ford JG, et al. Epidemiology of lung cancer: Diagnosis and management of lung cancer, 3rd ed: American College of Chest Physicians evidence-based clinical practice guidelines. Chest 2013;143:e1S-29S.
- Zheng R, Zeng H, Zhang S, et al. Estimates of cancer incidence and mortality in China, 2013. Chin J Cancer 2017;36:66. [Crossref] [PubMed]
- Wang Z, Wang Y, Huang Y, et al. Challenges and research opportunities for lung cancer screening in China. Cancer Commun (Lond) 2018;38:34. [Crossref] [PubMed]
- Wang Z, Han W, Zhang W, et al. Mortality outcomes of low-dose computed tomography screening for lung cancer in urban China: a decision analysis and implications for practice. Chin J Cancer 2017;36:57. [Crossref] [PubMed]
- Mazzone PJ, Silvestri GA, Souter LH, et al. Screening for Lung Cancer: CHEST Guideline and Expert Panel Report. Chest 2021;160:e427-94. [Crossref] [PubMed]
- Lim KP, Marshall H, Tammemägi M, et al. Protocol and Rationale for the International Lung Screening Trial. Ann Am Thorac Soc 2020;17:503-12. [Crossref] [PubMed]
- Braman N, Prasanna P, Whitney J, et al. Association of Peritumoral Radiomics With Tumor Biology and Pathologic Response to Preoperative Targeted Therapy for HER2 (ERBB2)-Positive Breast Cancer. JAMA Netw Open 2019;2:e192561. [Crossref] [PubMed]
- Litjens G, Kooi T, Bejnordi BE, et al. A survey on deep learning in medical image analysis. Med Image Anal 2017;42:60-88. [Crossref] [PubMed]
- Wu G, Jochems A, Refaee T, et al. Structural and functional radiomics for lung cancer. Eur J Nucl Med Mol Imaging 2021;48:3961-74. [Crossref] [PubMed]
- Park JE, Kim HS. Radiomics as a Quantitative Imaging Biomarker: Practical Considerations and the Current Standpoint in Neuro-oncologic Studies. Nucl Med Mol Imaging 2018;52:99-108. [Crossref] [PubMed]
- Coroller TP, Agrawal V, Huynh E, et al. Radiomic-Based Pathological Response Prediction from Primary Tumors and Lymph Nodes in NSCLC. J Thorac Oncol 2017;12:467-76. [Crossref] [PubMed]
- Wang X, Zhao X, Li Q, et al. Can peritumoral radiomics increase the efficiency of the prediction for lymph node metastasis in clinical stage T1 lung adenocarcinoma on CT? Eur Radiol 2019;29:6049-58. [Crossref] [PubMed]
- Beig N, Khorrami M, Alilou M, et al. Perinodular and Intranodular Radiomic Features on Lung CT Images Distinguish Adenocarcinomas from Granulomas. Radiology 2019;290:783-92. [Crossref] [PubMed]
- Peikert T, Duan F, Rajagopalan S, et al. Novel high-resolution computed tomography-based radiomic classifier for screen-identified pulmonary nodules in the National Lung Screening Trial. PLoS One 2018;13:e0196910. [Crossref] [PubMed]
- Chen CH, Chang CK, Tu CY, et al. Radiomic features analysis in computed tomography images of lung nodule classification. PloS One 2018;13:e0192002. [Crossref] [PubMed]
- Hawkins S, Wang H, Liu Y, et al. Predicting Malignant Nodules from Screening CT Scans. J Thorac Oncol 2016;11:2120-8. [Crossref] [PubMed]
- Ardila D, Kiraly AP, Bharadwaj S, et al. End-to-end lung cancer screening with three-dimensional deep learning on low-dose chest computed tomography. Nat Med 2019;25:954-61. [Crossref] [PubMed]
- Yasaka K, Abe O. Deep learning and artificial intelligence in radiology: Current applications and future directions. PloS Med 2018;15:e1002707. [Crossref] [PubMed]
- Lin D, Shen L, Luo M, et al. Circulating tumor cells: biology and clinical significance. Signal Transduct Target Ther 2021;6:404. [Crossref] [PubMed]
- Ignatiadis M, Sledge GW, Jeffrey SS. Liquid biopsy enters the clinic – implementation issues and future challenges. Nat Rev Clin Oncol 2021;18:297-312. [Crossref] [PubMed]
- Katz RL, Zaidi TM, Pujara D, et al. Identification of circulating tumor cells using 4-color fluorescence in situ hybridization: Validation of a noninvasive aid for ruling out lung cancer in patients with low-dose computed tomography-detected lung nodules. Cancer Cytopathol 2020;128:553-62. [Crossref] [PubMed]
- Katz RL, He W, Khanna A, et al. Genetically abnormal circulating cells in lung cancer patients: an antigen-independent fluorescence in situ hybridization-based case-control study. Clin Cancer Res 2010;16:3976-87. [Crossref] [PubMed]
- Feng M, Ye X, Chen B, et al. Detection of circulating genetically abnormal cells using 4-color fluorescence in situ hybridization for the early detection of lung cancer. J Cancer Res Clin Oncol 2021;147:2397-405. [Crossref] [PubMed]
- Peng M, Xie Y, Li X, et al. Resectable lung lesions malignancy assessment and cancer detection by ultra-deep sequencing of targeted gene mutations in plasma cell-free DNA. J Med Genet 2019;56:647-53. [Crossref] [PubMed]
- Ooki A, Maleki Z, Tsay JJ, et al. A Panel of Novel Detection and Prognostic Methylated DNA Markers in Primary Non-Small Cell Lung Cancer and Serum DNA. Clin Cancer Res 2017;23:7141-52. [Crossref] [PubMed]
- Holzinger A, Haibe-Kains B, Jurisica I. Why imaging data alone is not enough: AI-based integration of imaging, omics, and clinical data. Eur J Nucl Med Mol Imaging 2019;46:2722-30. [Crossref] [PubMed]
- Weng Q, Zhou L, Wang H, et al. A radiomics model for determining the invasiveness of solitary pulmonary nodules that manifest as part-solid nodules. Clin Radiol 2019;74:933-43. [Crossref] [PubMed]
- Kammer MN, Lakhani DA, Balar AB, et al. Integrated Biomarkers for the Management of Indeterminate Pulmonary Nodules. Am J Respir Crit Care Med 2021;204:1306-16. [Crossref] [PubMed]
- Kim HY, Jung KW, Lim KY, et al. Lung Cancer Screening with Low-Dose CT in Female Never Smokers: Retrospective Cohort Study with Long-term National Data Follow-up. Cancer Res Treat 2018;50:748-56. [Crossref] [PubMed]
- Papadimitroulas P, Brocki L, Christopher Chung N, et al. Artificial intelligence: Deep learning in oncological radiomics and challenges of interpretability and data harmonization. Phys Med 2021;83:108-21. [Crossref] [PubMed]