Prognostic factors of survival in patients with non-small cell lung cancer: a competing risk model using the SEER database
Introduction
Lung cancer is the leading cause of an extremely high mortality rate worldwide, accounting for approximately 27% of cancer deaths in the United States (1). Non-small cell lung cancer (NSCLC) accounts for about 80% of lung cancer cases and the 5-year survival rate is reduced to 5% or less (2,3), which indicated the poor prognosis of NSCLC.
Previous studies have investigated the prognostic factors in NSCLC patients, including age, gender, treatment method, tumor stage, examined lymph node count, etc. (4-8). To our knowledge, little attention has been paid to the existence of competing risks, that is, these patients may also die from other causes in addition to NSCLC. And previous studies tend to mix the two causes of death into one single endpoint event or delete the cases dead from other causes (9,10). The competing risk model developed a new method for regression analysis that corresponds to the hazard model of the cumulative incidence function (11). And it has been widely applied in clinical oncology studies for identifying influencing factors for improving the prognosis of malignant tumors such as lung cancer, breast cancer, and hepatocellular carcinoma (12-14). To date, few studies have used the competing risk model for survival analysis of NSCLC. Lobectomy and lymph node dissection are recognized as standard treatments for early-stage NSCLC (15). A study suggested that lobectomy should be considered the surgery of choice for pleural invasion patients with NSCLC (16). At present, the impact of different surgery types on the survival of patients with NSCLC needs further research.
Given this, we performed a competing risk model to explore the prognostic factors of the 1-, 3-, and 5-year survival of NSCLC, and explored the performance of the model under different surgery types, which may help clinicians to provide precision treatment and improve the quality of life for NSCLC patients. We present the following article in accordance with the STROBE reporting checklist (available at https://tcr.amegroups.com/article/view/10.21037/tcr-21-2114/rc).
Methods
Study population and data acquisition
NSCLC patients with complete data we studied in this retrospective cohort study were extracted from the Surveillance, Epidemiology and End Results (SEER) database between 2010 and 2015. The SEER database is representative of the US population, extracting patient-level data from 18 geographically diverse populations representing rural, urban, and regional populations (17). Patients without lymph node examination and with incomplete information on the variables we studied were excluded. The study was conducted in accordance with the Declaration of Helsinki (as revised in 2013). The study used the SEER database and all patient identifiers were removed from the SEER database, which was exempt from institutional review board approval. Individual consent for this retrospective analysis was waived.
Baseline variables including age (<65, 65–74, and ≥75 years old), gender (male and female), ethnicity (White, Asian, Black and others), primary tumor site (upper lobe, middle lobe, lower lobe, main bronchus and overlapping lesion), TNM staging [T stage (T1-4), N stage (N0-3) and M stage (M0-1)], surgery type (no surgery of primary site, excision or resection of less than one lobe, lobe or bilobectomy extended, resection of at least one lobe or bilobectomy, and pneumonectomy), tumor size, LNs count, follow-up time, and patient outcome were collected in the SEER database. The patients were staged according to the eighth edition of the TNM classification (18). The LNs count was divided into <16 and ≥16 (17). The outcomes of this study included cancer-specific mortality in NSCLC and other-causes mortality. Patients who were alive at the end of the study were defined as the censored. According to their outcomes, patients were divided into three groups: the censored, cancer-specific mortality, and other-cause mortality. In the present study, other-causes mortality was the competing event.
Statistical analysis
All statistical tests were performed using the two-sided test, and P<0.05 was considered statistically significant. All statistical analyses were completed using SAS 9.4 (SAS Institute Inc., Cary, NC, USA) and R 3.6.0 software. Characteristics of the included patients were described. Gray’s test (19) was used in univariable analysis to compare the cumulative incidences among three groups (the censored, cancer-specific mortality, and other-cause mortality). Predictor screening was performed using a multivariable Fine-Gray (19) competing risk model with backward elimination (P<0.05) to explore the prognostic factors of the survival of NSCLC. The screened variables were incorporated into a random survival forest (RSF) model (20) for the prediction of 1-, 3-, and 5-year survival in patients with NSCLC. The population was divided into training and testing sets in a ratio of 7:3. The performance of the RSF model was evaluated using receiver operator characteristic (ROC) curves, calibration curves, and the value of area under the curve (AUC). Decision curve analysis (DCA) was used to compare the performance of the RSF model we developed with the eighth edition of the American Joint Committee on Cancer (AJCC) staging. The performance of the RSF model was also reported under different surgery types, T, N, and M stages.
Results
Baseline description
Initially, a total of 17,923 NSCLC patients were extracted from the SEER database. After excluding patients without lymph node examination (n=14,059), with incomplete data including age, gender and ethnicity (n=2,508), tumor size (n=5), and surgery types (n=62), and patients with T0 or TX staging (n=38), we finally enrolled 1,251 eligible NSCLC patients (Figure 1). There were 721 (57.63%) males and 530 (42.37%) females. Among them, there were 997 (79.70%) White patients, 82 (6.55%) Asian patients, 162 (12.95%) Black patients, and 10 (0.80%) patients of other ethnicities. The median number of LNs was 5 [2, 11], where 1,064 (85.05%) patients have <16 LNs and 187 (14.95%) have ≥16 LNs. The median follow-up time was 26 [8, 55] months with a maximum follow-up of 95 months. Besides, 445 (35.57%) patients were censored, 678 (54.20%) patients were cancer-specific mortality, and 128 (10.23%) patients were other-cause mortality. All baseline characteristics were shown in Table 1.
Table 1
Variables | Description (n=1,251) |
---|---|
Age (years), n (%) | |
<65 | 491 (39.25) |
65–74 | 472 (37.73) |
≥75 | 288 (23.02) |
Gender, n (%) | |
Male | 721 (57.63) |
Female | 530 (42.37) |
Ethnicity, n (%) | |
White | 997 (79.70) |
Asian | 82 (6.55) |
Black | 162 (12.95) |
Others | 10 (0.80) |
Primary site, n (%) | |
Upper lobe | 810 (64.75) |
Middle lobe | 78 (6.24) |
Lower lobe | 316 (25.26) |
Main bronchus | 30 (2.40) |
Overlapping lesion | 17 (1.36) |
T stage, n (%) | |
T1 | 384 (30.70) |
T2 | 444 (35.49) |
T3 | 263 (21.02) |
T4 | 160 (12.79) |
N stage, n (%) | |
N0 | 587 (46.92) |
N1 | 126 (10.07) |
N2 | 382 (30.54) |
N3 | 156 (12.47) |
M stage, n (%) | |
M0 | 967 (77.30) |
M1 | 284 (22.70) |
Surgery type, n (%) | |
No surgery of primary site | 536 (42.85) |
Excision or resection of less than one lobe | 91 (7.27) |
Lobe or bilobectomy extended | 41 (3.28) |
Resection of at least one lobe or bilobectomy | 532 (42.53) |
Pneumonectomy | 51 (4.08) |
Tumor size (mm), M (Q1, Q3) | 35.00 (21.00, 52.00) |
LNs count, n (%) | |
<16 | 1,064 (85.05) |
≥16 | 187(14.95) |
LNs count, M (Q1, Q3) | 5.00 (2.00, 11.00) |
Follow-up time, months, M (Q1, Q3) | 26.00 (8.00, 55.00) |
Outcome, n (%) | |
Censored | 445 (35.57) |
Dead from NSCLC | 678 (54.20) |
Dead from other causes | 128 (10.23) |
1-year outcome, n (%) | |
Censored | 844 (68.01) |
Dead from NSCLC | 350 (28.20) |
Dead from other causes | 47 (3.79) |
3-year outcome*, n (%) | |
Censored | 653 (52.92) |
Dead from NSCLC | 514 (41.65) |
Dead from other causes | 67 (5.43) |
5-year outcome#, n (%) | |
Censored | 486 (41.65) |
Dead from NSCLC | 596 (51.07) |
Dead from other causes | 85 (7.28) |
*, 17 patients were lost to follow-up during 3-year follow-up duration; #, 84 patients were lost to follow-up during 5-year follow-up duration. M (Q1, Q3), median and interquartile range; LNs, lymph nodes; NSCLC, non-small cell lung cancer.
Cumulative incidences of cancer-specific mortality in NSCLC
The cumulative incidence of cancer-specific mortality in NSCLC was shown in Table 2. The cancer-specific mortality in NSCLC was significantly different in age (P=0.012), gender (P=0.040), primary tumor site (P<0.001), T stage (P<0.001), N stage (P<0.001), M stage (P<0.001), surgery type (P<0.001) and LNs count (P<0.001). The cumulative incidences of cancer-specific mortality in different age ranges were 56.685%, 58.863%, and 65.698%, respectively. The cumulative incidences of cancer-specific mortality in male and female patients were 63.372% and 56.347%, respectively. The cumulative incidences of cancer-specific mortality in patients undergoing different surgery types were 85.792%, 52.442%, 48.841%, 36.923% and 44.055%, respectively. More details were shown in Table 2 and Figure S1.
Table 2
Variables | P | CIF | S.E | 95% CI | |
---|---|---|---|---|---|
Lower | Upper | ||||
Age (years) | |||||
<65 | 0.012 | 56.685 | 0.097 | 56.495 | 56.875 |
65–74 | 58.863 | 0.069 | 58.728 | 58.998 | |
≥75 | 65.698 | 0.123 | 65.457 | 65.939 | |
Gender | |||||
Male | 0.040 | 63.372 | 0.098 | 63.180 | 63.564 |
Female | 56.347 | 0.061 | 56.227 | 56.467 | |
Ethnicity | |||||
White | 0.660 | 59.19 | 0.043 | 59.106 | 59.274 |
Asian | 69.913 | 0.423 | 69.084 | 70.742 | |
Black | 57.701 | 0.192 | 57.325 | 58.077 | |
Others | 63.333 | 3.456 | 56.559 | 70.107 | |
Primary site | |||||
Main bronchus | <0.001 | 70.988 | 0.893 | 69.238 | 72.738 |
Upper lobe | 58.501 | 0.051 | 58.401 | 58.601 | |
Middle lobe | 56.620 | 0.482 | 55.675 | 57.565 | |
Lower lobe | 60.286 | 0.109 | 60.072 | 60.5 | |
Overlapping lesion | 88.235 | 0.809 | 86.649 | 89.821 | |
T stage | |||||
T1 | <0.001 | 47.721 | 0.084 | 47.556 | 47.886 |
T2 | 56.672 | 0.078 | 56.519 | 56.825 | |
T3 | 68.247 | 0.176 | 67.902 | 68.592 | |
T4 | 79.841 | 0.132 | 79.582 | 80.100 | |
N stage | <0.001 | ||||
N0 | 42.409 | 0.066 | 42.280 | 42.538 | |
N1 | 47.223 | 0.230 | 46.772 | 47.674 | |
N2 | 77.780 | 0.077 | 77.629 | 77.931 | |
N3 | 88.086 | 0.079 | 87.931 | 88.241 | |
M stage | <0.001 | ||||
M0 | 51.051 | 0.037 | 50.978 | 51.124 | |
M1 | 88.494 | 0.108 | 88.282 | 88.706 | |
Surgery type | <0.001 | ||||
No surgery of primary site | 85.792 | 0.053 | 85.688 | 85.896 | |
Excision or resection of less than one lobe | 52.442 | 0.368 | 51.721 | 53.163 | |
Lobe or bilobectomy extended | 48.841 | 0.723 | 47.424 | 50.258 | |
Resection of at least one lobe or bilobectomy | 36.923 | 0.067 | 36.792 | 37.054 | |
Pneumonectomy | 44.055 | 0.644 | 42.793 | 45.317 | |
LNs count | <0.001 | ||||
<16 | 62.836 | 0.039 | 62.760 | 62.912 | |
≥16 | 41.812 | 0.191 | 41.438 | 42.186 |
NSCLC, non-small cell lung cancer; CIF, cumulative incidence function; S.E, standard error; CI, confidence interval; LNs, lymph nodes.
Multivariable competing risk analysis
Age, primary site, N stage, M stage, surgery type, tumor size, and LNs count were included in the multivariable Fine-Gray model for further analysis (Table 3). Patients aged ≥75 years had a 1.506-fold higher risk of cancer-specific mortality compared to those aged <65 years [hazard ratios (HR) =1.506, 95% confidence interval (CI): 1.235–1.837]. As compared with patients whose primary tumor site was the upper lobe, patients with the primary site of the lower lobe (HR =1.227, 95% CI: 1.018–1.479) and overlapping lesion (HR =2.057, 95% CI: 1.135–3.728) had higher risks of cancer-specific mortality. Higher T and M stages were associated with higher risks of cancer-specific mortality for tumor staging. Compared to patients with no surgery of the primary site, patients with excision or resection of less than one lobe (HR =2.130, 95% CI: 1.254–3.617) had an increased risk of cancer-specific mortality. Tumor size was associated with the increased risk of cancer-specific mortality (HR =1.007, 95% CI: 1.005–1.008) (Table 3). Patients with ≥16 LNs had a reduced risk of cancer-specific mortality compared with those with <16 LNs (HR =0.980, 95% CI: 0.965–0.995).
Table 3
Variables | β | S.E | P | HR | 95% CI | |
---|---|---|---|---|---|---|
Lower | Upper | |||||
Age (years) | ||||||
<65 | Ref | |||||
65–74 | 0.198 | 0.097 | 0.040 | 1.219 | 1.009 | 1.473 |
≥75 | 0.410 | 0.101 | <0.001 | 1.506 | 1.235 | 1.837 |
Primary site | ||||||
Upper lobe | Ref | |||||
Middle lobe | −0.010 | 0.165 | 0.953 | 0.990 | 0.717 | 1.368 |
Lower lobe | 0.205 | 0.095 | 0.032 | 1.227 | 1.018 | 1.479 |
Main bronchus | 0.054 | 0.237 | 0.819 | 1.056 | 0.664 | 1.680 |
Overlapping lesion | 0.721 | 0.303 | 0.018 | 2.057 | 1.135 | 3.728 |
N stage | ||||||
N0 | Ref | |||||
N1 | 0.212 | 0.163 | 0.192 | 1.236 | 0.899 | 1.700 |
N2 | 0.393 | 0.107 | <0.001 | 1.482 | 1.201 | 1.830 |
N3 | 0.593 | 0.144 | <0.001 | 1.810 | 1.363 | 2.402 |
M stage | ||||||
M0 | Ref | |||||
M1 | 0.707 | 0.096 | <0.001 | 2.029 | 1.680 | 2.450 |
Surgery type | ||||||
No surgery of primary site | Ref | |||||
Excision or resection of less than one lobe | 0.756 | 0.270 | 0.005 | 2.130 | 1.254 | 3.617 |
Lobe or bilobectomy extended | 0.304 | 0.300 | 0.311 | 1.355 | 0.753 | 2.440 |
Resection of at least one lobe or bilobectomy | 0.378 | 0.346 | 0.274 | 1.460 | 0.741 | 2.876 |
Pneumonectomy | −0.093 | 0.265 | 0.725 | 0.911 | 0.542 | 1.532 |
Tumor size | 0.007 | 0.001 | <0.001 | 1.007 | 1.005 | 1.008 |
LNs count | ||||||
<16 | Ref | |||||
≥16 | −0.020 | 0.008 | 0.008 | 0.980 | 0.965 | 0.995 |
Ref, reference; β, beta coefficient; S.E, standard error; HR, hazard ratios; CI, confidence interval; LNs, lymph nodes.
Development and validation of the RSF model for the prediction of survival in patients with NSCLC
There was no significant difference in the characteristics between the training set (n=876) and the testing set (n=375), as shown in Table S1. The six most important features (surgery type, tumor size, M stage, LNs count, N stage, and primary site) were shown in Table 4. Surgery type (0.1846) was the most crucial survival predictor. The ROC curves and calibration curves in the training and testing sets were in Figure 2A,2B and Figure 3A,3B, respectively. The value of AUC for predicting 1-year survival, 3-year survival, and 5-year survival in the testing set were 0.796, 0.804, and 0.792, respectively (Table 5). The results of DCA showed that the RSF model had a positive net benefit to patients, and compared with the 8th edition AJCC staging, the performance of the RSF model was similar or has greater clinical net benefits in 1-year (Figure 4A,4B), 3-year (Figure 4C,4D) and 5-year (Figure 4E,4F) survival evaluation in the training and testing sets. Table 5 shows the good performance of the RSF model under different surgery types, T stages, N stages, and M stages.
Table 4
Variables | Variable importance |
---|---|
Surgery type | 0.1846 |
Tumor size | 0.0762 |
M stage | 0.0751 |
LNs count | 0.0364 |
N stage | 0.0340 |
Primary site | 0.0265 |
RSF, random survival forest; LNs, lymph nodes.
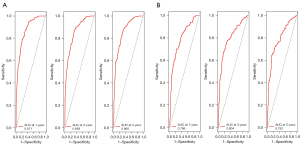
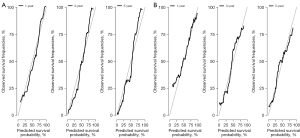
Table 5
Subgroups | AUC for predicting 1-year survival | AUC for predicting 3-year survival | AUC for predicting 5-year survival |
---|---|---|---|
Training set | 0.871 | 0.875 | 0.865 |
Testing set | 0.796 | 0.804 | 0.792 |
Surgery type | |||
No surgery of primary site | 0.880 | 0.779 | 0.714 |
Excision or resection of less than one lobe | 0.812 | 0.799 | 0.774 |
Lobe or bilobectomy extended | 0.841 | 0.807 | 0.756 |
Resection of at least one lobe or bilobectomy | 0.799 | 0.861 | 0.820 |
Pneumonectomy | 0.802 | 0.757 | 0.765 |
T stage | |||
T1 | 0.825 | 0.843 | 0.856 |
T2 | 0.852 | 0.825 | 0.831 |
T3 | 0.810 | 0.807 | 0.820 |
T4 | 0.817 | 0.889 | 0.893 |
N stage | |||
N0 | 0.807 | 0.790 | 0.781 |
N1 | 0.856 | 0.835 | 0.856 |
N2 | 0.809 | 0.777 | 0.781 |
N3 | 0.764 | 0.828 | 0.769 |
M stage | |||
M0 | 0.792 | 0.791 | 0.805 |
M1 | 0.771 | 0.862 | 0.851 |
RSF, random survival forest; NSCLC, non-small cell lung cancer; AUC, area under the curve.
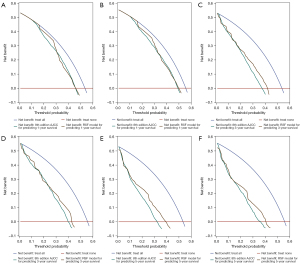
We generated a web calculator (https://github.com/YingChen19/Prognostic-factors-of-long-term-survival-of-non-small-cell-lung-cancer) for calculating the survival of NSCLC patients based on the RSF model, which could provide the convenient assessment.
Discussion
In the present study, surgery type, tumor size, M stage, LNs count, N stage, and primary site were included in the competing risk analysis using the RSF model for predicting 1-, 3-, and 5-year survival. The values of AUC in the training and testing sets were good, and calibration curves were well-fitted. DCA curves showed that the RSF model had similar or greater clinical net benefits in survival compared with the 8th edition AJCC staging. We generated a user-friendly web calculator to ease use in clinical practice.
The prognosis and survival of NSCLC are dependent on the stage of the disease, which is associated with the tumor size and nodal metastasis (21). Previous studies have demonstrated that the number of LNs was an independent prognostic factor for the survival of NSCLC patients (7,8). Sun et al. (22) established a model to predict the survival of elderly patients with metastatic NSCLC, and found that factors such as primary site and N stage were its prognostic factors. Dong et al. (23) found that factors such as primary site, N stage, and surgery were associated with cancer-specific survival in patients with NSCLC with bone metastases. These studies were consistent with our findings, which were surgery type, tumor size, M stage, LNs count, N stage, and primary site were prognostic factors in the RSF model.
To our knowledge, there are few studies on the long-term survival of NSCLC patients using competing risk models. In our study, cancer-specific mortality in NSCLC was set as an event of interest and other-causes mortality as a competing event. In the presence of competing risks, the relative risk of a patient dying from NSCLC differs from that when only a single endpoint event is considered. For example, in another database study, David et al. reported that the risks of death in NSCLC patients ≥75 years at stages I, II, and III were 1.29, 1.03, and 0.84 times significantly higher than those <65 years, respectively (21), while through the Fine-Gray model in our study, the risk of death from NSCLC in patients ≥75 years was only 0.506 times higher compared to those <65 years. Given this, we may propose that the existence of competing risks should be taken into full consideration when analyzing survival issues to avoid biased results. Through the Fine and Gray model, we could provide more direct and accurate estimates of the cumulative incidences of death from NSCLC. The screened prognostic factors were put into the RSF model, which could help clinicians provide precision treatment and improve the quality of life for NSCLC patients.
RSF has emerged as an attractive predictive tool as a machine learning method with less restrictive model assumptions (20,24). We predicted the 1-, 3-, and 5-year survival of NSCLC patients by the RSF model, which performed well by ROC, calibration curves, and DCA analysis. Clinicians should use prediction models in the practice, but machine learning models are difficult to interpret meaningfully. We built a web computing tool to visually demonstrate the clinical application value of our model. The variables in the RSF model are simple and easy to obtain.
However, this study is still subject to some limitations. First, this study is limited by its retrospective nature. Second, the study population in the SEER database was mainly American, which requires more cohorts from different regions for verification. Third, the follow-up time was relatively short, which may affect the estimation of cumulative incidence. Finally, we used the past version of the SEER Cancer Statistics Review that includes statistics from 1975 through 2016, we could not collect the histological type of NSCLC, marital status, rural or urban residence, income, and education level before, these variables might be associated with the prognosis of NSCLC. We were also unable to compare the survival of patients undergoing radical treatment and those with disseminated disease due to the lack of data on patients undergoing radical treatment. Herein, long-term, multicenter, and prospective clinical studies are therefore suggested.
Based on the SEER database, we established the RSF model for predicting the 1-, 3- and 5-year survival of NSCLC and generated a web calculator (https://github.com/YingChen19/Prognostic-factors-of-long-term-survival-of-non-small-cell-lung-cancer). The obtained results may provide a reference for survival prediction for NSCLC management, and could help improve the prognosis of NSCLC.
Acknowledgments
Funding: This research was funded by
Footnote
Reporting Checklist: The authors have completed the STROBE reporting checklist. Available at https://tcr.amegroups.com/article/view/10.21037/tcr-21-2114/rc
Conflicts of Interest: All authors have completed the ICMJE uniform disclosure form (available at https://tcr.amegroups.com/article/view/10.21037/tcr-21-2114/coif). The authors have no conflicts of interest to declare.
Ethical Statement: The authors are accountable for all aspects of the work in ensuring that questions related to the accuracy or integrity of any part of the work are appropriately investigated and resolved. The study was conducted in accordance with the Declaration of Helsinki (as revised in 2013). The study used the SEER database and all patient identifiers were removed from the SEER database, which was exempt from institutional review board approval. Individual consent for this retrospective analysis was waived.
Open Access Statement: This is an Open Access article distributed in accordance with the Creative Commons Attribution-NonCommercial-NoDerivs 4.0 International License (CC BY-NC-ND 4.0), which permits the non-commercial replication and distribution of the article with the strict proviso that no changes or edits are made and the original work is properly cited (including links to both the formal publication through the relevant DOI and the license). See: https://creativecommons.org/licenses/by-nc-nd/4.0/.
References
- Howlader N, NA KM, Miller D, et al. SEER cancer statistics factsheets: lung and bronchus cancer. National Cancer Institute, Bethesda, Maryland 2016.
- National Cancer Institute. SEER Cancer Statistics Review (CSR) 1975-2013. Available online: https://seer.cancer.gov/archive/csr/1975_2005/results_merged/sect_33_VA_adjustment.pdf
- Bittoni MA, Arunachalam A, Li H, et al. Real-World Treatment Patterns, Overall Survival, and Occurrence and Costs of Adverse Events Associated With First-line Therapies for Medicare Patients 65 Years and Older With Advanced Non-small-cell Lung Cancer: A Retrospective Study. Clin Lung Cancer 2018;19:e629-45. [Crossref] [PubMed]
- Thakur MK, Gadgeel SM. Predictive and Prognostic Biomarkers in Non-Small Cell Lung Cancer. Semin Respir Crit Care Med 2016;37:760-70. [Crossref] [PubMed]
- Chansky K, Sculier JP, Crowley JJ, et al. The International Association for the Study of Lung Cancer Staging Project. Prognostic factors and pathologic TNM stage in surgically managed non-small cell lung cancer. Zhongguo Fei Ai Za Zhi 2010;13:9-18. [PubMed]
- Sculier JP, Chansky K, Crowley JJ, et al. The impact of additional prognostic factors on survival and their relationship with the anatomical extent of disease expressed by the 6th Edition of the TNM Classification of Malignant Tumors and the proposals for the 7th Edition. J Thorac Oncol 2008;3:457-66.
- Saji H, Tsuboi M, Yoshida K, et al. Prognostic impact of number of resected and involved lymph nodes at complete resection on survival in non-small cell lung cancer. J Thorac Oncol 2011;6:1865-71. [Crossref] [PubMed]
- Fukui T, Mori S, Yokoi K, et al. Significance of the number of positive lymph nodes in resected non-small cell lung cancer. J Thorac Oncol 2006;1:120-5. [Crossref] [PubMed]
- Martinez-Meehan D, Lutfi W, Dhupar R, et al. Factors Associated With Survival in Complete Pathologic Response Non-Small Cell Lung Cancer. Clin Lung Cancer 2020;21:349-56. [Crossref] [PubMed]
- Wen YS, Xi KX, Xi KX, et al. The number of resected lymph nodes is associated with the long-term survival outcome in patients with T2 N0 non-small cell lung cancer. Cancer Manag Res 2018;10:6869-77. [Crossref] [PubMed]
- Kuk D, Varadhan R. Model selection in competing risks regression. Stat Med 2013;32:3077-88. [Crossref] [PubMed]
- Wu X, Wang Y, Lin X, et al. Racial and Ethnic Disparities in Lung Adenocarcinoma Survival: A Competing-Risk Model. Clin Lung Cancer 2020;21:e171-81. [Crossref] [PubMed]
- Li Y, Zhang H, Zhang W, et al. A Competing Risk Analysis Model to Determine the Prognostic Value of Isolated Tumor Cells in Axillary Lymph Nodes for T1N0M0 Breast Cancer Patients Based on the Surveillance, Epidemiology, and End Results Database. Front Oncol 2020;10:572316. [Crossref] [PubMed]
- He C, Zhang Y, Lin X. Increased Overall Survival and Decreased Cancer-Specific Mortality in Patients with Hepatocellular Carcinoma Treated by Transarterial Chemoembolization and Human Adenovirus Type-5 Combination Therapy: a Competing Risk Analysis. J Gastrointest Surg 2018;22:989-97. [Crossref] [PubMed]
- Xu J, Huang L, Wang Y, et al. A Retrospective Study of Effectiveness of Thoracoscopic Lobectomy and Segmentectomy in Patients with Early-Stage Non-Small-Cell Lung Cancer. Dis Markers 2022;2022:6975236. [Crossref] [PubMed]
- Song X, Xie Y, Zhu Y, et al. Is lobectomy superior to sub-lobectomy in non-small cell lung cancer with pleural invasion? A population-based competing risk analysis. BMC Cancer 2022;22:541. [Crossref] [PubMed]
- Liang W, He J, Shen Y, et al. Impact of Examined Lymph Node Count on Precise Staging and Long-Term Survival of Resected Non-Small-Cell Lung Cancer: A Population Study of the US SEER Database and a Chinese Multi-Institutional Registry. J Clin Oncol 2017;35:1162-70. [Crossref] [PubMed]
- Detterbeck FC, Chansky K, Groome P, et al. The IASLC Lung Cancer Staging Project: Methodology and Validation Used in the Development of Proposals for Revision of the Stage Classification of NSCLC in the Forthcoming (Eighth) Edition of the TNM Classification of Lung Cancer. J Thorac Oncol 2016;11:1433-46. [Crossref] [PubMed]
- Austin PC, Lee DS, Fine JP. Introduction to the Analysis of Survival Data in the Presence of Competing Risks. Circulation 2016;133:601-9. [Crossref] [PubMed]
- Ishwaran H, Kogalur UB, Blackstone EH, et al. Random survival forests. The Annals of Applied Statistics 2008;2:841-60. [Crossref]
- David EA, Cooke DT, Chen Y, et al. Does Lymph Node Count Influence Survival in Surgically Resected Non-Small Cell Lung Cancer? Ann Thorac Surg 2017;103:226-35. [Crossref] [PubMed]
- Sun H, Liu M, Yang X, et al. Construction and validation of prognostic nomograms for elderly patients with metastatic non-small cell lung cancer. Clin Respir J 2022;16:380-93. [Crossref] [PubMed]
- Dong Q, Deng J, Mok TN, et al. Construction and Validation of Two Novel Nomograms for Predicting the Overall Survival and Cancer-Specific Survival of NSCLC Patients with Bone Metastasis. Int J Gen Med 2021;14:9261-72. [Crossref] [PubMed]
- Yang L, Fan X, Qin W, et al. A novel deep learning prognostic system improves survival predictions for stage III non-small cell lung cancer. Cancer Med 2022;11:4246-55. [Crossref] [PubMed]