A CT-based radiomics signature for prediction of HER2 overexpression and treatment efficacy of trastuzumab in advanced gastric cancer
Introduction
Gastric cancer (GC) is the fifth most common malignancy and the fourth greatest cause of cancer-related death (1). In addition, GC is the most common and fatal cancer in men in several south-central Asian countries (1). Attributed to endoscopic screening, the mortality of GC has decreased in some countries, but given the low screening rates in China, most GC patients are still identified at an advanced stage with poor prognosis. Human epidermal growth factor receptor 2 (HER2) is one of the crucial therapeutic targets for various solid tumors, including GC. Overexpression of HER2 is associated with unfavorable prognosis and plays a crucial role in the tumorigenesis of GC (2,3). Trastuzumab, a monoclonal antibody targeting HER2, can inhibit tumor cell proliferation by blocking the downstream signal transduction pathways. The ToGA (Trastuzumab for Gastric Cancer) trial demonstrated that trastuzumab with chemotherapy prolonged the survival of patients with HER2-positive advanced gastric cancer (AGC) (4). For AGC patients with HER2 overexpression, trastuzumab is recommended as the first-line target therapy by the National Comprehensive Cancer Network (NCCN) guidelines (5). However, GC is a highly heterogeneous disease. Compared with breast cancer, HER2 expression exhibits higher intratumoral heterogeneity in GC (6,7). As histological examination of surgically resected or biopsied samples only covers a fraction of the tumor, patients with a false-negative for HER2 overexpression may miss out on targeted therapy. Therefore, accurate evaluation of HER2 status has particular significance for GC patients.
Artificial intelligence (AI) techniques provide new methods to process images and translate them into quantitative data, enabling identification of microscopic features of tumors that are invisible to the human eye (8). Accumulating evidence shows that radiomics can be applied to various aspects including diagnosis, prediction of metastasis risk, survival, and treatment response for GC patients (9,10). Previous studies have reported that gene mutation status can be predicted by radiomics features (11,12). Besides, radiomics analysis combined with 3-dimensional (3D) reconstruction technology allows the extraction of image features from the whole volume of the lesion, providing more comprehensive information of intratumoral heterogeneity (8). Therefore, radiomics can serve as a robust and noninvasive technique in the evaluation of tumor gene expression. Nevertheless, few studies have explored the clinical application value of the radiomics method for evaluation of HER2 status in AGC (13).
In the current study, we established and validated a contrast-enhanced computed tomography (CECT)-based radiomics signature for predicting HER2 overexpression in AGC patients. The association of the signature with survival and treatment efficacy of trastuzumab in AGC was also investigated. We present the following article in accordance with the STARD reporting checklist (available at https://tcr.amegroups.com/article/view/10.21037/tcr-22-1690/rc).
Methods
Study population
A total of 536 consecutive AGC patients including 377 males (median age, 60 years; interquartile range, 53–66 years) and 159 females (median age, 57 years; interquartile range, 49–64 years) from between January 2016 and December 2017 were retrospectively enrolled. According to the time of diagnosis, all patients were separated into a training set (n=357) and a testing set (n=179) at a ratio of 2:1. The inclusion criteria were as follows: (I) underwent radical gastrectomy or endoscopic biopsy; (II) AGC diagnosis confirmed pathologically; (III) abdominal CECT scans performed within 2 weeks before biopsy or surgery; (IV) HER2 status available; and (V) adequate imaging quality for analysis: (i) gastric cavity sufficiently distended; (ii) no severe peristaltic or respiratory artifacts. The exclusion criteria were as follows: (I) lack of complete clinical records; (II) administration of any treatment prior to CT scan; and (III) presence of other malignant disease.
The study was conducted in accordance with the Declaration of Helsinki (as revised in 2013). This study was approved by the Institutional Review Board of Tianjin Medical University Cancer Institute and Hospital (ID: ek2020125) and individual consent for this observational, retrospective analysis was waived.
The clinical and pathological information of all patients was obtained. Pathologic staging was determined in accordance with the 8th edition of the American Joint Committee on Cancer (AJCC) staging system (14). The patient’s recruitment flow chart is illustrated in Figure S1.
HER2 status assessment
HER2 status was assessed according to NCCN guidelines (5). HER2 testing was performed as previously described (4). In brief, immunohistochemistry (IHC) and fluorescence in situ hybridization (FISH) were used to determine HER2 status. An IHC staining score of 3+ or 2+ with FISH+ was deemed positive. Patients with IHC scores of 0, 1+, or 2+ with FISH− were considered negative.
Treatment response evaluation
The efficacy of trastuzumab was assessed according to the Response Evaluation Criteria in Solid Tumors (RESICT) 1.1 (15). The endpoints included 4 grades: complete response (CR), partial response (PR), stable disease (SD), and progressive disease (PD).
Lesion segmentation and feature extraction
Abdominal CECT images in the arterial phase (AP), portal phase (PP) and delayed phase (DP) were analyzed. 3D Slicer software (http://www.slicer.org) was used to construct the volume of interest (VOI) of lesions. The VOI of each lesion in the 3 phases was manually delineated along the margin of tumor. Adjacent fluid or air was carefully avoided, with contours and reconstructed sagittal and coronal images used as references. The process of segmentation was conducted under the consensus of 2 radiologists (XJG and TTM, with 7 and 10 years, respectively, of experience in the interpretation abdominal CT images). Feature extraction was implemented with an open-source platform (PyRadiomics 2.2.0; https://pyradiomics.readthedocs.io/_/downloads/en/2.2.0/pdf/) (16). A total of 859 features were extracted and grouped into four types: size and shape, first-order statistical, textural features, and wavelet features. The details of the CT scanning protocol and image pre-processing are given in the supplementary methods (Appendix 1).
Establishment of the radiomics signature
The flowchart of the overall radiomics analysis is shown in Figure 1. To ensure the reliability of the selected features, we assessed the intra- and inter-observer agreements using intra- and inter-class correlation coefficients. We randomly chose 120 patients (one-third of the patients) from the training set and the VOI segmentation was independently performed by 2 readers. Reader 1 repeated the procedure 2 weeks later. The features were regarded as stable if the intra- and inter-class correlation coefficient values were >0.85. The patients were divided into HER2+ and HER2– groups. Features that differed significantly between groups were identified using the Mann-Whitney U test. Bonferroni correction was used to control type 1 error, and features with false discovery rate (FDR)-adjusted P<0.05 were selected for further analysis. The least absolute shrinkage and selection operator (LASSO) model was subsequently utilized to build a radiomics signature with non-zero coefficients. The 10-fold cross-testing was used to identify the optimal regularization parameter λ. The radiomics score (R-score) was calculated based on the fitting formula of the radiomics signature for all patients (17). The predictive accuracy of the radiomics signature was evaluated in both sets.

Statistical analysis
All statistical analyses were performed using R (version 3.4.2; The R Foundation for Statistical Computing, Vienna, Austria) with packages including “glmnet”, “pROC”, and “Survminer”. Differences between categorical variables were compared with chi-squared test or Fisher’s exact test. Differences between continuous variables were compared with Student’s t-test or the Mann-Whitney test. The Dice similarity coefficient was used for evaluating the interobserver reproducibility of lesion segmentation. The discrimination ability of the radiomics signature was determined with the receiver operating characteristic (ROC) curve. Univariable and multivariable logistic regression models were created to identify the independent risk factors of HER2 overexpression. A multivariable Cox proportional hazards model with backward-stepwise approach was used to determine the independent predictor of poorer progression-free survival (PFS) and overall survival (OS). The survival predictive ability of the radiomics signature was evaluated by the Harrell concordance index (C-index).
Maximized Youden index was used to find the best cutoff threshold of the R-score for classifying patients into low and high risk of HER2 overexpression. The association of survival and the radiomics signature was assessed using the Kaplan-Meier survival method and log-rank test. Statistical significance was set at 2-sided P<0.05.
Results
Analysis of clinical information
The training set contained 54 (15.1%) HER2+ patients, and the testing set contained 26 (14.5%) HER2+ patients. The positive rate of HER2 in intestinal-type GC was significantly greater than that in diffuse-type GC. No significant differences were shown in the HER2+ and HER2– groups in both sets in terms of age, sex, tumor-node-metastasis (TNM) stage, tumor site, or differentiation status. Of the 80 HER2+ patients, 46 received trastuzumab therapy. The clinicopathological characteristics of all patients are shown in Table 1.
Table 1
Variables | Training set (n=357) | Testing set (n=179) | |||||
---|---|---|---|---|---|---|---|
HER2– | HER2+ | P value | HER2– | HER2+ | P value | ||
Age (years) | 0.64 | 0.32 | |||||
<60 | 162 (53.5) | 27 (50.0) | 78 (51.0) | 16 (61.5) | |||
≥60 | 141 (46.5) | 27 (50.0) | 75 (49.0) | 10 (38.5) | |||
Sex | 0.23 | 0.36 | |||||
Male | 211 (69.6) | 42 (77.8) | 104 (68.0) | 20 (76.9) | |||
Female | 92 (30.4) | 12 (22.2) | 49 (32.0) | 6 (23.1) | |||
Tumor site | 0.43 | 0.94 | |||||
Upper | 93 (30.7) | 19 (35.2) | 56 (36.6) | 9 (34.6) | |||
Middle | 46 (15.2) | 11 (20.4) | 33 (21.6) | 5 (19.2) | |||
Lower | 119 (39.3) | 15 (27.8) | 47 (30.7) | 8 (30.8) | |||
Overlap | 45 (14.8) | 9 (16.7) | 17 (11.1) | 4 (15.4) | |||
Pathologic T stage | 0.80 | 0.88 | |||||
T2 | 73 (24.1) | 15 (27.8) | 29 (19.0) | 6 (23.1) | |||
T3 | 34 (11.2) | 5 (9.3) | 11 (7.2) | 2 (7.7) | |||
T4 | 196 (64.7) | 34 (63.0) | 113 (73.9) | 18 (69.2) | |||
Pathologic N stage | 0.52 | 0.77 | |||||
N0 | 103 (34.0) | 21 (38.9) | 49 (32.0) | 9 (34.6) | |||
N1 | 53 (17.5) | 12 (22.2) | 23 (15.0) | 2 (7.7) | |||
N2 | 64 (21.1) | 11 (20.4) | 31 (20.3) | 5 (19.2) | |||
N3 | 83 (27.4) | 10 (18.5) | 50 (32.7) | 10 (38.5) | |||
Pathologic TNM stage | 0.12 | 0.62 | |||||
I | 39 (12.9) | 5 (9.3) | 13 (8.5) | 3 (11.5) | |||
II | 82 (27.1) | 8 (14.8) | 36 (23.5) | 8 (30.8) | |||
III | 167 (55.1) | 36 (66.7) | 97 (63.4) | 13 (50.0) | |||
IV | 15 (5.0) | 5 (9.3) | 7 (4.6) | 2 (7.7) | |||
Pathologic type | <0.001 | 0.004 | |||||
Intestinal | 35 (11.6) | 18 (33.3) | 19 (12.4) | 9 (34.6) | |||
Diffuse | 268 (88.4) | 36 (66.7) | 134 (87.6) | 17 (65.4) | |||
Differentiation | 0.31 | 0.24 | |||||
Well-moderate | 50 (16.5) | 12 (22.2) | 17 (11.1) | 5 (19.2) | |||
Poor | 253 (83.5) | 42 (77.8) | 136 (88.9) | 21 (80.8) |
Data are expressed as percentage (%) unless otherwise indicated. HER2, human epidermal growth factor receptor 2; TNM, tumor-node-metastasis.
Segmentation reproducibility
The Dice similarity coefficient of interobserver segmentation was 0.92, which indicated a favorable agreement between readers.
Radiomics feature extraction and signature establishment
Of the 2,577 features extracted from the VOIs of the three different phase images of the training set, 1,835 features with intra- and inter-class correlation coefficients <0.85 were excluded. Of the retained 742 features, 83 significantly differentially changed features were identified between the HER2– and HER2+ groups and were brought into the LASSO algorithm. The radiomics signature was constructed based on 8 features with non-zero coefficients (Figure 2), including 3 features from AP, 4 from PP, and 1 from DP. The R-score calculation formula is presented in the supplementary material (Appendix 1). The distribution of the R-scores is shown in Figure S2.
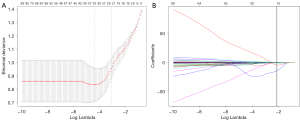
Evaluation of predictive performance of the radiomics signature
The R-scores of the HER2+ patients were significantly higher than those of the HER2– patients in the training (P<0.001, Figure 3A) and testing sets (P<0.001, Figure 3B). The radiomics signature demonstrated favorable predictive ability in both sets with AUCs of 0.85 [95% confidence interval (CI): 0.79–0.92, Figure 3C] and 0.81 (95% CI: 0.72–0.89, Figure 3D), respectively.
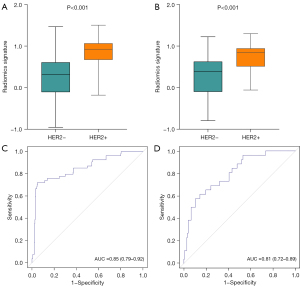
The uni- and multi-variable logistic regression models were used to assess the association of HER2 status with R-score and the clinical parameters in the entire cohort. The radiomics signature and pathological type were demonstrated to be independent predictors of HER2 overexpression (Table 2).
Table 2
Variables | Univariate logistic regression | Multivariate logistic regression | |||
---|---|---|---|---|---|
OR (95% CI) | P value | OR (95% CI) | P value | ||
Sex | 1.54 (0.88–2.70) | 0.13 | |||
Age, years (<60 vs. ≥60) | 0.77 (0.48–1.25) | 0.29 | |||
Tumor site | 0.96 (0.77–1.20) | 0.72 | |||
TNM stage | 1.29 (0.93–1.79) | 0.13 | |||
T stage | 0.93 (0.53–1.62) | 0.79 | |||
N stage | 1.01 (0.51–1.38) | 0.49 | |||
Pathological type | 3.79 (2.20–6.53) | <0.001 | 3.12 (2.01–6.12) | <0.001 | |
Differentiation | 1.57 (0.86–2.84) | 0.14 | |||
Radiomics signature | 7.90 (4.65–13.41) | <0.001 | 6.68 (3.78–15.08) | <0.001 |
HER2, human epidermal growth factor receptor 2; OR, odds ratio; CI, confidence interval; TNM, tumor-node-metastasis.
Prognostic value of radiomics signature for AGC patients
The median follow-up was 16.3 months for the entire cohort. As shown in Table 3, multivariable Cox analysis revealed that higher R-score was an independent predictor for worse PFS [hazard ratio (HR) =1.32, 95% CI: 1.19–2.12, P=0.01] and OS (HR =2.01, 95% CI, 1.38–2.90, P=0.001). Kaplan-Meier curves showed that the high R-score group significantly correlated with poorer OS (12.9 vs. 22.9 months, P<0.001, Figure 4A) and PFS (9.4 vs. 13.8 months, P=0.006, Figure 4B). The C-index was 0.83 (95% CI, 0.72–0.93) and 0.79 (95% CI, 0.63–0.89) for OS and PFS, respectively.
Table 3
Variables | Overall survival | Progression-free survival | |||||||||
---|---|---|---|---|---|---|---|---|---|---|---|
Univariate | Multivariate | Univariate | Multivariate | ||||||||
HR (95% CI) | P value | HR (95% CI) | P value | HR (95% CI) | P value | HR (95% CI) | P value | ||||
Sex | 1.00 (0.71–1.40) | 0.99 | 0.79 (0.67–1.37) | 0.92 | |||||||
Age, years (<60 vs. ≥60) | 0.97 (0.72–1.31) | 0.85 | 0.87 (0.62–1.03) | 0.82 | |||||||
Tumor site | 0.79 (0.66–1.01) | 0.75 | 0.89 (0.56–1.23) | 0.63 | |||||||
Differentiation | 1.13 (0.75–1.69) | 0.57 | 0.76 (0.50–1.15) | 0.20 | |||||||
Pathological type | 0.94 (0.63–1.40) | 0.77 | 1.13 (0.57–1.35) | 0.71 | |||||||
TNM stage | 1.66 (1.25–2.31) | <0.001 | 1.56 (1.22–2.04) | <0.001 | 2.13 (1.13–2.72) | <0.001 | 2.01 (1.32–2.63) | <0.001 | |||
T stage | 1.75 (1.24–2.48) | <0.001 | 1.60 (1.12–2.29) | 0.001 | 1.55 (1.21–1.99) | 0.002 | 1.36 (1.11–1.98) | 0.008 | |||
N stage | 1.53 (1.34–2.32) | <0.001 | 1.24 (1.22–1.83) | 0.005 | 2.04 (1.59–2.73) | <0.001 | 1.73 (1.58–2.17) | <0.001 | |||
Radiomics signature | 2.05 (1.42–2.98) | <0.001 | 2.01 (1.38–2.90) | 0.001 | 1.65 (1.14–2.38) | 0.008 | 1.32 (1.19–2.12) | 0.01 |
HR, hazard ratio; CI, confidence interval; TNM, tumor-node-metastasis.
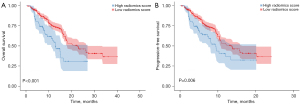
Predictive value of the radiomics signature for trastuzumab treatment efficacy
Of the 46 HER2+ AGC patients who received trastuzumab therapy, disease control was achieved in 39, including CR in 5 patients (10.9%), PR in 23 patients (50.0%), and SD in 11 (23.9%); 7 patients (15.2%) had PD. The disease control rate was 84.8% (39/46). The R-score of the disease control group (CR + PR + SD) was significantly lower than that of the PD group (P=0.023, Figure 5).
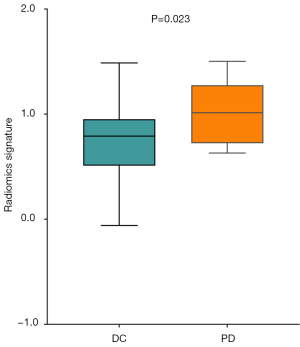
Discussion
In the current study, we developed a multiphase CECT-based radiomics signature as a noninvasive image biomarker of HER2 overexpression in AGC patients. The signature showed accurate discriminatory power in both the training and testing sets. The radiomics signature may help clinicians to detect patients at high risk of false-negative HER2 overexpression due to tumor heterogeneity, and to carefully re-evaluate their HER2 status.
Previous reports have shown that HER2 overexpression correlates with unfavorable survival in GC patients (18,19). Therefore, we additionally assessed the potential contribution of the signature to survival prediction of AGC patients. In line with most of the previous studies (18-20), the R-score was found to be an independent imaging biomarker of worse survival in AGC patients.
The ToGA trial firstly demonstrated that HER2+ AGC patients could benefit from trastuzumab treatment (4). Subsequently, 2 phase II studies also showed that trastuzumab could prolong the OS of Chinese HER2-positive AGC patients (21,22). Based on those results, trastuzumab plus chemotherapy is now the first-line regimen for patients with HER2+ AGC. However, compared with breast cancer, HER2 expression exhibits more heterogeneity in GC (6). Upregulation of HER2 induces cell proliferation, migration, invasion, and angiogenesis, which contribute to the significantly increased heterogeneity in GC (23). Accumulating evidence reveals that increased intratumoral heterogeneity of HER2 expression is associated with poor prognosis (24,25). For GC patients, the heterogeneity of HER2 status not only influences accurate interpretation of HER2 status, but is also associated with the treatment efficacy of anti-HER2 therapy (7,26). Traditional biopsy-based assays may be unsuitable for personalized therapy because the detected gene amplification or mutations does not always reflect the full landscape of tumor cells. Therefore, there is still a need for an effective way of estimating the clinical outcomes of HER2-targeted therapy.
The radiomics approach has been widely applied in the prediction of treatment efficacy in various tumors (27,28). Additionally, a growing body of evidence demonstrates that the radiomics method can quantify intratumoral heterogeneity. High-dimensional radiomics features, which can barely be visualized by the human eye, provide more details about the intratumoral environment such as cell density, hypoxia, microvessel density, and so on (29-31). Moreover, radiomics features extracted from 3D lesions represent the entire landscape of the tumor bulk (32). Previous studies have revealed that textural features such as uniformity and entropy correlate with worse survival of lung, esophageal, and head and neck squamous cell cancer (30,31,33). Recently, Waugh et al. reported that CT textural features such as higher entropy were associated with increased intratumoral heterogeneity in HER2+ breast cancer (34). Yoon et al. assessed the association of CT texture with outcomes of GC patients receiving trastuzumab treatment, but they only investigated the textural features extracted from the largest cross-sectional area of lesions (35). To date, no study has explored the value of the radiomics method for predicting treatment outcomes of anti-HER2 therapy for HER2+ AGC patients. In our study, the analysis of radiomics features was based on the volumetric tumor lesions from all 3 phases of CECT images, which enabled comprehensive characterization of tumor heterogeneity and the complex vascular microenvironment. Our results showed that higher R-score of patients was associated with a lower response rate to trastuzumab therapy. In line with previous reports, we found that entropy was a crucial feature for predicting HER2 status (34). In addition, gray level nonuniformity, which was selected in our signature, was suggested to be an important feature for measuring intratumoral heterogeneity (36). Our results revealed that the radiomics signature might provide insight into tumor heterogeneity and consolidated the ability of our radiomics signature for predicting the efficacy of trastuzumab therapy.
With advances in high-throughput sequencing techniques, several genomic classification systems that reflect the complicated genomic mechanisms underlying GC have been proposed (37,38). The complicated alternation of the signaling pathways induced by HER2 overexpression underlies the treatment efficacy of anti-HER2 therapy (39,40). Thus, further radiogenomics analysis that links radiomics features with the genomic profile is warranted in future research.
This study has some limitations. Firstly, although the radiomics analysis with volumetric features represented the status of the whole tumor bulk, bias may still have been introduced because the test results of the specimens may not reflect the actual status of HER2 expression. Secondly, this retrospective study was conducted in a single center. Thirdly, due to the low positive rate of HER2 in GC in the Chinese population, our study only enrolled 46 patients who had received trastuzumab treatment. Therefore, larger prospective multicenter studies are warranted to assess the generalizability of the radiomics signature.
Conclusions
We established and validated a radiomics signature that showed favorable predictive accuracy of HER2 status and prognosis in AGC patients. In addition, the radiomics signature could be applied as a noninvasive image biomarker for selecting appropriate patients for anti-HER2 target therapy.
Acknowledgments
Funding: The study was supported by
Footnote
Reporting Checklist: The authors have completed the STARD reporting checklist. Available at https://tcr.amegroups.com/article/view/10.21037/tcr-22-1690/rc
Data Sharing Statement: Available at https://tcr.amegroups.com/article/view/10.21037/tcr-22-1690/dss
Conflicts of Interest: All authors have completed the ICMJE uniform disclosure form (available at https://tcr.amegroups.com/article/view/10.21037/tcr-22-1690/coif). The authors have no conflicts of interest to declare.
Ethical Statement: The authors are accountable for all aspects of the work in ensuring that questions related to the accuracy or integrity of any part of the work are appropriately investigated and resolved. The study was conducted in accordance with the Declaration of Helsinki (as revised in 2013). This study was approved by the Institutional Review Board of Tianjin Medical University Cancer Institute and Hospital (ID: ek2020125) and individual consent for this observational, retrospective analysis was waived.
Open Access Statement: This is an Open Access article distributed in accordance with the Creative Commons Attribution-NonCommercial-NoDerivs 4.0 International License (CC BY-NC-ND 4.0), which permits the non-commercial replication and distribution of the article with the strict proviso that no changes or edits are made and the original work is properly cited (including links to both the formal publication through the relevant DOI and the license). See: https://creativecommons.org/licenses/by-nc-nd/4.0/.
References
- Sung H, Ferlay J, Siegel RL, et al. Global Cancer Statistics 2020: GLOBOCAN Estimates of Incidence and Mortality Worldwide for 36 Cancers in 185 Countries. CA Cancer J Clin 2021;71:209-49. [Crossref] [PubMed]
- Wang S, Zhao Y, Song Y, et al. ERBB2D16 Expression in HER2 Positive Gastric Cancer Is Associated With Resistance to Trastuzumab. Front Oncol 2022;12:855308. [Crossref] [PubMed]
- Ivey A, Pratt H, Boone BA. Molecular pathogenesis and emerging targets of gastric adenocarcinoma. J Surg Oncol 2022;125:1079-95. [Crossref] [PubMed]
- Bang YJ, Van Cutsem E, Feyereislova A, et al. Trastuzumab in combination with chemotherapy versus chemotherapy alone for treatment of HER2-positive advanced gastric or gastro-oesophageal junction cancer (ToGA): a phase 3, open-label, randomised controlled trial. Lancet 2010;376:687-97. [Crossref] [PubMed]
- Ajani JA, D'Amico TA, Bentrem DJ, et al. Gastric Cancer, Version 2.2022, NCCN Clinical Practice Guidelines in Oncology. J Natl Compr Canc Netw 2022;20:167-92. [Crossref] [PubMed]
- Nishida Y, Kuwata T, Nitta H, et al. A novel gene-protein assay for evaluating HER2 status in gastric cancer: simultaneous analyses of HER2 protein overexpression and gene amplification reveal intratumoral heterogeneity. Gastric Cancer 2015;18:458-66. [Crossref] [PubMed]
- Wakatsuki T, Yamamoto N, Sano T, et al. Clinical impact of intratumoral HER2 heterogeneity on trastuzumab efficacy in patients with HER2-positive gastric cancer. J Gastroenterol 2018;53:1186-95. [Crossref] [PubMed]
- Qin Y, Deng Y, Jiang H, et al. Artificial Intelligence in the Imaging of Gastric Cancer: Current Applications and Future Direction. Front Oncol 2021;11:631686. [Crossref] [PubMed]
- Song R, Cui Y, Ren J, et al. CT-based radiomics analysis in the prediction of response to neoadjuvant chemotherapy in locally advanced gastric cancer: A dual-center study. Radiother Oncol 2022;171:155-63. [Crossref] [PubMed]
- Chen T, Wu J, Cui C, et al. CT-based radiomics nomograms for preoperative prediction of diffuse-type and signet ring cell gastric cancer: a multicenter development and validation cohort. J Transl Med 2022;20:38. [Crossref] [PubMed]
- Zhang G, Deng L, Zhang J, et al. Development of a Nomogram Based on 3D CT Radiomics Signature to Predict the Mutation Status of EGFR Molecular Subtypes in Lung Adenocarcinoma: A Multicenter Study. Front Oncol 2022;12:889293. [Crossref] [PubMed]
- Cui Y, Liu H, Ren J, et al. Development and validation of a MRI-based radiomics signature for prediction of KRAS mutation in rectal cancer. Eur Radiol 2020;30:1948-58. [Crossref] [PubMed]
- Wang Y, Yu Y, Han W, et al. CT Radiomics for Distinction of Human Epidermal Growth Factor Receptor 2 Negative Gastric Cancer. Acad Radiol 2021;28:e86-92. [Crossref] [PubMed]
- American Joint Committee on Cancer. AJCC cancer staging manual, 8th ed. New York: Springer, 2010.
- Eisenhauer EA, Therasse P, Bogaerts J, et al. New response evaluation criteria in solid tumours: revised RECIST guideline (version 1.1). Eur J Cancer 2009;45:228-47. [Crossref] [PubMed]
- Gao X, Ma T, Cui J, et al. A CT-based Radiomics Model for Prediction of Lymph Node Metastasis in Early Stage Gastric Cancer. Acad Radiol 2021;28:e155-64. [Crossref] [PubMed]
- Zha X, Liu Y, Ping X, et al. A Nomogram Combined Radiomics and Clinical Features as Imaging Biomarkers for Prediction of Visceral Pleural Invasion in Lung Adenocarcinoma. Front Oncol 2022;12:876264. [Crossref] [PubMed]
- Wang HB, Liao XF, Zhang J. Clinicopathological factors associated with HER2-positive gastric cancer: A meta-analysis. Medicine (Baltimore) 2017;96:e8437. [Crossref] [PubMed]
- Lei YY, Huang JY, Zhao QR, et al. The clinicopathological parameters and prognostic significance of HER2 expression in gastric cancer patients: a meta-analysis of literature. World J Surg Oncol 2017;15:68. [Crossref] [PubMed]
- Jørgensen JT, Hersom M. HER2 as a Prognostic Marker in Gastric Cancer - A Systematic Analysis of Data from the Literature. J Cancer 2012;3:137-44. [Crossref] [PubMed]
- Gong J, Liu T, Fan Q, et al. Optimal regimen of trastuzumab in combination with oxaliplatin/ capecitabine in first-line treatment of HER2-positive advanced gastric cancer (CGOG1001): a multicenter, phase II trial. BMC Cancer 2016;16:68. [Crossref] [PubMed]
- Qiu MZ, Li Q, Wang ZQ, et al. HER2-positive patients receiving trastuzumab treatment have a comparable prognosis with HER2-negative advanced gastric cancer patients: a prospective cohort observation. Int J Cancer 2014;134:2468-77. [Crossref] [PubMed]
- Ciesielski M, Szajewski M, Pęksa R, et al. The relationship between HER2 overexpression and angiogenesis in gastric cancer. Medicine (Baltimore) 2018;97:e12854. [Crossref] [PubMed]
- Lee HE, Park KU, Yoo SB, et al. Clinical significance of intratumoral HER2 heterogeneity in gastric cancer. Eur J Cancer 2013;49:1448-57. [Crossref] [PubMed]
- Motoshima S, Yonemoto K, Kamei H, et al. Prognostic implications of HER2 heterogeneity in gastric cancer. Oncotarget 2018;9:9262-72. [Crossref] [PubMed]
- Yagi S, Wakatsuki T, Yamamoto N, et al. Clinical significance of intratumoral HER2 heterogeneity on trastuzumab efficacy using endoscopic biopsy specimens in patients with advanced HER2 positive gastric cancer. Gastric Cancer 2019;22:518-25. [Crossref] [PubMed]
- Liu Z, Li Z, Qu J, et al. Radiomics of Multiparametric MRI for Pretreatment Prediction of Pathologic Complete Response to Neoadjuvant Chemotherapy in Breast Cancer: A Multicenter Study. Clin Cancer Res 2019;25:3538-47. [Crossref] [PubMed]
- Mattonen SA, Davidzon GA, Benson J, et al. Bone Marrow and Tumor Radiomics at 18F-FDG PET/CT: Impact on Outcome Prediction in Non-Small Cell Lung Cancer. Radiology 2019;293:451-9. [Crossref] [PubMed]
- Ganeshan B, Miles KA. Quantifying tumour heterogeneity with CT. Cancer Imaging 2013;13:140-9. [Crossref] [PubMed]
- Ganeshan B, Panayiotou E, Burnand K, et al. Tumour heterogeneity in non-small cell lung carcinoma assessed by CT texture analysis: a potential marker of survival. Eur Radiol 2012;22:796-802. [Crossref] [PubMed]
- Zhang H, Graham CM, Elci O, et al. Locally advanced squamous cell carcinoma of the head and neck: CT texture and histogram analysis allow independent prediction of overall survival in patients treated with induction chemotherapy. Radiology 2013;269:801-9. [Crossref] [PubMed]
- Mei D, Luo Y, Wang Y, et al. CT texture analysis of lung adenocarcinoma: can Radiomic features be surrogate biomarkers for EGFR mutation statuses. Cancer Imaging 2018;18:52. [Crossref] [PubMed]
- Ganeshan B, Skogen K, Pressney I, et al. Tumour heterogeneity in oesophageal cancer assessed by CT texture analysis: preliminary evidence of an association with tumour metabolism, stage, and survival. Clin Radiol 2012;67:157-64. [Crossref] [PubMed]
- Waugh SA, Purdie CA, Jordan LB, et al. Magnetic resonance imaging texture analysis classification of primary breast cancer. Eur Radiol 2016;26:322-30. [Crossref] [PubMed]
- Yoon SH, Kim YH, Lee YJ, et al. Tumor Heterogeneity in Human Epidermal Growth Factor Receptor 2 (HER2)-Positive Advanced Gastric Cancer Assessed by CT Texture Analysis: Association with Survival after Trastuzumab Treatment. PLoS One 2016;11:e0161278. [Crossref] [PubMed]
- Aerts HJ, Velazquez ER, Leijenaar RT, et al. Decoding tumour phenotype by noninvasive imaging using a quantitative radiomics approach. Nat Commun 2014;5:4006. [Crossref] [PubMed]
- Cancer Genome Atlas Research Network. Comprehensive molecular characterization of gastric adenocarcinoma. Nature 2014;513:202-9. [Crossref] [PubMed]
- Cristescu R, Lee J, Nebozhyn M, et al. Molecular analysis of gastric cancer identifies subtypes associated with distinct clinical outcomes. Nat Med 2015;21:449-56. [Crossref] [PubMed]
- Blangé D, Stroes CI, Derks S, et al. Resistance mechanisms to HER2-targeted therapy in gastroesophageal adenocarcinoma: A systematic review. Cancer Treat Rev 2022;108:102418. [Crossref] [PubMed]
- Chen Y, Ye B, Wang C, et al. PLOD3 contributes to HER-2 therapy resistance in gastric cancer through FoxO3/Survivin pathway. Cell Death Discov 2022;8:321. [Crossref] [PubMed]
(English Language Editors: K. Brown and J. Gray)