Identification of novel prognostic biomarkers for osteosarcoma: a bioinformatics analysis of differentially expressed genes in the mesenchymal stem cells from single-cell sequencing data set
Introduction
Osteosarcoma (OS) is a type of bone cancer and is a common human malignancy. OS is mainly diagnosed in childhood and adolescence (1,2). Following significant improvements in chemotherapy, the 5-year survival rate of patients with non-metastatic OS is around 70%, but the 5-year survival rate for patients with the metastatic, chemo-resistant or recurrent OS is only about 20% (3). Various factors have been found to be associated with a poor prognosis of OS, but the complicated underlying mechanisms of OS remain elusive (4-6). Thus, novel targets need to be identified that can halt OS metastasis, recurrence, and chemo-resistance and thus improve the prognosis of patients with OS.
The importance of the tumor microenvironment (TME) in OS progression has been noted. The TME comprises a mix of cell types and tissues, including immune cells, extracellular matrixes, endothelial cells, and stromal cells (2,5,7). Among these cell types, mesenchymal stem cells (MSCs) have recently been extensively investigated (2,5,7). Studies have reported that MSCs play a crucial role in OS growth and progression. Du et al. found that CXCR1 (C-X-C motif chemokine receptor 1)/Akt signaling activation induced by MSC-derived interleukin (IL)-8 promotes OS cell anoikis resistance and pulmonary metastasis (8). Research has also shown that bone marrow-derived MSCs promote the invasiveness and transendothelial migration of OS cells via a mesenchymal to amoeboid transition (9). Cortini et al. showed that tumor-activated MSCs promote OS stemness and migratory potential via IL-6 secretion (10). Baglio et al. demonstrated that blocking tumor-educated MSC paracrine activity attenuates OS progression (11). Additionally, exosomal miR-21-5p derived from bone marrow MSCs was shown to promote OS cell proliferation and invasion by targeting PIK3R1(phosphoinositide-3-kinase regulatory subunit 1) (12). Xu et al. showed that MSC-derived exosomes carrying micro ribonucleic acid (miRNA)-150 suppressed the proliferation and migration of OS cells by targeting IGF2BP1(insulin-like growth dactor 2 mRNA binding protein 1) (13). And also, Xu et al. analysed the potential cellular communication networks in advanced osteosarcoma using single-cell RNA sequencing data and found in the cellular communication networks, mesenchymal stem cells and pericytes serve as important signal senders, mediators, and influencers (14). However, the role of MSCs in OS progression remains unclear.
With the aid of single-cell RNA sequencing (scRNA-seq) technology, the role of MSCs in the TME has been effectively deciphered at the transcriptomic and single-cell levels. Researchers have characterized the scRNA-seq-based resident MSCs and have conjectured that inflammatory signals from immune and endothelial cells are the main drivers of hyperoxia-induced changes in MSC transcriptomes (15). Stucky et al. conducted single-cell sequencing of undifferentiated MSCs and discovered a carcinogenic pathway in single-cell MSCs of differentiated-resistance subclones (16). Zhou et al. examined the scRNA-seq landscape of intratumoral heterogeneity and revealed an immunosuppressive microenvironment in advanced OS (17). Recently, Zeng et al. developed a chemo-resistant risk-scoring model for pre-chemotherapy OS by analyzing a scRNA-seq database (18).
In this study, we performed the bioinformatics analysis of a scRNA-seq data set and explored the MSC-specific differentially expressed genes (DEGs) of advanced OS. These DEGs were then subjected to a functional analysis and several key genes were identified and underwent a prognosis analysis. Finally, the key genes with potential prognostic roles were further verified using Gene Expression Omnibus (GEO) data sets. We present the following article in accordance with the STREGA reporting checklist (available at https://tcr.amegroups.com/article/view/10.21037/tcr-22-2370/rc).
Methods
The study was conducted in accordance with the Declaration of Helsinki (as revised in 2013).
ScRNA-seq data processing for GSE152048
A scRNA-seq data set, including expression matrix, cell meta-annotation, and dimensionality reduction coordinate data, was downloaded online. The Seurat R package (version 3.1.5.9900) (https://github.com/satijalab/seurat) was used to identify and interpret sources of heterogeneity from GSE152048, and to integrate diverse types of single-cell data.
DEG analysis of the cell types
DEGs between different cell types were identified by contrasting the gene expression of cells from certain clusters to that of others using the Seurat FindMarkers function with the default.
Functional GO enrichment analysis
The Gene Ontology (GO) enrichment analysis for the DEGs was performed using Apps ClueGO v2.5.9 of Cytoscape v3.7.2 (3) with a network specificity set.
PPI network analysis
The protein-protein interactions (PPIs) between proteins encoded by 130 specific DEGs in the MSCs versus the osteoblastic cells were predicted by STRING (Search Tool for Retrieval of Interacting Genes/Proteins) (version 11.5) and visualized by Cytoscape (v3.7.2).
Survival analysis
A survival analysis was conducted to identify biomarkers from specific genes. The log2(fpkm+1) expression data and clinical information of the OS samples in the TARGET (5) database were downloaded from the University of California Santa Cruz Genome Browser database (https://xenabrowser.net/datapages/?cohort=GDC%20TARGET-OS&removeHub=https%3A%2%2Fxena.treehouse.gi.ucsc.edu%3A443). Next, the samples were divided into 2 groups (i.e., high and low expression groups) based on the median expression level. The results were visualized using Kaplan-Meier plots.
Validation of the expression of the hub genes in GSE42352
The GSE42352 gene expression profile, including 118 samples with the genome-wide gene expression profiling of mesenchymal stem cells, OS cells, and OS cell lines, were downloaded from the GEO database (Platforms: GPL10295: Illumina human-6 v2.0 expression beadchip). All the processed gene expression matrix files were extracted by the R package GEOquery (version 2.54.1). With the expression matrix of the target genes in the samples (12 MSC samples, 84 high-grade OS pre-chemotherapy biopsy samples, and 19 high-grade OS cell samples), the R package ggplot2 (version 3.3.5) was used the compare the results of the different groups. The Wilcoxon test was used as the test method for the comparisons.
Statistical analysis
The statistical analysis was performed using R software. Wilcoxon rank test was used to evaluate the DEGs between different cell clusters. And fisher’s exact test was used to determine the GO function enrichment analysis. P<0.05 was considered statistically significant.
Results
Clustering analysis of scRNA-seq data of primary, metastatic, and recurrent OS tissues
As Figure 1A shows, 11 main clusters were identified based on the unbiased clustering of the cells in the GSE152048 data set. The t-SNE (t-distributed stochastic neighbor embedding) -plot of the different cell types based on the gene profiles and canonical markers in the OS tissues was determined. The proportions of each cell type are shown in Figure 1B. Additionally, Figure 1C shows the t-SNE plot of different cell types coloured according to the individual OS sample. Figure 1D shows the proportions of each cell cluster according to different types of OS, and all the cell types (except the chondroblast cells) were found in primary OS.
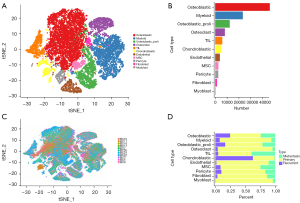
Analysis of MSC transcription characteristics between primary, metastatic and recurrent
In a further analysis, the DEGs between metastasis vs. primary and recurrent vs. primary from MSC were extracted (Figure S1A,S1B). A total of 15 and 72 DEGs were detected between metastasis vs. primary and recurrent vs. primary respectively (Figure S1B). These DEGs were further subjected to functional enrichment. As shown in Figure S1C, the DEGs between metastasis vs. primary were mainly enriched in “regulation of inflammatory response”, “ossification”,” leukocyte migration” and so on. The DEGs between recurrent vs. primary were mainly enriched in “extracellular structure organization”, “extracellular matrix organization”, “epithelial cell proliferation” and so on (Figure S1D).
Analysis of MSC-specific DEGs
The DEGs between the MSCs and other types of cells (except the osteoblastic cells), which are referred to as the “other cells”, were analyzed. As Figure 2A shows, a total of 234 upregulated and 280 downregulated DEGs were identified between the MSCs and other cells. Further, the DEGs between the MSCs and osteoblastic cells were extracted; these DEGs are illustrated in the volcano plot in Figure 2B. A total of 188 upregulated and 158 downregulated DEGs were identified between the MSCs and osteoblastic cells. The common DEGs of the MSCs versus the other cells, and the MSCs versus the osteoblastic cells are illustrated in a Venn diagram, and a total of 216 common DEGs were identified (Figure 2C). The common upregulated DEGs of the MSCs versus the other cells, and the MSCs versus the osteoblastic cells are illustrated in a Venn diagram, and a total of 157 common DEGs are shown (Figure 2D). The common downregulated DEGs of the MSCs versus the other cells, and the MSCs versus the osteoblastic cells are illustrated in a Venn diagram, and a total of 42 common DEGs are shown (Figure 2E).
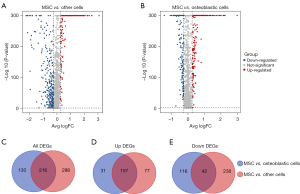
GO functional analysis of DEGs
A GO functional analysis of the DEGs was also performed. As Figure 3A shows, the upregulated common DEGs of the MSCs versus the other cells, and the MSCs versus the osteoblastic cells were enriched in GO terms including “cartilage development”, “regulation of mononuclear cell migration”, “endodermal cell differentiation”, “tumor necrosis factor production” and “negative regulation of angiogenesis”. Additionally, the specific upregulated DEGs between the MSCs and osteoblastic cells were mainly enriched in GO terms such as “response to selenium ion”, “hydrogen peroxide metabolic process”, “collagen catabolic process”, “positive regulation of pathway-restricted SMAD protein phosphorylation” and “glycolipid transport” (Figure 3B). In Figure 3C, the downregulated common DEGs of the MSCs versus the other cells, and the MSCs versus the osteoblastic cells were mainly enriched in GO terms such as “ATP generation from ADP”, “zymogen activation” and “regulation of ATP metabolic process”. Further, the specific downregulated DEGs between the MSCs and osteoblastic cells were mainly enriched in GO terms such as “osteoblast differentiation”, “regulation of release of cytochrome c from mitochondria”, “interleukin-1 production”, “cardiac ventricle morphogenesis”, “collagen biosynthetic process” and “chaperon-mediated protein folding” (Figure 3D).
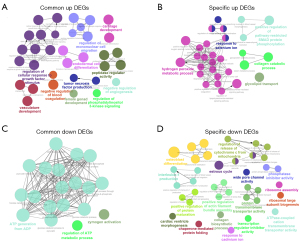
PPI network analysis of specific DEGs between the MSCs and osteoblastic cells
The specific DEGs between the MSCs and osteoblastic cells were subjected to PPI network analysis (Figure 4). Based on the combined score analysis, a total of 20 genes with combined scores >0.94 were selected for the survival analysis of patients with OS. The top 20 genes are shown in (available online: https://cdn.amegroups.cn/static/public/tcr-22-2370-1.xlsx).
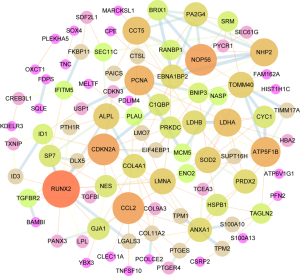
Survival analysis of ANXA1, FDPS, IFITM5, FKBP11, SP7, SQLE and TPM1 in patients with OS
Based on the survival analysis using the TARGET database, 7 genes (i.e., ANXA1, FDPS, IFITM5, FKBP11, SP7, SQLE, and TPM1) were found to be significantly associated with the 5-year overall survival of the OS patients (Figure 5). Among these genes, the low expression of ANXA1 and TPM1 was associated with the shorter overall survival of OS patients (Figure 5), while the high expression of FDPS, IFITM4, FKBP11, SP7 and SQLE was associated with the shorter overall survival of OS patients (Figure 5).
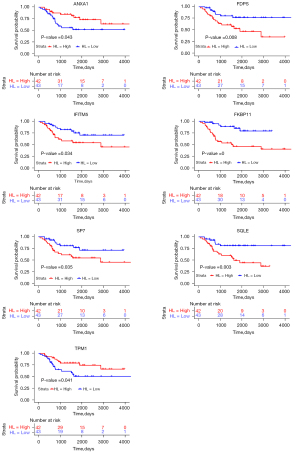
Validation of the expression of ANXA1, FDPS, IFITM5, FKBP11, SP7, SQLE, and TPM1 in GSE42352
In a further analysis, we compared the expression of ANXA1, FDPS, IFITM5, FKBP11, SP7, SQLE, and TPM1 between the MSCs and high-grade OS cells. As Figure 6 shows, ANXA1, FKBP11, SP7, and TPM1 were more upregulated in the MSCs than the high-grade OS cells, while FDPS, IFITM5, and SQLE were more downregulated in the MSCs than the high-grade OS cells (Figure 6).
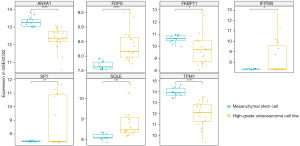
Discussion
OS is a type of bone cancer and is a common human malignancy. OS is mainly diagnosed in children and adolescence (1). Study has reported that MSCs play a key role in OS growth and progression (1). In this study, we performed the bioinformatics analysis of a scRNA-seq data set and explored the MSC-specific DEGs in advanced OS. These DEGs were then subjected to a functional analysis, and several key genes were identified and underwent a prognosis analysis. Based on the analysis of the GSE152048 data set, 234 upregulated and 280 downregulated DEGs were identified between the MSCs and other cells, and a total of 188 upregulated and 158 downregulated DEGs were identified between the MSCs and osteoblastic cells. The GO functional analysis showed that the specific DEGs between the MSCs and osteoblastic cells were enriched in GO terms such as “collagen catabolic process”, “positive regulation of pathway-restricted SMAD protein phosphorylation”, “osteoblast differentiation”, “regulation of release of cytochrome c from mitochondria”, and “interleukin-1 production”. The specific DEGs between the MSCs and osteoblastic cells were subjected to a PPI network analysis. A further survival analysis of 20 genes with combined scores >0.94 revealed that the low expression of ANXA1 and TPM1 was associated with the shorter overall survival of OS patients, while the high expression of FDPS, IFITM4, FKBP11, SP7, and SQLE was associated with the shorter overall survival of OS patients. In a further analysis, we compared the expression of ANXA1, FDPS, IFITM5, FKBP11, SP7, SQLE, and TPM1 between the MSCs and high-grade OS cells. Further validation studies using the GSE42352 data set showed that ANXA1, FKBP11, SP7, and TPM1 were more upregulated in the MSCs than the high-grade OS cells, while FDPS, IFITM5, and SQLE were more downregulated in the MSCs than the high-grade OS cells. In conclusion, our bioinformatics analysis revealed 7 hub genes derived from the specific DEGs between the MSCs and osteoblastic cells.
ANXA1 belongs to the annexin family of calcium-dependent phospholipid-binding proteins and can regulate tumor cell proliferation and apoptosis, and modulate the immune response in tumor progression. Feng et al. showed that ANXA1 binds to and stabilizes EphA2 to enhance nasopharyngeal carcinoma growth and metastasis (19). ANXA1 also enhances the function of Treg cells and reduces the survival rate of patients with breast cancer (20). Tang et al. demonstrated that the suppression of HSPA5 effectively upregulates ANXA1, resulting in OS cell proliferation arrest and apoptosis (21). In this study, we showed that ANXA1 was more downregulated in the MSCs than the osteoblastic cells in the scRNA-seq data set, while MSC was more upregulated in the MSCs than the high-grade OS cells in the GSE42352 data set. Moreover, a high expression of ANXA1 was associated with a better prognosis for patients with OS. Given the contrasting findings about ANXA1 expression in different data sets, further experimental studies need to be conducted, and the prognostic role of ANXA1 needs to be examined further in clinical studies.
FDPS is an enzyme in the mevalonate pathway and catalyzes the production of geranyl pyrophosphate and farnesyl pyrophosphate from isopentenyl pyrophosphate and dimethylallyl pyrophosphate. The dysregulation of FDPS has been shown to be associated with cancer progression. Studies have found that FDPS interacts with PTEN (phosphatase and tensin homolog) loss to promote prostate cancer progression through the modulation of small GTPases/the AKT axis (22). Abate et al. identified FDPS as a new candidate metabolic oncogene in glioblastoma (23). The disruption of FDPS was shown to radiosensitize pancreatic ductal adenocarcinoma by attenuating the deoxyribonucleic acid damage response and immunosuppressive signaling (24). Moreover, Chen et al. revealed that FDPS promotes glioma growth and macrophage recruitment by regulating CCL20 via the Wnt/β-catenin signalling pathway (25). The high expression of FDPS has also been shown to inhibit the apoptosis of human colorectal cancer cells (26). In our analysis, we found that FDPS was more downregulated in the MSCs than the osteoblastic cells or high-grade OS cells. Conversely, the high expression of FDPS was correlated with the shorter overall survival of patients with OS. The role of FPDS in the TME still requires further examination.
IFITM5 encodes bone-restricted IFITM-like protein and involves mineralization. Very few studies have examined the role of IFITM5 in tumor progression. Liu et al. found that the overexpression of IFITM5 promotes tumor cell apoptosis, but inhibits tumor invasion and promotes osteogenic differentiation in human OS cells (27). However, the prognostic role of IFITM5 has not been reported in the clinical studies. In our analysis, we showed that the high expression of IFITM5 was correlated with the shorter overall survival of patients with OS; however, its regulatory mechanism in OS needs to be explored in future studies.
FKBP11 belongs to the superfamily of FK-506 binding protein and is located on chromosome 12q13.12 with 8 exons. Studies have shown that FKBP11 is upregulated in melanoma, hepatocellular carcinoma, and renal carcinoma (28-30). Mechanistic studies have revealed that FKBP11 promotes cell proliferation and tumorigenesis via protein 53–related pathways in oral squamous cell carcinoma (31). In our analysis, we found that the high expression of FKBP11 was associated with the shorter overall survival of patients with OS Collectively, these findings suggest that FKBP11 plays an oncogenic role in OS.
SP is a zinc finger-containing transcription factor and is expressed in the osteoblasts of all endochondral and membranous bones (32). SP7 has been found to be dysregulated in various types of cancer. SP7 has also been shown to promote the migration and angiogenesis of breast cancer by upregulating S100A4 expression (33), and the high expression of SP7 has been shown to be associated with the poor prognosis of patients with breast cancer (33). Studies have found that SP7 downregulates IL-1α expression in mouse OS cells via the transcriptional repression of IL-1α, which in turn may affect the lytic activity of the tumor cells (34). Cao et al. showed that SP7, a transcription factor for osteoblast differentiation, mediates antitumor activity in murine OS (35). In our bioinformatics analysis, we showed that the high expression of SP7 was correlated with the shorter overall survival of patients with OS, which was consistent with previous findings on breast cancer; however, the role of SP7 in the OS TME still requires further examination.
SQLE is a key enzyme responsible for cholesterol synthesis, exerts a complex function in cancer occurrence and development, and commonly serves as a proto-oncogene (36). Sun et al. demonstrated that p53 transcriptionally regulates SQLE to repress cholesterol synthesis and tumor growth (37). Liu et al. showed that SQLE drives non-alcoholic fatty liver disease-induced hepatocellular carcinoma, and SQLE may represent a novel target for this disease (38). High SQLE expression and gene amplification are associated with a poor prognosis in head and neck squamous cell carcinoma (39). SQLE was also shown to mediate metabolic reprogramming to promote metastasis in castration-resistant prostate cancer (40). To date, the role of SQLE has not been examined in OS. In our analysis, we showed that the high expression of SQLE was associated with the shorter overall survival of patients with OS, which suggests that SQLE has an oncogenic role in OS.
TPM1 is a crucial tumor-suppressing gene, which exhibits low expression levels in various types of cancers. Wang et al. showed that TPM1 functions as a tumor suppressor with respect to cell proliferation, angiogenesis, and metastasis in renal cell carcinoma (41). Bharadwaj et al. showed that TPM1 is downregulated by promoter methylation in breast cancer cells (42). In OS, TPM1 could be targeted by miR-107 and has been shown to suppress the proliferation, migration and invasion of OS cells (43). We showed that the high expression of TPM1 was associated with a better prognosis for patients with OS, which suggests that TPM1 acts as a tumor suppressor in OS.
The present study regarding the bioinformatics analysis is subjected to several limitations. Firstly, the present study only focuses on the bioinformatics analysis, while the functional studies including the in vitro and in vivo studies should be conducted to verify the roles of the identified hub genes in osteosarcoma. Secondly, the present study has not performed the WGCNA analysis to reveal the potential genes, which may be investigated in our future studies. Thirdly, we should be cautious when interpreting the findings, as sc-RNA sequencing is still facing challenges such as the depth of sequencing as well as the quality of the clinical samples and isolated single cells.
Conclusions
In conclusion, our bioinformatics analysis revealed 7 hub genes derived from the specific DEGs between the MSCs and osteoblastic cells. The 7 hub genes may serve as potential prognostic biomarkers for patients with OS. The biological role of these hub genes in the MSC-mediated OS TME requires further investigation.
Acknowledgments
Funding: This study was funded by
Footnote
Reporting Checklist: The authors have completed the STREGA reporting checklist. Available at https://tcr.amegroups.com/article/view/10.21037/tcr-22-2370/rc
Conflicts of Interest: All authors have completed the ICMJE uniform disclosure form (available at https://tcr.amegroups.com/article/view/10.21037/tcr-22-2370/coif). The authors have no conflicts of interest to declare.
Ethical Statement: The authors are accountable for all aspects of the work in ensuring that questions related to the accuracy or integrity of any part of the work are appropriately investigated and resolved. The study was conducted in accordance with the Declaration of Helsinki (as revised in 2013).
Open Access Statement: This is an Open Access article distributed in accordance with the Creative Commons Attribution-NonCommercial-NoDerivs 4.0 International License (CC BY-NC-ND 4.0), which permits the non-commercial replication and distribution of the article with the strict proviso that no changes or edits are made and the original work is properly cited (including links to both the formal publication through the relevant DOI and the license). See: https://creativecommons.org/licenses/by-nc-nd/4.0/.
References
- Eaton BR, Schwarz R, Vatner R, et al. Osteosarcoma. Pediatr Blood Cancer 2021;68:e28352. [Crossref] [PubMed]
- Kansara M, Teng MW, Smyth MJ, et al. Translational biology of osteosarcoma. Nat Rev Cancer 2014;14:722-35. [Crossref] [PubMed]
- Gill J, Gorlick R. Advancing therapy for osteosarcoma. Nat Rev Clin Oncol 2021;18:609-24. [Crossref] [PubMed]
- Chen C, Xie L, Ren T, et al. Immunotherapy for osteosarcoma: Fundamental mechanism, rationale, and recent breakthroughs. Cancer Lett 2021;500:1-10. [Crossref] [PubMed]
- Corre I, Verrecchia F, Crenn V, et al. The Osteosarcoma Microenvironment: A Complex But Targetable Ecosystem. Cells 2020;9:976. [Crossref] [PubMed]
- Harrison DJ, Geller DS, Gill JD, et al. Current and future therapeutic approaches for osteosarcoma. Expert Rev Anticancer Ther 2018;18:39-50. [Crossref] [PubMed]
- Brown HK, Tellez-Gabriel M, Heymann D. Cancer stem cells in osteosarcoma. Cancer Lett 2017;386:189-95. [Crossref] [PubMed]
- Du L, Han XG, Tu B, et al. CXCR1/Akt signaling activation induced by mesenchymal stem cell-derived IL-8 promotes osteosarcoma cell anoikis resistance and pulmonary metastasis. Cell Death Dis 2018;9:714. [Crossref] [PubMed]
- Pietrovito L, Leo A, Gori V, et al. Bone marrow-derived mesenchymal stem cells promote invasiveness and transendothelial migration of osteosarcoma cells via a mesenchymal to amoeboid transition. Mol Oncol 2018;12:659-76. [Crossref] [PubMed]
- Cortini M, Massa A, Avnet S, et al. Tumor-Activated Mesenchymal Stromal Cells Promote Osteosarcoma Stemness and Migratory Potential via IL-6 Secretion. PLoS One 2016;11:e0166500. [Crossref] [PubMed]
- Baglio SR, Lagerweij T, Pérez-Lanzón M, et al. Blocking Tumor-Educated MSC Paracrine Activity Halts Osteosarcoma Progression. Clin Cancer Res 2017;23:3721-33. [Crossref] [PubMed]
- Qi J, Zhang R, Wang Y. Exosomal miR-21-5p derived from bone marrow mesenchymal stem cells promote osteosarcoma cell proliferation and invasion by targeting PIK3R1. J Cell Mol Med 2021;25:11016-30. [Crossref] [PubMed]
- Xu Z, Zhou X, Wu J, et al. Mesenchymal stem cell-derived exosomes carrying microRNA-150 suppresses the proliferation and migration of osteosarcoma cells via targeting IGF2BP1. Transl Cancer Res 2020;9:5323-35. [Crossref] [PubMed]
- Xu N, Wang X, Wang L, et al. Comprehensive analysis of potential cellular communication networks in advanced osteosarcoma using single-cell RNA sequencing data. Front Genet 2022;13:1013737. [Crossref]
- Mižíková I, Lesage F, Cyr-Depauw C, et al. Single-Cell RNA Sequencing-Based Characterization of Resident Lung Mesenchymal Stromal Cells in Bronchopulmonary Dysplasia. Stem Cells 2022;40:479-92. [Crossref] [PubMed]
- Stucky A, Gao L, Li SC, et al. Molecular Characterization of Differentiated-Resistance MSC Subclones by Single-Cell Transcriptomes. Front Cell Dev Biol 2022;10:699144. [Crossref] [PubMed]
- Zhou Y, Yang D, Yang Q, et al. Single-cell RNA landscape of intratumoral heterogeneity and immunosuppressive microenvironment in advanced osteosarcoma. Nat Commun 2020;11:6322. [Crossref] [PubMed]
- Zeng Z, Li W, Zhang D, et al. Development of a Chemoresistant Risk Scoring Model for Prechemotherapy Osteosarcoma Using Single-Cell Sequencing. Front Oncol 2022;12:893282. [Crossref] [PubMed]
- Feng J, Lu SS, Xiao T, et al. ANXA1 Binds and Stabilizes EphA2 to Promote Nasopharyngeal Carcinoma Growth and Metastasis. Cancer Res 2020;80:4386-98. [Crossref] [PubMed]
- Bai F, Zhang P, Fu Y, et al. Targeting ANXA1 abrogates Treg-mediated immune suppression in triple-negative breast cancer. J Immunother Cancer 2020;8:e000169. [Crossref] [PubMed]
- Tang X, Luo L, Li Y, et al. Therapeutic potential of targeting HSPA5 through dual regulation of two candidate prognostic biomarkers ANXA1 and PSAT1 in osteosarcoma. Aging (Albany NY) 2020;13:1212-35. [Crossref] [PubMed]
- Seshacharyulu P, Rachagani S, Muniyan S, et al. FDPS cooperates with PTEN loss to promote prostate cancer progression through modulation of small GTPases/AKT axis. Oncogene 2019;38:5265-80. [Crossref] [PubMed]
- Abate M, Laezza C, Pisanti S, et al. Deregulated expression and activity of Farnesyl Diphosphate Synthase (FDPS) in Glioblastoma. Sci Rep 2017;7:14123. [Crossref] [PubMed]
- Seshacharyulu P, Halder S, Nimmakayala R, et al. Disruption of FDPS/Rac1 axis radiosensitizes pancreatic ductal adenocarcinoma by attenuating DNA damage response and immunosuppressive signalling. EBioMedicine 2022;75:103772. [Crossref] [PubMed]
- Chen Z, Chen G, Zhao H. FDPS promotes glioma growth and macrophage recruitment by regulating CCL20 via Wnt/β-catenin signalling pathway. J Cell Mol Med 2020;24:9055-66. [Crossref] [PubMed]
- Notarnicola M, Messa C, Cavallini A, et al. Higher farnesyl diphosphate synthase activity in human colorectal cancer inhibition of cellular apoptosis. Oncology 2004;67:351-8. [Crossref] [PubMed]
- Liu BY, Lu YQ, Han F, et al. Effects of the overexpression of IFITM5 and IFITM5 c.-14C>T mutation on human osteosarcoma cells. Oncol Lett 2017;13:111-8. [Crossref] [PubMed]
- Lin IY, Yen CH, Liao YJ, et al. Identification of FKBP11 as a biomarker for hepatocellular carcinoma. Anticancer Res 2013;33:2763-9. [PubMed]
- Hagedorn M, Siegfried G, Hooks KB, et al. Integration of zebrafish fin regeneration genes with expression data of human tumors in silico uncovers potential novel melanoma markers. Oncotarget 2016;7:71567-79. [Crossref] [PubMed]
- Sun Z, Qin X, Fang J, et al. Multi-Omics Analysis of the Expression and Prognosis for FKBP Gene Family in Renal Cancer. Front Oncol 2021;11:697534. [Crossref] [PubMed]
- Qiu L, Liu H, Wang S, et al. FKBP11 promotes cell proliferation and tumorigenesis via p53-related pathways in oral squamous cell carcinoma. Biochem Biophys Res Commun 2021;559:183-90. [Crossref] [PubMed]
- Nakashima K, Zhou X, Kunkel G, et al. The novel zinc finger-containing transcription factor osterix is required for osteoblast differentiation and bone formation. Cell 2002;108:17-29. [Crossref] [PubMed]
- Qu S, Wu J, Bao Q, et al. Osterix promotes the migration and angiogenesis of breast cancer by upregulation of S100A4 expression. J Cell Mol Med 2019;23:1116-27. [Crossref] [PubMed]
- Cao Y, Jia SF, Chakravarty G, et al. The osterix transcription factor down-regulates interleukin-1 alpha expression in mouse osteosarcoma cells. Mol Cancer Res 2008;6:119-26. [Crossref] [PubMed]
- Cao Y, Zhou Z, de Crombrugghe B, et al. Osterix, a transcription factor for osteoblast differentiation, mediates antitumor activity in murine osteosarcoma. Cancer Res 2005;65:1124-8. [Crossref] [PubMed]
- Tang W, Xu F, Zhao M, et al. Ferroptosis regulators, especially SQLE, play an important role in prognosis, progression and immune environment of breast cancer. BMC Cancer 2021;21:1160. [Crossref] [PubMed]
- Sun H, Li L, Li W, et al. p53 transcriptionally regulates SQLE to repress cholesterol synthesis and tumor growth. EMBO Rep 2021;22:e52537. [Crossref] [PubMed]
- Liu D, Wong CC, Fu L, et al. Squalene epoxidase drives NAFLD-induced hepatocellular carcinoma and is a pharmaceutical target. Sci Transl Med 2018;10:eaap9840. [Crossref] [PubMed]
- Liu Y, Fang L, Liu W. High SQLE Expression and Gene Amplification Correlates with Poor Prognosis in Head and Neck Squamous Cell Carcinoma. Cancer Manag Res 2021;13:4709-23. [Crossref] [PubMed]
- Xu Z, Huang L, Dai T, et al. SQLE Mediates Metabolic Reprogramming to Promote LN Metastasis in Castration-Resistant Prostate Cancer. Onco Targets Ther 2021;14:4285-95. [Crossref] [PubMed]
- Wang J, Tang C, Yang C, et al. Tropomyosin-1 Functions as a Tumor Suppressor with Respect to Cell Proliferation, Angiogenesis and Metastasis in Renal Cell Carcinoma. J Cancer 2019;10:2220-8. [Crossref] [PubMed]
- Bharadwaj S, Prasad GL. Tropomyosin-1, a novel suppressor of cellular transformation is downregulated by promoter methylation in cancer cells. Cancer Lett 2002;183:205-13. [Crossref] [PubMed]
- Jiang R, Zhang C, Liu G, et al. MicroRNA-107 Promotes Proliferation, Migration, and Invasion of Osteosarcoma Cells by Targeting Tropomyosin 1. Oncol Res 2017;25:1409-19. [Crossref] [PubMed]
(English Language Editor: L. Huleatt)